the Creative Commons Attribution 4.0 License.
the Creative Commons Attribution 4.0 License.
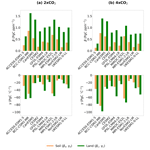
Soil carbon-concentration and carbon-climate feedbacks in CMIP6 Earth system models
Pierre Friedlingstein
Sarah E. Chadburn
Eleanor J. Burke
Peter M. Cox
Achieving climate targets requires mitigation against climate change but also understanding of the response of land and ocean carbon systems. In this context, global soil carbon stocks and their response to environmental changes are key. This paper quantifies the global soil carbon feedbacks due to changes in atmospheric CO2, and the associated climate changes, for Earth system models (ESMs) in CMIP6. A standard approach is used to calculate carbon cycle feedbacks, defined here as soil carbon-concentration (βs) and carbon-climate (γs) feedback parameters, which are also broken down into processes which drive soil carbon change. The sensitivity to CO2 is shown to dominate soil carbon changes at least up to a doubling of atmospheric CO2. However, the sensitivity of soil carbon to climate change is found to become an increasingly important source of uncertainty under higher atmospheric CO2 concentrations.
Global soil carbon stocks contain at least twice as much carbon than is stored in the world's vegetation, making soils the largest active store of carbon on the land surface of Earth (Canadell et al., 2021). In the absence of human disturbance and land-use change (Jones et al., 2018), future changes in soil carbon depend on the sensitivity to increases in atmospheric CO2 concentrations and the sensitivity to the associated impacts, such as increases in atmospheric temperatures and changes in precipitation patterns (Varney et al., 2023; Todd-Brown et al., 2014). The quantification of such carbon cycle feedbacks is required to determine the overall response of the climate system to given anthropogenic CO2 emissions and to help achieve Paris Agreement targets (Friedlingstein et al., 2022; Gregory et al., 2009).
Previous studies have defined land carbon cycle feedbacks within Earth system models (ESMs) from both CMIP6 and CMIP5 ensembles (Arora et al., 2020, 2013). In general, the overall response of carbon stores is separated into those due to changes in atmospheric CO2 (ΔCO2) and those due to changes in global temperature (ΔT), with the latter assumed to represent the overall impacts of climate change on large spatial scales. These components of land carbon cycle feedbacks are called carbon-concentration feedbacks (βL) and carbon-climate feedbacks (γL), respectively (Friedlingstein et al., 2003, 2006). An advantage of using this formulation is that it allows for the quantification of the feedbacks for a given atmospheric CO2 concentration, which can then be used as a simplified measure to compare amongst ESMs despite the increasing model complexities (Arora et al., 2020, 2013; Gregory et al., 2009). For example, it provides a consistent metric to measure land carbon feedbacks despite the differing climate sensitivities amongst ESMs (Boer and Arora, 2013).
In this study, soil-carbon-driven feedbacks in ESMs are quantified using this βγ formulation (Friedlingstein et al., 2006). Additionally, the βγ formulation is combined with the Varney et al. (2023) framework, which breaks down future changes in soil carbon (ΔCs) into individual processes which drive this response. This paper makes use of the latest generation of the Coupled Model Intercomparison Project (CMIP6) used within the Intergovernmental Panel on Climate Change 6th Assessment Report (IPCC AR6; IPCC, 2021; Eyring et al., 2016). To do this, soil carbon-concentration and carbon-climate feedback parameters are presented for CMIP6 ESMs, named βs and γs, respectively, together with components which make up βs and γs due to associated processes. The aim of this paper is to (1) quantify the sensitivity of soil carbon to increased atmospheric CO2 concentrations and associated climate impacts by calculating βs and γs for CMIP6 ESMs, (2) investigate the linearity of future soil carbon change at higher levels of atmospheric CO2 increase, and (3) identify the fraction of the land carbon response to climate change that is due to global soils.
2.1 C4MIP simulations
The Coupled Climate-Carbon Cycle Model Intercomparison Project (C4MIP) was set up to provide a common framework to allow for comparison and consistent evaluation of carbon cycle feedbacks within ESMs (Friedlingstein et al., 2006) and has been used across CMIP generations (Arora et al., 2013, 2020). This framework includes a set of idealised experiments to simplify and quantify the impact of increasing atmospheric CO2 on the climate system. In these experiments, additional effects such as land-use change, aerosols and non-CO2 greenhouse gases are not included, and nitrogen deposition is fixed at pre-industrial values (Jones et al., 2016).
The control simulation is known as the 1 % CO2 run (CMIP simulation 1pctCO2), where a consistent 1 % increase in atmospheric CO2 per year is prescribed (referred to in this study as the full 1 % CO2 simulation), starting from pre-industrial concentrations and running for 150 years. Additional experiments were designed to enable the CO2 and climate effects to be isolated, and these are known as biogeochemically coupled (referred to here as the “BGC” simulation) and radiatively coupled (referred to here as the “RAD” simulation) runs. In the BGC runs (CMIP6 simulation 1pctCO2-bgc and CMIP5 simulation esmFixClim1), the 1 % CO2 increase per year only affects the carbon cycle component of the ESM, while the radiation code continues to see pre-industrial CO2 values. Conversely, in the RAD runs (CMIP6 simulation 1pctCO2-rad and CMIP5 simulation esmFdbk1), the 1 % CO2 increase per year affects only the radiation code, and the carbon cycle component of the ESM continues to see just the pre-industrial CO2 value (285 ppm).
This study uses the full 1 % CO2, BGC and RAD C4MIP experiments with 10 CMIP6 ESMs (Eyring et al., 2016): ACCESS-ESM1-5, BCC-CSM2-MR, CanESM5, CESM2, GFDL-ESM4, IPSL-CM6A-LR, MIROC-ES2L, MPI-ESM1-2-LR, NorESM2-LM and UKESM1-0-LL (see Table 1). For comparison, the soil carbon feedback parameters were calculated using six CMIP5 ESMs (Taylor et al., 2012): CanESM2, GFDL-ESM2M, IPSL-CM5A-LR, MPI-ESM-LR, NorESM1-ME and HadGEM2-ES (see Table A2). The ESMs included were chosen due to the availability of the data required at the time of analysis (CMIP6: https://esgf-node.llnl.gov/search/cmip6/, last access: 4 February 2024, CMIP5: https://esgf-node.llnl.gov/search/cmip5/, last access: 6 February 2024).
Table 1The CMIP6 Earth system models included in this study and the relevant features of the associated land carbon cycle components: simulation of interactive nitrogen, the inclusion of dynamic vegetation, representation of fire and the soil decomposition functions used (Varney et al., 2022; Arora et al., 2020). Explanations of the temperature and moisture functions used within ESMs are given in Varney et al. (2022) and Todd-Brown et al. (2013).
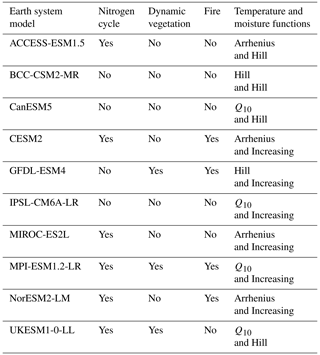
2.2 Defining soil carbon feedbacks
2.2.1 Friedlingstein et al. (2006) βγ formulation
The standard formulation uses a linear approximation to estimate carbon cycle feedbacks in a changing climate (Friedlingstein et al., 2003, 2006). The change in land carbon storage (ΔCL, PgC) is approximated linearly using feedback parameters which define separate sensitivities to changes in atmospheric CO2 (ΔCO2, ppm) and changes in global temperatures (ΔT, °C), defined as the land carbon-concentration (βL, PgC ppm−1) and carbon-climate (γL, PgC °C−1) (Eq. 1).
The Friedlingstein et al. (2006) methodology uses time-integrated fluxes, which represent the total change in the size of the land carbon pool (ΔCL). This is presented for the full 1 % CO2 simulation (Eq. 2), BGC simulation (Eq. 3) and RAD simulation (Eq. 4) below, where ΔCL, and are the changes in global land carbon pools (PgC) and FL, and are the net carbon fluxes to the land (PgC yr−1) for each simulation.
