the Creative Commons Attribution 4.0 License.
the Creative Commons Attribution 4.0 License.
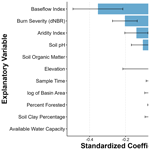
The influence of burn severity on dissolved organic carbon concentrations across a stream network differs based on seasonal wetness conditions
Katie A. Wampler
Kevin D. Bladon
Allison N. Myers-Pigg
Large, high-severity wildfires in many regions across the globe have increased concerns about their impacts on carbon cycling in watersheds. Altered sources of carbon and changes in catchment hydrology after wildfire can lead to shifts in dissolved organic carbon (DOC) concentrations in streams, which can have negative impacts on aquatic ecosystem health and downstream drinking-water treatment. Despite its importance, post-fire DOC responses remain relatively unconstrained in the literature, and we lack critical knowledge of how burn severity, landscape elements, and climate interact to affect DOC concentrations. To improve our understanding of the impact of burn severity on DOC concentrations, we measured DOC at 129 sites across a stream network extending upstream, within, and downstream of a large, high-severity wildfire in Oregon, USA. We collected samples across the study sub-basin during four distinct seasonal wetness conditions. We used our high-spatial-resolution data to develop spatial stream network (SSN) models to predict DOC across the stream network and to improve our understanding of the controls on DOC concentrations. Spatially, we found no obvious wildfire signal – instead, we observed a pattern of increasing DOC concentrations from the high-elevation headwaters to the sub-basin outlet, while the mainstem maintained consistently low DOC concentrations. This suggests that effects from large wildfires may be “averaged” out at higher stream orders and larger spatial scales. When we grouped DOC concentrations by burn severity group, we observed a significant decrease in the variability of DOC concentrations in the moderate and high burn severity sub-catchments. However, our SSN models were able to predict decreases in DOC concentrations with increases in burn severity across the stream network. Decreases in DOC concentrations were also highly variable across seasonal wetness conditions, with the greatest (−1.40 to −1.64 mg L−1) decrease occurring in the high-severity group during the wetting season. Additionally, our models indicated that in all seasons, baseflow index was more influential in predicting DOC concentrations than burn severity was, indicating that groundwater discharge can obscure the impacts of wildfire in a stream network. Overall, our results suggested that landscape characteristics can regulate the DOC response to wildfire. Moreover, our results also indicated that the seasonal timing of sampling can influence the observed response of DOC concentrations to wildfire.
- Article
(13827 KB) - Full-text XML
- BibTeX
- EndNote
Streams play an active role in transporting and processing terrestrial inputs of carbon from the landscape to oceans (Cole et al., 2007). It has been estimated that terrestrial ecosystems deliver between 1.1–5.1 Pg of carbon annually to inland waters (Drake et al., 2018). Thus, landscape-scale disturbances – such as extreme weather events, forest harvesting, insect and pathogen outbreaks, and wildfire – have the potential to substantially impact regional carbon cycling by altering the sources, transport, and processing of terrestrial organic matter (Achat et al., 2015; Amiro et al., 2009; Brando et al., 2019; Chambers et al., 2007; Kurz et al., 2008). In particular, impacts from large, high-severity wildfires are of increasing concern due to shifting wildfire regimes, which have led to recent and particularly notable wildfire seasons across many regions of the globe, including the Pacific Northwest (Abatzoglou et al., 2021; de la Barrera et al., 2018; Boer et al., 2020; Dodd et al., 2018; Lagouvardos et al., 2019; Turco et al., 2019). These extreme fire seasons have been primarily attributed to increasing temperatures and longer periods of fire weather due to climate change (Abatzoglou et al., 2018; Duane et al., 2021; Pausas and Keeley, 2021).
Wildfires can alter terrestrial sources of carbon through the significant loss and modification of vegetation and organic and shallow mineral soil layers (Johnson et al., 2007; Miesel et al., 2018). While a large portion of this burned material is volatized to the atmosphere as CO2 and other gases, an estimated 1 %–28 % of the carbon is transformed to pyrogenic organic matter (PyOM) (Forbes et al., 2006; Preston and Schmidt, 2006; Santín et al., 2015). PyOM contains a spectrum of molecules with labilities that vary based on the combustion temperature, the characteristics of the burned material, and the formation mechanisms (Masiello, 2004; Wagner et al., 2018). However, there is evidence that this PyOM can impact ecosystem functioning and drinking-water treatment (Hohner et al., 2017; Emelko et al., 2011). Since terrestrial ecosystems are a primary source of carbon for inland waters like streams, the wildfire-altered terrestrial carbon stocks can alter the amount and characteristics of dissolved and particulate organic carbon delivered to streams.
Wildfire can also substantially alter the catchment hydrology and flow paths of water through the landscape, further impacting dissolved organic carbon (DOC) transport from the terrestrial landscapes to streams. Specifically, the loss of vegetation post-fire often leads to decreased evapotranspiration (Ma et al., 2020; Nolan et al., 2014; Poon and Kinoshita, 2018) and increased net precipitation (Kusaka et al., 1983; Stoof et al., 2012; Williams et al., 2019). Moreover, wildfires can affect soil physicochemical properties, resulting in increased soil–water repellency, soil sealing, surface crust formation, soil pore clogging, and changes in bulk density due to the collapse of soil aggregates, further leading to shifts in hydrologic flow paths from hillslopes to streams (Balfour et al., 2014; Doerr et al., 2009; Ebel and Moody, 2017; Larson-Nash et al., 2018). These effects on soil hydraulic properties can produce increased surface runoff, lateral flow, or groundwater, increasing the potential for peak flows and annual water yields (Atwood et al., 2023; Jung et al., 2009; Onda et al., 2008; Rey et al., 2023; Stoof et al., 2014). The post-fire shifts in carbon stocks, hydrologic flow paths, and contact times of carbon with soil, water, and microbes thus have the potential to further influence post-fire DOC concentrations in streams (Olefeldt et al., 2013).
With both direct controls on carbon stocks and indirect controls on DOC movement, the net impact of wildfire on DOC in streams remains poorly constrained. With a loss of vegetation and soil organic matter, one might expect to observe decreased DOC concentrations post-fire. Indeed, there have been observations of decreases in DOC concentrations in permafrost and mountainous regions within the US, with declines ranging from ∼11 %–95 % (Betts and Jones, 2009; Caldwell et al., 2020; Chow et al., 2019; Rodríguez-Cardona et al., 2020; Santos et al., 2019). However, with PyOM remaining on the landscape and increased movement of water to streams, there is also the potential for increases in DOC concentrations. This too has been observed, with increases of ∼3 %–10 000 % measured across Mediterranean, humid, semi-arid, and subarctic climates (Burton et al., 2016; Caldwell et al., 2020; Chow et al., 2019; Emelko et al., 2011; Harris et al., 2015; Hohner et al., 2016; Oliver et al., 2012; Revchuk and Suffet, 2014; Uzun et al., 2020; Vila-Escalé et al., 2007). Wildfires across the Western US have also resulted in minimal to no impacts on in-stream DOC (Mast and Clow, 2008; Uzun et al., 2020; Wagner et al., 2015). The inconsistency in observed responses may also be due to the spatial variability in DOC across a stream network, which arises from the fact that DOC is not inertly transported and can lead to nonlinear trends across space (Casas-Ruiz et al., 2020; Wollheim et al., 2015). However, the impact of wildfire on the spatial variability across stream networks remains largely unexplored. Overall, the inconsistency of post-fire responses highlights the need to better understand the controls on stream DOC concentrations across burned basins.
In unburned landscapes, in-stream DOC concentrations tend to be tightly related to hydrology. Periods of increased streamflow are often associated with increased DOC concentrations (Butturini and Sabater, 2000; Koehler et al., 2009; Raymond and Saiers, 2010). However, this can also depend on the seasonal wetness conditions within the basin or sub-annual wetting and drying periods. For example, snowmelt or rewetting after long dry periods can cause increases in DOC that peak prior to streamflow (Dawson et al., 2008; Hornberger et al., 1994; Humbert et al., 2015; Lambert et al., 2013). This is often referred to as flushing, where finite pools of DOC are reconnected during the rewetting periods, causing temporary increases in DOC until the source is depleted (Hornberger et al., 1994).
Differences in seasonal wetness conditions can also impact the importance of other basin characteristics in controlling DOC concentrations (Ågren et al., 2007). Other basin characteristics that have been related to DOC concentrations include aridity, where more arid areas have been correlated with higher DOC concentrations (Kerins and Li, 2023). Vegetation type can also control the quality and leachability of the carbon (van den Berg et al., 2012), while elevation can control air and soil temperatures, which have been linked to microbial activity and DOC production in soils (Kalbitz et al., 2000). Subsurface soil properties like texture and organic matter content can influence the amount of carbon that is produced, held in soils, and available to leach, affecting stream DOC concentrations (van den Berg et al., 2012; Futter et al., 2007; Nelson et al., 1992; Wilson and Xenopoulos, 2008). Groundwater sourced from mineral soils or bedrock is usually quite low in DOC (Leenheer et al., 1974), and therefore higher groundwater contributions may result in lower stream DOC concentrations. Lastly, DOC concentrations have been related to basin area, although the direction of the impact is variable (Ågren et al., 2007; Mulholland, 1997), with some suggesting that increasing the basin area only acts to remove “extreme” measurements (Creed et al., 2015).
In burned landscapes, additional factors can also influence DOC concentrations. For example, DOC concentrations may be affected by the revegetation and recovery of landscapes over time after wildfire. While some have estimated that the recovery period is as short as 7 months (Wei et al., 2021), several studies have illustrated that wildfire effects may persist for 10 or more years (Chow et al., 2019; Santos et al., 2019; Parham et al., 2013; Rodríguez-Cardona et al., 2020). Moreover, results from several meta-analyses have shown that impacts from wildfire on DOC concentrations may persist for at least 5 years (Cavaiani et al., 2024; Raoelison et al., 2023; Hampton et al., 2022; Rust et al., 2019). Post-fire DOC concentrations can also be dependent on area burned (Rhoades et al., 2019; Uzun et al., 2020; Chow et al., 2019) and fire severity (Santos et al., 2019). In particular, increases in burn severity have previously been related to decreased DOC concentrations during baseflow conditions (Santos et al., 2019). However, the authors from the same study noted that site-level characteristics were also important in controlling solute responses. While existing work suggests that burn severity is highly influential on post-fire DOC concentrations, there have been few studies on this topic, and those studies are limited to a small number of sites and burn-severity groups (Santos et al., 2019). To improve our understanding of the impact of burn severity on DOC, a greater range of sites, burn severities, and streamflow conditions are needed to improve our knowledge of how wildfire effects on DOC propagate through a stream network. Additionally, while previous work has indicated that site-level characteristics can also be important post-fire, the importance of burn severity relative to landscape characteristics remains unknown.
In our study, we collected stream water samples to quantify DOC across space and time in a burned sub-basin with a range of landscape characteristics and burn severities in the Pacific Northwest, USA. We collected water samples across four distinct hydrologic time periods to answer the following questions. (1) How does DOC vary spatially across a stream network upstream, within, and downstream of a burn? (2) How do stream DOC concentrations vary within the basin according to the burn severity and the antecedent seasonal wetness conditions? (3) What are the relative influences of fire, climate, and landscape characteristics on DOC concentrations, and how do they vary with the antecedent seasonal wetness conditions?
2.1 Study area
The McKenzie River sub-basin (HUC 17090004) is a 3461 km2 catchment that is nested in the Willamette River basin, which is a tributary of the greater Columbia River basin on the west side of the Cascade Range in Oregon, USA (Fig. 1a). The sub-basin is the primary source of drinking water for ∼200 000 residents near Springfield and Eugene, OR. The upper two-thirds of the sub-basin are federally owned, while the lower one-third is a combination of industrial timberland, agricultural lands, and private ownership. The sub-basin is ∼85 % forested with primarily Douglas-fir (Pseudotsuga menziesii), western hemlock (Tsuga heterophylla), and Pacific silver fir (Abies amabilis). The elevation in the sub-basin ranges from 111 to 3149 m, with a median elevation of 954 m. The mean slope in the sub-basin is ∼16°, while the maximum slope reaches ∼50°. Across the sub-basin, the average annual maximum temperature ranges from 8.7 to 17.6 °C, while the average annual minimum temperature ranges from −2.3 to 6.2 °C (PRISM Climate Group, 2012). The sub-basin has a Mediterranean climate with cool, wet winters and dry, warm summers (Kottek et al., 2006; Snyder et al., 2002). The sub-basin receives approximately 2200 mm of annual precipitation, but spatially this can range from ∼1000 to 3500 mm, primarily due to orographic effects (PRISM Climate Group, 2012). At lower elevations, the precipitation falls almost exclusively as rain, but above ∼1200 m, the precipitation is generally snowfall dominated.
