the Creative Commons Attribution 4.0 License.
the Creative Commons Attribution 4.0 License.
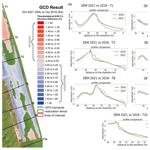
Dune belt restoration effectiveness assessed by UAV topographic surveys (northern Adriatic coast, Italy)
Regine Anne Faelga
Luigi Cantelli
Sonia Silvestri
Beatrice Maria Sole Giambastiani
Unoccupied aerial vehicle (UAV) monitoring surveys are used to assess a dune restoration project in the protected natural area of the Bevano River mouth on the northern Adriatic coast (Ravenna, Italy). The impacts of the installed fences to aid dune development are quantified in terms of sand volume and vegetation cover changes over 5 years using a systematic data processing workflow based on structure-from-motion (SfM) photogrammetry and the Geomorphic Change Detection (GCD) toolset applied to two drone surveys in 2016 and 2021. Accuracy assessment is performed using statistical analysis between ground-truth and model elevation data. Results show that the fence proves to be effective in promoting recovery and growth since significant sand deposition was observed along the dune foot and front – a total area of 3799 m2, volume of 1109 m3, and average depositional depth of 0.29 m. Progradation of around 3–5 m of the foredune and embryo development were also evident. There was a decrease in blowout features of about 155 m2 due to increased deposition and vegetation colonization. There was also an average percent increase of 160 % on wave-induced driftwood and/or debris along the beach and of 9.6 % vegetation within the fence based on the cover analysis on selected transects. Erosion of around 1439 m2 is apparent mostly at the northern portion of the structure, which could be accounted for by the aerodynamic and morphodynamic conditions around the fence and its configuration to trap sediments and efficiency in doing so. Overall, dune fencing coupled with limiting debris cleaning along the protected coast was effective. The proposed workflow can aid in creating transferable guidelines to stakeholders in integrated coastal zone management (ICZM) implementation on Mediterranean low-lying sandy coasts.
- Article
(8452 KB) - Full-text XML
- BibTeX
- EndNote
Coastal dunes are significant ecosystems that can provide flood protection, groundwater storage, salinization prevention, species habitat, and recreation. Their dynamics are driven by the complex interaction between the controlling winds, vegetation, and the nearshore-beach geomorphology (Sloss et al., 2012; Yousefi Lalimi et al., 2017). Their highly dynamic nature, in addition to climatic and anthropogenic pressures, makes these landforms extremely vulnerable. To prevent further degradation, soft or limited engineering, along with nature-based solutions (NBSs), have been the preferred intervention strategies for coastal zones as they enable more dynamic evolution and functioning. In Europe, coastal foredunes have been stabilized over the past century by reprofiling, planting vegetation and dune fencing, and/or beach nourishment (Nordstrom and Arens, 1998; Arens et al., 2001; Matias et al., 2005; Ruz and Anthony, 2008; De Vriend and Van Koningsveld, 2012; Laporte-Fauret et al., 2021).
Surface topography characterization using high-resolution data and remote sensing such as terrestrial laser scanning (TLS), light detection and ranging (lidar), and unoccupied aerial vehicles (UAVs) has led to the development of quantitative methods used for coastal monitoring purposes (Kasprak et al., 2019). Among these, UAV platforms have gained more traction due to their affordability and user-friendly interfaces compared to other surveying counterparts. The advances in the use of UAV and structure-from-motion (SfM) photogrammetry have made geomorphic change monitoring and sediment budget estimations become manageable approaches in research and practice (Wheaton et al., 2009b). SfM photogrammetry utilizes a structured acquisition of images to reconstruct 3D scene geometry and camera motion based on a new generation of automated image-matching algorithms (Mancini et al., 2013). These images can be used to create digital elevation models (DEMs) to produce DEM-of-difference (DoD) maps to estimate the net change in storage for morphological sediment budgets (Church and Ashmore, 1998; Wheaton et al., 2009b).
The use of UAV–SfM in monitoring seasonal coastal changes along the Emilia-Romagna coast has been evident in the works of Taramelli et al. (2015), Scarelli et al. (2017), Fernández-Montblanc et al. (2020), Sekovski et al. (2020), and Fabbri et al. (2021). These studies have noted that accuracy assessment of the surface and elevation models from UAV–SfM is important before performing further analysis. Understanding the effect of elevation data uncertainty was also highlighted in the recent work of Duo et al. (2021), where UAV-derived data were utilized for the morphodynamics study of a scraped artificial dune beach in Comacchio using change detection. The availability of UAV–SfM tools and methods for coastal applications can be further utilized in other highly dynamic areas like the Ravenna coastline and its remaining dunes.
Study site information
The dunes along the Ravenna coast (northern Adriatic Sea, Italy; Fig. 2) have been subjected to degradation due to combined natural, anthropogenic, and climate-induced pressures. Ravenna is a historical city, known for its beach tourism and for having one of the largest seaports in Italy. It is part of the 130 km coastline of Emilia-Romagna that is made up of a flat, alluvial, sandy system, with a gently sloping seabed of about 6 m in depth and shallow subtidal sediments from well-sorted fine to medium sand (Airoldi et al., 2016; Harley et al., 2016). The local hydrodynamic conditions include exposure to moderate wave action and a microtidal regime that ranges between 30 and 80 cm between neap and spring tides (Biolchi et al., 2022). Two wind patterns characterize the region – the bora wind from the northeast that brings shorter but energetic waves (dominant wind) and the longwave induced by levant and sirocco (prevailing winds) from the east and southeast, respectively. The wave climate and current circulation in the northern Adriatic are known to be strongly influenced by the bora wind given the coast orientation.
