the Creative Commons Attribution 4.0 License.
the Creative Commons Attribution 4.0 License.
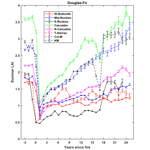
Divergent biophysical responses of western United States forests to wildfire driven by eco-climatic gradients
Surendra Shrestha
Christopher A. Williams
Brendan M. Rogers
John Rogan
Dominik Kulakowski
Understanding vegetation recovery after fire is critical for predicting vegetation-mediated ecological dynamics in future climates. However, information characterizing vegetation recovery patterns after fire and their determinants over large geographical extents is limited. This study uses Moderate Resolution Imaging Spectroradiometer (MODIS) leaf area index (LAI) and albedo to characterize patterns of post-fire biophysical dynamics across the western United States (US) and further examines the influence of topo-climatic variables on the recovery of LAI and albedo at two different time horizons, 10 and 20 years post-fire, using a random forest model. Recovery patterns were derived for all wildfires that occurred between 1986 and 2017 across seven forest types and 21 level III ecoregions of the western US. We found differences in the characteristic trajectories of post-fire vegetation recovery across forest types and eco-climatic settings. In some forest types, LAI had recovered to only 60 %–70 % of the pre-fire levels by 25 years after the fire, while it recovered to 120 %–150 % of the pre-fire levels in other forest types, with higher absolute post-fire changes observed in forest types and ecoregions that had a higher initial pre-fire LAI. Our random forest results showed very little influence of fire severity on the recovery of both summer LAI and albedo at both post-fire time horizons. Post-fire vegetation recovery was most strongly controlled by elevation, with faster rates of recovery at lower elevations. Similarly, annual precipitation and average summer temperature had significant impacts on the post-fire recovery of vegetation. Full recovery was seldom observed when annual precipitation was less than 500 mm and average summer temperature was above the optimal range, i.e., 15–20 °C. Climate influences, particularly annual precipitation, were a major driver of post-fire summer albedo change through its impact on ecological succession. This study provides quantitative measures of primary controls that could be used to improve the modeling of ecosystem dynamics post-fire.
- Article
(4267 KB) - Full-text XML
-
Supplement
(821 KB) - BibTeX
- EndNote
Wildfires have burned millions of hectares of forests in the western United States (Littell et al., 2009; Westerling et al., 2006) and have increased in both frequency and severity in recent decades. This trend has been attributed to temperature increases, more frequent droughts, below-average winter precipitation, and earlier spring snowmelt (Dale et al., 2001; Westerling et al., 2006; Rogers et al., 2011; Ghimire et al., 2012; Dennison et al., 2014; Abatzoglou and Williams, 2016; Williams and Abatzoglou, 2016; Williams et al., 2021), making ecosystem resilience and vegetation recovery post-fire a primary concern to researchers and land managers (Allen et al., 2015). Existing studies report that large wildfires in western US forests have increased 4-fold since 1970–1986, with the total burn area increasing by 6.5 times (Westerling et al., 2006). Expanded burning can profoundly alter a wide range of ecosystem characteristics such as stand structure, species composition, leaf area, canopy ecophysiology, and microclimate (Liu et al., 2005). The most immediate biophysical effect of wildfire on the land surface is the decrease in live vegetation and the deposition of black carbon on the soil surface (De Sales et al., 2018). The alteration in surface roughness directly influences the interaction between the land and the atmosphere by, typically, reducing the turbulent mixing and net radiation (Chambers et al., 2005). Moreover, the deposition of the black carbon on the surface changes net radiation through its impact on the surface albedo, which alters the partitioning of energy into latent heat and sensible heat (Jin and Roy, 2005). Fires have the potential to modify local to regional climate through these long-lived changes in land surface dynamics and other substantial forcing impacts such as greenhouse gas fluxes and aerosols (Bonan et al., 1995). In this study, we use contemporary spaceborne observing systems to quantify the magnitude and timing of ecosystem responses to severe wildfires as a crucial step in assessing their associated ecological, hydrological, and biogeophysical impacts.
In addition to quantification, it is equally important to document the factors that determine variability in post-fire recovery in order to develop a predictive understanding of ecosystem dynamics in response to wildfire, especially considering present and expected future increases in the frequency of large, severe wildfires (Scholze et al., 2006; IPCC, 2007; Seastedt et al., 2008; Urza et al., 2017; Hankin et al., 2019). Vegetation recovery is likely to vary considerably across the landscape, even when initial estimates of fire severity are similar (Keeley et al., 2008; Frazier et al., 2018). Some forest ecosystems have been shown to recover fully after large severe disturbances (Rodrigo et al., 2004; Knox and Clarke, 2012), while others have recovered little towards pre-fire levels (Barton, 2002; Rodrigo et al., 2004; Lippok et al., 2013). Variability in recovery rates has been shown to depend on the interactive effects of numerous biotic and abiotic factors related to the nature of the fire, life history traits of species, and environmental conditions following fire (Chambers et al., 2016; Johnstone et al., 2016; Stevens-Rumann et al., 2018). For example, post-fire recovery of dry mixed conifer forests in the western US is strongly affected by fire severity (Chappell and Agee, 1996; Meng et al., 2015, 2018; Kemp et al., 2016; Harvey et al., 2016; Vanderhoof et al., 2020) and pre-fire condition (Martin-Alcon and Coll, 2016; Zhao et al., 2016). Other factors that can be important in vegetation recovery after fire include vegetation type (Epting and Verbyla, 2005; Yang et al., 2017); site topography, including slope, aspect, and elevation (Wittenberg et al., 2007; Meng et al., 2015; Liu, 2016; Chambers et al., 2016; Haffey et al., 2018); and post-fire climate, including temperature and moisture conditions (Chappell and Agee, 1996; Meng et al., 2015; Stevens-Rumann et al., 2018; Kemp et al., 2019; Guz et al., 2021). Long-term assessment of post-fire vegetation recovery across forest types can offer valuable insights to researchers and land managers who seek to identify areas that could benefit from post-fire management and to develop potential management actions such as fuel treatment, prescribed fire, and carbon management.
Several studies have documented vegetation recovery and associated biogeophysical and biogeochemical dynamics in response to wildfires by employing field-based observations, including flux tower measurements (Chambers and Chapin III, 2002; Jin and Roy, 20005; Amiro et al., 2006; Randerson et al., 2006; Campbell et al., 2007; Dore et al., 2010; Kemp et al., 2016; Hankin et al., 2019; Ma et al., 2020), remote sensing observations (Veraverbeke et al., 2012a; O'Halloran et al., 2014; Micheletty et al., 2014; Rogers et al., 2015; Bright et al., 2019; Vanderhoof et al., 2020), and modeling approaches driven by remote sensing observations (Hicke et al., 2003; Bond-Lamberty et al., 2009; Williams et al., 2012; Rogers et al., 2013; Maina and Siirila-Woodburn, 2019). While instructive and critical for mechanistic understanding, local field-based studies on post-fire ecological dynamics tend to focus on small, localized areas and encompass only a single or a few wildfire events (Meigs et al., 2009; Montes-Helu et al., 2009; Downing et al., 2019). In contrast, large-scale regional analyses using remotely sensed observations and modeling approaches tend to focus on Mediterranean (Veraverbeke et all., 2012a, b; Meng et al., 2014; Yang et al., 2017) and boreal (Amiro et al., 2000, 2010; Chambers and Chapin, 2002; Randerson et al., 2006; Lyons et al., 2008; Jin et al., 2012; Rogers et al., 2013; Hislop et al., 2020) ecosystems or on only a few forest types (mostly ponderosa pine and mixed conifer forests of the western US) (Chen et al., 2011; Dore et al., 2012; Meng et al., 2015; Roche et al., 2018; Bright et al., 2019; Littlefield et al., 2020). Moreover, those studies did not examine how their results scale up to multiple fire events across broad regions.
The purpose of this study is to provide a more precise estimate of wildfire impacts on LAI and surface albedo in seven different forest types of the western US using observations derived from MODIS. Moreover, this study also examines the factors that influence the nature and rate of vegetation recovery in the post-fire environment. The hypotheses used in this work are that (1) the rate of recovery of LAI following wildfire varies across forest types and eco-climatic settings, (2) the change in vegetation cover post-fire induces a change in the albedo which varies with forest type and eco-climatic setting, and (3) the variability in the post-fire response of albedo is attributable to the same factors that explain the variability in LAI post-fire.
2.1 Study area
This study was carried out in the western US, a region that has been severely disturbed by wildfires in the last several decades. Its extent for the purpose of this study (Fig. 1) encompasses the conterminous US west of the 100th meridian (Thompson et al., 2003). This region is geographically diverse, with high physiographic relief and strong local and regional climatic gradients (Bartlein and Hostetler, 2003), including regions such as temperate rain forests, high mountain ranges, great plains, and deserts (Thompson et al., 2003). Our study considered seven forest types that are dominant across the western US, as defined by the US Forest Service's National Forest Type dataset (Ruefenacht et al., 2008), including Douglas fir, pinyon–juniper, ponderosa pine, spruce–fir–hemlock, mixed conifer, lodgepole pine, and oak. Within these forest types, we only considered areas that were burned with high severity (as defined by Monitoring Trends in Burn Severity, MTBS) to examine the post-fire biophysical dynamics. In the case of attributing post-fire recovery, we considered all fire severity classes from MTBS in our random forest model to determine the influences of these classes on the post-fire recovery of vegetation and surface albedo. Within each ecoregion, we selected only those forest types that cover >10 % of the ecoregion's forest area and had >1 % pixels burned with high severity. As a result, only 21 out of 35 level III ecoregions of the western US (Table S1 in the Supplement) (Omernik, 1987) had a sufficient number of 500 m×500 m pixels that saw high-severity burning within those forest types to support the generation of forest-type-specific chronosequences of post-fire ecological responses. Across these ecoregions, average annual precipitation (1981–2010) was 900±490 mm yr−1 (mean ± SD), while the mean summer minimum and maximum temperatures were 23°±2.8 and 7°±2.5 °C, respectively (Parameter-elevation Regressions on Independent Slopes Model – PRISM; Daly et al., 2008).
2.2 Remote sensing data and data products
The burned area and fire severity data for the period of 1986–2017 used in this study were obtained from Monitoring Trends in Burn Severity (MTBS) (Eidenshink et al., 2007). We divided our study into different forest types to analyze the recovery of LAI and albedo post-fire, utilizing a USFS forest type group map (Ruefenacht et al., 2008). We resampled the MTBS dataset from its native resolution of 30 m to a coarser (500 m) resolution. During this process, we retained only those 500 m pixels in which at least 75 % of the corresponding 30 m pixels were burned, thus reducing noise from pixels with an unclear mix of burned and unburned conditions. Similarly, we resampled the forest type grid from 250 to 500 m resolution and selected pixels where at least 75 % of the forest within the pixel belonged to a single forest type based on the 250 m forest type group map. We excluded pixels that were burned more than once between 1986 and 2017, as such pixels can add noise to the post-fire trajectory of biophysical properties.
This study analyzed two spatially and temporally consistent MODIS products, LAI and shortwave white-sky albedo, to assess fire-induced change in vegetation and surface albedo in the western US. The MODIS satellite data tile subsets (tiles h8v4, h8v5, h9v4, h9v5, h10v4, and h10v5) from 2001 to 2019 were downloaded from the MODIS data archive (https://www.earthdata.nasa.gov/, last access: 28 July 2022). Within each data tile, we employed the quality assurance (QA) bits embedded in the MODIS products to ensure that only the highest-quality values (flagged as “0”) were included. This process involved removing all retrievals affected by cloud cover and those flagged for low quality. The MODIS LAI product (MCD15A2H; Myneni et al., 2002) reports the green leaf area index, which represents the amount of one-sided green leaf area per unit ground area in broadleaf canopies or half the total surface area of needles per unit ground area in coniferous canopies. The MODIS LAI algorithm utilizes a main look-up table (LUT)-based procedure that makes use of spectral information contained in the red and NIR bands along with a back-up algorithm that relies on an empirical relationship between the normalized difference vegetation index (NDVI), canopy LAI, and fraction of photosynthetically active radiation (fPAR) (Myneni et al., 2002).