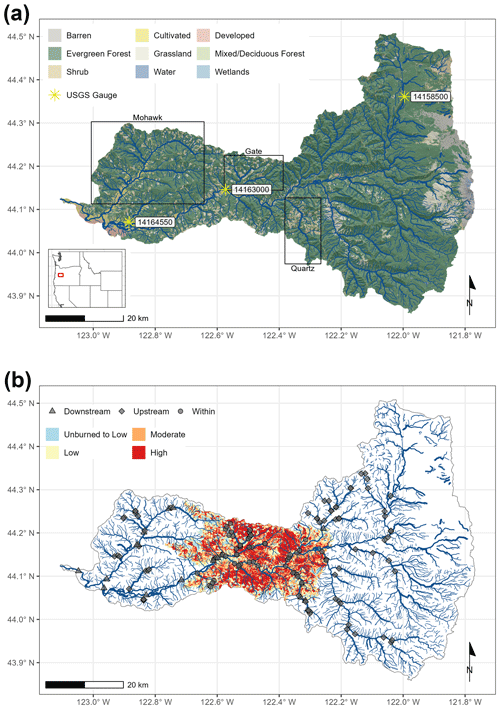
Figure 1(a) Map of the McKenzie River sub-basin, Oregon, USA (U.S. Geological Survey, 2020), and associated land uses (Dewitz, 2021). Sub-basins of particular interest and USGS gauges used in Fig. 2 are labeled. (b) Map of the burn severity of the 2020 Holiday Farm wildfire (MTBS Project, 2021) and water-sampling sites distributed across the stream network in the McKenzie sub-basin. The shape of the marker indicates the location of the site relative to the perimeter of the Holiday Farm wildfire.
The geology of the region consists of young volcanics (Late High Cascade Volcanics) in the upper basin, which is characterized by relatively flat slopes and high-permeability layers, resulting in substantial groundwater and springs across the region (Tague et al., 2008). In the lower portions of the sub-basin, old volcanics (Little Butte Volcanics and Late Western Cascade Volcanics) dominate and are characterized by steeper slopes with less groundwater discharge to streams (Tague et al., 2008).
In September 2020, the Holiday Farm fire burned ∼18 % (629 km2) of the McKenzie River sub-basin. This fire was noteworthy partially due to its size as well as its location, which was directly on the mainstem of the McKenzie River in the middle elevations of the basin (Fig. 1b). The fire was also relatively high severity, with the area burned classified as: <1 % increased greenness, 9.2 % unburned to low, 26.9 % low, 29.4 % moderate, and 33.3 % high severity (MTBS Project, 2021). Monitoring Trends in Burn Severity (MTBS) burn severity is determined from the difference in satellite-derived normalized burn ratio (dNBR) from the pre-fire to the post-fire period, with the burn severity classifications assigned based on thresholds in the data.
2.2 Site selection and sample collection
To measure the spatial variability of DOC across seasons, we selected 129 stream sites across the McKenzie sub-basin. There were 65 sites upstream of the Holiday Farm fire, 54 sites within the burn perimeter, and 10 sites downstream of the fire (Fig. 1b). We selected sites near confluences where we could collect water samples both upstream and downstream of a tributary. We also targeted an even spatial distribution of sample sites above, within, and downstream of the Holiday Farm fire burn perimeter to encompass the landscape and climate variability within the sub-basin.
We sampled our sites four times throughout the year to capture the general seasonal variation in DOC associated with catchment wetness (Fig. 2, Table A1). The first sampling campaign was on 1 November 2022, during one of the first few rain events in the fall as the basin was starting to re-wet. Our second sampling was on 13 March 2023, during a storm in the wet season (e.g., winter). The third was on 11 June 2023, during the drying period (e.g., late spring). Lastly, we sampled on 11 September 2023, which was toward the end of the dry period (e.g., summer). The number of sites sampled varied slightly by sampling campaign due to limitations on access caused by high flows, snow, and additional wildfire restrictions (Table A1).
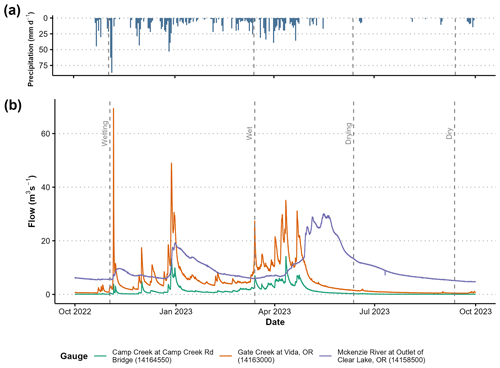
Figure 2(a) Daily precipitation from October 2022 to October 2023 in the McKenzie River sub-basin (44.2119, −122.2559) (Daly, 2023). (b) Discharge at three USGS stream gauges throughout the McKenzie sub-basin from October 2022 to October 2023, showing discharge patterns for the lower (Camp Creek), middle (Gate Creek), and upper (McKenzie River at Outlet of Clear Lake) regions of the sub-basin. Dates of water sample collection are labeled with vertical dashed lines. Data were obtained using the dataRetrieval package in R (Cicco et al., 2018; U.S. Geological Survey, 2024).
We collected stream water samples from highly mixed sections of the stream using extendable grab samplers. The grab sampler bottle was rinsed three times in the stream before collecting the sample. When feasible, samples were syringe filtered in the field using 0.2 µm PES syringe filters into acid washed and triple rinsed amber HDPE bottles. Samples that were too turbid to field filter were vacuum filtered in the lab within 24 h of collection using 0.2 µm PES filters. Samples were kept at 4 °C after collection. Samples that were not able to be run within 7 days of collection were frozen at −15 °C until analysis. Studies have shown that when DOC concentrations are low, as was observed across our study sub-basin (Carpenter et al., 2022; Kraus et al., 2010), freezing does not have demonstrable impacts on DOC concentration measurements (Fellman et al., 2008; Tupas et al., 1994).
In the laboratory, we analyzed the samples for the dissolved organic carbon (DOC) concentration (Shimadzu TOC-VCSH combustion analyzer). The analyzer acidified the samples and purged them to remove inorganic carbon, then the samples were combusted at 680 °C to convert all the remaining carbon (i.e., non-purgeable organic carbon) to CO2, which was measured with an infrared detector.
2.3 Spatial stream network models
We used spatial stream network (SSN) models to explore the spatial variability of DOC across the stream network and determine factors influencing DOC concentrations. SSN models are multiple linear regression models with added variance to account for spatial autocorrelation along the network (Ver Hoef and Peterson, 2010). Once a model is built, the response variable can be predicted at high resolution across the network using generated prediction points. For predictions across the basin, we used prediction points spaced 1 km apart on the network. For two streams within the burn perimeter, Gate Creek and Quartz Creek, we also generated prediction points at 100 m resolution. These streams were chosen because we had a high enough resolution of sampling points along those stream networks to generate satisfactory predictions. Models were built using the STARS toolbox in ArcMap (Peterson and Ver Hoef, 2014) and the SSN package in R (Ver Hoef et al., 2014). We built both a “mean” model, which included all the samples across sampling campaigns to examine the overall trends, and separate models for each sampling campaign because we expected controls on DOC concentrations to differ seasonally.
We used 13 potential explanatory variables to describe the landscape, climate, and fire at each sampling location (Table 1). We used USGS StreamStats (U.S. Geological Survey, 2019) to delineate the upstream area for each site. These polygons were used to determine the mean value of each geospatial explanatory variable at the sampling locations using zonal statistics in R (R Core Team, 2020, Table 1). In particular, burn severity was calculated as the average dNBR value across the upstream contributing area for each site, with unburned areas considered to have dNBR values of 0 (zero). Due to the number of points, basin characteristics at the prediction points were determined using the Watershed Attributes function in STARS; this function is less spatially explicit than StreamStats basin delineation and thus has more error, which is why it was not used for the sampling locations (Peterson and Ver Hoef, 2014).
Model selection was performed using a double-selection procedure (Belloni et al., 2014; Fan and Li, 2012) in which linear models were used to determine the set of explanatory variables to use in the SSN models. We chose to use the double-selection procedure because it is robust, allowing more accurate identification of potential confounding variables. Most importantly, the method prevents inflation of p values and standard errors for our variable of interest, burn severity (Belloni et al., 2014). For the first selection step, a linear model was fitted with DOC concentration as the dependent variable and all the potential explanatory variables besides the variable of interest for this study, which was burn severity. The second selection step used burn severity as the dependent variable and all the potential explanatory variables. Variables were included in the final model if the variable had a p value less than or equal to 0.1 in either selection step. This model selection step was performed for the mean model, which included all the observations. The selected variables from this step were also used in each of the seasonal models except for season, as this was constant in each seasonal model.
Table 1Descriptions of the explanatory variables used in the spatial stream network (SSN) models to model dissolved organic carbon concentrations across the McKenzie sub-basin. P signifies precipitation and ET evapotranspiration.
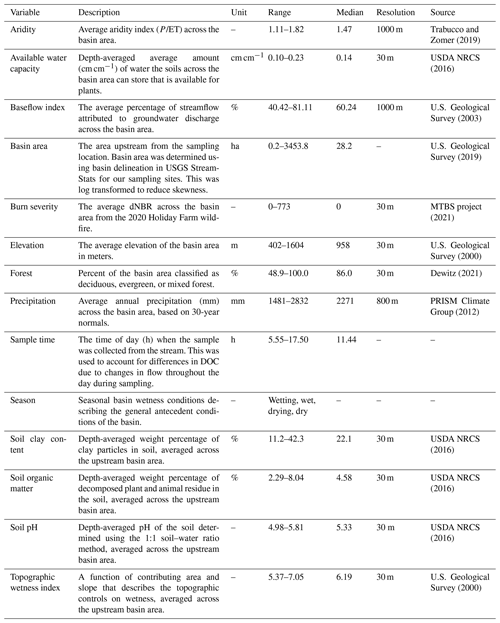
Following explanatory variable selection, the selected variables were used to create the SSN models. We fitted the SSN models by first determining the best autocorrelation structure. This variance was modeled with a moving average function. Autocorrelation can be modeled using a tail-up model, which only considers the autocorrelation between flow-connected streams weighted by watershed area. Additionally, a tail-down model can be used, which considers autocorrelation between both flow-connected and unconnected streams. Finally, a Euclidean distance model can be used; this disregards the stream network distances and uses the 2D distance from each other across the landscape. We tested all combinations of the three autocorrelation models with their averaging functions and chose based on Akaike information criterion (AIC) weight and root mean squared error (RMSE). We determined the best structure using the mean model and kept the same structure for each seasonal model. Since we had repeated observations in the mean model, we added a random factor for the sampling sites. Season was also included in the mean model as a fixed effect but was not included in the seasonal models since they were already separated by season. Lastly, we checked our models by examining the residuals and performing leave-one-out cross-validation to ensure that the assumptions of linearity, normality, and homoscedasticity were met.
2.4 Statistical analysis
Statistical analysis was performed in R (version 4.2.1, R Core Team, 2020). For descriptive statistics, we chose to bin the continuous burn severity dNBR values into “unburned”, “low”, “moderate”, or “high” based on the dNBR burn-severity thresholds determined by MTBS for the Holiday Farm fire specifically (MTBS Project, 2021). dNBR values less than 40 indicated an unburned area, values between 41 and 320 indicated low burn severity, values between 321 and 660 indicated moderate burn severity, and values greater than 661 indicated high burn severity. To avoid extrapolating, we used an upper limit of 772 for high severity, as this was the highest average dNBR at any of our sites. These dNBR thresholds were also used to estimate the impact of burn severity on DOC concentrations using the fitted dNBR coefficient in each SSN model. The standard error of the dNBR coefficient values were used to estimate the upper and lower 95 % confidence intervals for the change in DOC for each severity group.
To test for differences in DOC concentrations across burn severity and seasonal wetness groups, we used a Gaussian-family generalized linear mixed model from the glmmTMB package in R (Brooks et al., 2017), which allowed us to examine both the mean and variance across severity and wetness groups while accounting for the heteroscedasticity between groups and repeated measures across sites. Mean DOC was modeled using burn severity, seasonal wetness, and the interaction between the two, with site as a random variable. The variance was modeled using burn severity and seasonal wetness without an interaction. Confidence intervals for the mean and variance were calculated using the standard errors from the model.
To determine the relative importance of the explanatory variables in each SSN model, the values of each explanatory variable were standardized to a mean of 0 and a standard deviation of 1 by subtracting the mean value and dividing by the standard deviation of each variable. This sets all coefficients on the same scale, so larger coefficients indicate a larger impact on DOC concentrations, while the sign indicates the direction of the impact (positive vs. negative).
3.1 Spatial trends in dissolved organic carbon concentrations
We used our observed measurements of dissolved organic carbon (DOC) to predict DOC concentrations across the stream network using spatial stream network (SSN) models; those predictions were used to visualize spatial patterns across the sub-basin. Overall, we observed a general spatial trend of low DOC concentrations in the eastern headwaters of the sub-basin, with concentrations increasing as the flow moved downstream (west; Fig. 3). This trend was particularly visible in the wetting and dry seasons, but it was also present in the wet and drying seasons. Contrary to expectations, we did not observe an obvious fire signal as the flow moved through the burned area. The measured and predicted DOC concentrations within the fire perimeter did not appear to be distinctively lower or higher than the surrounding areas and were consistent with the east to west pattern observed. We also noted that the mainstem remained low in DOC throughout all the seasonal wetness conditions, regardless of higher DOC inputs from tributaries, especially in the wetting season. One of those tributaries that contributed high DOC concentrations across seasons was the Mohawk tributary in the NW portion of the sub-basin (Fig. 1). It consistently had some of the highest concentrations during each sampling period. The Mohawk tributary is primarily dominated by evergreen forest (80.2 %) and also has a relatively low baseflow index (47.4 %).