According to the 2016–2020 meteo-marine data from the Hydro-Meteo-Climate Report of the Regional Agency for Prevention, Environment and Energy of the Emilia-Romagna region (Arpae, 2020a), the majority of the stronger waves (0.2 to 4 m) are from the NE and ENE (Fig. 1). Waves blown from the eastern side are more frequent from 2016 to 2020 but are relatively weak (0.2 to 2.5 m). The number of storm surges per year ranges from 17 to 24, with an average duration of 12.8 to 27.9 h. Wind and wave data are recorded from the wave buoy every 30 min and are then archived to the Arpae service database that can be accessed online through Dext3r (https://simc.arpae.it/dext3r/, last access: 10 February 2023). Historical records of the storm surge characteristics from the 2007–2020 observations are summarized in Table 1.
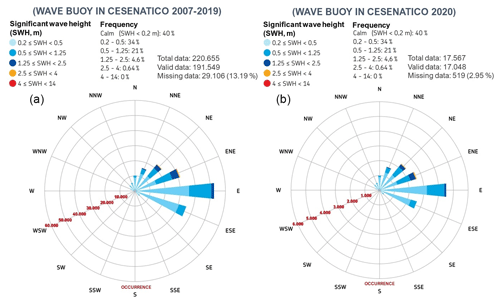
Figure 1Significant wave height (SWH in m) and frequency (%) for 2007–2019 (a) and 2020 (b) extracted from the Arpae 2020 report.
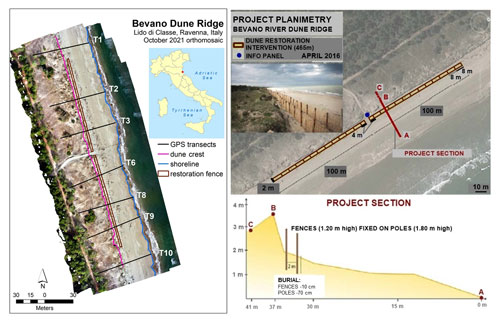
Figure 2Location of the study area in Ravenna (Italy) and the dune fence project planimetry (modified from Giambastiani et al., 2016). Points A, B, and C represent areas in the back dune, foredune, and beach along a section of the project.
Table 1Storm surge characteristics from 2016 to 2020 extracted from the Arpae database. SL denotes sea level.
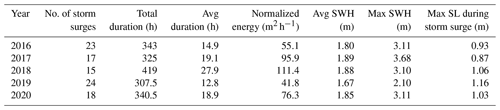
Significant land subsidence due to tectonic processes and sediment consolidation has been widespread and has intensified due to human activity since the second half of the 20th century (Airoldi et al., 2016). Erosive processes have also affected 105 km out of the 130 km coastline of the region during this period – along with the increase in vulnerability to storm surge, rapid coastal urbanization, the implementation of rigid coastal defenses, and massive dune destruction – igniting the need to implement strategic interventions to mitigate these problems (Arpae, 2020a). Hard coastal defenses (submerged and emerged breakwaters, groynes, and revetments) were constructed in the early years (Armaroli et al., 2019; Perini et al., 2017). However, these infrastructures had negative environmental impacts including increased sedimentation of silts and clay, loss of native habitats, eutrophication, and poor water quality (Airoldi et al., 2016; Preti and Zanuttigh, 2011; Sekovski et al., 2020). The progressive transition from hard coastal defenses in the 1970s to more integrated approaches with soft techniques and NBSs in recent years has been implemented in the region. NBSs and soft-engineering techniques such as beach nourishment and dune fence installation were eventually initiated as alternative solutions in the early 2000s. Collaborations between the regional environmental agency Arpae, research groups, and other regional services that deal with coastal management led to the collection of important databases that aided the implementation of several policies to address the impending issues along the Emilia-Romagna coast.
In 2016, the RIGED-RA Project – “Restoration and management of coastal dunes along the Ravenna coast” – was able to install a grid of windbreak fences that stretches across 465 m along a portion of the Bevano River dune ridge in Lido di Classe (Fig. 2) as an intervention strategy to reduce the vulnerability of the coast and the associated residual dunes in the area (Giambastiani et al., 2016). The selection of the most suitable NBS intervention was done after a geographic, environmental, lithological, hydrogeological, geomorphological, and hydrodynamical characterization of the study area collected during the 3-year project. The Bevano dune–beach system is a protected natural area with high biodiversity, with laterally continuous and sub-vertical foredunes. According to Giambastiani et al. (2016), the area was selected as the pilot site given that it has the potential for dune accumulation but has limited beach width and unstable sub-vertical foredune geometry. Blowout patches are also evident along the frontal dune area of the study site. The installed fences are called ganivelles – made up of highly resistant chestnut wood stakes and poles about 1.20 and 1.80 m in height, whose purpose is to block the wind loaded with sand and consequently favor its accumulation to recreate the dune. The spacing between stakes was set to 10 cm. The first fence was placed at the dune foot followed by the second fence 2 m seawards. The two fences were connected perpendicularly by 8 m fence portions. No planting activities were implemented due to the high presence of native sand-binding vegetation species such as psammophilous species. The fence configuration was in accordance with the mathematical model developed by Hanley et al. (2014) that illustrates the relationship between brushwood fence size and position and optimum sand accumulation and is applicable to microtidal environments.
The availability of repeated UAV topographic surveys after the fence installation in 2016 and the availability of open-source tools can address the gaps in quantifying the restoration efficacy. The study aims to provide aid for making informed decisions from quantitative data analysis with the proposed workflow for UAV data processing and elevation data analysis suited for sediment volume calculation. Error analysis was performed to validate the change detection results produced. Vegetation cover change using orthomosaic images derived from UAV was also explored to determine other contributing factors to the overall morphology of the dune ridge.