For albedo, we used the daily MODIS Collection 6 bidirectional reflectance distribution function (BRDF)/albedo product at 500 m resolution (MCD43A3; Schaaf et al., 2002). The use of both Terra and Aqua data in this product provides more diverse angular samplings and increases the probability of high-quality input data that allow more accurate BRDF and albedo retrievals. The MODIS albedo algorithm uses a bidirectional reflectance distribution and shortwave reflectances (0.3–5.0µm) and provides both black-sky and white-sky albedos. We used shortwave broadband white-sky albedo for this study because it is less biased in complex terrain and less sensitive to viewing and solar angles (Gao et al., 2005). We stratified the sampling of white-sky albedo into snow-free and snow-covered conditions based on the presence or absence of snow determined at pixel level by the MODIS daily snow cover 500 m product (MOD10A1; Salomonson and Appel, 2004). We assigned snow-free and snow-covered conditions using thresholds of less than 30 % and greater than 75 % snow cover. We chose these thresholds as a balance between inclusion (to achieve robust sampling) and exclusion (to reduce noise from pixels with an unclear mix of snow and snow-free conditions). We are aware that much of our study domain does not have considerable snow cover during winter, and these snow-free winter albedos had similar patterns and magnitudes to summer albedos (Fig. S1 in the Supplement). Therefore, the average summer (June–August) albedo values presented here represent the snow-free condition only, while the average winter (December–February) values presented include only snow-covered conditions. We did not report winter albedos for all forest types because of limits on the availability of high-quality snow-covered pixels.
As part of our attribution analysis that seeks to identify factors that influence the pattern of post-fire biophysical dynamics, we acquired a suite of climate variables – monthly mean summer precipitation, monthly mean summer temperature, monthly minimum summer temperature, monthly maximum summer temperature, and total annual precipitation – covering the 2001–2019 period from the Parameter-elevation Regressions on Independent Slopes Model (PRISM; Daly et al., 2008). PRISM utilizes point measurements of precipitation and temperature to generate continuous digital grid estimations for climate data with a 4 km spatial resolution (Daly et al., 1994). The elevations of all burned pixels were taken from the US Geological Survey (USGS) National Elevation Dataset (NED) at 30 m (U.S. Geological Survey, 2019). All topo-climatic variables were re-gridded to the 500 m MODIS resolution for uniformity.
2.3 Generating chronosequences of post-fire LAI and albedo
To address unrealistic variation in MODIS land surface products (Cohen et al., 2006), we computed mean monthly values by adding all samples and dividing the resulting value by the number of samples in each month within our stratified design. For the summer season, we computed mean summer-season values of LAI and albedo by averaging the data from June, July, and August. Similarly, for the winter season, yearly values of LAI and albedo were computed the same way using data from December, January, and February. Next, we analyzed the changes in post-fire LAI and albedo relative to the pre-fire LAI and albedo by sampling each of them as an annual time series from 3 years before wildfire events to 25 years after wildfire events. We grouped samples from each fire event based on the forest type, eco-climatic setting, and snow cover conditions. Within these groups, we composited burn events from different years and aligned them temporally to represent 3 years prior to the fire and 25 years after the fire. Consequently, chronosequences of biophysical properties as a function of time since fire were created for a combination of seven forest types, two snow cover conditions (in the case of albedo), and 21 sub-ecoregions.
2.4 Attribution of recovery
We explored the relationships between albedo, LAI recovery, and topo-climatic factors and subsequently attributed the recovery at 10 years post-fire and 20 years post-fire using random forest (RF) algorithms implemented in R (Breiman, 2001; Liaw and Wiener, 2002). We used a nonparametric modeling method because most variable distributions were non-normal and RF does not require the variables to be normally distributed. Additionally, RF can handle tens of thousands of data points and provides variable importance scores. We initially selected seven explanatory variables – fire severity class (low, medium, and high), three temperature variables, two precipitation variables, and elevation. Although RFs do not require collinear variables to be removed (Breiman, 2001), we employed a variance inflation factor (VIF) analysis for multicollinearity as a variable selection method to improve computation efficiency and enhance interpretation, particularly with respect to variable importance. VIF analysis involves (a) calculating VIF factors, (b) removing the predictors with VIF >10 from this set, and (c) repeating until no variable has VIF >10. This provided us with four uncorrelated predictors to be used in the RF model – fire severity class, total annual precipitation, mean summer temperature (June–August), and elevation. We pooled post-fire LAI and albedo responses across 21 ecoregions within a given forest type for both time horizons (10 years post-fire and 20 years post-fire). The dataset was divided into a training (80 %) dataset to train the RF model and a test (20 %) dataset to validate the model. We created four RF models with 500 binary decision trees for each forest type (one for each time horizon for both LAI and albedo). We tuned the model to generate a model with the highest accuracy, i.e., the lowest out-of-bag error among all tested combination of parameter values. The model's performance was assessed using the R2 metric. We used unscaled permutation accuracy instead of the traditional Gini-based importance metric to rank the explanatory variables by relative importance, as the Gini-based importance was shown to be more strongly biased towards continuous variables or variables with more categories compared to other importance metrics (Strobl et al., 2007). The unscaled permutation importance metric calculates variable importance scores as the amount of decrease in the accuracy when a target variable is excluded. We used partial dependence plots (PDPs) to visualize the influence of each explanatory variable on the degree of recovery of LAI and albedo at 10 and 20 years post-fire. A PDP quantifies the marginal effects of a given variable on an outcome and provides a mechanism to explore insight in big datasets, especially when the random forest is dominated by lower-order interactions (Martin, 2014).
3.1 Post-fire recovery of land surface properties
Burning caused a large decline in LAI for all forest types. Generally, compared to other forest types, high-productivity forests (e.g., Douglas fir and mixed conifers) experienced a larger decline in LAI in year 1 after fire (Fig. 2a–g). Compared to pre-fire levels, the decline in LAI ranged from 47 % in pinyon–juniper to 76 % in ponderosa pine forests (Table S2). After this initial decrease, the effects of vegetation regeneration became apparent. For all forest types, the magnitude of LAI change decreases with increasing time since fire. However, LAI did not recover to the pre-fire condition in most cases within the 25-year period of observation available for this study. We found large differences in the timing of LAI recovery across forest types, with forest types recovering at different rates, crossing the pre-fire levels at different times, and reaching different peaks in LAI (Fig. 2a–g). For example, Douglas fir in the Columbia Mountains, Klamath Mountains, and southern Rockies (Fig. 2g) and mixed conifers in Baja California and the Eastern Cascades (Fig. 2a) showed complete recovery of LAI to pre-fire levels within the 25-year study period, while lodgepole pine, oak, and ponderosa pine were characterized by a slower recovery rate and most did not recover to pre-fire levels by the end of the 25-year period (Fig. 2 and Table S2). We also found varied recovery rates across geographic regions even within a single forest type. This is presumably related to climate and soils. For example, the characteristic post-fire LAI trajectories for the high productivity Douglas fir forest type (Fig. 2g) showed a substantially faster recovery in the Cascades, Klamath Mountains, and Columbia Mountains regions compared to the Idaho Batholith region of the western US. Based on observations from all forest types, in general, faster recovery of LAI was observed in high-elevation, wet areas with substantial maritime influences.
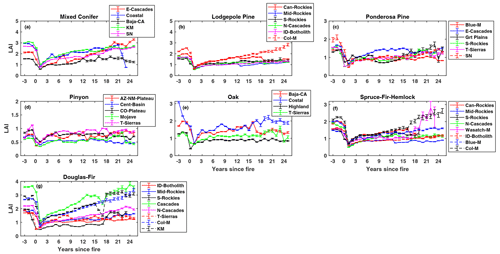
Figure 2Mean summer post-fire LAI (± SE) as a function of time since fire in seven different forest types of the western US. Sub-ecoregions: E-Cascades, Eastern Cascades; Coastal, Coastal Sage; Baja-CA, Baja California; KM, Klamath Mountains; SN, Sierra Nevada; Can-Rockies, Canadian Rockies; Mid-Rockies, Middle Rockies; S-Rockies, Southern Rockies; N-Cascades, Northern Cascades; ID-Batholith, Idaho Batholith; Col-M, Columbia Mountains; Blue-M, Blue Mountains; Grt Plains, Great Plains; T-Sierras, Temperate Sierras; AZ-NM-Plateau, Arizona–New Mexico Plateau; Cent-Basin, Central Basin; CO-Plateau, Colorado Plateau; Mojave, Mojave Basin; Highland, North American Highland; Wasatch-M, Wasatch Mountains.
Turning to albedo, we found significant changes in summer albedo post-fire for all forest types. Three important trends, which were similar for all the forest types, emerged from these post-fire summer albedo trajectories. First, for all forest types, summer albedo decreased immediately after fire (Fig. 3), likely due to the low reflectivity caused by black-carbon deposition on the soil surface and dead tree boles, which are both common immediately after high-severity burning. The decline in summer albedo ranged from 0.01–0.02 across forest types, with the greatest decline (20 % from pre-fire levels; Table S3) observed in Douglas fir forest of the Klamath Mountains region. Second, post-fire albedo increased gradually from year 2 since fire, crossing the pre-fire levels at around 3 years post-fire and peaking at different time horizons for different forest types and regions (Fig. 3a–g). The elevated post-burn albedo is presumably due to increasing canopy cover, the relative high albedo of grasses and shrubs that establish in early succession, and the loss of black carbon coatings on soil and woody debris (Chambers and Chapin, 2002). The timing and magnitude of peak post-fire albedo varied across forest types. For example, ponderosa pine showed its peak in post-fire albedo at 18 years post-fire (Fig. 3c) and the peak occurred at 11 years post-fire for one of the mixed conifer regions (Fig. 3a), while slow-growing species such as spruce–fir–hemlock may not have reached their peak by the end of the 25-year post-fire study period (Fig. 3f). Similarly, there were significant regional differences in the timing and magnitude of peak albedo for a given forest type group. For example, mixed conifer post-fire albedo peaked at 11 years post-fire in Baja California, while it continued to increase through to 25 years in the Klamath Mountains (Fig. 3a). Third, as the post-fire LAI approached the pre-fire LAI levels, post-fire albedo started to decline from the peak towards its pre-fire albedo, but it did not reach the pre-fire albedo levels by the end of the 25-year study period (Fig. 3a–g).
For each forest type, post-fire winter albedo had a similar pattern to summer albedo, except that it had a greater magnitude and it increased immediately after fire (Fig. 4a–f and Table S4). We observed greater interannual variability in the time series of post-fire winter albedo, which was likely related to variability in snow cover and a smaller signal-to-noise ratio associated with smaller sample sizes. The albedo response was more than 3-fold larger in winter than in summer, peaking in the range of 0.4 to 0.6 across forest types and showing an increase over pre-fire levels of about 0.25 to 0.50. Similar to summer albedos, winter albedos did not return to the pre-fire levels by the end of the 25-year study period (Fig. 4a–f).
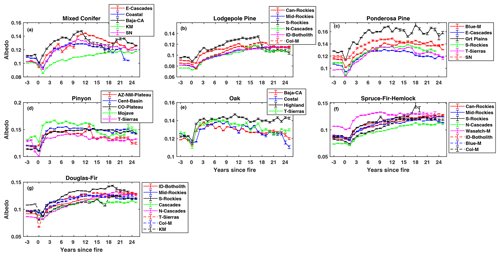
Figure 3Mean summer post-fire albedo (± SE) as a function of time since fire in seven different forest types of the western US.
3.2 Drivers of post-fire recovery of LAI and albedo
Our random forest model had high accuracy for the recovery of both LAI and albedo at 10 and 20 years post-fire. The out-of-bag (OOB) error rate of the random forest model for the relative recovery of LAI at 10 years post-fire was around 3 %–8 % (r2=0.66–0.78), while it was around 2.5 %–9 % (r2=0.65–0.78), 0.4 %–1.4 % (r2=0.55–0.83), and 0.3 %–1.6 % (r2=0.52–0.83) for the relative recovery of LAI at 20 years post-fire, the relative recovery of albedo at 10 years post-fire, and the relative recovery of albedo at 20 years post-fire, respectively (Table S5). The variable with the greatest importance for the LAI at 10 years post-fire agreed well with that for the LAI at 20 years post-fire for all forest types, indicating that the recovery of LAI at 10 years and that at 20 years post-fire were both largely determined by the same governing factors (Fig. S2). Among all the explanatory variables, the degrees of LAI recovery at 10 years and 20 years post-fire were both largely dominated by elevation and total annual precipitation (Fig. S2). In contrast, the factor with greatest influence on the post-fire summer albedo varied with forest type and time since fire. For example, in the mixed conifer forest type, annual precipitation was the major determinant of the albedo recovery at 10 years post-fire, while it was the average summer temperature at 20 years post-fire. Similarly, the degree of albedo recovery at 10 years post-fire in the spruce–fir–hemlock forest type was largely determined by average summer temperature, while that at 20 years post-fire was mainly determined by elevation. Fire severity, on the other hand, showed almost no explanatory power in predicting the recovery of LAI and albedo at both times for all forest types (Figs. S2, S3).