In these equations, ΔCO2(t) (ppm) is consistent between all the scenarios. Within the RAD simulation, however (Eq. 4), the carbon cycle does not see an increased CO2, so the ΔCO2 is neglected and only found in the full 1 % CO2 and BGC simulations (Eqs. 2 and 3, respectively). ΔT, ΔTBGC and ΔTRAD (°C) are the changes in global temperatures in the full 1 % CO2, BGC and RAD simulations, respectively. In Eq. (3), ΔTBGC is assumed to be negligible, following Friedlingstein et al. (2006). As the increased CO2 within the BGC simulation does not affect the radiation code, there is no direct increase in atmospheric temperatures within the model. Arora et al. (2020) explain however that local changes in the carbon cycle arising from increases in CO2 affect latent and sensible heat fluxes at the land surface, including changes to evaporative fluxes from stomatal closure over land and changes in vegetation structure and coverage if dynamic vegetation is included within the ESM (see Table 1). This study assumes that the global temperature changes in the BGC simulation are negligible in the context of the βγ formulation (Fig. A1).
2.2.2 Soil carbon-concentration and carbon-climate feedbacks
Global ΔCL can be written as the sum of the changes in vegetation carbon (ΔCv) and changes in soil carbon (ΔCs). Following the βγ formulation, a similar breakdown of the land carbon-concentration and carbon-climate feedback parameters can be derived, where and (Eq. 5).
Therefore, an equation for ΔCs can be obtained, with soil-specific carbon-concentration (βs) and carbon-climate (γs) feedback parameters, which represent the sensitivity of ΔCs to CO2 and T, respectively (Eq. 7).
2.3 Processes driving soil carbon change and the relation to the βγ formulation
To isolate the processes which make up each soil carbon feedback, we follow the framework presented in Varney et al. (2023). An equation for soil carbon (Eq. 8) is derived using the definition of soil carbon turnover time (), which is defined as the ratio of soil carbon storage (Cs) to the carbon output flux from the soil (heterotrophic respiration, Rh; Varney et al., 2020). Future soil carbon can then be defined as initial soil carbon (Cs,0) plus a change in soil carbon (ΔCs), as shown by Eq. 9, where the subscript 0 denotes the initial state (decadal time average at the start of C4MIP simulation). Equation (9) can be expanded to give Eq. (10), which can be simplified to give Eq. (11), as shown below.
To consider the above- and below-ground effects on soil carbon separately, the effects due to changes in vegetation productivity, represented by net primary productivity (NPP), and effects due to changes in soil carbon turnover time due to increased heterotrophic respiration (τs) are considered (Todd-Brown et al., 2014). However, due to the difference between the global fluxes NPP and Rh in a transient climate, an additional term is included which is defined as net ecosystem productivity (). Using the definition of NEP, this can be substituted into Eq. (11) to give Eq. (12) and expanded to give an equation for ΔCs in terms of NPP, NEP and τs (Eq. 13, where the subscript 0 denotes the initial state).
The individual terms in Eq. (13) are the change in soil carbon due to NPP changes (), the change in soil carbon due to the NEP transient term (), the change in soil carbon due to τs changes () and the transient effect on τs (). The two additional terms are the non-linear term between NPP and τs (ΔNPPΔτs) and the non-linear term between NEP and τs (ΔNEPΔτs).
The NEP term is used to represent the transient state of the system where NPP ≠ Rh. However, it is noted that, if the initial state is in equilibrium, then the initial NEP (NEP0) will be approximately equal to zero. This would mean the term (representing the difference in τs from using NPP or Rh in the definition) will be negligible. Despite initialising at the start of the C4MIP simulations (decadal time average at the start of C4MIP simulation), this term is included within the analysis for completeness to ensure exact values of ΔCs.
Following on from this Varney et al. (2023) framework, the equation for ΔCs (Eq. 13) can also be defined for the change in soil carbon in both the BGC simulations (, Eq. 14) and RAD simulations (, Eq. 15), where the superscripts denote the BGC and RAD simulations, respectively.
These equations can be used to investigate the sensitivity of these isolated processes to changes in atmospheric CO2 and global temperature (T), as shown by Eqs. (16) and (17). This is done by the explicit differentiation of Eqs. (14) and (15) with respect to CO2 and T, respectively.
Equations (16) and (17) can be used to relate these CO2 and T sensitivities to the βγ formulation, where β is used to represent the sensitivity to CO2 and γ is used to represent the sensitivity to T. Equation (7), which defines ΔCs in terms of the soil carbon-concentration (βs) and carbon-climate (γs) feedback parameters, can be rewritten in terms of partial derivatives, as shown by Eq. (18).
Then, Eqs. (16) and (17) can be used together with Eq. (18) to combine the βγ formulation with the Varney et al. (2023) framework. In this case, therefore, βs and γs can be defined as the contributions to ΔCs based on the individual sensitivities of the soil carbon controls to CO2 and T (by substituting Eqs. (14) and (15) into Eqs. (16) and (17), respectively), as shown by Eqs. (20) and (21).
where
Equations (20) and (21) can be rewritten by defining the βs and γs contribution terms, where each component of the equations makes up the total βs and γs sensitivities, as shown below for βs (Eq. 22) and γs (Eq. 23):
where βNPP and γNPP are the βγ contributions due to ΔNPP, βτ and γτ are the βγ contributions due to Δτs, βNEP and γNEP are the βγ contributions due to the transient NEP term, including and representing the βγ contributions due to the transient NEP term on Δτs, and then βΔNPPΔτ, βΔNEPΔτ, γΔNPPΔτ and γΔNEPΔτ are the non-linear effects on βγ.
2.4 Calculation of feedback parameters
2.4.1 Defining climate variables
For each of the CMIP6 ESMs, the CMIP output variables cSoil, cLitter and cVeg are considered in the land carbon storage analysis. Soil carbon (Cs) is defined as the sum of carbon stored in soils and the carbon stored in the litter (CMIP variable cSoil + CMIP variable cLitter), allowing for a more consistent comparison between the models despite differences in how soil carbon and litter carbon are simulated (Varney et al., 2022; Todd-Brown et al., 2013). For models that do not report a separate litter carbon pool (CMIP variable cLitter), soil carbon is taken to be simply the CMIP variable cSoil (UKESM1-0-LL). Land carbon (CL) is defined as the sum of carbon stored in soil + litter (Cs) plus the carbon stored in vegetation (Cv, CMIP variable cVeg). Global total values for Cs and CL (PgC) are calculated using an area-weighted sum (using the model land surface fraction, CMIP variable sftlf).
In the breakdown analysis of the βγ feedbacks, NPP (CMIP variable npp) is defined as the net carbon assimilated by plants via photosynthesis minus loss due to plant respiration and is used to represent the net carbon input flux to the system. Heterotrophic respiration (Rh, CMIP variable rh) is defined as the microbial respiration within global soils and is used to define an effective global soil carbon turnover time (τs). τs (years) is defined as the ratio of mean soil carbon to annual mean heterotrophic respiration, given as (where the mean represents an area-weighted global average). Carbon fluxes (NPP and Rh) in the calculation of feedback contributions are considered area-weighted global totals (PgC yr−1, using the model land surface fraction, CMIP variable sftlf).
Increases in global temperatures (ΔT) are considered using CMIP variable tas, which is defined as the change in near-surface air temperature (°C). To calculate changes in atmospheric CO2 (ΔCO2) in the C4MIP 1 % CO2 simulations, initial pre-industrial CO2 concentrations are assumed to be 285 ppm and then cumulatively increased by 1 % each year for 70 years (approximately 2 × CO2) or 140 years (approximately 4 × CO2).
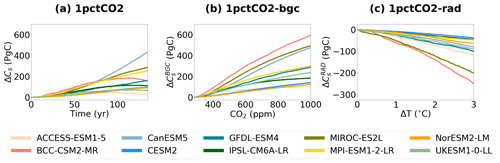
Figure 1Time series of projected changes in soil carbon (ΔCs) in CMIP6 ESMs for the (a) idealised 1 % CO2, (b) biogeochemically coupled 1 % CO2 (BGC) and (c) radiatively coupled 1 % CO2 (RAD) simulations. This figure has been adapted from Fig. A2 in Varney et al. (2023).
2.4.2 Carbon-concentration feedback parameter (β)
To calculate the soil carbon-concentration feedback parameter (βs), the BGC run was used. For each ESM, the change in soil carbon in the BGC run (, PgC) was divided by the change in CO2 concentration (ppm) up to that point in time (expressed in units of carbon uptake or release per unit change in CO2, PgC ppm−1). For this study, βs was calculated at the time of 2 × CO2 and 4 × CO2. To calculate the land carbon-concentration feedback parameter (βL), the same method was used but replacing with .