The methodological framework (Fig. 3) includes established workflows for data acquisition, geomorphology modeling, vegetation change, and geomorphic change detection. Annual monitoring campaigns were carried out after the fence installation in 2016. In this paper, only the first (October 2016) and last (October 2021) UAV and GPS surveys were selected to assess the dune evolution. GPS data points were collected using a Leica differential global positioning system (DGPS; Viva GNSS GS15 GPS) that worked with a real-time kinematic (RTK) system to ensure sub-metric accuracy. Collected data points include several profiles across the beach from the coastline to the back dune with a measuring interval of 1 m. Aerial photographs for the 3D reconstruction were captured using a DJI Phantom drone, with flight plans defined and executed in PIX4DCapture. The flying height used in both surveys are 20 m, with side and front overlap of 80 %. Ground control points (GCPs) were established on site and were geolocated using GPS to georeference the images and 3D models during the SfM processing in Agisoft Metashape Professional. The datum used for the flight plan was WGS 84, and the coordinate system was set to UTM 32N.
For the SfM processing, multiple overlapping photos were loaded and initial image filtering was performed before the alignment process. The two datasets have a total of 13 and 15 GCPs, respectively. Each GCP was assigned as either a control point or a checkpoint – the former is used to reference the model, while the latter is used to validate the camera alignment accuracy and optimization procedure results (Agisoft LLC, 2021). The 2016 data have 9 control points and 4 checkpoints, while the 2021 data have 10 and 5. After the alignment process, a dense point cloud was created and filtered to remove low-confidence points (0 %–5 %).
The dense-point-cloud data were then classified into ground and non-ground using the automatic ground point classification tool to remove vegetation points. The classified points were used as the boundary condition for creating the RGB orthomosaic with spatial resolution of 0.1 m×0.1 m. Ground points were imported and converted into raster in ArcMap 10.8.2 to create the DEMs for the change detection analysis. A concurrency shapefile and spatial resolution of 0.1 m×0.1 m were used in creating the elevation models to ensure coherence and comparability. The horizontal accuracy of the resulting DEMs was assessed using the calculated root-mean-square error (RMSE) of the checkpoints, while the vertical accuracy was evaluated by comparing the DEM values extracted using QGIS Profile Tool plugin version 4.2.0 to the GPS point values. The computed DEM points and the measured GPS points along each transect were visualized by a scatterplot in Python 3.9. Statistical measures include R2, RMSE, the mean absolute error (MAE), and the mean bias error (MBE).
The morphological assessment was implemented using the Geomorphic Change Detection (GCD) 7 AddIn tool in ArcMap. This tool was developed by the Riverscapes Consortium using the methodology of Wheaton (2008) and Wheaton et al. (2009a, b). It can compute for the extent, magnitude, and landscape form changes that occur within an inter-survey period to understand the overall sediment budget of the area of interest (AOI) or the spatial distribution changes of sediments through time (Grams et al., 2015; Collins et al., 2016; Sankey et al., 2016). In this study, the AOI included the foredune to beach area. Error rasters were created using the reported total RMSE values of the control points, which were 0.046 and 0.032 m, respectively. These values were used to calculate the propagated error applied to each cell (Eq. 1) and the t statistics (Eq. 2), adapting the following equations from Lane et al. (2003):
where σc is the root sum of square of uncertainty for each change interval [m]; and are the squares of uncertainty for the older and newer time steps [m];
where t is the t statistics and zt2 and zt1 are the elevation of the raster cell for the newer (t2) an older (t1) time step [m]. The t statistics can be used as a thresholding level of significant change, where values of t are more than 1.96 with a mean confidence interval of 95 % for a two-tailed t test. Values that fell below the confidence threshold were removed from the output change raster to improve the likelihood that a significant change was captured (Hilgendorf et al., 2021). The percent sediment imbalance metric SI was also calculated to characterize the sediment dynamics using Eq. (3) (Wheaton et al., 2013; Kasprak et al., 2015, 2019):
where VDEP is the volume of deposition and VEROS is the volume of erosion [m3].
The vegetation change analysis was performed based on a statistical approach similar to that of Silvestri et al. (2022), with some modifications applied. The process includes shoreline delineation using ISO cluster unsupervised classification in ArcMap, transect creation based on GPS profiles, and gridding and centroid creation at 1 m×1 m resolution using the transects as boundary conditions. The method applied to detect the presence and/or absence of vegetation is based on the visual inspection of the centroids of the grid cells. If the centroid falls on bare soil, there is an absence of vegetation. Consequently, if it falls on a vegetated pixel of the photo, there is a presence of vegetation. As the orthophotos have a resolution of 0.1 m×0.1 m, a 1 m×1 m grid resolution allows us to sample 1 pixel (corresponding to the centroid) every 100 pixels included in each grid cell. This method is similar to a classic visual ecological survey performed in the field with 0.1 m×0.1 m plots placed at a distance of 1 m from each other along a transect, but in this case it is performed on an orthophoto instead, with the assumption that the operator has a clear overview of the area and can clearly distinguish between vegetated and non-vegetated (with either bare sand or logs and/or debris) pixels. The accuracy of the method therefore depends on the ability of the operator as well as on the image quality. Percentage calculation of the cover types present in each transect was performed to quantify the change. The assessment of blowout features was also performed by visual inspection of the 2016 and 2021 orthomosaic images.