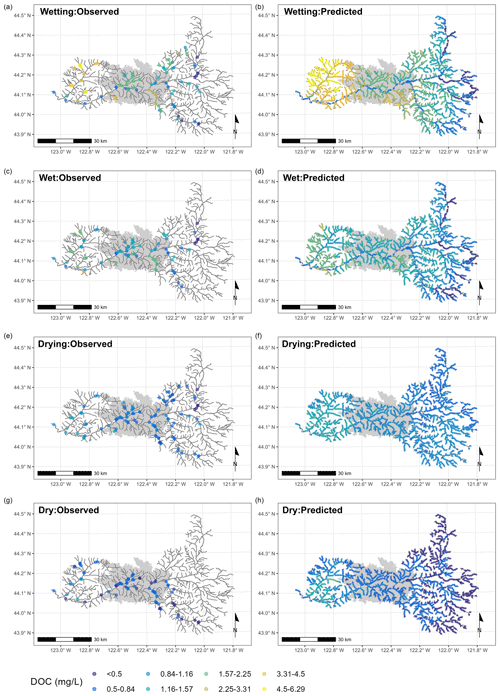
Figure 3Maps showing the observed (left) and predicted (right) dissolved organic carbon concentrations (DOC) across the McKenzie sub-basin, OR, for the four seasonal wetness conditions. The wildfire perimeter is shown in gray. Predictions were obtained using the final fitted spatial stream network (SSN) models and prediction points spaced every 1 km along the stream network. The size of the points in the predictions indicates the prediction error associated with the point.
3.2 Trends in DOC associated with burn severity and seasonal wetness conditions
To numerically explore the impact of burn severity on DOC in the basin, we summarized the observed DOC by burn severity group. Overall, DOC concentrations were relatively low for all severity groups. The mean for the unburned sites was 1.27±1.12 mg L−1, which was slightly greater than for the low-severity sites, with a mean of 1.16±0.83 mg L−1. The moderate-severity sites had a mean concentration of 1.22±0.58 mg L−1. Lastly, the high-severity sites had a mean concentration of 1.08±0.46 mg L−1. A generalized linear mixed model (GLMM) indicated that there was weak to minimal evidence of differences across severity groups (p values: 0.0984–0.7867). While there were no major shifts in DOC concentration, the model indicated significant differences in variance between severity groups. Specifically, the variance of the low-severity group was 0.36 times (95 % confidence interval (CI): 0.33–0.39) the variance of the unburned group. The variance for the moderate-severity group was 0.13 times (95 % CI: 0.12–0.15) the variance of the unburned group, and the variance for the high-severity group was 0.14 times (95 % CI: 0.10–0.19) the variance of the unburned group. While the moderate- and high-severity groups had smaller variance than the low-severity and unburned groups, there was not a distinguishable difference in variance between the moderate- and high-severity groups. The model estimated the ratio of variance between the moderate- and high-severity groups to be 1.04 (95 % CI: 0.76–1.44), but the 95 % CI crossed 1, indicating that at an α level of 0.05, the variance between the two groups was indistinguishable.
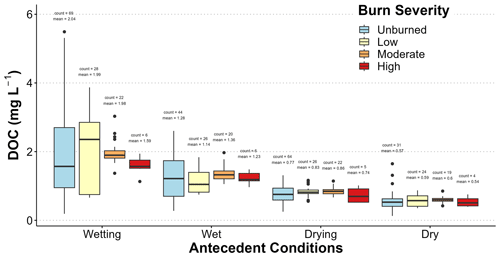
Figure 4The distribution of in-stream DOC concentrations in the McKenzie sub-basin, OR, for each burn-severity group over the four sampling campaigns (1 September 2022, 13 March 2023, 11 June 2023, and 11 September 2023), labeled by the seasonal wetness conditions. The number of samples and mean are labeled above each group.
In addition to burn-severity effects, we also noted that antecedent seasonal wetness conditions had an impact on DOC concentrations. Unlike burn severity, our GLMM model indicated that there was strong evidence (p<0.001; Table A2) of differences in mean DOC concentrations with the seasonal wetness conditions. DOC concentrations were highest in the wetting season, followed by the wet, drying, and dry seasons (Fig. 4). Additionally, the variance of DOC concentration was seasonally dependent, with the greatest variance occurring during the wetting season, with a variance that was 6.7 times (95 % CI: 6.19–7.33) greater than the variance during the wet season. Similarly, the wet season had a variance that was 7.5 times (95 % CI: 6.26–9.06) greater than that during the drying season. Statistically, there was no evidence for a difference in the variance between the drying season and the dry season (ratio =0.90, 95 % CI: 0.66–1.23).
3.3 Spatial stream network models of DOC
We created a spatial stream network (SSN) model using the DOC data collected in our sampling campaigns to predict the landscape, climate, and wildfire factors influencing DOC concentrations and to estimate the impact of burn severity across the McKenzie sub-basin. Post-double selection was used to select 12 of the 14 potential explanatory variables for inclusion in the final model (Table 2). Specifically, annual precipitation and topographic wetness index did not have sufficient empirical support to support inclusion. A search across all potential spatial autocorrelation structures and models resulted in the selection of exponential tail-up and Gaussian Euclidean models (Table 3). Overall, the model fit was moderately strong, with a leave-one-out cross-validation R2 of 0.602 (Table 3). Despite this, the mean model had a large nugget (38.0 %; this is the unexplained variance not explained by the covariates and spatial autocorrelation), suggesting that there was quite a bit of variance unaccounted for in the model. We standardized our non-categorical explanatory variables, allowing us to compare model coefficients. Overall, the most important factor influencing DOC across the sub-basin was baseflow area; this was followed by burn severity, aridity index, and soil pH. However, the confidence intervals for these variables overlap, suggesting uncertainty in the exact order of importance for these variables. All four exhibited negative relationships with DOC concentration (Fig. 5). Conversely, an increase in the percentage of soil organic matter was associated with increased DOC concentrations (Fig. 5). Other factors that were less important but still positively related to DOC were soil clay percentage and available water capacity, while sample time, log of basin area, and percent forested all had negative relationships with DOC (Fig. 5). As expected, given the variation in seasonal concentrations, the antecedent conditions also had a large impact on DOC concentrations. The wetting season had the highest mean DOC concentrations, with predicted decreases in mean DOC of 0.85 mg L−1 in the wet season, 1.18 mg L−1 the drying season, and 1.50 mg L−1 in the dry season.
Table 2Standardized coefficients for the covariates used in the mean model and the four seasonal spatial stream network (SSN) models describing the concentration of dissolved organic carbon (DOC) within the McKenzie sub-basin, OR. All non-categorical explanatory variables were standardized by calculating the z-score for each individual value in the explanatory variable.
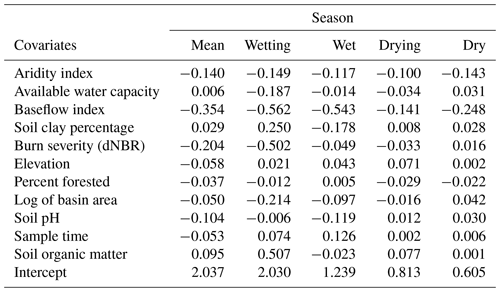
Table 3The proportion of the variance explained by the covariates, the autocorrelation functions (tail-up and Euclidean), and the unexplained variance (nugget). The total autocorrelation variance is the sum explained by the tail-up and Euclidean components (shown in italics). Below the variance metrics is the R2 determined from the leave-one-out cross-validation and the root-mean-squared error for each of the mean model and the four seasonal spatial stream network (SSN) models of dissolved organic carbon (DOC) concentrations in the McKenzie sub-basin, OR.
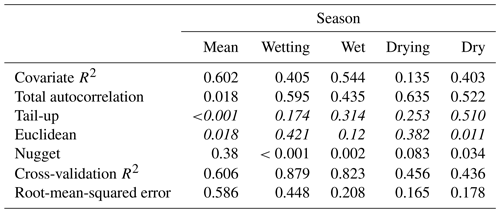
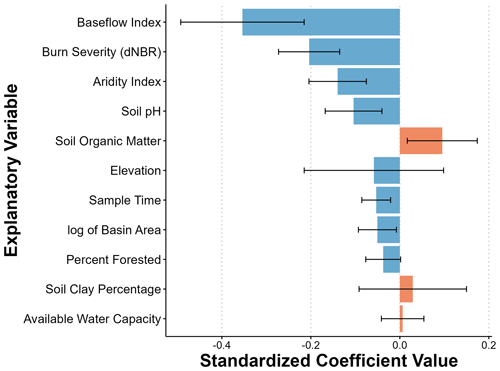
Figure 5The standardized coefficient values of explanatory variables driving spatial differences in dissolved organic carbon (DOC) concentrations across the McKenzie sub-basin, OR, from 2022 to 2023, 2 years post-fire. Values were determined by standardizing the explanatory variables prior to developing a spatial stream network (SSN) model using water quality samples across four distinct seasonal antecedent wetness conditions. The color indicates the direction of the relationship between the variable and DOC concentrations. Error bars indicate the standard error from only the final model. They do not represent the error associated with the model selection performed to determine the final model.
Since seasonal antecedent wetness conditions were strongly related to DOC concentrations and the mean model exhibited substantial unexplained variation, we suspected that the impact of some of the other factors influencing DOC concentration may change seasonally. Because of this, we created a unique SSN model for each antecedent condition. The wetting and wet models exhibited improved fits with leave-one-out cross-validations R2 of 0.879 for the wetting period and 0.823 for the wet period (Table 3). The drying and dry models had poorer fits, with cross-validation R2 values of 0.456 for the drying period and 0.436 for the dry period. Compared to the mean model, all models had smaller nuggets, suggesting that the fixed effects and spatial autocorrelation did a better job of explaining the DOC concentrations in the separate seasonal models (Table 3). Notably, while ∼50 % of the variation in DOC was explained by the covariates in the wetting-, wet,- and dry-season models, they only accounted for 13.5 % of the variance in the drying-season model (Table 3). Interestingly, the majority of the spatial autocorrelation was accounted for by the tail-up (flow-connected) structure in the wet and dry seasons, while the Euclidean structure was most important in the wetting and drying seasons (Table 3).
In terms of factors related to DOC concentrations, the importance and direction of many covariates changed seasonally (Fig. 6). Notably, the percentage of clay in the soil influenced DOC concentrations in both the wetting and wet seasons. However, it was associated with increased DOC during the wetting season but decreased DOC concentrations during the wet season. The percentage of soil organic matter also influenced DOC concentrations in the wetting and drying seasons but appeared to have minimal importance in the wet and dry seasons. Despite some factors being seasonally variable, the most influential factor in the mean model was the baseflow index, which remained the most important variable in all seasons. As the baseflow index increased in a sub-catchment, DOC concentrations consistently decreased. Similarly, as the aridity index increased, DOC concentrations consistently decreased. During the drying and dry seasons, the aridity index was the second most influential variable on DOC concentrations. However, the aridity index tended to have less influence on DOC during the wetting and wet seasons.
Contrary to expectations, burn severity was not the most influential factor on DOC concentrations in any season (Fig. 6). Across seasonal wetness conditions, it was most important in the wetting season, where it was one of the top three factors, similar in magnitude to the impact of soil organic matter. However, in the other seasons, it was only of moderate (wet and drying) or minimal (dry) importance. Overall, like the mean model, the models predicted that an increase in burn severity would decrease DOC concentrations, despite the dry-season model prediction of a slight increase in DOC during that season.
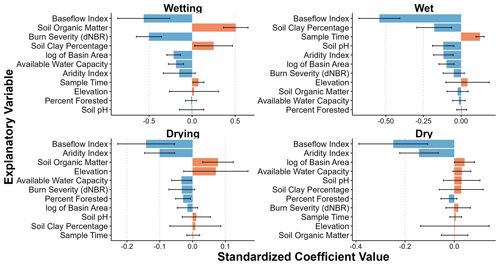
Figure 6The standardized coefficient values of explanatory variables driving spatial differences in dissolved organic carbon (DOC) concentrations across the McKenzie sub-basin, OR, during four distinct seasonal antecedent wetness conditions. Values were determined by standardizing the explanatory variables prior to developing spatial stream network (SSN) models. The color indicates the direction of the relationship between the variable and DOC concentrations. Error bars indicate the standard error from only the final model. They do not represent the error associated with the model selection performed to determine the final model.
To better contextualize our results, we estimated the average change in DOC across each MTBS burn-severity group using the coefficients from our SSN models (Fig. 7). For the high-severity burn classification, DOC concentrations were estimated to decrease by between −1.40 and −1.64 mg L−1 during the wetting season. The impact was much lower in the other seasons, with an estimated decrease of −0.14 to −0.16 mg L−1 for the wet season and −0.09 to −0.10 in the drying season. In the dry season, DOC was predicted to increase by between 0.03 and 0.04 mg L−1. However, it should be noted that the wetting season was the only season for which the 95 % confidence interval did not cross 0, indicating some uncertainty in the directionality of burn-severity influence on DOC concentrations during the wet, drying, and dry seasons (Fig. 7).