2.4.3 Carbon-climate feedback parameter (γ)
To calculate the soil carbon-climate feedback parameter (γs), the RAD run was used. For each ESM, the change in soil carbon in the RAD run (, PgC) was divided by the change in temperature T (°C) up to that point in time (expressed in units of carbon uptake or release per unit change in temperature, PgC °C−1). For this study, γs was calculated at 2 × CO2 and 4 × CO2. To calculate the land carbon-climate feedback parameter (γL), the same method was used but replacing with .
2.4.4 Feedback parameter contributions
To calculate the isolated contributions which make up β and γ, as shown in Eqs. (22) and (23), the BGC and RAD simulations are again used for each CMIP6 ESM. To calculate gradients with respect to CO2 and T, the methodology presented above is used but with the relevant component against CO2 or T, such as NPP or τs. The βs contributions are expressed in units of carbon uptake or release per unit change in CO2 (PgC ppm−1) and the γs contributions are expressed in units of carbon uptake or release per unit change in temperature (PgC °C−1), using the definitions presented in Eqs. (22) and (23).
3.1 Projections of soil carbon change
Projections of soil carbon change in CMIP6 ESMs for the full 1 % CO2 (ΔCs), BGC () and RAD () simulations are presented in Fig. 1. Soil carbon is projected to increase in the full 1 % CO2 simulation amongst CMIP6 ESMs (ensemble mean 88.2 ± 40.4 PgC at 2 × CO2 and 177 ± 141 PgC at 4 × CO2). However, the magnitude of the increase varies amongst the ESMs, with a range of 38 PgC (NorESM2-LM) to 145 PgC (BCC-CSM2-MR) at 2 × CO2 and a range of 15 PgC (ACCESS-ESM1-5) to 502 PgC (CanESM5) at 4 × CO2. Six of the ESMs (CanESM5, CESM2, GFDL-ESM4, MIROC-ES2L, MPI-ESM1-2-LR, NorESM2-LM) see an increased ΔCs value with increasing climate forcing. However, the remaining four ESMs (ACCESS-ESM1-5, BCC-CSM2-MR, IPSL-CM6A-LR, UKESM1-0-LL) see a saturation in the rate of increase or even a turning point where carbon starts to decrease again, from 70 years (≈ 2 × CO2) in the simulation (Fig. 1a).
The projected increase in soil carbon can be approximated by the increases projected in the BGC run (; ensemble mean 132 ± 66.5 PgC at 2 × CO2 and 348 ± 203 PgC at 4 × CO2, Fig. 1b) and the decreases projected in the RAD run (; ensemble mean −45.5 ± 22.9 PgC at 2 × CO2 and −170 ± 94.7 PgC at 4 × CO2, Fig. 1c). The responses due to increases in atmospheric CO2 (BGC simulation) are found to dominate the overall response (full 1 % CO2 simulation) in the majority of the models, where greater magnitudes of change are seen compared with the RAD simulation (exception ACCESS-ESM1-5). The BGC simulation also sees a greater spread in projected ΔCs, with ranges of 218 PgC at 2 × CO2 and 603 PgC at 4 × CO2 () compared with ranges of 68 PgC at 2 × CO2 and 312 PgC at 4 × CO2 in the RAD simulation ().
Figure 2 shows patterns of soil carbon changes at 4 × CO2 for the full 1 % CO2 (ΔCs), BGC () and RAD (). In the BGC simulation, increases in are seen across the majority of regions within CMIP6 ESMs, though exceptions are found at the northern latitudes for two ESMs (CanESM5 and NorESM2-LM). Across the ensemble, the projected increases in have spatially varying magnitudes, where generally the greatest increases are seen in the tropical regions. Conversely, the RAD simulation generally sees reductions in globally, with the greatest reductions seen in the tropical regions. However, disagreement is seen at the northern latitudes, where four models (ACCESS-ESM1-5, CanESM5, MIROC-ES2L, UKESM1-0-LL) see an increased and three models (BCC-CSM2-MR, CESM2, NorESM2-LM) see a decreased . The overall ΔCs values seen in the full 1 % CO2 simulation are again found to be mostly dominated by the BGC simulation (Fig. 2), though exceptions are seen where the RAD simulation is shown to dominate the response for certain regions. Specifically, the reduced ΔCs within the RAD simulation dominates the net response at the northern latitudes of three ESMs (BCC-CSM2-MR, CESM2 and NorESM2-LM, the only models where decreases were seen) as well as in the tropical regions of three different ESMs (ACCESS-ESM1-5, GFDL-ESM4 and UKESM1-0-LL).
3.2 Soil carbon-concentration and carbon-climate feedback parameters
The calculated βs and γs values for CMIP6 ESMs are presented in Table 2. Values for βs are found to be positive amongst the CMIP6 ESMs, which is consistent with increased Cs with increasing CO2, and values for γs are found to be negative, which is consistent with decreased Cs with increasing temperature (Fig. 3). The magnitudes of the feedback parameters (βs and γs) are found to vary amongst the CMIP6 ensemble, suggesting uncertainty in the magnitude of the soil carbon response to climate change. Generally, models with higher sensitivities to CO2 (βs) also have higher sensitivities to temperature (γs), where r2 values of 0.64 (2 × CO2) and 0.60 (4 × CO2) are found between the βs and γs values (Table 2). The ranges in projected βs parameters are found to be relatively consistent between 2 × CO2 and 4 × CO2 (where a small decrease is seen), with a range of 0.704 PgC ppm−1 and a range of 0.636 PgC ppm−1, respectively. Conversely, the ranges of calculated γs parameters are found to be less consistent between 2 × CO2 and 4 × CO2 (increasing range with increased global warming), with ranges of 42.7 and 68.0 PgC °C−1, respectively (Table 2).
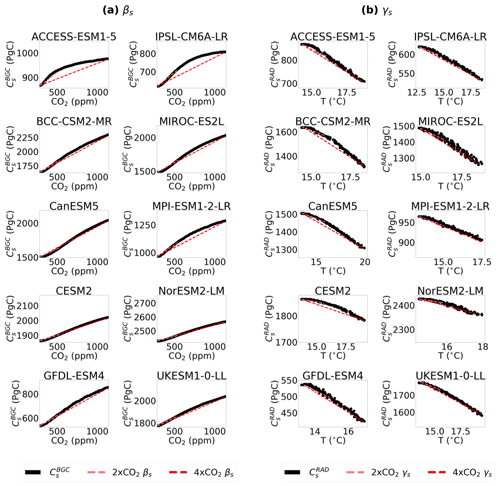
Figure 3Time series plots used to calculate the soil feedback parameters. (a) Soil carbon in the BGC simulation (, PgC) vs. CO2 (ppm) for the carbon-concentration feedback parameters (βs, PgC ppm−1) and (b) soil carbon in the RAD simulation (, PgC) vs. temperature (T, °C) for the soil carbon-climate feedback parameters (γs, PgC °C−1), for each CMIP6 ESM. The lines show the gradients at 2 × CO2 (lighter line) and 4 × CO2 (darker line), respectively.
The linearity of future soil carbon changes can be investigated by comparing the 2 × CO2 and 4 × CO2 lines for βs and γs in Fig. 3. A future linear response is shown to be a good approximation. However, the figure suggests a slight non-linearity in the soil carbon response to both CO2 () and temperature () in the majority of ESMs. The BGC simulation generally sees greater consistency between 2 × CO2 and 4 × CO2 βs values, for example in the CESM2 and NorESM2-LM models. However, the majority of ESMs (ACCESS-ESM1-5, BCC-CSM2-MR, GFDL-ESM4, IPSL-CM6A-LR, MIROC-ES2L, MPI-ESM1-2-LR and UKESM1-0-LL) see a reduction in βs and a saturation in the sensitivity with greater CO2 levels (Fig. 3a). In the RAD simulation, generally inconsistencies are seen between 2 × CO2 and 4 × CO2 (exception MPI-ESM1-2-LR), and an increased sensitivity of to temperature (T) with increased climate forcing is suggested by the majority of CMIP6 ESMs (Fig. 3b). As an example, in CESM2, where one of the lowest sensitivities to T at 2 × CO2 is seen, the ESM sees an approximate 50 % increase in γs by 4 × CO2 (Table 2).