The degree of LAI recovery at 10 years post-fire increased with increasing total annual precipitation for all forest types, but it varied little when the total annual precipitation exceeded 1000 mm. Annual precipitation was the major determinant of the 10-year LAI recovery post-fire for dry forests like ponderosa pine, pinyon–juniper, and oak, and these forest types tended to recover to levels above pre-fire levels as the annual precipitation increased. However, when the annual precipitation is less than 500 mm, the relative change in LAI is below 0 for all forest types, indicating that the complete recovery of LAI at 10 years post-fire was unlikely when annual precipitation was less than 500 mm (Fig. 5c). In contrast, five out of seven forest types recovered to above pre-fire levels at 20 years post-fire with increased annual precipitation, indicating that mixed conifers and Douglas fir need more time and higher annual precipitation to recover to the pre-fire level. Only oak and ponderosa pine showed increased LAI at 20 years post-fire, as the annual precipitation exceeded 2000 mm (Fig. 6c). As with LAI, annual precipitation was one of the major determinants of both the 10-year and 20-year albedo recovery post-fire. The post-fire increase in albedo was greater for sites with less annual precipitation (Figs. 7c and 8c); this was particularly noticeable in dry forest types such as Douglas fir, ponderosa pine, and oak, where increased precipitation triggered a rapid increase in post-fire vegetation recovery. The oak forest type showed a particular anomaly in albedo at 20 years post-fire, exhibiting a decline of around 20 % below pre-fire levels for sites with an annual precipitation of 2000 mm or above (Fig. 8c), consistent with a rapid increase in vegetation recovery.
Regarding the average summer temperature, we found an interesting divergence in the pattern of LAI response between cool and hot climates. For forests growing in hotter conditions, the magnitude of LAI recovery at both time horizons decreased in areas with higher temperatures, particularly in oak, pinyon–juniper, and ponderosa pine forest types, as these forest types grow at the warmer end of the species distribution. In contrast, increases in the average summer temperature assisted the recovery of forest types growing at the colder end of the species distribution, such as lodgepole pine and spruce–fir–hemlock (Figs. 5d and 6d). It should be noted that LAI was consistently lower than pre-fire levels for these forest types at both time horizons. Albedo does not show the same divergence in pattern with warmer conditions, and instead we find a somewhat surprising pattern. Hotter sites tend to experience a larger enhancement of summertime albedo over the pre-fire condition at both time horizons in spite of the faster recovery of LAI with a hotter temperature (Figs. 7d and 8d).
Elevation was consistently found to be an important variable for determining the trajectory of post-fire vegetation recovery. The post-fire recovery of LAI was slower at a higher elevation at both 10 years and 20 years post-fire. Most forest types showed a complete recovery to pre-fire levels at an elevation below 1500 m. Only pinyon–juniper and ponderosa pine forest types saw a faster, more complete recovery of LAI with a higher elevation (Figs. 5b and 6b). Turning to albedo response, we found that a higher elevation led to a smaller increase in albedo over its pre-fire value for both time periods for the two forest types for which elevation was the most important predictor of post-fire albedo change, namely for pinyon–juniper and ponderosa pine forests. This is consistent with faster post-fire recovery of LAI at higher-elevation portions of the ranges for these two forest types. In contrast, the post-fire albedo of Douglas fir, mixed conifer, and oak forest types showed little dependence on elevation (Figs. 7b and 8b).
Although fire severity was the least important predictor of both post-fire LAI and albedo recovery at both time horizons, our results showed significant variation in post-fire recovery among severity classes for all forest types. As expected, the overall recovery of LAI at 10 years post-fire was greater for low fire severity, where the recovery ranged between 85 % and 95 % of pre-fire LAI levels (Fig. 5a). Only in the case of oak and pinyon–juniper forest types that burned with high severity did we see full recovery of LAI to pre-fire levels or above by 10 years post-fire. By 20 years post-fire, lodgepole pine and spruce–fir–hemlock still show a suppression of LAI relative to the pre-burn condition and less recovery for more severe burn conditions (Fig. 6a), while for oak, LAI is elevated over the pre-burn condition and the largest LAI is seen at sites that had the most severe fires (Fig. 6a). The four other forest types had LAI values equal to the pre-burn condition and showed no variation across fire severities. For albedo, all forest types showed a larger elevation of albedo over their pre-fire values under medium fire severity (Fig. 7a). Oak had the lowest change in albedo at both time horizons owing to a rapid post-fire recovery. Overall, post-fire albedo was consistently higher than pre-fire levels at both time horizons in all forest types, indicating that albedo requires more than 2 decades to return to pre-fire levels in these forest types (Figs. 7a and 8a).
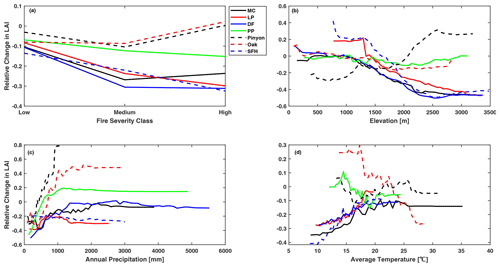
Figure 5Partial dependence of change in summer LAI at 10 years post-fire relative to the pre-fire condition on (a) fire severity, (b) elevation, (c) annual precipitation, and (d) mean monthly summer temperature. Forest types: MC, mixed conifer; LP, lodgepole pine; DF, Douglas fir; PP, ponderosa pine; Pinyon, pinyon–juniper; SFH, spruce–fir–hemlock. The y axis represents the change in LAI post-fire relative to the pre-fire LAI (degree of recovery), where negative values represent recovery to below pre-fire levels, 0 represents recovery to pre-fire levels, and positive values represent recovery to above pre-fire levels.
Here, we extended the regional research by Shrestha et al. (2022) with much broader sampling to study the post-fire responses for seven forest types in 21 sub-ecoregions of the western US. In addition, this study also uses a machine learning approach (random forest) to examine the influence of several topo-climatic variables on the nature and rate of vegetation recovery and the associated albedo in the post-fire environment.
4.1 Post-fire vegetation recovery
In this study, we used MODIS-derived LAI to increase our understanding of variability in the recovery of vegetation in the post-fire environment across seven forest types and 21 sub-ecoregions of the western United States. Similar to other studies (Morresi et al., 2019; Vanderhoof et al., 2020), we found rapid vegetation recovery in the first 10 years after fire. While LAI rebounded rapidly in the initial 10 years post-fire, this cannot be taken as a definitive indicator of successional trajectory, especially for slow-growing forests like subalpine fir (Ferguson and Carlson, 2010) or for forests with episodic post-fire germination such as ponderosa pine (Savage et al., 1996; Brown and Wu, 2005; Rodman et al., 2020). Leaf area recovery then slowed in most cases, and for many it did not return to the pre-fire level by the end of study period. We anticipate that the recovery of LAI to its pre-fire condition continues to unfold over time, extending beyond the 25-year duration covered by our study. In some cases, we see LAI at 20 or 25 years post-fire exceeding that prior to burning, suggesting that wildfire may have stimulated canopy renewal or the release of the understory. Evaluating post-fire LAI trajectories on these, and longer, timescales can be of value from a management perspective, for example, to identify regions where there is a risk of regeneration failure for dominant native species (Welch et al., 2016).
Our findings demonstrated differences in characteristic trajectories across forest types and ecoregions. Wildfire caused a similar proportional reduction in LAI across forest types and ecoregions, generally with a 30 % to 70 % reduction in year 1 post-fire but with smaller reductions in some pinyon–juniper settings (Table S2). We also found varied rates of LAI recovery post-fire across forest types and ecoregions. Some forest types saw LAI recover to only 60 % to 70 % by 25 years, while others saw LAI recover to 120 % to 150 % of the pre-fire condition (Table S2). Many factors are likely to contribute to these patterns across forest types and eco-climatic settings. First and foremost, it is no surprise that areas that are more suitable for growth show faster and more complete recovery with a higher absolute LAI within a given forest type. For example, Douglas fir stands in the Cascades, Columbia Mountains, and Klamath Mountains had faster recovery rates and greater changes in absolute LAI after year 1 post-fire than did stands in the Rockies and Temperate Sierras (Table S2). Similarly, we observed a consistent slow trend in the rate of conifer regeneration in the interior of the western US with a continental climate, where high-severity fire is common. This is likely due to reduced seed availability in response to larger high-severity fires in these areas (Cansler and McKenzie, 2014). Other factors include the regeneration capacity of the dominant tree species post-fire, with some readily and actively resprouting or having serotiny, while others lack these fire-adaptation traits (Howard, 2003; Meng et al., 2018), and competition with species such as early colonizers is common after burning (Hansen et al., 2016; Stoddard et al., 2018). The post-fire dynamics presented here are not stratified by post-fire species composition; they only characterize the biophysical characteristics that unfold after the burning of a particular forest type. Naturally, the post-fire species composition can differ from the pre-fire species composition depending on the seed and nutrient availability, fire severity, and climate, and these effects are embedded in the post-fire biophysical trajectories that we present. Further exploration of how post-fire species composition and other regeneration characteristics influence biophysical trajectories is warranted.
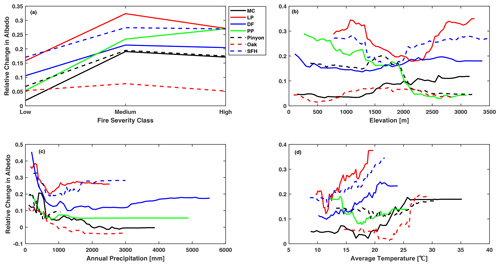
Figure 7Partial dependence of the change in summer snow-free albedo at 10 years post-fire relative to the pre-fire condition on (a) fire severity, (b) elevation, (c) annual precipitation, and (d) mean monthly summer temperature.
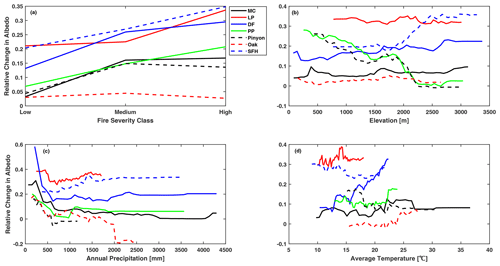
Figure 8Partial dependence of the change in summer snow-free albedo at 20 years post-fire relative to the pre-fire condition on (a) fire severity, (b) elevation, (c) annual precipitation, and (d) mean monthly summer temperature.
Our findings of post-fire LAI trajectories across eco-climatic settings suggest that the range of Douglas fir stands may be less limited due to climate warming compared to ponderosa pine, as their current range tends to extend into cooler and moister areas, where they recover to above pre-fire levels. This indicates that the worsening of climate change in the future (more periods of prolonged drought) could have implications for the migration of ponderosa pine due to worsening regeneration under climate stress. Although pinyon–juniper forests recovered rapidly in the first few post-fire years, our observed decline in the rate of pinyon–juniper recovery is consistent with the findings of Vanderhoof et al. (2020). This forest type is recognized for its slow regeneration and susceptibility to drought (Hartsell et al., 2020). Existing studies of the post-fire recovery of pinyon–juniper suggest that this forest type recovers to its pre-fire condition in <5 years after fire in the case of low to moderate fire severity (Jameson, 1962; Dwyer and Preper, 1967), while it takes >100 years for recovery to the pre-fire condition under high fire severity with heavy pinyon–juniper mortality (Erdman, 1970; Koniak, 1985). Other forest types showed faster or similar rates of recovery; for instance, mixed conifer recovered completely in most of the ecoregions of the western US, possibly due to richer species diversity and relatively high precipitation (Bright et al., 2019).