3.1 DEM development and validation
The DEMs, at 0.1 m×0.1 m resolution, resulting from the UAV surveys in 2016 and 2021 are shown in Fig. 4. There is an elevation range of −0.37 to 6.30 m, with the minimum and maximum values observed along the beach area and the back dunes. Validation on the transects (Fig. 4b) was performed using linear regression shown in Fig. 5. Only the 2021 survey was validated for the vertical accuracy since there are no ground-truth GPS profiles available for the 2016 dataset. The R2 values range from 0.97 to 1, while the RMSE values range from 0.07 to 0.15 m. The MAE and error bias values range from 0.06 to 0.10 and −0.01 to 0.05 m, respectively. Elevation difference at the back dune area was observed in transects 1, 3, 6, and 8. There is also variation observed from the dune crest and foot in transects 2, 8, and 9. Overall, the GPS data and model comparison for 2021 have a good fit in all the sample profiles. In terms of horizontal accuracy, the reported RMSEs(x,y) of the 2016 and 2021 checkpoints are 0.020 and 0.022 m.
3.2 Geomorphic and vegetation cover changes
The change detection results of the 2021 vs. 2016 DEMs are shown in Figs. 6 and 7. Considering the total area of 9154 m2, 6020 m2 (66 % of the AOI) had detectable changes after applying the 95 % confidence interval (CI) threshold; 2221 m2 had erosional change that is equivalent to 584 m3 in volume and 0.26 m in average depth. On the other hand, 3799 m2 had depositional change that corresponds to 1109 m3 and 0.29 m. A net rise of 1692 m3 has been detected, with a net volume difference of +525 m3. The average total thickness of difference is 0.18 m, with a net thickness difference of 0.06 m. Looking at the DEM profile comparison (Fig. 6b), embryo dune formation is apparent in transects 3, 6, 8, 9, and 10. There is also a noticeable variation in elevation values in T1; nonetheless, deposition along the dune foot is still noticeable. Overall, deposition was mostly observed within the fence and the beach at the central part of the AOI, while erosion was mostly at the northern part of the structure and at the beach part of T10. A tabular summary of the GCD is included in the Appendix.
Orthomosaic images from 2016 and 2021 were used in the vegetation cover change assessment (Fig. 8). Considering the average percent change of all the transects, there was an overall increase in the cover extent of vegetation (9.6 %) and areas with logs or debris (160 %) and, consequently, an obvious decrease in the bare-sand extent (26 %). The highest positive percent changes for vegetation increase were in transects 2, 3, 8, and 9. An increase in logs and debris was more evident in transects 2, 3, 6, 8, and 9 (Fig. 9). There is also an evident decrease in blowout features on some transects located along the fence close to the dune foot, which has a total area of 155 m2. In Fig. 10, the blowout patterns close to transects 2, 3, and 8 have been covered by sand and vegetation over time and are comparable to the deposition patterns in Fig. 6.
4.1 Change detection – geomorphic and vegetation cover
Within the littoral cell where the study site is located, sand accumulation and shoreline advancement by 15–20 m were observed in 2018 in comparison to the 2012 baseline data (Arpae, 2020b). The 2018 report on the coastal state of Emilia-Romagna mentioned defense intervention as one of the factors that could have influenced this change. The result of the 2021 vs. 2016 GCD further establishes the efficacy of the dune fencing since significant deposition – in terms of area, average depth, and volume along the dune foot and a portion of the beach – was evident. Progradation of around 3 to 5 m from the foredune is apparent, and some profiles exhibit embryo development (Fig. 6b; profiles 3, 6, 8, and 9). Profiles with significant deposition were the ones located at the middle and the southern part of the AOI. Embryo foredunes are formed due to sand deposition within clumps of vegetation, individual plants, or driftwood and/or log debris (Hesp, 2002; van Puijenbroek et al., 2017). An increase in vegetation and wave-transported driftwood has been observed within and near the fence (Fig. 8). Most of the vegetation change appeared in between the fences as pioneer species colonized the pillow sand deposits. No vegetation change on the more stabilized back dune was observed. The increase in vegetation colonization contributed to the stabilization of sand accumulation within the dunes over 5 years. The result is comparable to the study of van Puijenbroek et al. (2016) regarding the effect of vegetation on embryo dune development in the Netherlands. Tolerant vegetation facilitates sand deposition and aids in increasing the stabilization and growth of dune systems (Laporte-Fauret et al., 2021; Wootton et al., 2016). The result is also in agreement with the published work of Dong et al. (2008) and Hesp (2002), where it was mentioned that the establishment of vegetation on bare sand or beach forms a roughness element that may allow localized sand deposition and reduced erosion.
An erosional pattern is apparent in the northern beach portion towards the northern head of the structure (Fig. 6a), which may be accounted for by the aerodynamic and morphodynamic conditions around the dune fence, the efficiency of the fence, and its configuration to trap sediments. The effects of sand-trapping fences are primarily determined by their geometry, orientation relative to the main wind direction, aerodynamic roughness of the wind profile, undisturbed flow, and shelter distance (Eichmanns et al., 2021). Their influence is dependent on the given sediment boundary conditions and the wind field. In this case, the northern beach portion and the fence peripheries have less debris and vegetation change, which could have caused the erosion along the beach.
No significant increase in the foredune heights is evident in all the profiles (Fig. 6b). Similar findings have been observed in the study of Itzkin et al. (2020), where new embryo dunes were created seawards of the original dune following the emplacement of sand fences, but this impeded additional sediment being received by the natural foredune. Hence, dune fencing may not always be the best singular management action as it can also prevent deposition to the natural dune behind the fence that could limit vertical growth.