The βs and γs values were also calculated for CMIP5 ESMs (Table A3), which can be compared with a subset of generationally related CMIP6 ESMs considered in this study (Fig. A3). The CMIP6 ensemble means for both βs and γs parameters are found to be lower compared with the CMIP5 ensemble means (Tables 2 and A3). The relationship of βs and γs values between CMIP5 and CMIP6, however, is not found to be consistent amongst the ensembles. For βs, reductions are seen in four ESMs (GFDL-ESM2M vs. GFDL-ESM4, IPSL-CM5A-LR vs. IPSL-CM6A-LR, MPI-ESM-LR vs. MPI-ESM1-2-LR and HadGEM2-ES vs. UKESM1-0-LL) compared with increases in the remaining two (CanESM2 vs. CanESM5 and NorESM1-ME vs. NorESM2-LM). For γs, a greater value (closer to 0) is seen in four ESMs (CanESM2 vs. CanESM5, GFDL-ESM2M vs. GFDL-ESM4, IPSL-CM5A-LR vs. IPSL-CM6A-LR and MPI-ESM-LR vs. MPI-ESM1-2-LR) compared with a lower value (greater negative) seen in the remaining two ESMs (NorESM1-ME vs. NorESM2-LM and HadGEM2-ES vs. UKESM1-0-LL).
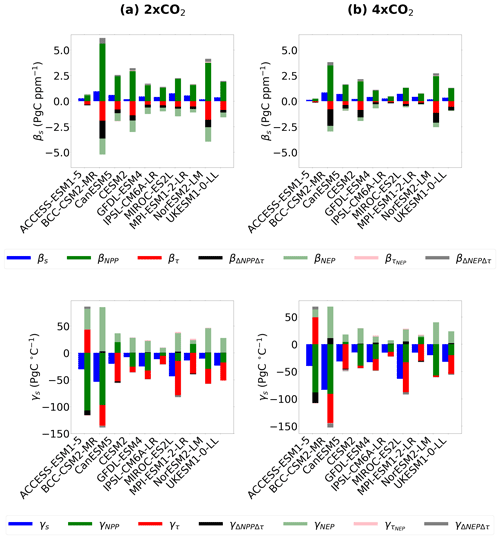
Figure 4Investigating the contribution of individual soil carbon drivers to the soil carbon-concentration (βs, top row) and carbon-climate (γs, bottom row) feedback parameters, for each CMIP6 ESM, for (a) 2 × CO2 and (b) 4 × CO2. The figure shows soil carbon feedback parameter contributions from NPP (βNPP and γNPP), τs (βτ and γτ), the non-linearity in NPP and τs (βΔNPPΔτ and γΔNPPΔτ) and the effect from the non-equilibrium term NEP (βNEP, , βΔNEPΔτ and γNEP, , γΔNEPΔτ).
3.3 Breakdown of the feedback parameters into soil carbon drivers
In this section, βs and γs are broken down into the individual sensitivities of drivers of soil carbon change which make up the net response. As shown in Fig. 4, the total soil carbon sensitivities (βs and γs, blue bars) can be considered as the sum of the sensitivity due to ΔNPP (βNPP and γNPP, green bars), the sensitivity due to Δτs (βτ and γτ, red bars) and additional terms due to the transient land carbon sink, such as NEP (βNEP and γNEP, light green bars) and the NEP effect on τs ( and , pink bars). Additionally, there are non-negligible contributions due to non-linear sensitivities between NPP and τs (βΔNPPΔτ and γΔNPPΔτ, black bars) and a small contribution from non-linear sensitivities between NEP and τs (βΔNEPΔτ and γΔNEPΔτ, grey bars).
Table 2The soil carbon-concentration (βs, PgC ppm−1) and carbon-climate (γs, PgC °C−1) feedback parameters for 2 × CO2 and 4 × CO2 for the CMIP6 ESMs.
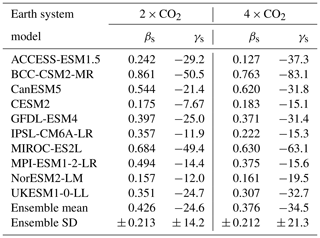
Investigating the sensitivity of soil carbon to ΔNPP, βNPP is found to be positive amongst CMIP6 ESMs (Fig. 4). At 2 × CO2, βNPP ranges from 0.567 PgC ppm−1 (ACCESS-ESM1-5) to 5.62 PgC ppm−1 (BCC-CSM2-MR), with an ensemble mean of 2.37 ± 1.37 PgC ppm−1. There is some evidence of a saturation of global NPP at higher CO2, with the sensitivity of NPP to CO2 (βNPP) decreasing at 4 × CO2 to an ensemble mean of 1.44 ± 0.933 PgC ppm−1. The sensitivity of NPP to global temperature changes (γNPP) is found to be more variable in the ensemble. The majority of models find γNPP to be negative. However, it is found to be positive in two ESMs (CanESM5 and MPI-ESM1-2-LR). The sensitivity of NPP to temperature (γNPP) is found to be more consistent with climate change than the sensitivity to CO2 (βNPP), where the γNPP ensemble mean changes from −29.4 ± 40.1 PgC °C−1 at 2 × CO2 to −35.3 ± 33.1 PgC °C−1 at 4 × CO2 (Fig. 4). At 4 × CO2, the lowest sensitivity of NPP to temperature is seen in CanESM5 (3.95 PgC °C−1) and the highest sensitivity in BCC-CSM2-MR (−90.8 PgC °C−1).
Investigating the sensitivity of soil carbon to Δτs, negative βτ and γτ values are mostly found amongst the CMIP6 models (Fig. 4). An anomaly is found where τs is found to increase with temperature in the ACCESS-ESM1-5 model, where the reason for this is unclear (Fig. A2). The sensitivity of τs to T (γτ) is also found to be more consistent with increasing climate change than the sensitivity to CO2, where an ensemble mean of −25.2 ± 27.9 PgC °C−1 at 2 × CO2 and −20.5 ± 29.5 PgC °C−1 at 4 × CO2 is seen. At 4 × CO2, the greatest sensitivity of τs to temperature is seen in the MIROC-ES2L model (−54.6 PgC °C−1) and the lowest sensitivity is seen in the NorESM2-LM model (−2.80 PgC °C−1). τs is found to decrease non-linearly with increasing CO2 (βτ). At 2 × CO2, βτ ranges from −0.329 PgC ppm−1 (ACCESS-ESM1-5) to −1.90 PgC ppm−1 (BCC-CSM2-MR), with an ensemble mean of −0.900 ± 0.574 PgC ppm−1. Due to the non-linearity, a reduced ensemble mean of −0.450 ± 0.359 PgC ppm−1 is found at 4 × CO2 compared with 2 × CO2 (Fig. 4).
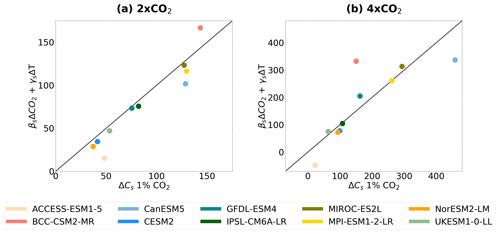
Figure 5Comparison of ΔCs (PgC) in the full 1 % CO2 simulation (x axis) against the estimated ΔCs using the calculated βs and γs feedback parameters (y axis), where estimated for each CMIP6 ESM at (a) 2 × CO2 and (b) 4 × CO2.
It is apparent from Fig. 4 that the sensitivities of NPP and τs to both CO2 and T must be accounted for to understand and quantify the sensitivities of soil carbon. The magnitude of βτ is found to be approximately one-third of the magnitude of βNPP at both 2 × CO2 and 4 × CO2 but with counteracting signs of change. Models with the lowest βNPP sensitivities also see the lowest βτ sensitivities (e.g. ACCESS-ESM1-5) and vice versa. The magnitude of γNPP is generally found to be greater across the ensemble compared with γτ, with however a greater range of sensitivities. Additionally, the apparent sensitivity of soil carbon to CO2 is less than the individual sensitivities of NPP and τs, due to a cancellation effect from opposing signs, leading to a lower apparent βs. The magnitudes of βNPP and βτ are lower at 4 × CO2 than 2 × CO2, which means a reduced sensitivity of NPP and τs to CO2 at greater levels of climate change. However, due to this cancellation effect, the same reduced sensitivity is not seen in βs. Conversely, a reduced sensitivity of NPP and τs to temperature is not suggested under increasing climate forcing. No clear relationship between γNPP and γτ is seen amongst the CMIP6 ESMs (Fig. 4).