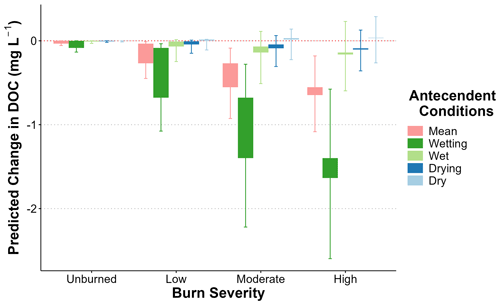
Figure 7The change in dissolved organic carbon (DOC) predicted by each of the seasonal spatial stream network models at each burn-severity level for the McKenzie River sub-basin, OR. Predictions were based on the burn-severity coefficient in the fitted spatial stream network (SSN) models. The central box illustrates the range of change based on the low- and high-threshold values for each burn-severity group. The error bars are based on the standard error of the burn-severity coefficient, where the upper bar is the upper 95 % confidence interval for the low-threshold values, and the lower bar is the lower 95 % confidence interval for the high-threshold values.
4.1 Landscape factors were more influential than burn severity in controlling DOC concentrations
In our analysis of 129 sites across the stream network of the McKenzie River sub-basin in Oregon, USA, we did not observe an obvious effect from the Holiday Farm wildfire on the spatial pattern of DOC (Fig. 3). Wildfires are known to impact organic matter availability on burned hillslopes and to shift hydrologic flow paths (Atwood et al., 2023; Jung et al., 2009; Onda et al., 2008; Rey et al., 2023; Stoof et al., 2014; Certini et al., 2011), which theoretically influence the delivery of DOC to streams. Therefore, we expected DOC concentrations to be substantially influenced by the fire as the streamflow moved from upstream to within the burn perimeter and to downstream sites. However, there was not a clearly evident impact of the wildfire on DOC concentrations across the sub-basin. Interestingly, Rüegg et al. (2015) observed a similar lack of response of DOC concentrations to burning in a prairie basin in Kansas, USA. They posited that this was due to limited changes in terrestrial carbon due to the generally low severity of the controlled burns at their study sites. However, this was likely not the case in our basin due to the mixed burn severity of the Holiday Farm fire, with ∼63 % of the area burning at moderate to high burn severity. Moreover, research at high-burn-severity sites across the Holiday Farm fire area found evidence of decreases in the percentage of soil organic carbon in the first 2 cm of soil (56 % change) and in the particulate organic carbon fraction (61 % change, (Katz et al., 2023). The lack of a fire signal in the DOC concentrations may be related to the thick and active organic horizons and extremely high rates of saturated hydraulic conductivity (Ksat) in soils of the Pacific Northwest (Jarecke et al., 2021), which would have enabled the infiltration and vertical percolation of water and DOC through the soil profile despite the burn. A similar phenomenon was observed in NE Victoria in Australia, as the soil saturated hydraulic conductivity (Ksat) remained very high (100–1000 mm h−1) following wildfire (Sheridan et al., 2007). Additionally, landscape characteristics such as groundwater contributions, elevation, land use, slope, aspect, and aridity may also have influenced DOC concentrations across space and muted the effects from the wildfire. A similar effect was observed in the Northwest Territories in Canada – site factors explained ∼50 % of the variation in DOC concentrations, compared to only ∼5 % that was explained by wildfire following repeat sampling across 50 burned and unburned sites (Hutchins et al., 2023). Lastly, the lack of signal could be due to the timing of sampling, since we started sample collection 2 years post-fire. Past research has found that the first few storms post-fire can be critical periods of flushing of ash and wildfire debris from the landscape, often leading to the most substantial increases in DOC concentrations (Writer et al., 2012). However, this was not necessarily observed following the Holiday Farm fire. A previous study in the McKenzie River sub-basin quantified DOC concentrations between 1.5 to 3.0 mg L−1 across five burned tributaries during the first major storm post-fire (Roebuck et al., 2022), which are similar to the concentrations we observed (Fig. 4), suggesting a similar DOC response to the storm. Indeed, results from a recent meta-analysis did not illustrate a relationship between the time between the wildfire, water sampling, and post-fire DOC concentrations (Raoelison et al., 2023).
Despite the lack of a clear effect of the wildfire on the spatial pattern of DOC, we did observe a pattern of low DOC concentrations in the high-elevation headwaters, with DOC generally increasing at lower-elevation sites near the outlet of the basin (Fig. 3). This observed pattern could be attributable to a number of landscape factors. First, elevation is tightly coupled with air temperature in our basin, with strong lapse rates driving warmer temperatures at lower elevations. Warmer temperatures can increase rates of litter decomposition (Salinas et al., 2011), which could have contributed to larger DOC source pools in soils and greater stream DOC concentrations at lower elevations. A similar trend was observed in Germany, where there was strong evidence of increased DOC concentrations with decreasing elevation and increasing air temperatures (Borken et al., 2011). Similarly, DOC was predicted to increase by 0.71 mg L−1 with a 500 m decrease in elevation in the Rocky Mountains of Colorado, USA (Rodríguez-Jeangros et al., 2018). However, the spatial pattern of DOC at our sites could also be due to the correlation of elevation with other landscape factors such as land use. Rodríguez-Jeangros et al. (2018) also noted a greater proportion of agricultural and urban land-use areas at lower elevations, which was correlated with increasing concentrations of DOC. Similarly, in our basin, agricultural land use is increased in the lower one-third of the basin centered around the mainstem and Mohawk tributary (Fig. 1). As agricultural areas are often sources of high organic matter (Chen et al., 2021; Shang et al., 2018), the spatial pattern of land uses may have influenced the spatial DOC concentrations across the McKenzie River sub-basin. Indeed, some of the highest DOC concentrations were observed in the Mohawk tributary at the lower end of the basin (Fig. 3). While spatial patterns of DOC have also been tied to locations within watersheds with a substantial wetland or peatland influence (Ågren et al., 2007; Dawson et al., 2001; Dupas et al., 2021; Piatek et al., 2009; Vidon et al., 2014), this was unlikely to have been a substantial influence in our study, as the McKenzie sub-basin has a minimal wetland influence.
Increases in DOC concentrations from headwaters to outlet may have been influenced by the relative proportion of groundwater inputs along the stream network. The headwaters of the McKenzie sub-basin is primarily classified as High Cascades geology, which is known for its high proportions of deep groundwater inputs (Jefferson et al., 2006). Thus, we were not surprised that our SSN models suggested that groundwater inputs, as represented by the baseflow index (Figs. 5 and 6), were strongly influential on DOC concentrations across the McKenzie sub-basin. Indeed, the variability in DOC due to differences in groundwater inputs may have been greater than the variability created by the Holiday Farm wildfire, muting the impacts of burn severity on DOC. This is consistent with previous work in the McKenzie sub-basin, which found the lowest DOC concentrations to be associated with areas with significant groundwater discharge (Kraus et al., 2010). Others have also noted the importance of typically low-DOC groundwater inputs in controlling the spatial patterns of DOC. For example, for two basins in the Northeast US, groundwater seeps led to headwater streams with some of the lowest DOC concentrations observed across the stream network (Vidon et al., 2014; Zimmer et al., 2013). Additionally, in spatial studies in Sweden and Japan, researchers found significant negative relationships between the percentage of groundwater and DOC concentrations (Egusa et al., 2021; Peralta-Tapia et al., 2015). The importance of groundwater for DOC concentrations after wildfire was also noted following a wildfire in Alberta, Canada, where there was no measurable impact of wildfire on DOC concentrations in fens (Davidson et al., 2019) or boreal lakes (Olefeldt et al., 2013). In both studies, the lack of a wildfire effect on the regulation of DOC concentrations was attributed to the processes of selective adsorption, degradation, and desorption, as water and DOC move slowly downwards through mineral soils to groundwater tables (Kaiser and Kalbitz, 2012). This is consistent with studies from California and Alaska, USA, where the hydrology and the hydrologic connectivity of burned hillslopes and streams were also observed to regulate DOC concentrations (Barton et al., 2023; Larouche et al., 2015). The importance of landscape variables compared to fire impacts highlights the importance of including local landscape characteristics in models to predict post-fire DOC responses or to enable the interpretation of empirical DOC data.
Despite variable DOC inputs from tributaries, the mainstem remained remarkably stable, with low DOC concentrations along its length (Fig. 3). This is consistent with our conceptual understanding of DOC throughout a stream network, where DOC decreases with increasing drainage area due to in-stream removal (Bertuzzo et al., 2017). This pattern of homogenization has also been noted by others (Bhattacharya and Osburn, 2020; Creed et al., 2015), who similarly attributed the lower variability in DOC concentrations at high stream orders to hydrological averaging and a dominance of in-stream processes. Our findings suggest that the impacts from large wildfires, which affect a large portion of the stream network, may also be “averaged out”. While additional research is needed to better quantify how wildfire impacts on DOC concentrations propagate through a stream network, future post-fire studies should carefully consider the scale at which measurements are collected to account for the impact of potentially confounding landscape factors and hydrologic averaging along the stream network. Future work should also consider the extent to which wildfires impact the stream network water quality downstream, as effects on stream network biogeochemistry have been observed (Ball et al., 2021).
4.2 Wildfire decreased the variability of DOC concentrations and led to seasonal variable decreases in concentration
We observed no differences between the mean DOC concentrations across burn-severity groups; however, we observed that the variability in DOC concentrations was lowest in the moderate- and high-severity burned areas (Fig. 4). This change in variability has been relatively unexplored in terms of wildfire effects, as researchers typically focus on the magnitude of change in DOC following fire. However, understanding the variability of post-fire responses is critical when making predictions of post-fire water quality impacts. We believe that the decrease in variability at higher severities is caused by homogenization of the landscape in the wildfire, which removes factors (i.e., soil characteristics and vegetation) that would normally lead to spatial variability in DOC. This is similar to what was found 13 years post-fire in Colorado (Chow et al., 2019). Basins that burned to a high extent (>75 % of the area burned; 50 %–60 % at high severity) exhibited less seasonal variability than those that burned to a moderate extent or were unburned, which was linked to a slow vegetation recovery and bare landscapes. However, the decrease in variability may not be observed immediately post-fire. A 2-year study following wildfire in California found no obvious differences in variability in the first year post-fire, but in the second year, one of the two burned sites exhibited noticeably less variability than the unburned site (Uzun et al., 2020). However, initial flushing of post-fire material may lead to short-term increases in variability in burned areas. In the first year post-fire, DOC variability was increased following wildfire in Colorado and Utah in the US, likely due to debris flows and ash flushed from the burn scar (Crandall et al., 2021; Hohner et al., 2016). Despite the likely homogenization of the post-fire landscape observed in our study during the 2 years post-fire, remaining landscape characteristics remained the dominant control on the magnitude of post-fire DOC concentrations.
Burn severity was never the most important factor controlling DOC, but it was still important for predicting DOC in the wetting season (Fig. 6). In previous work, researchers have shown that DOC is flushed through shallower flow paths during wet periods and is more connected to the landscape than during dry periods (Tiwari et al., 2014). As such, we expected the largest wildfire impacts to occur during the wet season, when the burned hillslopes were most connected to the streams. However, this was not what we observed, with a stronger fire signal occurring during the wetting season. This could be partially due to the wet-season storm we captured, which was a relatively small storm and may not have led to as much hillslope connectivity as we expected (Fig. 2). If we had sampled our sites during a larger storm, we may have found that burn severity was more important. Regardless, the variability in the importance of burn severity across antecedent conditions is a critical finding, as it could help explain the variable nature of wildfire impacts on DOC observed across the literature. A review of post-fire impacts found that of the 27 post-fire effects reviewed, the range of DOC concentration changes had the most variability between the 25th and 75th quantiles (Paul et al., 2022). Given our results, it is likely that this spread in post-fire impacts is partially due to studies sampling across different antecedent conditions. Notably, two of the studies which found that wildfire had no impact on DOC used long-term periodic sampling, which likely included many seasonal periods where burn severity was not important (i.e., the drying and dry seasons); this could have contributed to the conclusion that wildfire had no impact (Mast and Clow, 2008; Wagner et al., 2015).
Our SSN models predicted significant decreases in DOC with increasing burn severity during the wetting period, with minimal and uncertain decreases in the other seasons (Fig. 7). There are two potential explanations for this. First, decreases in evapotranspiration due to vegetation loss post-fire could have led to increased groundwater in burned streams. Drops in ET have been measured following several wildfires in different regions (Ma et al., 2020; Nolan et al., 2014; Poon and Kinoshita, 2018). In recent work, authors have reported that wildfire can lead to increased groundwater contributions to the overall streamflow (MacNeille et al., 2020; Rey et al., 2023); others doing previous research in the same basin found that the lowest DOC concentration occurred in the areas with the greatest groundwater inputs (Kraus et al., 2010). This hypothesis was also proposed by Santos et al. (2019) to explain the decreases in DOC they measured during baseflow periods in California, USA. A second possibility is that we observed lower DOC due to combustion loss of soil organic matter during the wildfire, which decreased the sources of carbon within the basin. This explanation matches well with our seasonal findings, where we primarily observed an effect of burn severity in the wetting period (Fig. 6). Previous work in the region has noted that DOC is often highest during the wetting season due to the flushing of available organics that build up during the dry summer period, with fresh plant residues playing a significant role (Sanderman et al., 2008). Indeed, a previous study within our basin found that during the rising limbs of storms, DOC was sourced from the organic horizon (van Verseveld et al., 2008). So, a decrease in soil organic matter and a loss of vegetation post-fire would likely mute this flushing behavior, resulting in decreased DOC during the wetting period. A post-fire soil study in the McKenzie sub-basin found that total soil organic carbon was decreased along a burn-severity gradient (Katz et al., 2023). Other studies have also proposed decreased soil organic stocks as the cause of the decreased DOC observed post-fire in California and Alaska, USA (Betts and Jones, 2009; Santos et al., 2019).