4.2 Post-fire albedo changes
Our results provide evidence for significant effects of wildfires on the albedo across forest types and eco-climatic settings in the western US, with post-fire albedo being much higher in winter than in summer. All forest types showed noticeable age-dependent albedo patterns, with a transient peak in summer albedo at around 10–18 years post-fire. Except for pinyon–juniper, we observed a decline in summer albedo during the first year after fire (Table S3), presumably due to the charred surface and the deposition of black carbon. The increase in albedo in the first year after a fire in pinyon–juniper may be associated with a low pre-fire LAI, leading to lower levels of charcoal and black carbon deposition that absorb incoming radiation. Our finding is comparable to previously published findings that report albedo drops in the range of 0.01–0.05 when using MODIS albedo (Jin and Roy, 2005; Randerson et al., 2006; Lyons et al., 2008; Veraverbeke et al., 2012b). The slight differences are likely related to the variability in the domain of each study (e.g., western US vs. boreal, western US vs. Mediterranean), the spatial resolution of MODIS pixels (500 m) that include unburned patches and non-forest fractions, the illumination conditions of the MODIS albedo products (black sky, white sky, blue sky), and the method used to calculate albedo differences. Regarding the latter, we compared a pixel in a pre-fire year to the same pixel in a post-fire year. The approach in which burned pixels are compared to unburned neighboring pixels used as a control is also common (e.g., Myhre et al., 2005; Randerson et al., 2006; Lyons et al., 2008; Gatebe et al., 2014). One issue with this approach is that it does not consider the heterogeneity of the land surface. Burned and control pixels may not be equivalent in the pre-burn period (Dintwe et al., 2017), as the control pixels do not necessarily represent a comparable vegetation state and therefore may not be a good proxy for the pre-fire state.
Soon after fire, we observed an increase in post-fire albedo during the summer period, presumably due to a combination of char removal and the presence of early-successional plants (Johnstone et al., 2010) that have a higher albedo than mature species (Betts and Ball, 1997; Pinty et al., 2000; Amiro et al., 2006; Dintwe et al., 2017). Summer post-fire albedo recovered faster than LAI regardless of vegetation type. This pattern suggests that, in contrast to the findings of Pinty et al. (2000) and Tsuyuzaki et al. (2009), the post-fire recovery of albedo is driven by multiple factors in addition to the early regeneration of vegetation, such as vegetation destruction and the charcoal left behind (Jin et al., 2012), differences in fuel combustion and consumption (Jin and Roy, 2005), species composition during early succession (Beck et al., 2011), and the seasonal variation in soil moisture and the removal of black carbon (Montes-Helu et al., 2009; Veraverbeke et al., 2012b). As the regenerating vegetation matures, the increase in post-fire albedo progressively weakens, as suggested by Amiro et al. (2006), with the peak albedo reached at ∼10–18 years post-fire, after which it gradually declines towards pre-fire levels. We did not observe the complete recovery of the post-fire albedo within the study period of 25 years post-fire. Many studies that used a remote sensing technique have suggested that the albedo in post-fire stands commonly equilibrates at ∼40–80 years post-fire (Randerson et al., 2006; Lyons et al., 2008; Kuusinen et al., 2014; Bright et al., 2015; Halim et al., 2019, Potter et al., 2020).
We found that the greatest increase in post-fire albedo occurred during winter (a finding consistent with others: Liu et al., 2005; Randerson et al., 2006; Montes-Helu et al., 2009; Gleason et al., 2019) due to the increased exposure of snow resulting from the loss of canopy and tree mortality. In our analysis, post-fire winter snow-covered albedo increased with time since fire until a peak was reached, the timing of which varied across forest types. We hypothesize that this increase with time may result from the fall of standing dead snags (O'Halloran et al., 2014) and a lower rate of reestablishment during succession (Fig. S4). Our finding showed similar post-fire winter albedo patterns across forest types in a region. For example, winter albedo in lodgepole pine, spruce–fir–hemlock, and Douglas fir forest types in the Idaho Batholith region increased at a similar rate with time since fire, which corresponds to a consistently lower LAI recovery rate across these forest types in this region (Fig. S4b, f, g). However, the variation in winter albedo was greater across ecoregions within a forest type (e.g., mixed conifer) owing to variable rates of post-fire LAI recovery (Fig. S4a). Overall, our findings indicate a strong dependency of post-fire seasonal albedo on the proportion of vegetative cover, irrespective of forest type, in the post-fire environment. This observed effect provides a strong connection between albedo and successional patterns observed in these specific forest types.
4.3 Controls on the post-fire recovery of biophysical parameters
One of the major contributions of our approach is that it not only generates the post-fire trajectories of land surface biophysical properties across a range of forest types and geographic regions, but it also distinguishes the contributions of the nature of the fire, climate, and topography to the post-fire LAI and albedo recovery for each forest type. Previous work has shown fire severity to be an important driver of regeneration (Crotteau et al., 2013; Meng et al., 2015; Chambers et al., 2016; Vanderhoof et al., 2020). In contrast, our analysis suggested that fire severity was of relatively low importance relative to other variables considered (Fig. S2). Despite being of lesser importance, we found that higher rates of post-fire recovery were associated with low-severity fire, and the lowest recovery rates were associated with high fire severity. The lower recovery rates associated with high fire severity are possibly due to lower seed availability and a greater distance to live seed sources (Haire and McGarigal, 2010; Kemp et al., 2016, 2019), but high fire severity can also create mineral seed beds and free up essential resources such as moisture, light, and nutrients, which promote the growth of vegetation (Gray et al., 2005; Moghaddas et al., 2008). Only oak and pinyon–juniper showed higher recovery rates under high fire severity among the forest types, which is primarily due to rapid regeneration by resprouting in oak (Meng et al., 2018) and colonization by resprouting shrubs in pinyon–juniper (Wangler and Minnich, 1996). The low importance of fire severity in determining post-fire vegetation growth indicates that the variability across a single fire may be outweighed at a regional level by climate and its proxies. It also suggests that, at some sites, the impact of wildfire may be restricted to causing tree mortality under a changing climate, rather than also significantly influencing the post-fire regeneration through its impact on seed availability (Kemp et al., 2019).
Our analysis indicated that, among all the factors considered, elevation had the highest variable importance score in predicting the LAI at 10 years and 20 years post-fire. We found greater rates of vegetation recovery at lower elevations. Less successful recovery at higher elevations is likely associated with cooler temperatures at higher elevations for many of the forest types, and those cool temperatures still appear to limit forest establishment and growth under general warming in the region (Stevens-Rumann et al., 2018). Only pinyon–juniper showed increased recovery with elevation (Figs. 5b and 6b), likely due to relief from the hot, dry conditions at lower elevations but also possibly due to the increasing resistance to invasion with elevation for this forest type (Urza et al., 2017). This suggests that warming temperatures are having a detrimental effect on post-fire regeneration at warmer sites but are not yet promoting post-fire regeneration at cooler sites at all spatial scales (Harvey et al., 2016). Elevation was found to be important, but with opposite directionality, in various studies of post-fire regeneration of conifer forests in the western US (Casady et al., 2010; Rother and Veblen, 2016; Vanderhoof et al., 2020). However, Van Mantgem et al. (2006) reported a strongly negative correlation with seedling density of mixed conifer forests in the Sierra Nevada. In higher-elevation forests such as lodgepole pine, most studies demonstrated increased recovery post-fire (e.g., Harvey et al., 2016), which contrasted with our findings. These findings collectively highlight that there is a large degree of uncertainty around individual forest type responses to post-fire climatic variability.
Our study adds to a growing body of literature emphasizing the importance of climate for post-fire vegetation growth among different forest types (Meng et al., 2015; Buechling et al., 2016; Rother and Veblen, 2017; Hankin et al., 2019; Vanderhoof et al., 2020). Our data suggest that high average summer temperatures and low water availability limit the recovery of LAI at 10 years and 20 years post-fire for these forest types. Drier forests such as oak, ponderosa pine, Douglas fir, and pinyon–juniper were strongly associated with annual precipitation and mean summer temperature, which is consistent with the findings of Meng et al. (2015) and Kemp et al. (2019). Our analysis also suggests that the critical thresholds for annual precipitation and mean summer temperature are 500 mm and 15–20 °C, respectively, in these forest types. Our finding of a higher sensitivity of oak, ponderosa pine, Douglas fir, and pinyon–juniper to annual precipitation and average summer temperature suggests that future increases in temperature and water deficit may affect these forest types more than other forest types. With a trend toward warmer springs and summers in recent decades throughout the western US (Westerling, 2006; Ghimire et al., 2012; IPCC, 2013; Williams et al., 2021), conditions for post-fire vegetation growth and survival are changing, as even a slight increase in water deficit at the drier sites can have adverse effects on tree regeneration (Stevens-Rumann et al., 2018). While a warming temperature has been shown to affect the post-fire regeneration of conifer forests growing at the warmer end of the species distribution, such as Douglas fir and ponderosa pine (Haffey et al., 2018; Kemp et al., 2019), it could promote the rate of post-fire recovery for conifer forests growing at the colder end of the species distribution that were previously limited by frozen soils, cold temperatures, and snow (Stevens-Rumann et al., 2018; Vanderhoof et al., 2020).
Similar to LAI, our results for variable importance in random forests showed a low importance of fire severity compared to other variables in the post-fire recovery of summer albedo at both time horizons (Fig. S3). However, we noticed a difference in albedo change across fire severity classes. For example, we found lower albedo values in low-fire-severity areas compared to medium- and high-severity areas at both time horizons, which is associated with a greater degree of LAI recovery in low-severity areas, as vegetation has a lower albedo than bare areas. Moreover, the lower albedo at 10 years post-fire in high-severity areas compared to medium-severity areas could be due to standing snags absorbing sunlight, given that it takes 5–15 years for just half of the dead snags to fall (Russell et al., 2006). We did not find a significant impact of elevation on post-fire albedo change in these forest types, except for pinyon–juniper and ponderosa pine, which showed a decreased albedo post-fire in response to an increased LAI with elevation. As expected, climate, particularly annual precipitation, was the major determinant of post-fire albedo change. Annual precipitation was found to be highly associated with changes in post-fire albedo in all forest types. Increased precipitation decreased the albedo post-fire, with the most prominent impact of this seen at 20 years post-fire. Annual precipitation impacts the post-fire albedo through two different mechanisms. First, increased annual precipitation is associated with a greater recovery of LAI in these forest types (Fig. 6c) where the mid-age stands replace the initial post-fire establishments, reducing the albedo (Chambers and Chapin, 2002). Second, soil moisture depends on precipitation. With greater precipitation leading to an increased soil water content, we could expect a corresponding decrease in albedo due to darkening of the soil, particularly in open-canopy conditions, where the soil received direct radiation (Montes-Helu et al., 2009). Furthermore, an increase in leaf area within the understory during the wet season could have a similar effect, as reported in Thompson et al. (2004). Regarding temperature, the pattern of albedo recovery did not correspond well to the pattern of LAI recovery at both time horizons in these forest types. Albedo is more elevated over the pre-fire condition in the warmer part of a forest type's range, even in forest types that show a faster recovery of LAI in that warmer domain. We might expect that a higher LAI would be associated with a lower albedo, but evidently the association is not as simple as this, and it might have something to do with species composition rather than simply leaf area. Our results point to the importance of climate patterns as a driver of post-fire summer albedo recovery through their influence on ecological succession in the post-fire environment.