The contribution of the non-linearity between NPP and τs to the net soil carbon sensitivity is also investigated (βΔNPPΔτ and γΔNPPΔτ). Figure 4 suggests that the non-linearity between NPP and τs is more robustly projected to result from increasing CO2 (βs). However, non-linearities in γs are also seen in the models with the greatest temperature sensitivities. The ensemble mean predicted βΔNPPΔτ is found to be −0.462 ± 0.462 at 2 × CO2 and −0.463 ± 0.468 PgC ppm−1 at 4 × CO2. As expected from Fig. 4, predicted γΔNPPΔτ is found to have a low sensitivity, where the ensemble means of −0.374 ± 3.12 at 2 × CO2 and −0.0478 ± 7.42 PgC °C−1 at 4 × CO2 are found. Additionally, the NEP terms (βNEP and γNEP) are shown to contribute to both CO2 and T sensitivities (Fig. 4), due to the disequilibrium of land carbon changes under 1 % increasing CO2.
3.4 Investigating the robustness of the ΔCs approximation
Projections of ΔCs in ESMs in the full 1 % CO2 simulation were compared with the estimated ΔCs derived using Eq. (7), which uses the derived βs and γs feedback parameters together with model-specific ΔT and estimates for ΔCO2 (Fig. 5). This investigates the approximation that changes in the full 1 % CO2 simulation are equal to the sum of changes in the BGC and RAD simulations. At 2 × CO2, the approximation is found to predict ΔCs within 20 % of the actual projected values in the 1 % CO2 simulation for 7 out of the 10 CMIP6 ESMs (BCC-CSM2-MR, CESM2, GFDL-ESM4, IPSL-CM6A-LR, MIROC-ES2L, MPI-ESM1-2-LR and UKESM1-0-LL). At 4 × CO2, the robustness of the assumption between the BGC and RAD simulations decreases for future changes in soil carbon. However, βsΔCO2+γsΔT is within 20 % of the projected ΔCs for 5 out of the 10 ESMs (GFDL-ESM4, IPSL-CM6A-LR, MIROC-ES2L, MPI-ESM1-2-LR and UKESM1-0-LL). The models where the approximation is the least consistent with projected ΔCs are ACCESS-ESM1-5 and BCC-CSM2-MR, where at 4 × CO2 the greatest non-linearities are present between BGC and RAD simulations (Fig. 5).
3.5 Comparisons between soil and land feedback parameters
The contribution of the sensitivity of soil carbon stocks (Cs) to the total sensitivity of land carbon stocks (CL) was investigated by comparing the β and γ feedback parameters for land (Table A1) and soil (Table 2) for both 2 × CO2 and 4 × CO2 in CMIP6 ESMs (Fig. 6). Here, the assumption from Eq. (5) is followed that the land sensitivity is made up of the sum of the soil and vegetation responses. For the carbon-concentration feedback (β), the portion of the land sensitivity to CO2 (βL) that is due to global soils (βs) ranges from 19 % (NorESM2-LM) to 53 % (BCC-CSM2-MR), with a mean of 38 ± 11 % seen across the CMIP6 ESMs at 2 × CO2 (Fig. 6a). Similar proportions are found at 4 × CO2, ranging from 22 % (NorESM2-LM) to 58 % (MIROC-ES2-L), with a mean of 42 ± 12 % seen across the CMIP6 ESMs (Fig. 6b). The portion of βL due to βs is estimated to be close to half of the total land response. For the carbon-climate feedback (γ), the portion of the land sensitivity to climate (γL) that is due to global soils (γs) ranges from approximately 42 % (CESM2) to 147 % (MPI-ESM1-2-LM), with a mean of 75 ± 30 % seen across the CMIP6 ESMs at 2 × CO2 (Fig. 6a), and at 4 × CO2 the range is from 48 % (ACCESS-ESM1-5) to 157 % (MPI-ESM1-2-LM), with a mean of 75 ± 31 % seen across the CMIP6 ESMs (Fig. 6b). Therefore, the portion of γL due to γs is estimated to be the majority of the sensitivity, suggesting that soil dominates the response of land carbon to climate. Note that the MPI-ESM1-2-LR model sees a greater γs value compared with γL, resulting in the percentage of the land response attributed to soil being greater than 100 %. This suggests a positive γv response in this model, meaning a predicted increased vegetation carbon globally with global warming.
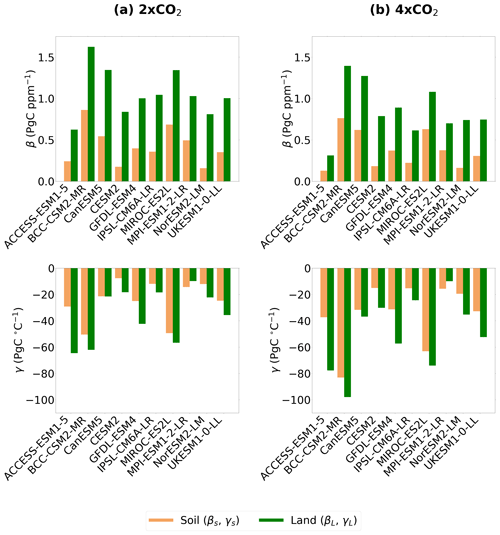
Figure 6Comparisons of the land carbon-concentration (βL) feedback parameters with the soil carbon-concentration (βs) feedback parameters (top row) and the land carbon-climate (γL) feedback parameters with the soil carbon-climate (γs) feedback parameters (bottom row), for (a) 2 × CO2 and (b) 4 × CO2.
Quantifying the future sensitivity of global soil carbon stocks to anthropogenic CO2 emissions and their role in future land carbon storage is vital in order to understand future changes in the Earth's climate system (Canadell et al., 2021). Global changes in soil carbon (ΔCs), in the absence of human disturbance and land-use change, will result from responses due to changes in atmospheric CO2 and the associated changes in global temperatures (T), which are used to represent climate effects on a global scale. By separating the sensitivities due to increasing CO2 and T, the idealised C4MIP ESM simulations allow for these effects on soil carbon to be examined individually, and the use of the βγ formulation allows these sensitivities to be quantified and compared for CMIP6 ESMs (Jones et al., 2016; Friedlingstein et al., 2006). Further, combining the βγ formulation with the Varney et al. (2023) ΔCs framework allows us to isolate the sensitivities of soil carbon processes which influence βs and γs within models.
Across CMIP6 ESMs, soil carbon is projected to increase in the BGC simulation (“CO2-only”) and decrease in the RAD simulation (“climate-only”), consistent with projections of the overall land carbon response (Arora et al., 2020). The BGC simulation has been used to quantify the sensitivity of soil carbon to ΔCO2 (βs), where positive βs values were defined according to the projected increase in soil carbon with increased atmospheric CO2 (Fig. 1b). The positive βs has been shown here to mostly be a result of a positive βNPP term (Fig. 4), which represents the increased CO2 fertilisation effect describing increased vegetation productivity under higher atmospheric CO2 concentrations, which leads to an increased input of litter carbon into soil carbon pools (Schimel et al., 2015; Koven et al., 2015). A negative contribution of βτ to βs is also shown (Fig. 4). Previously, Varney et al. (2023) presented a transient reduction in τs in CMIP6 ESMs due to an increased rate of carbon input into the soil (i.e. negative βτ due to positive βNPP), a phenomenon known as false priming (Koven et al., 2015). However, it can be seen that the magnitude of this effect is small compared with the CO2 fertilisation effect across the ESMs (βτ vs. βNPP, Fig. 4). Despite agreement on a net increase in soil carbon stocks globally (positive βs), this study highlights uncertainty in the projected magnitude of this sensitivity amongst the CMIP6 models, which is seen to be driven by uncertainties in βNPP (Fig. 4).
The RAD simulation has been used to quantify the sensitivity of soil carbon to changes in climate (ΔT; γs), where negative γs values were defined due to the projected decrease in soil carbon with global warming (Fig. 1c). The negative γs term has been shown here to be a result of negative γτ and, in many cases, negative γNPP (Fig. 4). The negative sensitivity of τs to global warming (negative γτ) is known to be due to an increased rate of heterotrophic respiration (Rh) at warmer temperatures as a result of increased microbial activity (Varney et al., 2020; Crowther et al., 2016). The global sensitivity of NPP to climate changes (γNPP) is less certain where both negative and positive values are seen across the CMIP6 ESMs (Fig. 4). This is likely due to more spatially varying responses, where the resultant ΔCs can be seen in Fig. 2. For example, increased temperatures at northern latitudes could result in the northward expansion of boreal forests (Pugh et al., 2018), which would increase forest productivity and subsequently carbon storage in these regions. However, future changes in precipitation patterns could lead to regions with reduced soil moisture, which would conversely lead to reduced vegetation productivity and carbon uptake (Green et al., 2019). The uncertainties associated with projected spatial changes (γNPP), together with the uncertainties in the magnitude of carbon turnover times within the soil (γτ; Varney et al., 2020; Koven et al., 2017), result in uncertainties in the sensitivity of soil carbon to climate changes (γs) amongst the CMIP6 models.