In our study, we quantified DOC concentrations 2 years post-fire across four distinct seasonal wetness conditions at high spatial resolution across a large sub-basin affected by wildfire in the western Cascade Mountains of Oregon. This enabled us to relate DOC to landscape characteristics, sub-catchment burn conditions, and seasonal wetness conditions. Our findings suggested that increased burn severity may decrease DOC concentrations in streams, most notably during the wetting season. However, this result is complicated by landscape hydrologic pathways and catchment characteristics. For example, we found that DOC responses to wildfire may be substantially dampened in systems dominated by deep sub-surface flow paths of water and groundwater discharge. While our results provided additional context for the wide variability of post-fire DOC responses reported in the literature, a universal understanding of the response of DOC to wildfire remains unresolved. For example, while we observed little shifts in DOC concentrations following wildfire, our study did not address the potential changes in dissolved organic matter character that may occur, which can influence its fate in the environment. Thus, further work exploring how DOC concentrations and dissolved organic matter character change with burn severity across both space and time could further improve our understanding of the mechanisms of delivery of DOC from burned hillslopes to streams (Roebuck et al., 2022, 2023). This type of research is necessary to improve the mechanistic representation of DOC and dissolved organic matter character in models to facilitate important post-fire predictions of the likely range of responses. However, our work also highlights the need to consider a broad range of potentially confounding landscape factors that can influence the hydrobiogeochemical response to wildfire.
Table A1Descriptions of the date, the start and end times of the sampling campaigns, the average rainfall intensity during the sampling period, the antecedent precipitation index (API 1, 7, and 31), and the number of sites sampled. Sampling occurred across the McKenzie sub-basin in Oregon, USA. Precipitation data are based on data from the PRIMET station in the HJ Andrews, OR (44.2119, −122.2559; Daly, 2023).
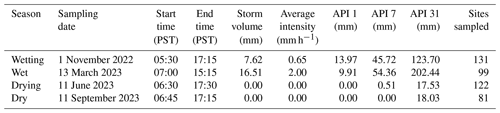
Table A2Comparison of differences in mean DOC concentration between seasons determined using a glmmTMB model and the emmeans package in R (Lenth, 2021; Brooks et al., 2017). The degrees of freedom (df), t-ratio, and p value are reported for each contrast.
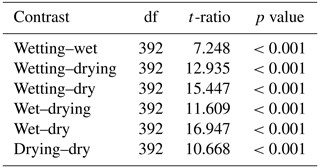
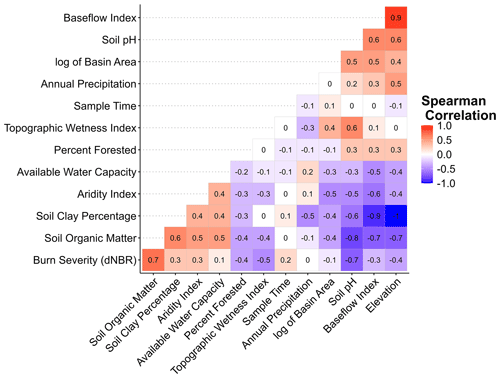
Figure A1Spearman correlation matrix showing the correlation between the explanatory variables used in model selection for the spatial stream network models in the McKenzie sub-basin, OR. For descriptions of the variables, see Table 1.
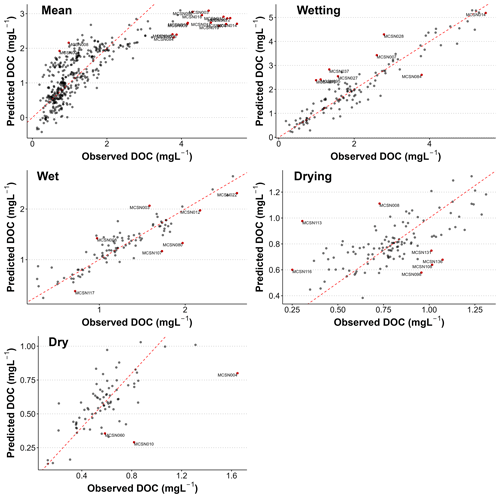
Figure A2Leave-one-out cross-validation results for the mean and seasonal spatial stream network (SSN) models. The dashed red line is the 1:1 line. Potential outliers are labeled with the site number and indicated by red points.
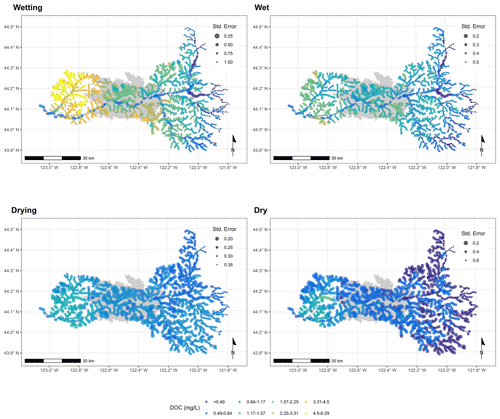
Figure A3Maps showing the predicted DOC concentrations across the McKenzie sub-basin, OR, for the four seasonal wetness conditions. The wildfire perimeter is shown in gray. Predictions were obtained using the final fitted SSN models and prediction points spaced every 1 km along the stream network. The size of a point indicates the prediction error associated with that point.
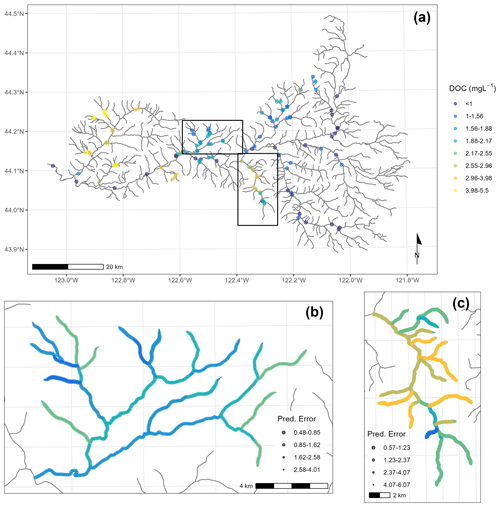
Figure A4DOC across the McKenzie sub-basin, OR, during the wetting season. (a) Observed DOC concentrations; the regions covered by panels (b) and (c) are also indicated. (b) Predicted DOC concentrations across the Gate Creek stream network at 100 m resolution. (c) Predicted DOC concentrations across the Quartz Creek stream network at 100 m resolution. Predictions were made with an SSN model.
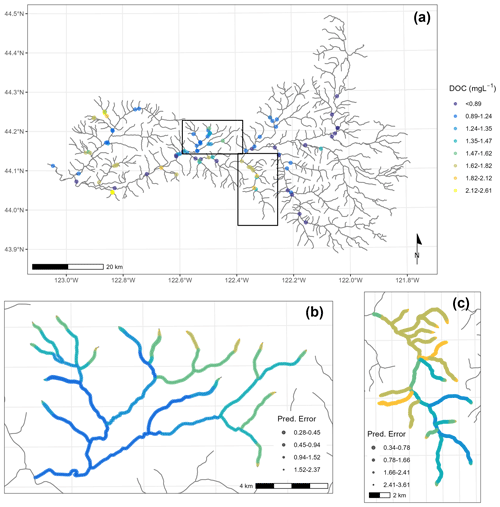
Figure A5DOC across the McKenzie sub-basin, OR, during the wet season. (a) Observed DOC concentrations; the regions covered by panels (b) and (c) are also indicated. (b) Predicted DOC concentrations across the Gate Creek stream network at 100 m resolution. (c) Predicted DOC concentrations across the Quartz Creek stream network at 100 m resolution. Predictions were made with an SSN model.
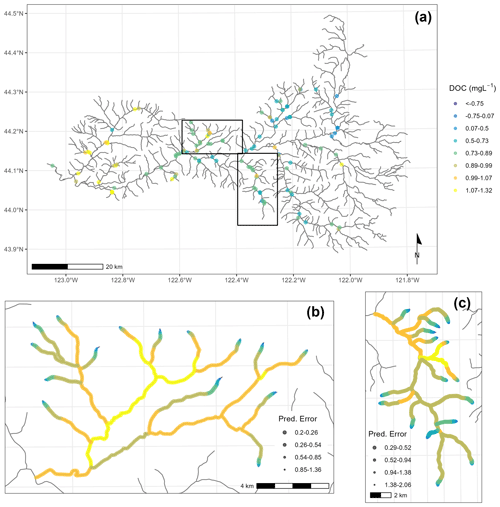
Figure A6DOC across the McKenzie sub-basin, OR, during the drying season. (a) Observed DOC concentrations; the regions covered by panels (b) and (c) are also indicated. (b) Predicted DOC concentrations across the Gate Creek stream network at 100 m resolution. (c) Predicted DOC concentrations across the Quartz Creek stream network at 100 m resolution. Predictions were made with an SSN model.
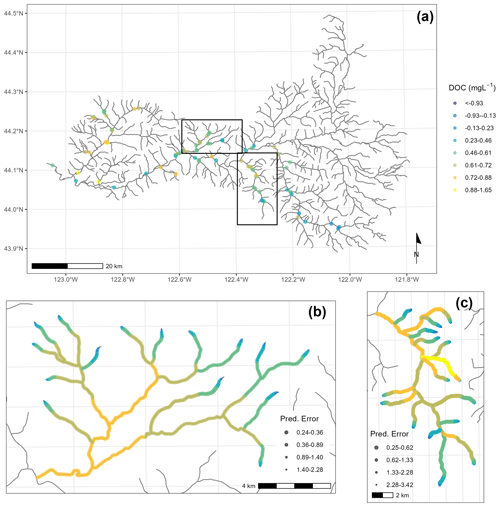
Figure A7DOC across the McKenzie sub-basin, OR, during the dry season. (a) Observed DOC concentrations; the regions covered by panels (b) and (c) are also indicated. (b) Predicted DOC concentrations across the Gate Creek stream network at 100 m resolution. (c) Predicted DOC concentrations across the Quartz Creek stream network at 100 m resolution. Predictions were made with an SSN model.
The data and code that support the findings of this study are openly available at Scholars Archive at Oregon State University, https://doi.org/10.7267/zc77sz60m (Wampler et al., 2024).
KDB and ANMP were responsible for funding acquisition, supervision, and writing – reviewing and editing. KDB provided the resources and conceptualization. KAW performed the investigation, formal analysis, methodology, visualization, and writing – original draft preparation.
The contact author has declared that none of the authors has any competing interests.
Publisher’s note: Copernicus Publications remains neutral with regard to jurisdictional claims made in the text, published maps, institutional affiliations, or any other geographical representation in this paper. While Copernicus Publications makes every effort to include appropriate place names, the final responsibility lies with the authors.
We would like to thank Dustin Gannon for his help with the statistics in this paper. We also want to thank Kelly Andrus, Alessandra Bertucci, Gillian Campbell, Brenna Cody, Ryan Cole, Weylin Crouch, Alex Foote, Bradley Gerdes, Indy Gerhardt, Jansen Ivie, Hyunwoo Kang, Katherine McCool, Spencer McMaster, Lorrayne Miralha, Humyra Murshed, Josiah Nelson, Emily Nussdorfer, Sam Ogle, Cedric Pimont, Will Potter, and Dave Roon for their hard work in the field and lab; this project would not have been possible without their help.
Funding to support the collection of this data set was provided by the US Forest Service (agreement numbers 22-JV-11261952-071 and 23-JV-11261954-057) and the US Department of Energy (DOE), Office of Science, Biological and Environmental Research, Environmental System Science (ESS) program as part of the River Corridors Science Focus Area (54737) at the Pacific Northwest National Laboratory (PNNL). PNNL is operated for the DOE by Battelle Memorial Institute under contract DE-AC05-76RL01830.
This paper was edited by Sebastian Naeher and reviewed by two anonymous referees.
Abatzoglou, J. T., Williams, A. P., Boschetti, L., Zubkova, M., and Kolden, C. A.: Global patterns of interannual climate–fire relationships, Glob. Change Biol., 24, 5164–5175, https://doi.org/10.1111/gcb.14405, 2018.
Abatzoglou, J. T., Rupp, D. E., O'Neill, L. W., and Sadegh, M.: Compound Extremes Drive the Western Oregon Wildfires of September 2020, Geophys. Res. Lett., 48, e2021GL092520, https://doi.org/10.1029/2021GL092520, 2021.
Achat, D. L., Fortin, M., Landmann, G., Ringeval, B., and Augusto, L.: Forest soil carbon is threatened by intensive biomass harvesting, Sci. Rep., 5, 15991, https://doi.org/10.1038/srep15991, 2015.
Ågren, A., Buffam, I., Jansson, M., and Laudon, H.: Importance of seasonality and small streams for the landscape regulation of dissolved organic carbon export, J. Geophys. Res.-Biogeo., 112, G03003, https://doi.org/10.1029/2006JG000381, 2007.
Amiro, B. D., Cantin, A., Flannigan, M. D., and de Groot, W. J.: Future emissions from Canadian boreal forest fires, Can. J. Forest Res., 39, 383–395, https://doi.org/10.1139/X08-154, 2009.
Atwood, A., Hille, M., Clark, M. K., Rengers, F., Ntarlagiannis, D., Townsend, K., and West, A. J.: Importance of subsurface water for hydrological response during storms in a post-wildfire bedrock landscape, Nat. Commun., 14, 3814, https://doi.org/10.1038/s41467-023-39095-z, 2023.