4.4 Significance and limitations of our analysis
Our results should be interpreted in light of four constraints. First, the accuracy of the MODIS product algorithm is dependent on biome-specific values, which, following extensive fire-caused mortality, can introduce additional uncertainty due to the assumption of a fixed land cover type. In addition, we utilized the recovery of MODIS LAI as an indicator of vegetation recovery. One significant limitation of this LAI-based analysis is that it captures some of the aggregate effects of mortality and regrowth but does not fully characterize shifts in species composition and community structure on the ground. Therefore, detailed, intensive field monitoring of the vegetation structure both before and after fires can serve as a valuable complement to LAI-based analysis (Williams et al., 2014). Additionally, incorporating additional remote observations at the species level from the fusion of very-high-spatial-resolution lidar or hyperspectral data (Huesca et al., 2013; Polychronaki et al., 2013; Kane et al., 2014) can further enhance the assessment. Second, in terms of albedo, we used a 500 m MODIS albedo product, which reflects a somewhat larger area (Campagnolo et al., 2016). Each 500 m grid may in fact include a mix of burned and unburned patches, which could result in underestimation of the post-fire albedo. Although the use of MODIS data, with their relatively low spatial resolution, will cause some of the details of fine-scale spatial variability in burn severity, land cover type, and so forth to be missed (Key, 2006), MODIS data have advantages in terms of their higher temporal frequency of sampling, which can be important in post-fire biophysical dynamics (Lhermitte et al, 2010; Veraverbeke et al., 2010, 2012b), and these data also have good temporal coverage going back decades. Furthermore, higher-resolution datasets on biophysical properties are still not operationally available. Third, the quality of our results may be constrained by the accuracy of the fire severity from the MTBS product, as differenced normalized burn ratio (dNBR) is not a perfect metric of severity and may struggle to capture some variations in severity (Roy et al., 2006; De Santis and Chuvieco, 2009). However, several new-generation fire remote sensing products (Csiszar et al., 2014; Parks et al., 2014; Boschetti et al., 2015) have emerged in recent years, and these hold the potential for further improvements in post-fire recovery studies. Finally, post-fire vegetation recovery in burned areas may vary from one location to another due to the influence of several other factors that this study did not cover. To gain a comprehensive understanding of the trajectory of post-fire vegetation recovery, future studies should, in addition to topo-climatic variables, consider species competition, the scorching of the seed bank, the distance to the seed tree, other post-fire disturbances, the physiology of cones, seeds, and seedlings, and the interactions among all influencing drivers in these settings.
Despite these limitations, by aggregating multiple fire events in 21 different sub-ecoregions and arraying observations along a 25-year chronosequence, our results demonstrate the spatial and temporal variability of fire effects on the post-fire environment. Understanding such variability of fire effects and vegetation in space and time is important for achieving a comprehensive understanding of the drivers of natural regeneration and vegetation recovery in post-fire environments (Stevens-Rumann and Morgan, 2019). Our analysis could also help improve the modeling of post-fire recovery pathways by identifying the most important predictors of post-fire recovery and by approximating related thresholds of response. For example, our results suggest a full recovery of LAI in dry, low-elevation forest types like pinyon–juniper, ponderosa pine, and oak within 10 years post-fire when the annual precipitation exceeds the threshold of 500 mm and the average summer temperature is ∼15–20 °C. A quantitative measure of primary controls is needed if efforts to develop realistic post-fire LAI trajectories for ecohydrological modeling studies are to be successful, as suggested by McMichael et al. (2004).
One major significance of our approach and findings is its potential to advance the land surface models (LSMs) embedded in Earth system models (ESMs). Currently, these models lack robust representations of the ecological and biophysical consequences resulting from wildfire events (Lawrence and Chase, 2007; Williams et al., 2009). Modelers could use the pattern of post-fire biophysical dynamics as a function of time since fire that emerged from our data analysis to inform the LSMs and thus more accurately represent biophysical and ecological functions of severely disturbed landscapes.
4.5 Implications of our research
There is mounting evidence of increased extreme fire incidents in the western US due to ongoing climate change (Westerling et al., 2006; Williams et al., 2014), leading to rapid alteration and considerable uncertainty regarding species composition (McDowell et al., 2015) and ecological dynamics (Johnstone et al., 2016). This study provides an estimate of the effect of the post-fire environment on the vegetation and surface albedo balance in the western US. The chronosequence data show clear patterns with time since fire for both biophysical parameters. Our results show that conifer forest ecosystems, particularly Douglas fir and ponderosa pine, are slower to recover post-fire, which may indicate that they face greater risks from the projected increase in fire severity and frequency as forecasted for drier interiors of the western US (Abatzoglou and Williams, 2016; Littell et al., 2018). The post-fire biophysical changes documented here could be of significance for local to regional climates, as they potentially elicit feedbacks that influence regional climate change and adaptation needs.
All of the research input data and codes supporting the results reported in this paper are available in a repository (https://doi.org/10.5281/zenodo.7927852, Shrestha et al., 2023).
The supplement related to this article is available online at: https://doi.org/10.5194/bg-21-2207-2024-supplement.
SS conceptualized and designed the research, curated data, ran the analysis, and wrote a draft. CAW provided substantial input into the research conceptualization, research framework, and polishing of the paper. BMR, JR, and DK offered insight into the paper's data analysis presentation and contributed to the draft paper's finalization.
The contact author has declared that none of the authors has any competing interests.
Publisher's note: Copernicus Publications remains neutral with regard to jurisdictional claims made in the text, published maps, institutional affiliations, or any other geographical representation in this paper. While Copernicus Publications makes every effort to include appropriate place names, the final responsibility lies with the authors.
This paper was edited by Xi Yang and reviewed by two anonymous referees.
Abatzoglou, J. T. and Williams, A. P.: Impact of anthropogenic climate change on wildfire across western US forests, P. Natl. Acad. Sci. USA, 113, 11770–11775, https://doi.org/10.1073/pnas.1607171113, 2016.
Allen, C. D., Breshears, D. D., and McDowell, N. G.: On underestimation of global vulnerability to tree mortality and forest die-off from hotter drought in the Anthropocene, Ecosphere, 6, 1–55, https://doi.org/10.1890/ES15-00203.1, 2015.
Amiro, B. D., Chen, J. M., and Liu, J.: Net primary productivity following forest fire for Canadian ecoregions, Can. J. Forest Res., 30, 939–947, https://doi.org/10.1139/cjfr-30-6-939, 2000.
Amiro, B. D., Orchansky, A. L., Barr, A. G., Black, T. A., Chambers, S. D., Chapin, F. S., Goulden, M. L., Litvak, M., Liu, H. P., McCaughey, J. H., McMillan, A., and Randerson, J. T.: The effect of post-fire stand age on the boreal forest energy balance, Agr. Forest Meteorol., 140, 41–50, https://doi.org/10.1016/j.agrformet.2006.02.014, 2006.
Amiro, B. D., Barr, A. G., Barr, J. G., Black, T. A., Bracho, R., Brown, M., Chen, J., Clark, K. L., Davis, K. J., Desai, A. R., Dore, S., Engel, V., Fuentes, J. D., Goldstein, A. H., Goulden, M. L., Kolb, T. E., Lavigne, M. B., Law, B. E., Margolis, H. A., Martin, T., McCaughey, J. H., Misson, L., Montes-Helu, M., Noormets, A., Randerson, J. T., Starr, G., and Xiao, J.: Ecosystem carbon dioxide fluxes after disturbance in forests of North America, J. Geophys. Res.-Biogeo., 115, G00K02, https://doi.org/10.1029/2010JG001390, 2010.
Bartlein, P. J. and Hostetler, S. W.: Modeling paleoclimates, Dev. Quat. Sci. 1, 565–584, https://doi.org/10.1016/S1571-0866(03)01027-3, 2003.
Barton, A. M.: Intense wildfire in southeastern Arizona: Transformation of a Madrean oak-pine forest to oak woodland, Forest Ecol. Manag., 165, 205–212, https://doi.org/10.1016/S0378-1127(01)00618-1, 2002.
Beck, P. S. A., Goetz, S. J., Mack, M. C., Alexander, H. D., Jin, Y., Randerson, J. T., and Loranty, M. M.: The impacts and implications of an intensifying fire regime on Alaskan boreal forest composition and albedo, Glob. Change Biol., 17, 2853–2866, https://doi.org/10.1111/j.1365-2486.2011.02412.x, 2011.
Besnard, S., Koirala, S., Santoro, M., Weber, U., Nelson, J., Gütter, J., Herault, B., Kassi, J., N'Guessan, A., Neigh, C., Poulter, B., Zhang, T., and Carvalhais, N.: Mapping global forest age from forest inventories, biomass and climate data, Earth Syst. Sci. Data, 13, 4881–4896, https://doi.org/10.5194/essd-13-4881-2021, 2021.
Betts, A. and Ball, J.: Albedo over the boreal forest, J. Geophys.-Res., 102, 28901–28609, https://doi.org/10.1029/96JD03876, 1997.
Bonan, G. B., Chapin, F. S., and Thompson, S. L.: Boreal forest and tundra ecosystems as components of the climate system, Clim. Change, 29, 145–167, https://doi.org/10.1007/BF01094014, 1995.
Bond-Lamberty, B., Peckham, S. D., Gower, S. T., and Ewers, B. E.: Effects of fire on regional evapotranspiration in the central Canadian boreal forest, Glob. Change Biol., 15, 1242–1254, https://doi.org/10.1111/j.1365-2486.2008.01776.x, 2009.
Boschetti, L., Roy, D. P., Justice, C. O., and Humber, M. L.: MODIS–Landsat fusion for large area 30 m burned area mapping, Remote Sens. Environ., 161, 27–42, 2015.
Breiman, L.: Random forests, Mach. Learn., 45, 5–32, https://doi.org/10.1023/A:1010933404324, 2001.
Bright, B. C., Hudak, A. T., Kennedy, R. E., Braaten, J. D., and Henareh Khalyani, A.: Examining post-fire vegetation recovery with Landsat time series analysis in three western North American forest types, Fire Ecol., 15, 8, https://doi.org/10.1186/s42408-018-0021-9, 2019.
Bright, R. M., Zhao, K., Jackson, R. B., and Cherubini, F.: Quantifying surface albedo and other direct biogeophysical climate forcings of forestry activities, Glob. Change Biol., 21, 3246–3266, https://doi.org/10.1111/gcb.12951, 2015.
Brown, P. M. and Wu, R.: Climate and disturbance forcing of episodic tree recruitment in a Southwestern ponderosa pine landscape, Ecology, 86, 3030–3038, https://doi.org/10.1890/05-0034, 2005.
Buechling, A., Martin, P. H., Canham, C. D., Shepperd, W. D., Battaglia, M. A., and Rafferty, N.: Climate drivers of seed production in picea engelmannii and response to warming temperatures in the Southern Rocky Mountains, J. Ecol., 104, 1051–1062, https://doi.org/10.1111/1365-2745.12572, 2016.
Campagnolo, M. L., Sun, Q., Liu, Y., Schaaf, C., Wang, Z., and Román, M. O.: Estimating the effective spatial resolution of the operational BRDF, albedo, and nadir reflectance products from MODIS and VIIRS, Remote Sens. Environ., 175, 52–64, https://doi.org/10.1016/j.rse.2015.12.033, 2016.
Campbell, J., Donato, D., Azuma, D., and Law, B.: Pyrogenic carbon emission from a large wildfire in Oregon, United States, J. Geophys. Res., 112, G04014, https://doi.org/10.1029/2007JG000451, 2007.
Cansler, C. A. and Mckenzie, D.: Climate, fire size, and biophysical setting control fire severity and spatial pattern in the northern Cascade Range, USA, Ecol. Appl., 24, 1037–1056, https://doi.org/10.1890/13-1077.1, 2014.
Casady, G. M., van Leeuwen, W. J. D., and Marsh, S. E.: Evaluating Post-wildfire Vegetation Regeneration as a Response to Multiple Environmental Determinants, Environ. Model. Assess., 15, 295–307, https://doi.org/10.1007/s10666-009-9210-x, 2010.
Chambers, M. E., Fornwalt, P. J., Malone, S. L., and Battaglia, M. A.: Patterns of conifer regeneration following high severity wildfire in ponderosa pine-dominated forests of the Colorado Front Range, Forest Ecol. Manag., 378, 57–67, 2016.
Chambers, S. D. and Chapin III, F. S.: Fire effects on surface-atmosphere energy exchange in Alaskan black spruce ecosystems Fire effects on surface-atmosphere energy exchange in Alaskan black spruce ecosystems: Implications for feedbacks to regional climate, J. Geophys. Res., 107, 8145, https://doi.org/10.1029/2001JD000530, 2002.
Chambers, S. D., Beringer, J., Randerson, J. T., and Chapin, I. S.: Fire effects on net radiation and energy partitioning: Contrasting responses of tundra and boreal forest ecosystems, J. Geophys. Res., 110, 1–9, https://doi.org/10.1029/2004JD005299, 2005.
Chappell, C. B. and Agee, J. K.: Fire severity and tree seedling establishment in Abies magnifica forests, southern Cascades, Oregon, Ecol. Appl., 6, 628–640, https://doi.org/10.2307/2269397, 1996.