This paper highlights the importance of soils within the land carbon response to global warming (Fig. 6). Despite the ΔCs sensitivity to CO2 dominating net soil carbon changes (βs), it could be argued that the significance of the ΔCs climate sensitivity (γs) will increase under more extreme levels of climate change. This is suggested by both a projected saturation of βs and an increase in γs between 2 × CO2 and 4 × CO2 shown in the CMIP6 ensemble means (Table 2). The saturation, or reduced rate of increase, in βs seen in CMIP6 is likely due to a limit of the CO2 fertilisation effect, based on the reduced βNPP values between 2 × CO2 and 4 × CO2 (Fig. 4). The rate of CO2 fertilisation in the future is expected to be limited by nutrient availability (Wieder et al., 2015), which in CMIP6 is now more explicitly represented by the inclusion of an interactive nitrogen cycle in multiple models (see Table 1). This implementation is expected to limit the increased productivity from CO2 fertilisation within ESMs (Davies-Barnard et al., 2020) and has previously been found to lower the magnitude of the land feedback parameters (Arora et al., 2020). However, it is noted that warming within the soil could accelerate nutrient mineralisation, which could result in a liberation of nitrogen due to increased microbial breakdown of plant litter, alleviating the nutrient limitation in plants (Todd-Brown et al., 2014).
Unlike the βs parameter, the sensitivity of soil carbon to climate changes (γs) has been shown to increase with global warming in CMIP6. The greater γs values at 4 × CO2 compared with 2 × CO2 found here imply an increased rate of soil carbon loss under increased amounts of global warming (Table 2). Additionally, it could be hypothesised that limitations within CMIP6 ESMs in the representation of soil carbon and related processes could lead to a potential underestimation of γs. In Fig. 2, reductions in soil carbon stocks at the high northern latitudes are only seen in three models for the full 1 % CO2 simulation (BCC-CSM2-MR, CESM2 and NorESM2-LM). Varney et al. (2022) find that these CMIP6 models represent quantities of northern-latitude carbon stocks most consistently with observational estimates, which could imply an increased likelihood of soil carbon loss from the northern latitudes based on consistency with observations. It is noted however that CESM2 and NorESM2-LM contain the same land surface model and so are expected to show similar results (Lawrence et al., 2019). Furthermore, the majority of ESMs do not include explicit representation of permafrost carbon (Burke et al., 2020). Including permafrost within ESMs would result in increased quantities of carbon within the soil known to be especially sensitive to global warming (increased γs), which currently are not included in the calculation of these feedbacks (Schuur et al., 2015).
The βγ formulation has many benefits in allowing the quantification and comparison of land and soil carbon feedbacks amongst ESMs. However, one limitation is due to ΔCs not being consistently linear with increasing CO2 and temperature (Fig. 3), so the parameter values depend on the point in time at which they are calculated (for example, 2 × CO2 or 4 × CO2). This has been shown to be due to non-linearities in the processes driving soil carbon feedbacks (Fig. 4), such as the discussed saturation of the CO2 fertilisation effect (βNPP; Wang et al., 2020) and additionally a known Q10 dependence of heterotrophic (soil) respiration on temperature (γτ; Zhou et al., 2009).
Non-linearities between CO2 and T responses are also known and have previously been shown within ESMs in the future land carbon responses (Schwinger et al., 2014; Zickfeld et al., 2011; Gregory et al., 2009). Zickfeld et al. (2011) suggest that the non-linearity in the land response is due to significantly differing vegetation responses which depend on whether or not climate effects are combined with the CO2 fertilisation effect, e.g. forest dieback (Cox et al., 2004). However, this is model-dependent, as not all models within CMIP6 simulate dynamic vegetation (Table 1). The spatial variations in the response of soil carbon to CO2 and climate that are seen in Fig. 2 could also contribute to the non-linearity. For example, a different spatial pattern of soil carbon under elevated CO2 could lead to a different overall temperature response, e.g. if more carbon is at the high latitudes where greater temperature changes are seen. Arora et al. (2020) find that climate responses in the BGC simulation account for a difference of 1 %–5 % in the calculation of the feedbacks, suggesting a small but non-negligible effect of climate in the BGC runs. This response was shown to be dependent on the representation of vegetation within the model, as with the non-linearities found in Zickfeld et al. (2011). Despite this, isolating and quantifying the key sensitivities with the βγ method provides a useful benchmark for feedbacks within CMIP.
The Friedlingstein et al. (2006) methodology adapted in this study suggests that βs and γs linearity is a valid assumption for projected soil carbon changes in ESMs up until a doubling of CO2. However, under more extreme levels of climate change, the results here suggest the need for the non-linearity in feedbacks to be further investigated. Soil carbon is found to have a greater impact on carbon-climate feedbacks than vegetation carbon responses, which means that the sensitivity of soil carbon to changes in global temperature is the dominant response of the land carbon cycle when considering climate effects. Therefore, further understanding and quantifying the sensitivity of global soils under global warming is necessary to quantify future changes in the climate system. Moreover, the sensitivity of soil carbon to temperature increases with increasing climate forcing, suggesting that soil carbon is particularly important in the long-term land carbon response under extreme levels of global warming.
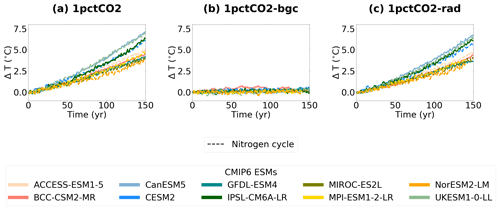
Figure A1Time series of projected global mean temperature changes (ΔT) in CMIP6 ESMs for the idealised simulations 1 % CO2 (a), biogeochemically coupled 1 % CO2 (BGC, b) and radiatively coupled 1 % CO2 (RAD, c).
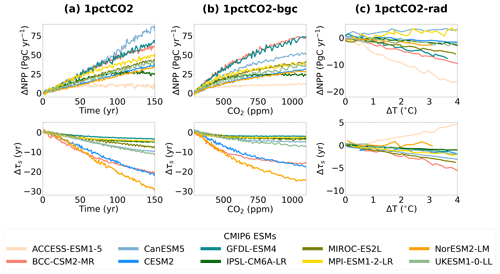
Figure A2Time series of projected changes in net primary productivity (ΔNPP, top row) and soil carbon turnover time (Δτs, bottom row) in CMIP6 ESMs for the idealised simulations 1 % CO2 (a), BGC (b) and RAD (c). This figure has been adapted from Fig. A2 in Varney et al. (2023).
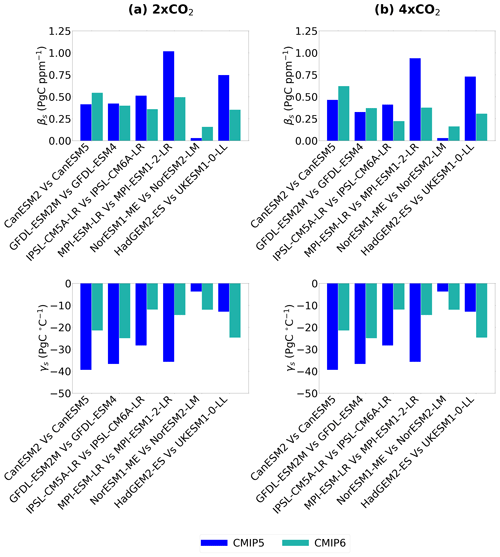
Figure A3Comparison of the soil carbon-concentration (βs) feedback parameters (top row) and the soil carbon-climate (γs) feedback parameters (bottom row) from generationally related ESMs from CMIP5 and CMIP6, for (a) 2 × CO2 and (b) 4 × CO2.
Table A1The land carbon-concentration (βL, PgC ppm−1) and carbon-climate (γL, PgC °C−1) feedback parameters for 2 × CO2 and 4 × CO2 for the CMIP6 ESMs.
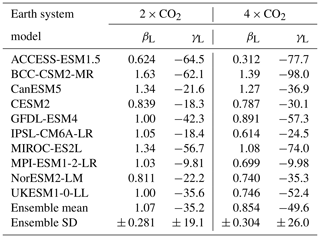
Table A2The CMIP5 Earth system models included in this study and the relevant features of the associated land carbon cycle components: simulation of interactive nitrogen, the inclusion of dynamic vegetation and the soil decomposition functions used (Varney et al., 2022; Arora et al., 2013; Anav et al., 2013; Friedlingstein et al., 2014). Explanations of the temperature and moisture functions used within ESMs are given in Varney et al. (2022) and Todd-Brown et al. (2013).