Human disturbances by mass tourism and coastal urbanization have a detrimental impact on pioneer vegetation species and can prejudice the sustainability of foredune restoration especially in the Mediterranean (Della Bella et al., 2021). The fence has halted human trampling on dune vegetation that had limited the formation of erosional features such as blowouts. The enforcement of limited human disturbance and the fact that the location is part of a protected area supported the restoration efficacy and the spontaneous recovery of the dunes. Compared to beaches that are mechanically cleaned for recreational purposes, driftwood that was not removed in this area acted as a form of passive restoration as well. These soft-engineering approaches and NBSs were able to induce sand accretion and vegetation colonization that can be considered ecologically sustainable, technologically feasible, and economically viable. The fence configuration used was overall effective in trapping sediments along the dune foot and within its central bounds. However, the possibility of the fences being washed away or degraded over time due to storm impacts should still be considered; hence, continuous monitoring and maintenance must be ensured to guarantee long-term efficiency. Given the results, dune fencing and limiting debris cleaning can also be implemented along other coastal zones of Emilia-Romagna and other low-lying sandy coasts as they can aid the sediment exchange system over time since both sediment contribution from the nearshore to the dunes and accretion rates in the foredune are vital in a beach–dune system. Sand reservoirs and driftwood within and surrounding the fence can act as barriers to dissipate energy further offshore in case of major storm surge events.
4.2 Error analysis
The accuracy of the change detection model heavily depends on the quality of the input DEMs. Precision issues with the SfM-derived DEM and GPS have been encountered due to target data quality and systemic issues. The model fitting statistics of the 2021 dataset show a good fit within 0.96 to 0.99. However, a slight shift in values in the back dune is evident, which can be attributed to human error during the GPS survey as the pole can be dragged a few centimeters from the ground. Possible variance between beach surfaces, systematic collection inconsistencies related to survey setup, and susceptibility to external factors such as possible digging around the pole and wind speed influence may be encountered in beach environment surveys (Talavera et al., 2018; Casella et al., 2020; Hilgendorf et al., 2021). Misclassification of vegetation as ground points may have also affected the accuracy of the surface reconstruction of the DEMs. Drone-based topographic reconstructions of beach environments tend to exhibit higher inaccuracies compared to other environments such as outcrops due to the low texture and contrast of sand surfaces, making photogrammetric methods, such as feature matching, difficult (Eltner et al., 2016; Casella et al., 2020). Another probable source of error is the lack of validation information for the vertical accuracy of the 2016 data. The GCP configurations and the number used may have also contributed to the overall model error.
Notwithstanding this, the results show that assessing the spatio-temporal evolution of the erosional and depositional processes on the Bevano dune ridge is possible using multi-temporal drone data. Elevation model accuracy on the order of ∼5 to 8 cm has been achieved. The results of the study may be further improved by ensuring consistency in camera and build parameters for the elevation and change detection models. Classifying the area of interest into geomorphic units can also be done to enhance the geomorphic change detection result.
A dune restoration project on the northern Adriatic coast (Ravenna, Italy) was assessed using UAV monitoring surveys. SfM photogrammetry, elevation differencing, and statistical analysis were utilized to quantify dune development in terms of sand volume and vegetation cover change over time.
Despite the natural factors affecting the overall deposition dynamics in the area, results show that dune fencing proved to be an effective intervention to prevent dune erosion since significant geomorphological changes and vegetation colonization occurred in the 2016–2021 interval time. The main sand accumulation was observed along the dune foot where the wood fences were established. The following changes have also been observed: progradation of the front dune, development of embryo dunes, decrease in blowout features on the frontal dune area due to increase in vegetation colonization, and increase in vegetation and debris cover within and near the fences.
GCD can be an effective monitoring tool for coastal dunes for as long as the sources of uncertainties are considered. The results of the study can supplement the showcasing of the importance of implementing dune fencing and limiting debris cleaning as nature-based solutions in preventing dune degradation along the coastal zones of Emilia-Romagna. The proposed systematic workflow developed within this research can be transferred to other similar coastal zones and implemented into guidelines for integrated coastal zone management (ICZM).
The Python code used for the validation process can be accessed at Zenodo (Faelga et al., 2023, https://doi.org/10.5281/zenodo.10253228). The Geomorphic Change Detection (GCD) 7 AddIn for ArcGIS 10.x versions can be accessed through Bailey et al. (2020, https://gcd.riverscapes.net/Download/, last access: 8 December 2021).
The dataset used for the validation and vegetation cover analysis can be accessed at Zenodo (Faelga et al., 2023, https://doi.org/10.5281/zenodo.10253228)
Conception and study design: RAF, BMSG; data collection: RAF, BMSG, LC; data analysis and interpretation: RAF, BMSG, LC, SS; article drafting: RAF; critical revision of the article: RAF, BMSG, SS; final approval of the version to be published: BMSG, SS, LC.
At least one of the (co-)authors is a guest member of the editorial board of Biogeosciences for the special issue “Monitoring coastal wetlands and the seashore with a multi-sensor approach”. The peer-review process was guided by an independent editor, and the authors also have no other competing interests to declare.
Publisher's note: Copernicus Publications remains neutral with regard to jurisdictional claims made in the text, published maps, institutional affiliations, or any other geographical representation in this paper. While Copernicus Publications makes every effort to include appropriate place names, the final responsibility lies with the authors.
This article is part of the special issue “Monitoring coastal wetlands and the seashore with a multi-sensor approach”. It is not associated with a conference.