Balfour, V. N., Doerr, S. H., Robichaud, P. R., Balfour, V. N., Doerr, S. H., and Robichaud, P. R.: The temporal evolution of wildfire ash and implications for post-fire infiltration, Int. J. Wildland Fire, 23, 733–745, https://doi.org/10.1071/WF13159, 2014.
Ball, G., Regier, P., González-Pinzón, R., Reale, J., and Van Horn, D.: Wildfires increasingly impact western US fluvial networks, Nat. Commun., 12, 2484, https://doi.org/10.1038/s41467-021-22747-3, 2021.
Barton, R., Richardson, C. M., Pae, E., Montalvo, M. S., Redmond, M., Zimmer, M. A., and Wagner, S.: Hydrology, rather than wildfire burn extent, determines post-fire organic and black carbon export from mountain rivers in central coastal California, Limnology and Oceanography Letters, 9, 70–80, https://doi.org/10.1002/lol2.10360, 2023.
Belloni, A., Chernozhukov, V., and Hansen, C.: Inference on Treatment Effects after Selection among High-Dimensional Controls, The Rev. Econ. Stud., 81, 608–650, https://doi.org/10.1093/restud/rdt044, 2014.
Bertuzzo, E., Helton, A. M., Hall, Robert O., and Battin, T. J.: Scaling of dissolved organic carbon removal in river networks, Adv. Water Resour., 110, 136–146, https://doi.org/10.1016/j.advwatres.2017.10.009, 2017.
Betts, E. F. and Jones, J. B.: Impact of Wildfire on Stream Nutrient Chemistry and Ecosystem Metabolism in Boreal Forest Catchments of Interior Alaska, Arct. Antarct. Alp. Res., 41, 407–417, 2009.
Bhattacharya, R. and Osburn, C. L.: Spatial patterns in dissolved organic matter composition controlled by watershed characteristics in a coastal river network: The Neuse River Basin, USA, Water Res., 169, 115248, https://doi.org/10.1016/j.watres.2019.115248, 2020.
Boer, M., Dios, V., and Bradstock, R.: Unprecedented burn area of Australian mega forest fires, Nat. Clim. Change, 10, 1–2, https://doi.org/10.1038/s41558-020-0716-1, 2020.
Borken, W., Ahrens, B., Schulz, C., and Zimmermann, L.: Site-to-site variability and temporal trends of DOC concentrations and fluxes in temperate forest soils, Glob. Change Biol., 17, 2428–2443, https://doi.org/10.1111/j.1365-2486.2011.02390.x, 2011.
Brando, P., Paolucci, L., Ummenhofer, C., Ordway, E., Hartmann, H., Cattau, M., Rattis, L., Medjibe, V., Coe, M., and Balch, J.: Droughts, Wildfires, and Forest Carbon Cycling: A Pantropical Synthesis, Annu. Rev. Earth Pl. Sc., 47, 555–581, https://doi.org/10.1146/annurev-earth-082517-010235, 2019.
Brooks, M. E., Kristensen, K., Benthem, K. J. van, Magnusson, A., Berg, C. W., Nielsen, A., Skaug, H. J., Mächler, M., and Bolker, B. M.: glmmTMB Balances Speed and Flexibility Among Packages for Zero-inflated Generalized Linear Mixed Modeling, R J., 9, 378–400, 2017.
Burton, C. A., Hoefen, T. M., Plumlee, G. S., Baumberger, K. L., Backlin, A. R., Gallegos, E., and Fisher, R. N.: Trace Elements in Stormflow, Ash, and Burned Soil following the 2009 Station Fire in Southern California, PLOS ONE, 11, e0153372, https://doi.org/10.1371/journal.pone.0153372, 2016.
Butturini, A. and Sabater, F.: Seasonal variability of dissolved organic carbon in a Mediterranean stream, Biogeochemistry, 51, 303–321, https://doi.org/10.1023/A:1006420229411, 2000.
Caldwell, P. V., Elliott, K. J., Liu, N., Vose, J. M., Zietlow, D. R., and Knoepp, J. D.: Watershed-scale vegetation, water quantity, and water quality responses to wildfire in the southern Appalachian mountain region, United States, Hydrol. Process., 34, 5188–5209, https://doi.org/10.1002/hyp.13922, 2020.
Carpenter, K. D., Kraus, T. E. C., Hansen, A. M., Downing, B. D., Goldman, J. H., Haynes, J., Donahue, D., and Morgenstern, K.: Sources and characteristics of dissolved organic carbon in the McKenzie River, Oregon, related to the formation of disinfection by-products in treated drinking water, Reston, VA, https://doi.org/10.3133/sir20225010, 2022.
Casas-Ruiz, J. P., Spencer, R. G. M., Guillemette, F., von Schiller, D., Obrador, B., Podgorski, D. C., Kellerman, A. M., Hartmann, J., Gómez-Gener, L., Sabater, S., and Marcé, R.: Delineating the Continuum of Dissolved Organic Matter in Temperate River Networks, Global Biogeochem. Cy., 34, e2019GB006495, https://doi.org/10.1029/2019GB006495, 2020.
Cavaiani, J., Regier, P., Roebuck, A., Barnes, M. E., Garayburu-Caruso, V. A., Gillespie, X., McKever, S., Renteria, L., and Allison N. Myers-Pigg: Catchment characteristics modulate the influence of wildfires on nitrate and dissolved organic carbon across space and time: A meta-analysis, ESS Open Archive [preprint], https://doi.org/10.22541/essoar.171052482.22663736/v1, 2024.
Certini, G., Nocentini, C., Knicker, H., Arfaioli, P., and Rumpel, C.: Wildfire effects on soil organic matter quantity and quality in two fire-prone Mediterranean pine forests, Geoderma, 167–168, 148–155, https://doi.org/10.1016/j.geoderma.2011.09.005, 2011.
Chambers, J. Q., Fisher, J. I., Zeng, H., Chapman, E. L., Baker, D. B., and Hurtt, G. C.: Hurricane Katrina's Carbon Footprint on U.S. Gulf Coast Forests, Science, 318, 1107–1107, https://doi.org/10.1126/science.1148913, 2007.
Chen, S., Du, Y., Das, P., Lamore, A. F., Dimova, N. T., Elliott, M., Broadbent, E. N., Roebuck., J. A., Jaffé, R., and Lu, Y.: Agricultural land use changes stream dissolved organic matter via altering soil inputs to streams, Sci. Total Environ., 796, 148968, https://doi.org/10.1016/j.scitotenv.2021.148968, 2021.
Chow, A. T., Tsai, K.-P., Fegel, T. S., Pierson, D. N., and Rhoades, C. C.: Lasting Effects of Wildfire on Disinfection By-Product Formation in Forest Catchments, J. Environ. Qual., 48, 1826–1834, https://doi.org/10.2134/jeq2019.04.0172, 2019.
Cicco, L. A. D., Lorenz, D., Hirsch, R. M., and Watkins, W.: dataRetrieval: R packages for discovering and retrieving water data available from U.S. federal hydrologic web services, CRAN [code], https://doi.org/10.5066/P9X4L3GE, 2018.
Cole, J. J., Prairie, Y. T., Caraco, N. F., McDowell, W. H., Tranvik, L. J., Striegl, R. G., Duarte, C. M., Kortelainen, P., Downing, J. A., Middelburg, J. J., and Melack, J.: Plumbing the Global Carbon Cycle: Integrating Inland Waters into the Terrestrial Carbon Budget, Ecosystems, 10, 172–185, https://doi.org/10.1007/s10021-006-9013-8, 2007.
Crandall, T., Jones, E., Greenhalgh, M., Frei, R. J., Griffin, N., Severe, E., Maxwell, J., Patch, L., Clair, S. I. S., Bratsman, S., Merritt, M., Norris, A. J., Carling, G. T., Hansen, N., Clair, S. B. S., and Abbott, B. W.: Megafire affects stream sediment flux and dissolved organic matter reactivity, but land use dominates nutrient dynamics in semiarid watersheds, PLOS ONE, 16, e0257733, https://doi.org/10.1371/journal.pone.0257733, 2021.
Creed, I. F., McKnight, D. M., Pellerin, B. A., Green, M. B., Bergamaschi, B. A., Aiken, G. R., Burns, D. A., Findlay, S. E. G., Shanley, J. B., Striegl, R. G., Aulenbach, B. T., Clow, D. W., Laudon, H., McGlynn, B. L., McGuire, K. J., Smith, R. A., and Stackpoole, S. M.: The river as a chemostat: fresh perspectives on dissolved organic matter flowing down the river continuum, Can. J. Fish. Aquat. Sci., 72, 1272–1285, https://doi.org/10.1139/cjfas-2014-0400, 2015.
Daly, C.: Meteorological data from Primet (NOAHIV) at the Andrews Forest, HJ Andrews Data Portal [dataset], https://andrewsforest.oregonstate.edu/sites/default/files/lter/data/weather/portal/PRIMET/data/primet_or10_15min_2023.html (last access: 28 June 2024), 2023.
Davidson, S. J., Elmes, M. C., Rogers, H., van Beest, C., Petrone, R., Price, J. S., and Strack, M.: Hydrogeologic setting overrides any influence of wildfire on pore water dissolved organic carbon concentration and quality at a boreal fen, Ecohydrology, 12, e2141, https://doi.org/10.1002/eco.2141, 2019.
Dawson, J. J. C., Bakewell, C., and Billett, M. F.: Is in-stream processing an important control on spatial changes in carbon fluxes in headwater catchments?, Sci. Total Environ., 265, 153–167, https://doi.org/10.1016/S0048-9697(00)00656-2, 2001.
Dawson, J. J. C., Soulsby, C., Tetzlaff, D., Hrachowitz, M., Dunn, S. M., and Malcolm, I. A.: Influence of hydrology and seasonality on DOC exports from three contrasting upland catchments, Biogeochemistry, 90, 93–113, https://doi.org/10.1007/s10533-008-9234-3, 2008.
de la Barrera, F., Barraza, F., Favier, P., Ruiz, V., and Quense, J.: Megafires in Chile 2017: Monitoring multiscale environmental impacts of burned ecosystems, Sci. Total Environ., 637–638, 1526–1536, https://doi.org/10.1016/j.scitotenv.2018.05.119, 2018.
Dewitz, J.: National Land Cover Database (NLCD) 2019 Products, ScienceBase, https://doi.org/10.5066/P9KZCM54, 2021.
Dodd, W., Scott, P., Howard, C., Scott, C., Rose, C., Cunsolo, A., and Orbinski, J.: Lived experience of a record wildfire season in the Northwest Territories, Canada, Can. J. Public Health, 109, 327–337, https://doi.org/10.17269/s41997-018-0070-5, 2018.
Doerr, S. H., Woods, S. W., Martin, D. A., and Casimiro, M.: “Natural background” soil water repellency in conifer forests of the north-western USA: Its prediction and relationship to wildfire occurrence, J. Hydrol., 371, 12–21, https://doi.org/10.1016/j.jhydrol.2009.03.011, 2009.
Drake, T. W., Raymond, P. A., and Spencer, R. G. M.: Terrestrial carbon inputs to inland waters: A current synthesis of estimates and uncertainty, Limnology and Oceanography Letters, 3, 132–142, https://doi.org/10.1002/lol2.10055, 2018.
Duane, A., Castellnou, M., and Brotons, L.: Towards a comprehensive look at global drivers of novel extreme wildfire events, Clim. Change, 165, 43, https://doi.org/10.1007/s10584-021-03066-4, 2021.
Dupas, R., Causse, J., Jaffrezic, A., Aquilina, L., and Durand, P.: Flowpath controls on high-spatial-resolution water-chemistry profiles in headwater streams, Hydrol. Process., 35, e14247, https://doi.org/10.1002/hyp.14247, 2021.
Ebel, B. A. and Moody, J. A.: Synthesis of soil-hydraulic properties and infiltration timescales in wildfire-affected soils, Hydrol. Process., 31, 324–340, https://doi.org/10.1002/hyp.10998, 2017.
Egusa, T., Kumagai, T., Oda, T., and Ohte, N.: Effects of bedrock groundwater discharge on spatial variability of dissolved carbon, nitrogen, and phosphorous concentrations in stream water within a forest headwater catchment, Hydrol. Process., 35, e13993, https://doi.org/10.1002/hyp.13993, 2021.
Emelko, M. B., Silins, U., Bladon, K. D., and Stone, M.: Implications of land disturbance on drinking water treatability in a changing climate: Demonstrating the need for “source water supply and protection” strategies, Water Res., 45, 461–472, https://doi.org/10.1016/j.watres.2010.08.051, 2011.
Fan, Y. and Li, R.: Variable Selection in Linear Mixed Effects Models, Ann. Stat., 40, 2043–2068, https://doi.org/10.1214/12-AOS1028, 2012.
Fellman, J. B., D'Amore, D. V., and Hood, E.: An evaluation of freezing as a preservation technique for analyzing dissolved organic C, N and P in surface water samples, Sci. Total Environ., 392, 305–312, https://doi.org/10.1016/j.scitotenv.2007.11.027, 2008.
Forbes, M. S., Raison, R. J., and Skjemstad, J. O.: Formation, transformation and transport of black carbon (charcoal) in terrestrial and aquatic ecosystems, Sci. Total Environ., 370, 190–206, https://doi.org/10.1016/j.scitotenv.2006.06.007, 2006.