Chen, X., Vogelmann, J. E., Rollins, M., Ohlen, D., Key, C. H., Yang, L., Huang, C., and Shi, H.: Detecting post-fire burn severity and vegetation recovery using multitemporal remote sensing spectral indices and field-collected composite burn index data in a ponderosa pine forest, Int. J. Remote Sens., 32, 7905–7927, https://doi.org/10.1080/01431161.2010.524678, 2011.
Cohen, W. B., Maiersperger, T. K., Turner, D. P., Ritts, W. D., Pflugmacher, D., Kennedy, R. E., Kirschbaum, A., Running, S. W., Costa, M., and Gower, S. T.: MODIS land cover and LAI collection 4 product quality across nine sites in the western hemisphere, IEEE T. Geosci. Remote, 44, 1843–1857, https://doi.org/10.1109/TGRS.2006.876026, 2006.
Crotteau, J. S., Varner III, J. M., and Ritchie, M. W.: Post-fire regeneration across a fire severity gradient in the southern Cascades, Forest Ecol. Manag., 287, 103–112, https://doi.org/10.1016/j.foreco.2012.09.022, 2013.
Csiszar, I., Schroeder, W., Giglio, L., Ellicott, E., Vadrevu, K. P., Justice, C. O., and Wind, B.: Active fires from the Suomi NPP Visible Infrared Imaging Radiometer Suite: Product status and first evaluation results, J. Geophys. Res.-Atmos., 119, 803–881, 2014.
Dale, V. H., Joyce, L. A., Mcnulty, S., Neilson, R. P., Ayres, M. P., Flannigan, M. D., Hanson, P. J., Irland, L. C., Ariel, E., Peterson, C. J., Simberloff, D., Swanson, F. J., Stocks, B. J., Wotton, B. M., Dale, V. H., Joyce, L. A., Mcnulty, S., Ronald, P., Matthew, P., Simberloff, D., Swanson, F. J., Stocks, B. J., and Wotton, B. M.: Climate Change and Forest Disturbances, Bioscience, 51, 723–734, https://doi.org/10.1641/0006-3568(2001)051[0723:CCAFD]2.0.CO;2, 2001.
Daly, C., Neilson, R. P., and Phillips, D. L.: A statistical-topographic model for mapping climatological precipitation over mountainous terrain, J. Appl. Meteorol., 33, 140–158, 1994.
Daly, C., Halbleib, M., Smith, J. I., Gibson, W. P., Doggett, M. K., Taylor, G. H., Curtis, J., and Pasteris, P. A.: Physiographically-sensitive mapping of temperature and precipitation across the conterminous United States, Int. J. Climatol., 28, 2031–2064, 2008.
De Sales, F., Okin, G. S., Xue, Y., and Dintwe, K.: On the effects of wildfires on precipitation in southern Africa, Clim. Dynam., 52, 951–967, https://doi.org/10.1007/s00382-018-4174-7, 2018.
De Santis, A. and Chuvieco, E.: GeoCBI: A modified version of the Composite Burn Index for the initial assessment of the short-term burn severity from remotely sensed data, Remote Sens. Environ., 113, 554–562, 2009.
Dennison, P. E., Brewer, S. C., Arnold, J. D., and Moritz, M. A.: Large wildfire trends in the western United States, 1984–2011, Geophys. Res. Lett., 41, 2928–2933, https://doi.org/10.1002/2014GL059576, 2014.
Dintwe, K., Okin, G. S., and Xue, Y.: Fire-induced albedo change and surface radiative forcing in sub-Saharan Africa savanna ecosystems: Implications for the energy balance, J. Geophys. Res., 122, 6186–6201, https://doi.org/10.1002/2016JD026318, 2017.
Dore, A. S., Kolb, T. E., Eckert, S. E., Sullivan, B. W., Hungate, B. A., Kaye, J. P., Hart, S. C., Koch, G. W., Finkral, A., Applications, S. E., April, N., Dore, S., Kolb, T. E., Eckert, S. E., Sullivan, W., Hungate, B. A., and Kaye, J. P.: Carbon and water fluxes from ponderosa pine forests disturbed by wildfire and thinning, Ecol. Appl., 20, 663–683, 2010.
Downing, W. M., Krawchuk, M. A., Meigs, G. W., Haire, S. L., Coop, J. D., Walker, R. B., Whitman, E., Chong, G., and Miller, C.: Influence of fire refugia spatial pattern on post-fire forest recovery in Oregon's Blue Mountains, Landscape Ecol., 34, 771–792, https://doi.org/10.1007/s10980-019-00802-1, 2019.
Dwyer, D. D. and Pieper, R. D.: Fire effects on blue gramma-piny on-juniper rangeland in New Mexico, J. Range Manage., 20, 359–362, 1967.
Eidenshink, J., Schwind, B., Brewer, K., Zhu, Z., Quayle, B., Howard, S., Falls, S., and Falls, S.: A project for monitoring trends in burn severity, Fire Ecology Special Issue 3, 3–21, 2007.
Epting, J. and Verbyla, J.: Landscape-level interactions of prefire vegetation, burn severity, and postfire vegetation over a 16-year period in interior Alaska, Can. J. Forest Res., 35, 1367–1377, https://doi.org/10.1139/X05-060, 2005.
Erdman, J. A.: Pinyon-juniper succession alter natural hres on residual soils of Mesa Verde, Colorado, BYU Science Bulletin in Biology Series, 11, 1970.
Ferguson, D. E. and Carlson, C. E.: Height-age relationships for regeneration-size trees in the northern Rocky Mountains, USA, Research Paper RMRS-RP-82WWW, USDA Forest Service, Rocky Mountain Research Station, Fort Collins, Colorado, USA, 2010.
Frazier, R. J., Coops, N. C., Wulder, M. A., Hermosilla, T., and White, J. C.: Analyzing spatial and temporal variability in short-term rates of post-fire vegetation return from Landsat time series, Remote Sens. Environ., 205, 32–45, 2018.
Gao, F., Schaaf, C. B., Strahler, A. H., Roesch, A., Lucht, W., and Dickinson, R.: MODIS bidirectional reflectance distribution function and albedo Climate Modeling Grid products and the variability of albedo major global vegetation types, J. Geophys. Res.-Atmos. 110, 1–13, https://doi.org/10.1029/2004JD005190, 2005.
Gatebe, C. K., Ichoku, C. M., Poudyal, R., Román, M. O., and Wilcox, E.: Surface albedo darkening from wildfires in northern sub-Saharan Africa, Environ. Res. Lett., 9, 065003, https://doi.org/10.1088/1748-9326/9/6/065003, 2014.
Ghimire, B., Williams, C. A., Collatz, G. J., and Vanderhoof, M.: Fire-induced carbon emissions and regrowth uptake in western U.S. forests: Documenting variation across forest types, fire severity, and climate regions, J. Geophys. Res., 117, G03036, https://doi.org/10.1029/2011JG001935, 2012.
Gleason, K. E., McConnell, J. R., Arienzo, M. M., Chellman, N., and Calvin, W. M.: Four-fold increase in solar forcing on snow in western U.S. burned forests since 1999, Nat. Commun., 10, 1–8, https://doi.org/10.1038/s41467-019-09935-y, 2019.
Gray, A. N., Zald, H. S., Kern, R. A., and North, M.: Stand conditions associated with tree regeneration in Sierran mixed-conifer forests, Forest Sci., 51, 198–210, 2005.
Guz, J., Gill, N. S., and Kulakowski, D.: Long-term empirical evidence shows post-disturbance climate controls post-fire regeneration, J. Ecol., 109, 4007–4024, https://doi.org/10.1111/1365-2745.13771, 2021.
Haffey, C., Sisk, T. D., Allen, C. D., Thode, A. E., and Margolis, E. Q.: Limits to Ponderosa Pine Regeneration following Large High-Severity Forest Fires in the United States Southwest, Fire Ecol., 14, 143–163, https://doi.org/10.4996/fireecology.140114316, 2018.
Haire, S. L. and McGarigal, K.: Effect of landscape patterns of fire severity on regenerating ponderosa pine forests (Pinus ponderosa) in New Mexico and Arizona, USA, Landscape Ecol., 25, 1055–1069, 2010.
Halim, M. A., Chen, H. Y. H., and Thomas, S. C.: Stand age and species composition effects on surface albedo in a mixedwood boreal forest, Biogeosciences, 16, 4357–4375, https://doi.org/10.5194/bg-16-4357-2019, 2019.
Hankin, L. E., Higuera, P. E., Davis, K. T., and Dobrowski, S. Z.: Impacts of growing-season climate on tree growth and post-fire regeneration in ponderosa pine and Douglas-fir forests, Ecosphere, 10, e02679, https://doi.org/10.1002/ecs2.2679, 2019.
Hansen, W. D., Romme, W. H., Ba, A., and Turner, M. G.: Shifting ecological filters mediate postfire expansion of seedling aspen (Populus tremuloides) in Yellowstone, Forest Ecol. Manage., 362, 218–230, 2016.
Hartsell, J. A., Copeland, S. M., Munson, S. M., Butterfield, B. J., and Bradford, J. B.: Gaps and hotspots in the state of knowledge of pinyon-juniper communities, Forest Ecol. Manage., 455, 1–23, 2020.
Harvey, B. J., Donato, D. C., and Turner, M. G.: High and dry: postfire tree seedling establishment in subalpine forests decreases with post-fire drought and large stand-replacing burn patches, Global Ecol. Biogeogr., 25, 655–669, https://doi.org/10.1111/geb.12443, 2016.
Hicke, J. A., Asner, G. P., Kasischke, E. S., French, N. H. F., Randerson, J. T., Collatz, G. J., Stocks, B. J., Tucker, C. J., Los, S. O., and Field, C. B.: Postfire response of North American boreal forest net primary productivity analyzed with satellite observations, Glob. Change Biol., 9, 1145–1157, https://doi.org/10.1046/j.1365-2486.2003.00658.x, 2003.
Hislop, S., Haywood, A., Jones, S., Soto-Berelov, M., Skidmore, A., and Nguyen, T. H.: A satellite data driven approach to monitoring and reporting fire disturbance and recovery across boreal and temperate forests, Int. J. Appl. Earth Obs., 87, 102034, https://doi.org/10.1016/j.jag.2019.102034, 2020.
Howard, J. L.: Pinus ponderosa var. brachyptera, P. p. var. scopulorum. Fire Effects Information System. US Department of Agriculture, Forest Service, Rocky Mountain Research Station, Fire Sciences Laboratory, Missoula, Montana, USA, https://www.fs.usda.gov/database/feis/plants/tree/pinpons/all.html (last access: 8 January 2022), 2003.
Huesca, M., Merino-de-Miguel, S., González-Alonso, F., Martínez, S., Miguel Cuevas, J., and Calle, A.: Using AHS hyper-spectral images to study forest vegetation recovery after a fire, Int. J. Remote Sens., 34, 4025–4048, 2013.
IPCC [Intergovernmental Panel on Climate Change]: Climate change 2007: Synthesis report, Intergovernmental Panel on Climate Change, 2007.
IPCC [Intergovernmental Panel on Climate Change]: Climate change 2013: the physical science basis, in: Contribution of Working Group I to the fifth assessment report of the Intergovernmental Panel on Climate Change, edited by: Stocker, T. F., Qin, D., Plattner, G.-K., Tignor, M., Allen, S. K., Boschung, J., Nauels, A., Xia, Y., Bex, V., and Midgley, P. M., Cambridge University Press, Cambridge, England, United Kingdom, and New York, New York, USA, 1–1535, 2013.
Jameson, D. A.: Effects of burning on galleta-black gramma range invaded by juniper, Ecology, 43, 760–763, 1962.
Jin, Y. and Roy, D. P.: Fire-induced albedo change and its radiative forcing at the surface in northern Australia, Geophys. Res. Lett., 32, 1–4, https://doi.org/10.1029/2005GL022822, 2005.
Jin, Y., Randerson, J. T., Goetz, S. J., Beck, P. S. A., Loranty, M. M., and Goulden, M. L.: The influence of burn severity on postfire vegetation recovery and albedo change during early succession in North American boreal forests, J. Geophys. Res.-Biogeo., 117, 1–15, https://doi.org/10.1029/2011JG001886, 2012.
Johnstone, J. F., Hollingsworth, T. N., Chapin, F. S., and Mack, M. C.: Changes in fire regime break the legacy lock on successional trajectories in Alaskan boreal forest, Glob. Change Biol., 16, 1281–1295, https://doi.org/10.1111/j.1365-2486.2009.02051.x, 2010.