The first author would like to thank the Erasmus Mundus Joint Master Degree (EMJMD) program in Water and Coastal Management (WACOMA) (University of Bologna – Italy, University of Cadiz – Spain, and University of Algarve – Portugal) for the scholarship provided as part of the 2020–2022 cohort. The authors would like to thank the Carabinieri for Biodiversity, which allowed the access to the protected natural areas that was crucial for this research. Finally, the authors would also like to thank the anonymous reviewers for their valuable comments.
Regine Anne Faelga and collaboration among the authors were supported by the European Commission under the Erasmus Mundus Joint Master Degree program in Water and Coastal Management (grant no. 586596-EPP-1-2017-1-ITEPPKA1-JMDMOB). This study was also carried out within the RETURN Extended Partnership and received funding from the European Union NextGenerationEU (National Recovery and Resilience Plan – NRRP, Mission 4, Component 2, Investment 1.3 – D.D. 1243 2/8/2022, PE0000005).
This paper was edited by Manudeo Singh and reviewed by three anonymous referees.
Agisoft LLC: Agisoft Metashape User Manual: Professional Edition, Version 1.7, https://www.agisoft.com/pdf/metashape-pro_1_7_en.pdf (last access: 8 September 2021), 2021.
Airoldi, L., Ponti, M., and Abbiati, M.: Conservation challenges in human dominated seascapes: The harbour and coast of Ravenna, Regional Studies in Marine Science, 8, 308–318, https://doi.org/10.1016/j.rsma.2015.11.003, 2016.
Arens, S. M., Baas, A. C. W., Van Boxel, J. H., and Kaleman, C.: Influence of reed stem density on foredune development, Earth Surf. Proc. Land., 26, 1161–1176, https://doi.org/10.1002/esp.257, 2001.
Armaroli, C., Duo, E., and Viavattene, C.: From Hazard to Consequences: Evaluation of Direct and Indirect Impacts of Flooding Along the Emilia-Romagna Coastline, Italy, Front. Earth Sci., 7, 203, https://doi.org/10.3389/feart.2019.00203, 2019.
Arpae Emilia-Romagna: Rapporto IdroMeteoClima Emilia-Romagna: Dati 2020, https://www.arpae.it/it/temi-ambientali/meteo/report-meteo/rapporti-annuali (last access: 5 May 2022), 2020a.
Arpae Emilia-Romagna: Stato del litorale Emiliano-Romagnolo al 2018 Erosione e interventi di difesa, ISBN 978-88-87854-48-0, 2020b.
Bailey, P., Wheaton, J., Reimer, M., and Brasington, J.: Geomorphic Change Detection Software, Riverscapes Consortium, Version 7.5.0, Zenodo [software], https://doi.org/10.5281/zenodo.7248344, 2020.
Biolchi, L. G., Unguendoli, S., Bressan, L., Giambastiani, B. M. S., and Valentini, A.: Ensemble technique application to an XBeach-based coastal Early Warning System for the Northwest Adriatic Sea (Emilia-Romagna region, Italy), Coast. Eng., 173, 104081, https://doi.org/10.1016/j.coastaleng.2022.104081, 2022.
Casella, E., Drechsel, J., Winter, C., Benninghoff, M., and Rovere, A.: Accuracy of sand beach topography surveying by drones and photogrammetry, Geo-Mar. Lett., 40, 255–268, https://doi.org/10.1007/s00367-020-00638-8, 2020.
Church, M. and Ashmore, P. E.: Sediment transport and river morphology: a paradigm for study, Gravel-bed Rivers in the Environment, 345, 115–139, 1998.
Collins, B. D., Bedford, D. R., Corbett, S. C., Cronkite-Ratcliff, C., and Fairley, H. C.: Relations between rainfall-runoff-induced erosion and aeolian deposition at archaeological sites in a semi-arid dam-controlled river corridor, Earth Surf. Proc. Land., 41, 899–917, https://doi.org/10.1002/esp.3874, 2016.
De Vriend, H. J. and Van Koningsveld, M.: Building with Nature: Thinking, Acting and Interacting Differently, Ecoshape, Dordrecht, the Netherlands, 39, ISBN 978-94-6190-957-2, 2012.
Della Bella, A., Fantinato, E., Scarton, F., and Buffa, G.: Mediterranean developed coasts: what future for the foredune restoration?, J. Coast. Conserv., 25, 49, https://doi.org/10.1007/s11852-021-00838-z, 2021.
Dong, Z., Luo, W., Qian, G., and Lu, P.: Wind tunnel simulation of the three-dimensional airflow patterns around shrubs, J. Geophys. Res.-Earth, 113, F02016, https://doi.org/10.1029/2007JF000880, 2008.
Duo, E., Fabbri, S., Grottoli, E., and Ciavola, P.: Uncertainty of Drone-Derived DEMs and Significance of Detected Morphodynamics in Artificially Scraped Dunes, Remote Sens., 13, 1823, https://doi.org/10.3390/rs13091823, 2021.
Eichmanns, C., Lechthaler, S., Zander, W., Pérez, M. V., Blum, H., Thorenz, F., and Schüttrumpf, H.: Sand Trapping Fences as a Nature-Based Solution for Coastal Protection: An International Review with a Focus on Installations in Germany, Environments, 8, 135, https://doi.org/10.3390/environments8120135, 2021.
Eltner, A., Kaiser, A., Castillo, C., Rock, G., Neugirg, F., and Abellán, A.: Image-based surface reconstruction in geomorphometry – merits, limits and developments, Earth Surf. Dynam., 4, 359–389, https://doi.org/10.5194/esurf-4-359-2016, 2016.