Futter, M. N., Butterfield, D., Cosby, B. J., Dillon, P. J., Wade, A. J., and Whitehead, P. G.: Modeling the mechanisms that control in-stream dissolved organic carbon dynamics in upland and forested catchments, Water Resour. Res., 43, W02424, https://doi.org/10.1029/2006WR004960, 2007.
Hampton, T. B., Lin, S., and Basu, N. B.: Forest fire effects on stream water quality at continental scales: a meta-analysis, Environ. Res. Lett., 17, 064003, https://doi.org/10.1088/1748-9326/ac6a6c, 2022.
Harris, H. E., Baxter, C. V., and Davis, J. M.: Debris flows amplify effects of wildfire on magnitude and composition of tributary subsidies to mainstem habitats, Freshw. Sci., 34, 1457–1467, https://doi.org/10.1086/684015, 2015.
Hohner, A. K., Cawley, K., Oropeza, J., Summers, R. S., and Rosario-Ortiz, F. L.: Drinking water treatment response following a Colorado wildfire, Water Res., 105, 187–198, https://doi.org/10.1016/j.watres.2016.08.034, 2016.
Hohner, A. K., Terry, L. G., Townsend, E. B., Summers, R. S., and Rosario-Ortiz, F. L.: Water treatment process evaluation of wildfire-affected sediment leachates, Environ. Sci.-Wat. Res., 3, 352–365, https://doi.org/10.1039/C6EW00247A, 2017.
Hornberger, G. M., Bencala, K. E., and McKnight, D. M.: Hydrological controls on dissolved organic carbon during snowmelt in the Snake River near Montezuma, Colorado, Biogeochemistry, 25, 147–165, https://doi.org/10.1007/BF00024390, 1994.
Humbert, G., Jaffrezic, A., Fovet, O., Gruau, G., and Durand, P.: Dry-season length and runoff control annual variability in stream DOC dynamics in a small, shallow groundwater-dominated agricultural watershed, Water Resour. Res., 51, 7860–7877, https://doi.org/10.1002/2015WR017336, 2015.
Hutchins, R., Tank, S., Olefeldt, D., Quinton, W., Spence, C., Dion, N., and Mengistu, S.: Influence of Wildfire on Downstream Transport of Dissolved Carbon, Nutrients, and Mercury in the Permafrost Zone of Boreal Western Canada, J. Geophys. Res.-Biogeo., 128, e2023JG007602, https://doi.org/10.1029/2023JG007602, 2023.
Jarecke, K. M., Bladon, K. D., and Wondzell, S. M.: The Influence of Local and Nonlocal Factors on Soil Water Content in a Steep Forested Catchment, Water Resour. Res., 57, e2020WR028343, https://doi.org/10.1029/2020WR028343, 2021.
Jefferson, A., Grant, G., and Rose, T.: Influence of volcanic history on groundwater patterns on the west slope of the Oregon High Cascades, Water Resour. Res., 42, W12411, https://doi.org/10.1029/2005WR004812, 2006.
Johnson, D., Murphy, J. D., Walker, R. F., Glass, D. W., and Miller, W. W.: Wildfire effects on forest carbon and nutrient budgets, Ecol. Eng., 31, 183–192, https://doi.org/10.1016/j.ecoleng.2007.03.003, 2007.
Jung, H. Y., Hogue, T. S., Rademacher, L. K., and Meixner, T.: Impact of wildfire on source water contributions in Devil Creek, CA: evidence from end-member mixing analysis, Hydrol. Process., 23, 183–200, https://doi.org/10.1002/hyp.7132, 2009.
Kaiser, K. and Kalbitz, K.: Cycling downwards – dissolved organic matter in soils, Soil Biol. Biochem., 52, 29–32, https://doi.org/10.1016/j.soilbio.2012.04.002, 2012.
Kalbitz, K., Solinger, S., Park, J.-H., Michalzik, B., and Matzner, E.: Controls on the Dynamics of Dissolved Organic Matter in Soils: A Review, Soil Sci., 165, 277–304, 2000.
Katz, S. M., Gavin, D., and Silva, L. C. R.: Mapping Soil Organic Carbon in Wildfire-Affected Areas of the McKenzie River Basin, Oregon, USA, ESS Open Archive [preprint], https://doi.org/10.22541/essoar.168695523.32925211/v1, 2023.
Kerins, D. and Li, L.: High Dissolved Carbon Concentration in Arid Rocky Mountain Streams, Environ. Sci. Technol., 57, 4656–4667, https://doi.org/10.1021/acs.est.2c06675, 2023.
Koehler, A.-K., Murphy, K., Kiely, G., and Sottocornola, M.: Seasonal variation of DOC concentration and annual loss of DOC from an Atlantic blanket bog in South Western Ireland, Biogeochemistry, 95, 231–242, https://doi.org/10.1007/s10533-009-9333-9, 2009.
Kottek, M., Grieser, J., Beck, C., Rudolf, B., and Rubel, F.: World Map of the Köppen-Geiger climate classification updated, Meteorol. Z., 15, 259–263, https://doi.org/10.1127/0941-2948/2006/0130, 2006.
Kraus, T. E. C., Anderson, C. A., Morgenstern, K., Downing, B. D., Pellerin, B. A., and Bergamaschi, B. A.: Determining sources of dissolved organic carbon and disinfection byproduct precursors to the McKenzie River, Oregon, J. Environ. Qual., 39, 2100–2112, https://doi.org/10.2134/jeq2010.0030, 2010.
Kurz, W. A., Dymond, C. C., Stinson, G., Rampley, G. J., Neilson, E. T., Carroll, A. L., Ebata, T., and Safranyik, L.: Mountain pine beetle and forest carbon feedback to climate change, Nature, 452, 987–990, https://doi.org/10.1038/nature06777, 2008.
Kusaka, S., Nakane, K., and Mitsudera, M.: Effect of fire on water and major nutrient budgets in forest ecosystems: I. Water Balance, Japanese Journal of Ecology, 33, 323–332, https://doi.org/10.18960/seitai.33.3_323, 1983.
Lagouvardos, K., Kotroni, V., Giannaros, T. M., and Dafis, S.: Meteorological Conditions Conducive to the Rapid Spread of the Deadly Wildfire in Eastern Attica, Greece, B. Am. Meteor. Soc., 100, 2137–2145, https://doi.org/10.1175/BAMS-D-18-0231.1, 2019.
Lambert, T., Pierson-Wickmann, A.-C., Gruau, G., Jaffrezic, A., Petitjean, P., Thibault, J.-N., and Jeanneau, L.: Hydrologically driven seasonal changes in the sources and production mechanisms of dissolved organic carbon in a small lowland catchment, Water Resour. Res., 49, 5792–5803, https://doi.org/10.1002/wrcr.20466, 2013.
Larouche, J. R., Abbott, B. W., Bowden, W. B., and Jones, J. B.: The role of watershed characteristics, permafrost thaw, and wildfire on dissolved organic carbon biodegradability and water chemistry in Arctic headwater streams, Biogeosciences, 12, 4221–4233, https://doi.org/10.5194/bg-12-4221-2015, 2015.
Larson-Nash, S. S., Robichaud, P. R., Pierson, F. B., Moffet, C. A., Williams, C. J., Spaeth, K. E., Brown, R. E., and Lewis, S. A.: Recovery of small-scale infiltration and erosion after wildfires, J. Hydrol. Hydromech., 66, 261–270, https://doi.org/10.1515/johh-2017-0056, 2018.
Leenheer, J. A., Malcolm, R. L., McKinley, P. W., and Eccles, L. A.: Occurrence of dissolved organic carbon in selected ground-water samples in the United States, J. Res. US Geol. Surv., 2, 361–369, 1974.
Lenth, R. V.: emmeans: Estimated Marginal Means, aka Least-Squares Means, CRAN [code], https://doi.org/10.32614/CRAN.package.emmeans, 2021.
Ma, Q., Bales, R. C., Rungee, J., Conklin, M. H., Collins, B. M., and Goulden, M. L.: Wildfire controls on evapotranspiration in California's Sierra Nevada, J. Hydrol., 590, 125364, https://doi.org/10.1016/j.jhydrol.2020.125364, 2020.
MacNeille, R. B., Lohse, K. A., Godsey, S. E., Perdrial, J. N., and Baxter, C. V.: Influence of Drying and Wildfire on Longitudinal Chemistry Patterns and Processes of Intermittent Streams, Frontiers in Water, 2, 563841, https://doi.org/10.3389/frwa.2020.563841, 2020.
Masiello, C. A.: New directions in black carbon organic geochemistry, Mar. Chem., 92, 201–213, https://doi.org/10.1016/j.marchem.2004.06.043, 2004.
Mast, M. A. and Clow, D. W.: Effects of 2003 wildfires on stream chemistry in Glacier National Park, Montana, Hydrol. Process., 22, 5013–5023, https://doi.org/10.1002/hyp.7121, 2008.
Miesel, J., Reiner, A., Ewell, C., Maestrini, B., and Dickinson, M.: Quantifying Changes in Total and Pyrogenic Carbon Stocks Across Fire Severity Gradients Using Active Wildfire Incidents, Front. Earth Sci., 6, 41, https://doi.org/10.3389/feart.2018.00041, 2018.
MTBS Project: MTBS Data Access: Fire Level Geospatial Data, http://mtbs.gov/direct-download (last access: 18 January 2023), 2021.
Mulholland, P. J.: Dissolved Organic Matter Concentration and Flux in Streams, J. N. Am. Benthol. Soc., 16, 131–141, https://doi.org/10.2307/1468246, 1997.
Nelson, P. N., Baldock, J. A., and Oades, J. M.: Concentration and composition of dissolved organic carbon in streams in relation to catchment soil properties, Biogeochemistry, 19, 27–50, https://doi.org/10.1007/BF00000573, 1992.
Nolan, R., Lane, P., Benyon, R., Bradstock, R., and Mitchell, P.: Changes in evapotranspiration following wildfire in resprouting eucalypt forests, Ecohydrology, 7, 1363–1377, https://doi.org/10.1002/eco.1463, 2014.
Olefeldt, D., Devito, K. J., and Turetsky, M. R.: Sources and fate of terrestrial dissolved organic carbon in lakes of a Boreal Plains region recently affected by wildfire, Biogeosciences, 10, 6247–6265, https://doi.org/10.5194/bg-10-6247-2013, 2013.
Oliver, A. A., Reuter, J. E., Heyvaert, A. C., and Dahlgren, R. A.: Water quality response to the Angora Fire, Lake Tahoe, California, Biogeochemistry, 111, 361–376, 2012.
Onda, Y., Dietrich, W. E., and Booker, F.: Evolution of overland flow after a severe forest fire, Point Reyes, California, Catena, 72, 13–20, https://doi.org/10.1016/j.catena.2007.02.003, 2008.
Parham, L. M., Prokushkin, A. S., Pokrovsky, Oleg. S., Titov, S. V., Grekova, E., Shirokova, L. S., and McDowell, W. H.: Permafrost and fire as regulators of stream chemistry in basins of the Central Siberian Plateau, Biogeochemistry, 116, 55–68, https://doi.org/10.1007/s10533-013-9922-5, 2013.
Paul, M. J., LeDuc, S. D., Lassiter, M. G., Moorhead, L. C., Noyes, P. D., and Leibowitz, S. G.: Wildfire Induces Changes in Receiving Waters: A Review With Considerations for Water Quality Management, Water Resour. Res., 58, e2021WR030699, https://doi.org/10.1029/2021WR030699, 2022.
Pausas, J. G. and Keeley, J. E.: Wildfires and global change, Front. Ecol. Environ., 19, 387–395, https://doi.org/10.1002/fee.2359, 2021.
Peralta-Tapia, A., Sponseller, R. A., Ågren, A., Tetzlaff, D., Soulsby, C., and Laudon, H.: Scale-dependent groundwater contributions influence patterns of winter baseflow stream chemistry in boreal catchments, J. Geophys. Res.-Biogeo., 120, 847–858, https://doi.org/10.1002/2014JG002878, 2015.
Peterson, E. and Ver Hoef, J. M.: STARS: An ArcGIS Toolset Used to Calculate the Spatial Information Needed to Fit Spatial Statistical Models to Stream Network Data, J. Stat. Softw., 56, 1–17, https://doi.org/10.18637/jss.v056.i02, 2014.
Piatek, K. B., Christopher, S. F., and Mitchell, M. J.: Spatial and temporal dynamics of stream chemistry in a forested watershed, Hydrol. Earth Syst. Sci., 13, 423–439, https://doi.org/10.5194/hess-13-423-2009, 2009.
Poon, P. K. and Kinoshita, A. M.: Spatial and temporal evapotranspiration trends after wildfire in semi-arid landscapes, J. Hydrol., 559, 71–83, https://doi.org/10.1016/j.jhydrol.2018.02.023, 2018.
Preston, C. M. and Schmidt, M. W. I.: Black (pyrogenic) carbon: a synthesis of current knowledge and uncertainties with special consideration of boreal regions, Biogeosciences, 3, 397–420, https://doi.org/10.5194/bg-3-397-2006, 2006.
PRISM Climate Group: 30-Year Normals, Prism Climate Group [dataset], https://www.prism.oregonstate.edu/normals/ (last access: 28 June 2024), 2012.
R Core Team: R: A language and environment for statistical computing, R Foundation for Statistical Computing [code], https://www.R-project.org/ (last access: 28 June 2024), 2020.