Johnstone, J. F., Allen, C. D., Franklin, J. F., Frelich, L. E., Harvey, B. J., Higuera, P. E., Mack, M. C., Meentemeyer, R. K., Metz, M. R., Perry, G. L., Schoennagel, T., and Turner, M. G.: Changing disturbance regimes, ecological memory, and forest resilience, Front. Ecol. Environ., 14, 369–378, https://doi.org/10.1002/fee.1311, 2016.
Kane, V. R., North, M. P., Lutz, J. A., Churchill, D. J., Roberts, S. L., Smith, D. F., McGaughey, R. J., Kane, J. T., and Brooks, M. L.: Assessing fire effects on forest spatial structure using a fusion of Landsat and airborne LiDAR data in Yosemite National Park, Remote Sens. Environ., 151, 89–101, https://doi.org/10.1016/j.rse.2013.07.041, 2014.
Keeley, J. E., Brennan, T., and Pfaff, A. H.: Fire severity and ecosytem responses following crown fires in California shrublands, Ecol. Appl., 18, 1530–1546, https://doi.org/10.1890/07-0836.1, 2008.
Kemp, K. B., Higuera, P. E., and Morgan, P.: Fire legacies impact conifer regeneration across environmental gradients in the US northern Rockies, Landscape Ecol., 31, 619–636, https://doi.org/10.1007/s10980-015-0268-3, 2016.
Kemp, K. B., Higuera, P. E., Morgan, P., and Abatzoglou, J. T.: Climate will increasingly determine post-fire tree regeneration success in low-elevation forests, Northern Rockies, USA, Ecosphere, 10, 17, https://doi.org/10.1002/ecs2.2568, 2019.
Key, C.: Ecological and sampling constraints on defining landscape fire severity, Fire Ecol., 2, 34–59, https://doi.org/10.4996/FIREECOLOGY, 2006.
Koniak, S.: Succession in pinyon-juniper woodlands following wildfire in the Great Basin, Great Basin Nat., 45, 556–566, 1985.
Kuusinen, N., Tomppo, E., Shuai, Y., and Berninger, F.: Effects of forest age on albedo in boreal forests estimated from MODIS and Landsat albedo retrievals, Remote Sens. Environ., 145, 145–153, https://doi.org/10.1016/j.rse.2014.02.005, 2014.
Lawrence, P. J. and Chase, T. N.: Representing a new MODIS consistent land surface in the Community Land Model (CLM 3.0), J. Geophys. Res.-Biogeo., 112, G01023, https://doi.org/10.1029/2006JG000168, 2007.
Lhermitte, S., Verbesselt, J., Verstraeten, W. W., and Coppin, P.: A pixel-based regeneration index using time series similarity and spatial context, Photogramm. Eng. Rem. S., 76, 673–682, 2010.
Liaw, A. and Wiener, M.: Classification and regression by random forest, R News, 2, 18–22, 2002.
Lippok, D., Beck, S. G., Renison, D., Gallegos, S. C., Saavedra, F. V., Hensen, I., and Schleuning, M.: Forest recovery of areas deforested by fire increases with elevation in the tropical Andes, Forest Ecol. Manage., 295, 69–76, https://doi.org/10.1016/j.foreco.2013.01.011, 2013.
Littell, J. S., Mckenzie, D., Peterson, D. L., and Westerling, A. L.: Climate and wildfire area burned in western U.S. ecoprovinces, Ecol. Appl., 19, 1003–1021, 2009.
Littell J. S., Mckenzie, D., Wan, H. Y., and Cushman, S. A.: Climate change and future wildfire in the Western United States: an ecological approach to nonstationarity, Earth's Future, 6, 1097–111, 2018.
Littlefield, C. E., Dobrowskia, S. Z., Abatzoglouc, J. T., Parksd, S. A., and Davise, K. T.: A climatic dipole drives short- and long-term patterns of postfire forest recovery in the western United States, P. Natl. Acad. Sci. USA, 117, 29730–29737, https://doi.org/10.1073/pnas.2007434117, 2020.
Liu, H., Randerson, J. T., Lindfors, J., and Iii, F. S. C.: Changes in the surface energy budget after fire in boreal ecosystems of interior Alaska: An annual perspective, J. Geophys. Res.-Atmos., 110, 1–12, https://doi.org/10.1029/2004JD005158, 2005.
Liu, Z.: Effects of climate and fire on short-term vegetation recovery in the boreal larch forests of northeastern China, Sci. Rep., 6, 37572, https://doi.org/10.1038/srep37572, 2016.
Lyons, E. A., Jin, Y., and Randerson, J. T.: Changes in surface albedo after fire in boreal forest ecosystems of interior Alaska assessed using MODIS satellite observations, J. Geophys. Res.-Biogeo., 113, 1–15, https://doi.org/10.1029/2007JG000606, 2008.
Ma, Q., Bales, R. C., Rungee, J., Conklin, M. H., Collins, B. M., and Goulden, M. L.: Wildfire controls on evapotranspiration in California's Sierra Nevada, J. Hydrol., 590, 125364, https://doi.org/10.1016/j.jhydrol.2020.125364, 2020.
Maina, F. Z. and Siirila-Woodburn, E. R.: Watersheds dynamics following wildfires: Nonlinear feedbacks and implications on hydrologic responses, Hydrol. Process., 34, 33–50, https://doi.org/10.1002/hyp.13568, 2019.
Martin, D. P.: Partial dependence plots, http://dpmartin42.github.io/posts/r/partial-dependence (last access: 8 May 2022), 2014.
Martín-Alcon, S. and Coll, L.: Unraveling the relative importance of factors driving post-fire regeneration trajectories in non-serotinous Pinus nigra forests, Forest Ecol. Manage., 361, 13–22, 2016.
McDowell, N. G., Williams, A. P., Xu, C., Pockman, W. T., Dickman, L. T., Sevanto, S., Pangle, R., Limousin, J. M., Plaut, J., Mackay, D. S., Ogee, J., Domec, J. C., Allen, C. D., Fisher, R. A., Jiang, X., Muss, J. D., Breshears, D. D., Rauscher, S. A., and Koven, C.: Multi-scale predictions of massive conifer mortality due to chronic temperature rise, Nat. Clim. Change, 6, 295–300, https://doi.org/10.1038/nclimate2873, 2015.
McMichael, C. E., Hope, A. S., Roberts, D. A., and Anaya, M. R.: Post-fire recovery of leaf area index in California chaparral: A remote sensing-chronosequence approach, Int. J. Remote Sens., 25, 4743–4760, https://doi.org/10.1080/01431160410001726067, 2004.
Meigs, G. W., Donato, D. C., Campbell, J. L., Martin, J. G., and Law, B. E.: Forest fire impacts on carbon uptake, storage, and emission: The role of burn severity in the Eastern Cascades, Oregon, Ecosystems, 12, 1246–1267, https://doi.org/10.1007/s10021-009-9285-x, 2009.
Meng, R., Dennison, P. E., D'Antonio, C. M., and Moritz, M. A.: Remote sensing analysis of vegetation recovery following short-interval fires in Southern California Shrublands, PLoS One, 9, 14–17, https://doi.org/10.1371/journal.pone.0110637, 2014.
Meng, R., Dennison, P. E., Huang, C., Moritz, M. A., and D'Antonio, C.: Effects of fire severity and post-fire climate on short-term vegetation recovery of mixed-conifer and red fir forests in the Sierra Nevada Mountains of California, Remote Sens. Environ., 171, 311–325, https://doi.org/10.1016/j.rse.2015.10.024, 2015.
Meng, R., Wu, J., Zhao, F., Cook, B. D., Hanavan, R. P., and Serbin, S. P.: Measuring short-term post-fire forest recovery across a burn severity gradient in a mixed pine-oak forest using multi-sensor remote sensing techniques, Remote Sens. Environ., 210, 282–296, https://doi.org/10.1016/j.rse.2018.03.019, 2018.
Micheletty, P. D., Kinoshita, A. M., and Hogue, T. S.: Application of MODIS snow cover products: wildfire impacts on snow and melt in the Sierra Nevada, Hydrol. Earth Syst. Sci., 18, 4601–4615, https://doi.org/10.5194/hess-18-4601-2014, 2014.
Moghaddas, J. J., York, R. A., and Stephens, S. L.: Initial response of conifer and California black oak seedlings following fuel reduction activities in a Sierra Nevada mixed conifer forest, Forest Ecol. Manage., 255, 3141–3150, 2008.
Montes-Helu, M. C., Kolb, T., Dore, S., Sullivan, B., Hart, S. C., Koch, G., and Hungate, B. A.: Persistent effects of fire-induced vegetation change on energy partitioning and evapotranspiration in ponderosa pine forests, Agr. Forest Meteorol., 149, 491–500, https://doi.org/10.1016/j.agrformet.2008.09.011, 2009.
Morresi, D., Vitali, A., Urbinati, C., and Garbarino, M.: Forest spectral recovery and regeneration dynamics in stand replacing wildfires of central Apennines derived from Landsat time series, Remote Sens., 11, 308, 1–18, 2019.
Myhre, G., Kvalevåg, M. M., and Schaaf, C. B.: Radiative forcing due to anthropogenic vegetation change based on MODIS surface albedo data, Geophys. Res. Lett., 32, L21410, https://doi.org/10.1029/2005GL024004, 2005.
Myneni, R. B., Hoffman, S., Knyazikhin, Y., Privette, J. L., Glassy, J., Tian, Y., Wang, Y., Song, X., Zhang, Y., Smith, G. R., Lotsch, A., Friedl, M., Morisette, J. T., Votava, P., Nemani, R. R., and Running, S. W.: Global products of vegetation leaf area and fraction absorbed PAR from year one of MODIS data, Remote Sens. Environ., 83, 214–231, https://doi.org/10.1016/S0034-4257(02)00074-3, 2002.
O'Halloran, T. L., Acker, S. A., Joerger, V. M., Kertis, J., and Law, B. E.: Postfire influences of snag attrition on albedo and radiative forcing, Geophys. Res. Lett., 41, 9135–9142, https://doi.org/10.1002/2014GL062024, 2014.
Omernik, J. M.: Ecoregions of the Conterminous United States, Ann. Assoc. Am. Geogr., 77, 118–125, https://doi.org/10.1111/j.1467-8306.1987.tb00149.x, 1987.
Parks, S. A., Dillon, G. K., and Miller, C.: A new metric for quantifying burn severity: the Relativized Burn Ratio, Remote Sens., 6, 1827–1844, 2014.
Pinty, B., Verstraete, M. M., Gobron, N., Govaerts, Y., and Roveda, F.: Do human-induced fires affect the Earth surface reflectance at continental scales?, EOS Trans. Am. Geophys. Union, 81, 381–389, 2000.
Polychronaki, A., Gitas, I. Z., and Minchella, A.: Monitoring post-fire vegetation recovery in the Mediterranean using SPOT and ERS imagery, Int. J. Wildland Fire, 23, 631–642, 2013.
Potter, S., Solvik, K., Erb, A., Goetz, S. J., Johnstone, J. F., Mack, M. C., Randerson, J. T., Roman, M. O., Schaaf, C. L., Turetsky, M. R., Veraverbeke, S., Walker, X. J., Wang, Z., Massey, R., and Rogers, B. M.: Climate change decreases the cooling effect from postfire albedo in boreal North America, Glob. Change Biol., 26, 1592–1607, https://doi.org/10.1111/gcb.14888, 2020.
Randerson, J. T., Liu, H., Flanner, M. G., Chambers, S. D., Jin, Y., Hess, P. G., Pfister, G., Mack, M. C., Treseder, K. K., Welp, L. R., Chapin, F. S., Harden, J. W., Goulden, M. L., Neff, J. C., Schuur, E. A. G., and Zender, C. S.: The impact of Boreal forest fire on climate warming, Science, 314, 1130, https://doi.org/10.1126/science.1132075, 2006.
Roche, J. W., Goulden, M. L., and Bales, R. C.: Estimating evapotranspiration change due to forest treatment and fire at the basin scale in the Sierra Nevada, California, Ecohydrology, 11, e1978, https://doi.org/10.1002/eco.1978, 2018.
Rodman, K. C., Veblen, T. T., Chapman, T. B., Rother, M. T., Wion, A. P., and Redmond, M. D.: Limitations to recovery following wildfire in dry forests of southern Colorado and northern New Mexico, USA, Ecol. Appl., 30, e02001, https://doi.org/10.1002/eap.2001, 2020.