Fabbri, S., Grottoli, E., Armaroli, C., and Ciavola, P.: Using High-Spatial Resolution UAV-Derived Data to Evaluate Vegetation and Geomorphological Changes on a Dune Field Involved in a Restoration Endeavour, Remote Sens.-Basel, 13, 1987, https://doi.org/10.3390/rs13101987, 2021.
Faelga, R. A., Cantelli, L., Silvestri, S., and Giambastiani, B. M. S.: Dataset for the study 'Dune belt restoration effectiveness assessed by UAV topographic surveys (northern Adriatic coast, Italy), v1.0.0, Zenodo [data set and code], https://doi.org/10.5281/zenodo.10253228, 2023.
Fernández-Montblanc, T., Duo, E., and Ciavola, P.: Dune reconstruction and revegetation as a potential measure to decrease coastal erosion and flooding under extreme storm conditions, Ocean Coast. Manage., 188, 105075, https://doi.org/10.1016/j.ocecoaman.2019.105075, 2020.
Giambastiani, B., Greggio, N., Sistilli, F., Fabbri, S., Scarelli, F., Candiago, S., Anfossi, G., Lipparini, C., Cantelli, L., Antonellini, M., and Gabbianelli, G.: RIGED-RA project – Restoration and management of Coastal Dunes in the Northern Adriatic Coast, Ravenna Area – Italy, World Multidisciplinary Earth Sciences Symposium (WMESS 2016), IOP C. Ser. Earth Env., 44, 052038, https://doi.org/10.1088/1755-1315/44/5/052038, 2016.
Grams, P. E., Schmidt. J. C., Wright, S. A., Topping, D. J., Melis, T. S., and Rubin, D. M.: Building sandbars in the Grand Canyon, EOS T. Am. Geophys. Un., 96, https://doi.org/10.1029/2015EO030349, 2015.
Hanley, M. E., Hoggart, S. P. G., Simmonds, D. J., Bichot, A., Colangelo, M. A., Bozzeda, F., Heurtefeux, H., Ondiviela, B., Ostrowski, R., Recio, M., Trude, R., Zawadzka-Kahlau, E., and Thompson, R. C.: Shifting sands? Coastal protection by sand banks, beaches and dunes, Coast. Eng., 87, 136–146, https://doi.org/10.1016/j.coastaleng.2013.10.020, 2014.
Harley, M. D., Valentini, A., Armaroli, C., Perini, L., Calabrese, L., and Ciavola, P.: Can an early-warning system help minimize the impacts of coastal storms? A case study of the 2012 Halloween storm, northern Italy, Nat. Hazards Earth Syst. Sci., 16, 209–222, https://doi.org/10.5194/nhess-16-209-2016, 2016.
Hesp, P.: Foredunes and blowouts: initiation, geomorphology, and dynamics, Geomorphology, 48, 245–268, https://doi.org/10.1016/S0169-555X(02)00184-8, 2002.
Hilgendorf, Z., Marvin, M. C., Turner, C., and Walker, I. J.: Assessing Geomorphic Change in Restored Coastal Dune Ecosystems Using a Multi-Platform Aerial Approach, Remote Sens.-Basel, 13, 354, https://doi.org/10.3390/rs13030354, 2021.
Itzkin, M., Moore, L. J., Ruggiero, P., and Hacker, S. D.: The effect of sand fencing on the morphology of natural dune systems, Geomorphology, 352, 106995, https://doi.org/10.1016/j.geomorph.2019.106995, 2020.
Kasprak, A., Wheaton, J. M., Ashmore, P. E., Hensleigh, J. W., and Peirce, S.: The relationship between particle travel distance and channel morphology: results from physical models of braided rivers, J. Geophys. Res.-Earth, 120, 55–74, https://doi.org/10.1002/2014JF003310, 2015.
Kasprak, A., Bransky, N. D., Sankey, J. B., Caster, J., and Sankey, T. T.: The effects of topographic surveying technique and data resolution on the detection and interpretation of geomorphic change, Geomorphology, 333, 1–15, https://doi.org/10.1016/j.geomorph.2019.02.020, 2019.
Lane, S. N., Westaway, R. M., and Hicks, M.: Estimation of erosion and deposition volumes in a large, gravel-bed, braided river using synoptic remote sensing, Earth Surf. Proc. Land., 28, 249–271, https://doi.org/10.1002/esp.483, 2003.
Laporte-Fauret, Q., Castelle, B., Michalet, R., Marieu, V., Bujan, S., and Rosebery, D.: Morphological and ecological responses of a managed coastal sand dune to experimental notches, Sci. Total Environ., 782, 146813, https://doi.org/10.1016/j.scitotenv.2021.146813, 2021.
Mancini, F., Dubbini, M., Gattelli, M., Stecchi, F., Fabbri, S., and Gabbianelli, G.: Using Unmanned Aerial Vehicles (UAV) for High-Resolution Reconstruction of Topography: The Structure from Motion Approach on Coastal Environments, Remote Sens.-Basel, 5, 6880–6898, https://doi.org/10.3390/rs5126880, 2013.
Matias, A., Ferreira, O., Mendes, I., Dias, J. A., and Vila-Consejo, A.: Artificial construction of dunes in the south of Portugal, J. Coast. Res., 21, 472–481, https://doi.org/10.2112/03-0047.1, 2005.
Nordstrom, K. F. and Arens, S. M.: The role of human actions in evolution and management of foredunes in the Netherlands and New Jersey, USA, Journal of Conservation, 4, 169–180, 1998.
Perini, L., Calabrese, L., Luciani, P., Olivieri, M., Galassi, G., and Spada, G.: Sea-level rise along the Emilia-Romagna coast (Northern Italy) in 2100: scenarios and impacts, Nat. Hazards Earth Syst. Sci., 17, 2271–2287, https://doi.org/10.5194/nhess-17-2271-2017, 2017.