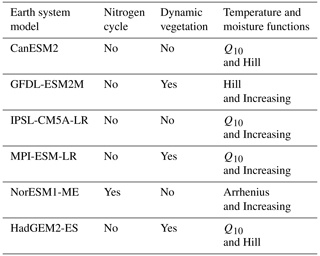
Code is available on GitHub (https://github.com/rebeccamayvarney/CMIP6-soil-beta-gamma, last access: 10 January 2024) and Zenodo (https://doi.org/10.5281/zenodo.10927091, Varney, 2024).
The CMIP6 and CMIP5 data analysed during this study are available online (CMIP6: https://esgf-node.llnl.gov/search/cmip6/, ESGF, 2024a, CMIP5: https://esgf-node.llnl.gov/search/cmip5/, ESGF, 2024b).
RMV and PMC outlined the study. RMV completed the analysis and produced the figures. All the co-authors provided useful guidance on the study at various times and suggested edits to the draft manuscript.
The contact author has declared that none of the authors has any competing interests.
Publisher’s note: Copernicus Publications remains neutral with regard to jurisdictional claims made in the text, published maps, institutional affiliations, or any other geographical representation in this paper. While Copernicus Publications makes every effort to include appropriate place names, the final responsibility lies with the authors.
We thank the World Climate Research Programme’s Working Group on Coupled Modelling and the climate modelling groups for producing and making their model output available.
This research has been supported by the European Research Council's Climate–Carbon Interactions in the Current Century project (4C; grant no. 821003) (Rebecca M. Varney, Peter M. Cox and Pierre Friedlingstein) and the Emergent Constraints on Climate–Land feedbacks in the Earth system project (ECCLES; grant no. 742472) (Rebecca M. Varney and Peter M. Cox). Sarah E. Chadburn was supported by a Natural Environment Research Council independent research fellowship (grant no. NE/R015791/1). Eleanor J. Burke was supported by the joint UK BEIS–Defra Met Office Hadley Centre Climate Programme (grant no. GA01101).
This paper was edited by Daniel S. Goll and reviewed by Ben Bond-Lamberty and one anonymous referee.
Anav, A., Friedlingstein, P., Kidston, M., Bopp, L., Ciais, P., Cox, P., Jones, C., Jung, M., Myneni, R., and Zhu, Z.: Evaluating the land and ocean components of the global carbon cycle in the CMIP5 Earth System Models, J. Climate, 26, 6801–6843, 2013. a
Arora, V. K., Boer, G. J., Friedlingstein, P., Eby, M., Jones, C. D., Christian, J. R., Bonan, G., Bopp, L., Brovkin, V., Cadule, P., and Hajima, T.: Carbon–concentration and carbon–climate feedbacks in CMIP5 Earth system models, J. Climate, 26, 5289–5314, 2013. a, b, c, d
Arora, V. K., Katavouta, A., Williams, R. G., Jones, C. D., Brovkin, V., Friedlingstein, P., Schwinger, J., Bopp, L., Boucher, O., Cadule, P., Chamberlain, M. A., Christian, J. R., Delire, C., Fisher, R. A., Hajima, T., Ilyina, T., Joetzjer, E., Kawamiya, M., Koven, C. D., Krasting, J. P., Law, R. M., Lawrence, D. M., Lenton, A., Lindsay, K., Pongratz, J., Raddatz, T., Séférian, R., Tachiiri, K., Tjiputra, J. F., Wiltshire, A., Wu, T., and Ziehn, T.: Carbon–concentration and carbon–climate feedbacks in CMIP6 models and their comparison to CMIP5 models, Biogeosciences, 17, 4173–4222, https://doi.org/10.5194/bg-17-4173-2020, 2020. a, b, c, d, e, f, g, h
Boer, G. and Arora, V.: Feedbacks in emission-driven and concentration-driven global carbon budgets, J. Climate, 26, 3326–3341, 2013. a
Burke, E. J., Zhang, Y., and Krinner, G.: Evaluating permafrost physics in the Coupled Model Intercomparison Project 6 (CMIP6) models and their sensitivity to climate change, The Cryosphere, 14, 3155–3174, https://doi.org/10.5194/tc-14-3155-2020, 2020. a
Canadell, J., Monteiro, P., Costa, M., Cotrim da Cunha, L., Cox, P., Eliseev, A., Henson, S., Ishii, M., Jaccard, S., Koven, C., Lohila, A., Patra, P., Piao, S., Rogelj, J., Syampungani, S., Zaehle, S., and Zickfeld, K.: Global Carbon and other Biogeochemical Cycles and Feedbacks, Cambridge University Press, Cambridge, United Kingdom and New York, NY, USA, https://doi.org/10.1017/9781009157896.007, 2021. a, b
Cox, P. M., Betts, R., Collins, M., Harris, P. P., Huntingford, C., and Jones, C.: Amazonian forest dieback under climate-carbon cycle projections for the 21st century, Theor. Appl. Climatol., 78, 137–156, 2004. a
Crowther, T. W., Todd-Brown, K. E., Rowe, C. W., Wieder, W. R., Carey, J. C., Machmuller, M. B., Snoek, B. L., Fang, S., Zhou, G., Allison, S. D., and Blair, J. M.: Quantifying global soil carbon losses in response to warming, Nature, 540, 104–108, 2016. a
Davies-Barnard, T., Meyerholt, J., Zaehle, S., Friedlingstein, P., Brovkin, V., Fan, Y., Fisher, R. A., Jones, C. D., Lee, H., Peano, D., Smith, B., Wårlind, D., and Wiltshire, A. J.: Nitrogen cycling in CMIP6 land surface models: progress and limitations, Biogeosciences, 17, 5129–5148, https://doi.org/10.5194/bg-17-5129-2020, 2020. a
ESGF: CMIP6, ESGF [data set], https://esgf-node.llnl.gov/search/cmip6/, last access: 4 February 2024a. a
ESGF: CMIP5, ESGF [data set], https://esgf-node.llnl.gov/search/cmip5/, last access: 6 February 2024b. a
Eyring, V., Bony, S., Meehl, G. A., Senior, C. A., Stevens, B., Stouffer, R. J., and Taylor, K. E.: Overview of the Coupled Model Intercomparison Project Phase 6 (CMIP6) experimental design and organization, Geosci. Model Dev., 9, 1937–1958, https://doi.org/10.5194/gmd-9-1937-2016, 2016. a, b
Friedlingstein, P., Dufresne, J.-L., Cox, P., and Rayner, P.: How positive is the feedback between climate change and the carbon cycle?, Tellus B, 55, 692–700, 2003. a, b
Friedlingstein, P., Cox, P., Betts, R., Bopp, L., von Bloh, W., Brovkin, V., Cadule, P., Doney, S., Eby, M., Fung, I., and Bala, G.: Climate–carbon cycle feedback analysis: results from the C4MIP model intercomparison, J. Climate, 19, 3337–3353, 2006. a, b, c, d, e, f, g, h
Friedlingstein, P., Meinshausen, M., Arora, V. K., Jones, C. D., Anav, A., Liddicoat, S. K., and Knutti, R.: Uncertainties in CMIP5 climate projections due to carbon cycle feedbacks, J. Climate, 27, 511–526, 2014. a