Raoelison, O. D., Valenca, R., Lee, A., Karim, S., Webster, J. P., Poulin, B. A., and Mohanty, S. K.: Wildfire impacts on surface water quality parameters: Cause of data variability and reporting needs, Environ. Pollut., 317, 120713, https://doi.org/10.1016/j.envpol.2022.120713, 2023.
Raymond, P. A. and Saiers, J. E.: Event controlled DOC export from forested watersheds, Biogeochemistry, 100, 197–209, https://doi.org/10.1007/s10533-010-9416-7, 2010.
Revchuk, A. D. and Suffet, I. H.: Effect of Wildfires on Physicochemical Changes of Watershed Dissolved Organic Matter, Water Environ. Res., 86, 372–381, https://doi.org/10.2175/106143013X13736496909671, 2014.
Rey, D. M., Briggs, M. A., Walvoord, M. A., and Ebel, B. A.: Wildfire-induced shifts in groundwater discharge to streams identified with paired air and stream water temperature analyses, J. Hydrol., 619, 129272, https://doi.org/10.1016/j.jhydrol.2023.129272, 2023.
Rhoades, C. C., Chow, A. T., Covino, T. P., Fegel, T. S., Pierson, D. N., and Rhea, A. E.: The Legacy of a Severe Wildfire on Stream Nitrogen and Carbon in Headwater Catchments, Ecosystems, 22, 643–657, https://doi.org/10.1007/s10021-018-0293-6, 2019.
Rodríguez-Cardona, B. M., Coble, A. A., Wymore, A. S., Kolosov, R., Podgorski, D. C., Zito, P., Spencer, R. G. M., Prokushkin, A. S., and McDowell, W. H.: Wildfires lead to decreased carbon and increased nitrogen concentrations in upland arctic streams, Sci. Rep., 10, 8722, https://doi.org/10.1038/s41598-020-65520-0, 2020.
Rodríguez-Jeangros, N., Hering, A. S., and McCray, J. E.: Analysis of Anthropogenic, Climatological, and Morphological Influences on Dissolved Organic Matter in Rocky Mountain Streams, Water, 10, 534, https://doi.org/10.3390/w10040534, 2018.
Roebuck Jr., J. A., Bladon, K. D., Donahue, D., Graham, E. B., Grieger, S., Morgenstern, K., Norwood, M. J., Wampler, K. A., Erkert, L., Renteria, L., Danczak, R., Fricke, S., and Myers-Pigg, A. N.: Spatiotemporal Controls on the Delivery of Dissolved Organic Matter to Streams Following a Wildfire, Geophys. Res. Lett., 49, e2022GL099535, https://doi.org/10.1029/2022GL099535, 2022.
Roebuck Jr., J. A., Prestegaard Jr., K., Gaviria, C., Myers-Pigg, A., and Ziegler, S. E.: Hydrobiogeochemical Controls on the Delivery of Dissolved Organic Matter to Boreal Headwater Streams, Water Resour. Res., 59, e2022WR033358, https://doi.org/10.1029/2022WR033358, 2023.
Rüegg, J., Eichmiller, J. J., Mladenov, N., and Dodds, W. K.: Dissolved organic carbon concentration and flux in a grassland stream: spatial and temporal patterns and processes from long-term data, Biogeochemistry, 125, 393–408, https://doi.org/10.1007/s10533-015-0134-z, 2015.
Rust, A. J., Randell, J., Todd, A. S., and Hogue, T. S.: Wildfire impacts on water quality, macroinvertebrate, and trout populations in the Upper Rio Grande, Forest Ecol. Manage., 453, 117636, https://doi.org/10.1016/j.foreco.2019.117636, 2019.
Salinas, N., Malhi, Y., Meir, P., Silman, M., Roman Cuesta, R., Huaman, J., Salinas, D., Huaman, V., Gibaja, A., Mamani, M., and Farfan, F.: The sensitivity of tropical leaf litter decomposition to temperature: results from a large-scale leaf translocation experiment along an elevation gradient in Peruvian forests, New Phytol., 189, 967–977, https://doi.org/10.1111/j.1469-8137.2010.03521.x, 2011.
Sanderman, J., Baldock, J. A., and Amundson, R.: Dissolved organic carbon chemistry and dynamics in contrasting forest and grassland soils, Biogeochemistry, 89, 181–198, https://doi.org/10.1007/s10533-008-9211-x, 2008.
Santín, C., Doerr, S. H., Preston, C. M., and González-Rodríguez, G.: Pyrogenic organic matter production from wildfires: a missing sink in the global carbon cycle, Glob. Change Biol., 21, 1621–1633, https://doi.org/10.1111/gcb.12800, 2015.
Santos, F., Wymore, A. S., Jackson, B. K., Sullivan, S. M. P., McDowell, W. H., and Berhe, A. A.: Fire severity, time since fire, and site-level characteristics influence streamwater chemistry at baseflow conditions in catchments of the Sierra Nevada, California, USA, Fire Ecol., 15, 3, https://doi.org/10.1186/s42408-018-0022-8, 2019.
Shang, P., Lu, Y., Du, Y., Jaffé, R., Findlay, R. H., and Wynn, A.: Climatic and watershed controls of dissolved organic matter variation in streams across a gradient of agricultural land use, Sci. Total Environ., 612, 1442–1453, https://doi.org/10.1016/j.scitotenv.2017.08.322, 2018.
Sheridan, G. J., Lane, P. N. J., and Noske, P. J.: Quantification of hillslope runoff and erosion processes before and after wildfire in a wet Eucalyptus forest, J. Hydrol., 343, 12–28, https://doi.org/10.1016/j.jhydrol.2007.06.005, 2007.
Snyder, K., Sullivan, T., Raymond, R., Moore, D., and Gilbert, E.: North Santiam River Watershed Assessment, E&S Environmental Chemistry, Inc., 2002.
Stoof, C. R., Vervoort, R. W., Iwema, J., van den Elsen, E., Ferreira, A. J. D., and Ritsema, C. J.: Hydrological response of a small catchment burned by experimental fire, Hydrol. Earth Syst. Sci., 16, 267–285, https://doi.org/10.5194/hess-16-267-2012, 2012.
Stoof, C. R., Slingerland, E. C., Mol, W., van den Berg, J., Vermeulen, P. J., Ferreira, A. J. D., Ritsema, C. J., Parlange, J.-Y., and Steenhuis, T. S.: Preferential flow as a potential mechanism for fire-induced increase in streamflow, Water Resour. Res., 50, 1840–1845, https://doi.org/10.1002/2013WR014397, 2014.
Tague, C., Grant, G., Farrell, M., Choate, J., and Jefferson, A.: Deep groundwater mediates streamflow response to climate warming in the Oregon Cascades, Clim. Change, 86, 189–210, https://doi.org/10.1007/s10584-007-9294-8, 2008.
Tiwari, T., Laudon, H., Beven, K., and Ågren, A. M.: Downstream changes in DOC: Inferring contributions in the face of model uncertainties, Water Resour. Res., 50, 514–525, https://doi.org/10.1002/2013WR014275, 2014.
Trabucco, A. and Zomer, R.: Global Aridity Index and Potential Evapotranspiration (ET0) Climate Database v2, figshare [dataset], https://doi.org/10.6084/m9.figshare.7504448.v3, 2019.
Tupas, L. M., Popp, B. N., and Karl, D. M.: Dissolved organic carbon in oligotrophic waters: experiments on sample preservation, storage and analysis, Mar. Chem., 45, 207–216, https://doi.org/10.1016/0304-4203(94)90004-3, 1994.
Turco, M., Jerez, S., Augusto, S., Tarín-Carrasco, P., Ratola, N., Jiménez-Guerrero, P., and Trigo, R. M.: Climate drivers of the 2017 devastating fires in Portugal, Sci. Rep., 9, 13886, https://doi.org/10.1038/s41598-019-50281-2, 2019.
U.S. Geological Survey: Shuttle Radar Topography Mission (SRTM) 1 Arc-Second Global, U.S. Geological Survey [data set], https://doi.org/10.5066/F7PR7TFT, 2000.
U.S. Geological Survey: Base-flow index grid for the conterminous United States, U.S. Geological Survey [data set], https://doi.org/10.5066/P9MCTH3J, 2003.
U.S. Geological Survey: The StreamStats program, https://streamstats.usgs.gov/ss/ (last access: 23 June 2023), 2019.
U.S. Geological Survey: National Hydrography Dataset (NHD) – USGS National Map Downloadable Data Collection, U.S. Geological Survey [data set], https://www.usgs.gov/national-hydrography (last access: 28 June 2024), 2020.
U.S. Geological Survey: Water Data for the Nation, https://waterdata.usgs.gov/nwis, last access: 12 January 2024.
USDA NRCS: Oregon SSURGO STATSGO Soils Compilation, Oregon Spatial Library [data set], https://spatialdata.oregonexplorer.info/geoportal/details;id=668a7a8824e5456797bec858cc14ca74, 2016.
Uzun, H., Dahlgren, R. A., Olivares, C., Erdem, C. U., Karanfil, T., and Chow, A. T.: Two years of post-wildfire impacts on dissolved organic matter, nitrogen, and precursors of disinfection by-products in California stream waters, Water Res., 181, 115891, https://doi.org/10.1016/j.watres.2020.115891, 2020.
van den Berg, L. J. L., Shotbolt, L., and Ashmore, M. R.: Dissolved organic carbon (DOC) concentrations in UK soils and the influence of soil, vegetation type and seasonality, Sci. Total Environ., 427–428, 269–276, https://doi.org/10.1016/j.scitotenv.2012.03.069, 2012.
Ver Hoef, J. M. and Peterson, E. E.: A Moving Average Approach for Spatial Statistical Models of Stream Networks, J. Am. Stat. Assoc., 105, 6–18, https://doi.org/10.1198/jasa.2009.ap08248, 2010.
Ver Hoef, J. M., Peterson, E., Clifford, D., and Shah, R.: SSN: An R Package for Spatial Statistical Modeling on Stream Networks, J. Stat. Softw., 56, 1–45, https://doi.org/10.18637/jss.v056.i03, 2014.
van Verseveld, W. J., McDonnell, J. J., and Lajtha, K.: A mechanistic assessment of nutrient flushing at the catchment scale, J. Hydrol., 358, 268–287, https://doi.org/10.1016/j.jhydrol.2008.06.009, 2008.
Vidon, P., Carleton, W., and Mitchell, M. J.: Spatial and temporal variability in stream dissolved organic carbon quantity and quality in an Adirondack forested catchment, Appl. Geochem., 46, 10–18, https://doi.org/10.1016/j.apgeochem.2014.04.008, 2014.
Vila-Escalé, M., Vegas-Vilarrúbia, T., and Prat, N.: Release of polycyclic aromatic compounds into a Mediterranean creek (Catalonia, NE Spain) after a forest fire, Water Res., 41, 2171–2179, https://doi.org/10.1016/j.watres.2006.07.029, 2007.
Wagner, S., Cawley, K. M., Rosario-Ortiz, F. L., and Jaffé, R.: In-stream sources and links between particulate and dissolved black carbon following a wildfire, Biogeochemistry, 124, 145–161, https://doi.org/10.1007/s10533-015-0088-1, 2015.
Wagner, S., Jaffé, R., and Stubbins, A.: Dissolved black carbon in aquatic ecosystems, Limnology and Oceanography Letters, 3, 168–185, https://doi.org/10.1002/lol2.10076, 2018.
Wampler, K. A., Bladon, K. D., and Myers-Pigg, A. N.: Data and Scripts describing dissolved organic carbon concentrations across a stream network in Oregon following the 2020 Holiday Farm Wildfire, Oregon State University [data set], https://doi.org/10.7267/zc77sz60m, 2024.
Wei, X., Hayes, D. J., and Fernandez, I.: Fire reduces riverine DOC concentration draining a watershed and alters post-fire DOC recovery patterns, Environ. Res. Lett., 16, 024022, https://doi.org/10.1088/1748-9326/abd7ae, 2021.
Williams, C. H. S., Silins, U., Spencer, S. A., Wagner, M. J., Stone, M., and Emelko, M. B.: Net precipitation in burned and unburned subalpine forest stands after wildfire in the northern Rocky Mountains, Int. J. Wildland Fire, 28, 750–760, https://doi.org/10.1071/WF18181, 2019.
Wilson, H. F. and Xenopoulos, M. A.: Ecosystem and Seasonal Control of Stream Dissolved Organic Carbon Along a Gradient of Land Use, Ecosystems, 11, 555–568, https://doi.org/10.1007/s10021-008-9142-3, 2008.
Wollheim, W. M., Stewart, R. J., Aiken, G. R., Butler, K. D., Morse, N. B., and Salisbury, J.: Removal of terrestrial DOC in aquatic ecosystems of a temperate river network, Geophys. Res. Lett., 42, 6671–6679, https://doi.org/10.1002/2015GL064647, 2015.
Writer, J. H., McCleskey, R. B., and Murphy, S. F.: Effects of wildfire on source-water quality and aquatic ecosystems, Colorado Front Range, IAHS Red Book, 354, 117–122, 2012.
Zimmer, M. A., Bailey, S. W., McGuire, K. J., and Bullen, T. D.: Fine scale variations of surface water chemistry in an ephemeral to perennial drainage network, Hydrol. Process., 27, 3438–3451, https://doi.org/10.1002/hyp.9449, 2013.