Preti, M. and Zanuttigh, B.: Integrated beach management at Igea Marina, Italy: results of ten-years monitoring, Coastal Engineering Proceedings, 1, 33, https://doi.org/10.9753/icce.v32.management.33, 2011.
Ruz, M. H. and Anthony, E. J.: Sand trapping by brushwood fences on a beach-foredune contact: The primacy of the local sediment budget, Z. Geomorphol. Supp., 52, 179–194, https://doi.org/10.1127/0372-8854/2008/0052S3-0179, 2008.
Sankey, J. B., Kasprak, A., and Caster, J.: Geomorphic Process from Topographic Form: Automating the Interpretation of Repeat Survey Data to Understand Sediment Connectivity for Source-Bordering Aeolian Dunefields in River Valleys, Advancing Earth and Space Science (AGU), Vol. 2016, https://ui.adsabs.harvard.edu/abs/2016AGUFMEP21A0856S/abstract (last access: 1 December 2023), https://doi.org/10.1002/esp.4143, 2016.
Scarelli, F. M., Sistilli, F., Fabbri, S., Cantelli, L., Barboza, E. G., and Gabbianelli, G.: Seasonal dune and beach monitoring using photogrammetry from UAV surveys to apply in the ICZM on the Ravenna coast (Emilia-Romagna, Italy), Remote Sensing Applications: Society and Environment, 7, 27–39, https://doi.org/10.1016/j.rsase.2017.06.003, 2017.
Sekovski, I., Del Río, L., and Armaroli, C.: Development of a coastal vulnerability index using analytical hierarchy process and application to Ravenna province (Italy), Ocean Coast. Manage., 183, 104982, https://doi.org/10.1016/j.ocecoaman.2019.104982, 2020.
Silvestri, S., Nguyen Ngoc, D., and Chiapponi, E.: Comment on “Soil salinity assessment by using near-infrared channel and Vegetation Soil Salinity Index derived from Landsat 8 OLI data: a case study in the Tra Vinh Province, Mekong Delta, Vietnam” by Kim-Anh Nguyen, Yuei-An Liou, Ha-Phuong Tran, Phi-Phung Hoang and Thanh-Hung Nguyen, Progress in Earth and Planetary Science, 9, 1–8, https://doi.org/10.1186/s40645-022-00490-7, 2022.
Sloss, C. R., Shepherd, M., and Hesp, P.: Coastal Dunes: Geomorphology, Nature Education Knowledge, 3, 2, 2012.
Talavera, L., Del Río, L., Benavente, J., Barbero, L., and López-Ramírez, J.: UAS as tools for rapid detection of storm-induced morphodynamic changes at Camposoto beach, SW Spain, Int. J. Remote Sens., 39, 15–16, https://doi.org/10.1080/01431161.2018.1471549, 2018.
Taramelli, A., Di Matteo, L., Ciaoval, P., Guadagnano, F., and Tolomei, C.: Temporal evolution of patterns and processes related to subsidence of the coastal area surrounding the Bevano River mouth (Northern Adriatic) – Italy, Ocean Coast. Manage., 108, 74–88, https://doi.org/10.1016/j.ocecoaman.2014.06.02, 2015.
Van Puijenbroek, M., Limpens, J., De Groot, A., Riksen, M., Gleichman, M., Slim, P., van Dobben, H., and Berendse, F.: Embryo dune development drivers: beach morphology, growing season precipitation, and storms: Embryo Dune Development and Climate, Earth Surf. Proc. Land., 42, 1733–1744, https://doi.org/10.1002/esp.4144, 2017.
Wheaton, J.: Uncertainty in Morphological Sediment Budgeting of Rivers, Unpublished PhD thesis, University of Southampton, Southampton, 412 pp., https://sites.google.com/a/joewheaton.org/www/Home/research/projects-1/morphological-sediment-budgeting/phdthesis (last access: 9 June 2022), 2008.
Wheaton, J., Brasington, J., Darby, S., Merz, J., Pasternack, G., and Sear, D., Vericat, D.: Linking geomorphic changes to salmonid habitat at a scale relevant to fish, River Res. Appl., 26, 469–486, https://doi.org/10.1002/rra.1305, 2009a.
Wheaton, J., Brasington, J., Darby, S., and Sear, D.: Accounting for uncertainty in DEMs from repeat topographic surveys: improved sediment budgets, Earth Surf. Proc. Land., 35, 136–156, https://doi.org/10.1002/esp.1886, 2009b.
Wheaton, J. M., Brasington, J., Darby, S. E., Kasprak, A., Sear, D., and Vericat, D.: Morphodynamic signatures of braiding mechanisms as expressed through change in sediment storage in a gravel-bed river, J. Geophys. Res.-Earth, 118, 759–779, https://doi.org/10.1002/jgrf.20060, 2013.
Wootton, L., Miller, J., Miller, C., Peek, M., Williams, A., and Rowe, P.: New Jersey Sea grant consortium dune manual, New Jersey Sea Grant Consortium, https://njseagrant.org/wp-content/uploads/2016/12/Dune-Manual-Pgs-for-website.pdf (last access: 9 June 2022), 2016.
Yousefi Lalimi, F., Silvestri, S., Moore, L. J., and Marani, M.: Coupled topographic and vegetation patterns in coastal dunes: Remote sensing observations and ecomorphodynamic implications, J. Geophys. Res.-Biogeo., 122, 119–130, https://doi.org/10.1002/2016JG003540, 2017.