Rodrigo, A., Retana, J., and Picó, F. X.: Direct regeneration is not the only response of Mediterranean forests to large fires, Ecology, 85, 716–729, 2004.
Knox, K. J. E. and Clarke, P. J.: Fire severity, feedback effects and resilience to alternative community states in forest assemblages, Forest Ecol. Manage., 265, 47–54, 2012.
Rogers, B. M., Neilson, R. P., Drapek, R., Lenihan, J. M., Wells, J. R., Bachelet, D., and Law, B. E.: Impacts of climate change on fire regimes and carbon stocks of the U.S. Pacific Northwest, J. Geophys. Res.-Biogeo., 116, 1–13, https://doi.org/10.1029/2011JG001695, 2011.
Rogers, B. M., Randerson, J. T., and Bonan, G. B.: High-latitude cooling associated with landscape changes from North American boreal forest fires, Biogeosciences, 10, 699–718, https://doi.org/10.5194/bg-10-699-2013, 2013.
Rogers, B. M., Soja, A. J., Goulden, M. L., and Randerson, J. T.: Influence of tree species on continental differences in boreal fires and climate feedbacks, Nat. Geosci., 8, 228–234, https://doi.org/10.1038/ngeo2352, 2015.
Rother, M. T. and Veblen, T. T.: Limited conifer regeneration following wildfires in dry ponderosa pine forests of the Colorado Front Range, Ecosphere, 7, 17, https://doi.org/10.1002/ecs2.1594, 2016.
Rother, M. T. and Veblen, T. T.: Climate drives episodic conifer establishment after fire in dry ponderosa pine forests of the Colorado Front Range, USA, Forests, 8, 1–14, https://doi.org/10.3390/f8050159, 2017.
Roy, D. P., Boschetti, L., and Trigg, S. N.: Remote sensing of fire severity: assessing the performance of the normalized burn ratio, IEEE Geosci. Remote Sens., 3, 112–116, 2006.
Ruefenacht, B., Finco, M., Czaplewski, R., Helmer, E., Blackard, J., Holden, G., Lister, A., Salajanu, D., Weyermann, D., and Winterberger, K.: Conterminous US and Alaska forest type mapping using forest inventory and analysis data, Photogramm. Eng. Rem. S., 74, 1379–1388, 2008.
Russell, R. E., Saab, V. A., Dudley, J. G., and Rotella, J. J.: Snag longevity in relation to wildfire and postfire salvage logging, Forest Ecol. Manage., 232, 179–187, 2006.
Salomonson, V. V. and Appel, I.: Estimating fractional snow cover from MODIS using the normalized difference snow index, Remote Sens. Environ., 89, 351–360, https://doi.org/10.1016/j.rse.2003.10.016, 2004.
Savage, M., Brown, P. M., and Feddema, J.: The role of climate in a pine forest regeneration pulse in the southwestern United States, Ecoscience, 3, 310–318, 1996.
Schaaf, C. B., Gao, F., Strahler, A. H., Lucht, W., Li, X., Tsang, T., Strugnell, N. C., Zhang, X., Jin, Y., Muller, J., Lewis, P., Barnsley, M., Hobson, P., Disney, M., Roberts, G., Dunderdale, M., Doll, C., Robert, P., Hu, B., Liang, S., Privette, J. L., and Roy, D.: First operational BRDF, albedo nadir reflectance products from MODIS, Remote Sens. Environ., 83, 135–148, 2002.
Scholze, M., Knorr, W., Arnell, N. W., and Prentice, I. C.: A climate-change risk analysis for world ecosystems, P. Natl. Acad. Sci. USA, 103, 13116–13120, 2006.
Seastedt, T. R., Hobbs, R. J., and Suding, K. N.: Management of novel ecosystems: Are novel approaches required?, Front. Ecol. Environ., 6, 547–553, 2008.
Shrestha, S., Williams, C. A., Rogers, B. M., Rogan, J., and Kulakowski, D.: Wildfire controls on land surface properties in mixed conifer and ponderosa pine forests of Sierra Nevada and Klamath mountains, Western US, Agr. Forest Meteorol., 320, 108939, https://doi.org/10.1016/j.agrformet.2022.108939, 2022.
Shrestha, S., Williams, C. A., Rogan, J., Kulakowski, D., and Rogers, B.: Forest types show divergent biophysical responses after fire: challenges to ecological modeling, Zenodo [code, data set], https://doi.org/10.5281/zenodo.7927852, 2023.
Stevens-Rumann, C. S., Kemp, K. B., Higuera, P. E., Harvey, B. J., Rother, M. T., Donato, D. C., Morgan, P., and Veblen, T. T.: Evidence for declining forest resilience to wildfires under climate change, Ecol. Lett., 21, 243–252, https://doi.org/10.1111/ele.12889, 2018.
Stevens-Rumann, C. S. and Morgan, P.: Tree regeneration following wildfires in the western US: a review, Fire Ecol., 15, 1–17, 2019.
Stoddard, M. T., Huffman, D. W., Fulé, P. Z., Crouse, J. E., and Meador, A. J. S.: Forest structure and regeneration responses 15 years after wildfire in a ponderosa pine and mixed-conifer ecotone, Arizona, USA, Fire Ecol., 14, 1–12, https://doi.org/10.1186/s42408-018-0011-y, 2018.
Strobl, C., Boulesteix, A.-L., Zeileis, A., and Hothorn, T.: Bias in random forest variable importance measures: Illustrations, sources and a solution, BMC Bioinformatics, 8, 25, https://doi.org/10.1186/1471-2105-8-25, 2007.
Thompson, C., Beringer, J., Chapin, F. S., and McGuire, A. D.: Structural complexity and land-surface energy exchange along a gradient from arctic tundra to boreal forest, J. Veg. Sci., 15, 397–406, https://doi.org/10.1111/j.1654-1103.2004.tb02277.x, 2004.
Thompson, R. S., Shafer, S. L., Strickland, L. E., Van de Water, P. K., and Anderson, K. H.: Quaternary vegetation and climate change in the western United States: Developments, perspectives, and prospects, Dev. Quat. Sci., 1, 403–426, https://doi.org/10.1016/S1571-0866(03)01018-2, 2003.
Tsuyuzaki, S., Kushida, K., and Kodama, Y.: Recovery of surface albedo and plant cover after wildfire in a Picea mariana forest in interior Alaska, Climatic Change, 93, 517–525, https://doi.org/10.1007/S10584-008-9505-Y, 2009.
Urza, A. K., Weisberg, P. J., Chambers, J. C., Dhaemers, J. M., and Board, D.: Post-fire vegetation response at the woodland–shrubland interface is mediated by the pre-fire community, Ecosphere, 8, e01851, https://doi.org/10.1002/ecs2.1851, 2017.
U.S. Geological Survey.: 3D Elevation Program 30-Meter Resolution Digital Elevation Model, https://www.usgs.gov/the-national-map-data-delivery (30 December 2019), 2019.
Van Mantgem, P. J., Stephenson, N. L., and Keeley, J. E.: Forest reproduction along a climatic gradient in the Sierra Nevada, California, Forest Ecol. Manage., 225, 391–399, https://doi.org/10.1016/j.foreco.2006.01.015, 2006.
Vanderhoof, M. K., Hawbaker, T. J., Ku, A., Merriam, K., Berryman, E., and Cattau, M.: Tracking rates of postfire conifer regeneration vs. deciduous vegetation recovery across the western United States, Ecol. Appl., 31, e02237, https://doi.org/10.1002/eap.2237, 2020.
Veraverbeke, S., Lhermitte, S., Verstraeten, W. W., and Goossens, R.: The temporal dimension of differenced Normalized Burn Ratio (dNBR) fire/burn severity studies: The case of the large 2007 Peloponnese wildfires in Greece, Remote Sens. Environ., 114, 2548–2563, https://doi.org/10.1016/j.rse.2010.05.029, 2010.
Veraverbeke, S., Gitas, I., Katagis, T., Polychronaki, A., Somers, B., and Goossens, R.: Assessing post-fire vegetation recovery using red-near infrared vegetation indices: Accounting for background and vegetation variability, ISPRS J. Photogramm., 68, 28–39, https://doi.org/10.1016/j.isprsjprs.2011.12.007, 2012a.
Veraverbeke, S., Verstraeten, W. W., Lhermitte, S., Van De Kerchove, R., and Goossens, R.: Assessment of post-fire changes in land surface temperature and surface albedo, and their relation with fire burn severity using multitemporal MODIS imagery, Int. J. Wildland Fire, 21, 243–256, https://doi.org/10.1071/WF10075, 2012b.
Wangler, M. J. and Minnich, R. A.: Fire and Succession in Pinyon-Juniper Woodlands of the San Bernardino Mountains, California, California Botanical Society Stable, 43, 493–514, 1996.
Welch, K. R., Safford, H. D., and Young, T. P.: Predicting conifer establishment post wildfire in mixed conifer forests of the North American Mediterranean-climate zone, Ecosphere, 7, e01609, https://doi.org/10.1002/ecs2.1609, 2016.
Westerling, A. L., Hidalgo, H. G., Cayan, D. R., and Swetnam, T. W.: Warming and earlier spring increase Western U.S. forest wildfire activity, Science, 313, 940–943, https://doi.org/10.1126/science.1128834, 2006.
Williams, A. P. and Abatzoglou, J. T.: Recent Advances and Remaining Uncertainties in Resolving Past and Future Climate Effects on Global Fire Activity, Current Climate Change Reports, 2, 1–14, https://doi.org/10.1007/s40641-016-0031-0, 2016.
Williams, A. P., Seager, R., Berkelhammer, M., Macalady, A. K., Crimmins, M. A., Swetnam, T. W., Trugman, A. T., Buenning, N., Hryniw, N., McDowell, N. G., Noone, D., Mora, C. I., and Rahn T.: Causes and implications of extreme atmospheric moisture demand during the record-breaking 2011 wildfire season in the southwestern United States, J. Appl. Meteorol. Clim., 53, 2671–2684, https://doi.org/10.1175/JAMC-D-14-0053.1, 2014.
Williams, C. A., Collatz, G. J., Masek, J., and Goward, S. N.: Carbon consequences of forest disturbance and recovery across the conterminous United States, Global Biogeochem. Cy., 26, GB1005, https://doi.org/10.1029/2010GB003947, 2012.
Williams, C. A., Vanderhoof, M. K., Khomik, M., and Ghimire, B.: Post-clearcut dynamics of carbon, water and energy exchanges in a midlatitude temperate, deciduous broadleaf forest environment, Glob. Change Biol., 20, 992–1007, https://doi.org/10.1111/gcb.12388, 2014.
Williams, C. A., Gu, H., and Jiao, T.: Climate impacts of U.S. forest loss span net warming to net cooling, Sci. Adv., 7, 1–7, https://doi.org/10.1126/sciadv.aax8859, 2021.
Williams, M., Richardson, A. D., Reichstein, M., Stoy, P. C., Peylin, P., Verbeeck, H., Carvalhais, N., Jung, M., Hollinger, D. Y., Kattge, J., Leuning, R., Luo, Y., Tomelleri, E., Trudinger, C. M., and Wang, Y.-P.: Improving land surface models with FLUXNET data, Biogeosciences, 6, 1341–1359, https://doi.org/10.5194/bg-6-1341-2009, 2009.
Wittenberg, L., Malkinson, D., Beeri, O., Halutzy, A., and Tesler, N.: Spatial and temporal patterns of vegetation recovery following sequences of forest fires in a Mediterranean landscape, Mt. Carmel Israel, Catena 71, 76–83, https://doi.org/10.1016/j.catena.2006.10.007, 2007.
Yang, J., Pan, S., Dangal, S., Zhang, B., Wang, S., and Tian, H.: Continental-scale quantification of post-fire vegetation greenness recovery in temperate and boreal North America, Remote Sens, Environ., 199, 277–290, https://doi.org/10.1016/j.rse.2017.07.022, 2017.
Zhao, F. R., Meng, R., Huang, C., Zhao, M., Zhao, F. A., Gong, P., Yu, L., and Zhu, Z.: Long-term post-disturbance forest recovery in the Greater Yellowstone ecosystem analyzed using Landsat time series stack, Remote Sens., 8, 1–22, 2016.