Friedlingstein, P., O'Sullivan, M., Jones, M. W., Andrew, R. M., Gregor, L., Hauck, J., Le Quéré, C., Luijkx, I. T., Olsen, A., Peters, G. P., Peters, W., Pongratz, J., Schwingshackl, C., Sitch, S., Canadell, J. G., Ciais, P., Jackson, R. B., Alin, S. R., Alkama, R., Arneth, A., Arora, V. K., Bates, N. R., Becker, M., Bellouin, N., Bittig, H. C., Bopp, L., Chevallier, F., Chini, L. P., Cronin, M., Evans, W., Falk, S., Feely, R. A., Gasser, T., Gehlen, M., Gkritzalis, T., Gloege, L., Grassi, G., Gruber, N., Gürses, Ö., Harris, I., Hefner, M., Houghton, R. A., Hurtt, G. C., Iida, Y., Ilyina, T., Jain, A. K., Jersild, A., Kadono, K., Kato, E., Kennedy, D., Klein Goldewijk, K., Knauer, J., Korsbakken, J. I., Landschützer, P., Lefèvre, N., Lindsay, K., Liu, J., Liu, Z., Marland, G., Mayot, N., McGrath, M. J., Metzl, N., Monacci, N. M., Munro, D. R., Nakaoka, S.-I., Niwa, Y., O'Brien, K., Ono, T., Palmer, P. I., Pan, N., Pierrot, D., Pocock, K., Poulter, B., Resplandy, L., Robertson, E., Rödenbeck, C., Rodriguez, C., Rosan, T. M., Schwinger, J., Séférian, R., Shutler, J. D., Skjelvan, I., Steinhoff, T., Sun, Q., Sutton, A. J., Sweeney, C., Takao, S., Tanhua, T., Tans, P. P., Tian, X., Tian, H., Tilbrook, B., Tsujino, H., Tubiello, F., van der Werf, G. R., Walker, A. P., Wanninkhof, R., Whitehead, C., Willstrand Wranne, A., Wright, R., Yuan, W., Yue, C., Yue, X., Zaehle, S., Zeng, J., and Zheng, B.: Global Carbon Budget 2022, Earth Syst. Sci. Data, 14, 4811–4900, https://doi.org/10.5194/essd-14-4811-2022, 2022. a
Green, J. K., Seneviratne, S. I., Berg, A. M., Findell, K. L., Hagemann, S., Lawrence, D. M., and Gentine, P.: Large influence of soil moisture on long-term terrestrial carbon uptake, Nature, 565, 476–479, 2019. a
Gregory, J. M., Jones, C., Cadule, P., and Friedlingstein, P.: Quantifying carbon cycle feedbacks, J. Climate, 22, 5232–5250, 2009. a, b, c
IPCC: Climate Change 2021: The Physical Science Basis, Contribution of Working Group I to the Sixth Assessment Report of the Intergovernmental Panel on Climate Change, Cambridge University Press, Cambridge, UK and New York, NY, USA, https://doi.org/10.1017/9781009157896, 2021. a
Jones, A. D., Calvin, K. V., Shi, X., Di Vittorio, A. V., Bond-Lamberty, B., Thornton, P. E., and Collins, W. D.: Quantifying human-mediated carbon cycle feedbacks, Geophys. Res. Lett., 45, 11–370, 2018. a
Jones, C. D., Arora, V., Friedlingstein, P., Bopp, L., Brovkin, V., Dunne, J., Graven, H., Hoffman, F., Ilyina, T., John, J. G., Jung, M., Kawamiya, M., Koven, C., Pongratz, J., Raddatz, T., Randerson, J. T., and Zaehle, S.: C4MIP – The Coupled Climate–Carbon Cycle Model Intercomparison Project: experimental protocol for CMIP6, Geosci. Model Dev., 9, 2853–2880, https://doi.org/10.5194/gmd-9-2853-2016, 2016. a, b
Koven, C. D., Chambers, J. Q., Georgiou, K., Knox, R., Negron-Juarez, R., Riley, W. J., Arora, V. K., Brovkin, V., Friedlingstein, P., and Jones, C. D.: Controls on terrestrial carbon feedbacks by productivity versus turnover in the CMIP5 Earth System Models, Biogeosciences, 12, 5211–5228, https://doi.org/10.5194/bg-12-5211-2015, 2015. a, b
Koven, C. D., Hugelius, G., Lawrence, D. M., and Wieder, W. R.: Higher climatological temperature sensitivity of soil carbon in cold than warm climates, Nat. Clim. Change, 7, 817–822, 2017. a
Lawrence, D. M., Fisher, R. A., Koven, C. D., Oleson, K. W., Swenson, S. C., Bonan, G., Collier, N., Ghimire, B., van Kampenhout, L., Kennedy, D., and Kluzek, E.: The Community Land Model version 5: Description of new features, benchmarking, and impact of forcing uncertainty, J. Adv. Model. Earth Sy., 11, 4245–4287, 2019. a
Pugh, T. A. M., Jones, C. D., Huntingford, C., Burton, C., Arneth, A., Brovkin, V., Ciais, P., Lomas, M., Robertson, E., Piao, S. L., and Sitch, S.: A large committed long-term sink of carbon due to vegetation dynamics, Earth's Future, 6, 1413–1432, 2018. a
Schimel, D., Pavlick, R., Fisher, J. B., Asner, G. P., Saatchi, S., Townsend, P., Miller, C., Frankenberg, C., Hibbard, K., and Cox, P.: Observing terrestrial ecosystems and the carbon cycle from space, Glob. Change Biol., 21, 1762–1776, 2015. a
Schuur, E. A., McGuire, A. D., Schädel, C., Grosse, G., Harden, J. W., Hayes, D. J., Hugelius, G., Koven, C. D., Kuhry, P., Lawrence, D. M., and Natali, S. M.: Climate change and the permafrost carbon feedback, Nature, 520, 171–179, 2015. a
Schwinger, J., Tjiputra, J. F., Heinze, C., Bopp, L., Christian, J. R., Gehlen, M., Ilyina, T., Jones, C. D., Salas-Mélia, D., Segschneider, J., and Séférian, R.: Nonlinearity of ocean carbon cycle feedbacks in CMIP5 earth system models, J. Climate, 27, 3869–3888, 2014. a
Taylor, K. E., Stouffer, R. J., and Meehl, G. A.: An overview of CMIP5 and the experiment design, Bull. Am. Meteorol. Soc., 93, 485–498, 2012. a
Todd-Brown, K. E. O., Randerson, J. T., Post, W. M., Hoffman, F. M., Tarnocai, C., Schuur, E. A. G., and Allison, S. D.: Causes of variation in soil carbon simulations from CMIP5 Earth system models and comparison with observations, Biogeosciences, 10, 1717–1736, https://doi.org/10.5194/bg-10-1717-2013, 2013. a, b, c
Todd-Brown, K. E. O., Randerson, J. T., Hopkins, F., Arora, V., Hajima, T., Jones, C., Shevliakova, E., Tjiputra, J., Volodin, E., Wu, T., Zhang, Q., and Allison, S. D.: Changes in soil organic carbon storage predicted by Earth system models during the 21st century, Biogeosciences, 11, 2341–2356, https://doi.org/10.5194/bg-11-2341-2014, 2014. a, b, c
Varney, R.: Soil carbon-concentration and carbon-climate feedbacks in CMIP6 Earth system models, Zenodo [code], https://doi.org/10.5281/zenodo.10927091, 2024. a
Varney, R. M., Chadburn, S. E., Friedlingstein, P., Burke, E. J., Koven, C. D., Hugelius, G., and Cox, P. M.: A spatial emergent constraint on the sensitivity of soil carbon turnover to global warming, Nat. Commun., 11, 5544, https://doi.org/10.1038/s41467-020-19208-8, 2020. a, b, c
Varney, R. M., Chadburn, S. E., Burke, E. J., and Cox, P. M.: Evaluation of soil carbon simulation in CMIP6 Earth system models, Biogeosciences, 19, 4671–4704, https://doi.org/10.5194/bg-19-4671-2022, 2022. a, b, c, d, e, f
Varney, R. M., Chadburn, S. E., Burke, E. J., Wiltshire, A. J., and Cox, P. M.: Simulated responses of soil carbon to climate change in CMIP6 Earth System Models: the role of false priming, EGUsphere [preprint], https://doi.org/10.5194/egusphere-2023-383, 2023. a, b, c, d, e, f, g, h, i
Wang, S., Zhang, Y., Ju, W., Chen, J. M., Ciais, P., Cescatti, A., Sardans, J., Janssens, I. A., Wu, M., Berry, J. A., and Campbell, E.: Recent global decline of CO2 fertilization effects on vegetation photosynthesis, Science, 370, 1295–1300, 2020. a
Wieder, W. R., Cleveland, C. C., Lawrence, D. M., and Bonan, G. B.: Effects of model structural uncertainty on carbon cycle projections: biological nitrogen fixation as a case study, Environ. Res. Lett., 10, 044016, https://doi.org/10.1088/1748-9326/10/4/044016, 2015. a
Zhou, T., Shi, P., Hui, D., and Luo, Y.: Global pattern of temperature sensitivity of soil heterotrophic respiration (Q10) and its implications for carbon-climate feedback, J. Geophys. Res.-Biogeo., 114, G02016, https://doi.org/10.1029/2008JG000850, 2009. a
Zickfeld, K., Eby, M., Matthews, H. D., Schmittner, A., and Weaver, A. J.: Nonlinearity of carbon cycle feedbacks, J. Climate, 24, 4255–4275, 2011. a, b, c