the Creative Commons Attribution 4.0 License.
the Creative Commons Attribution 4.0 License.
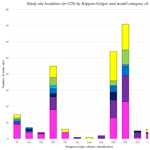
Reviews and syntheses: A scoping review evaluating the potential application of ecohydrological models for northern peatland restoration
Mark G. Healy
Laurence Gill
Peatland restoration and rehabilitation action has become more widely acknowledged as a necessary response to mitigating climate change risks and improving global carbon storage. Peatland ecosystems require restoration time spans of the order of decades and, thus, cannot be dependent upon the shorter-term monitoring often carried out in research projects. Hydrological assessments using geospatial tools provide the basis for planning restoration works as well as analysing associated environmental influences. “Restoration” encompasses applications to pre-restoration and post-restoration scenarios for both bogs and fens, across a range of environmental impact fields. The aim of this scoping review is to identify, describe, and categorize current process-based modelling uses in peatlands in order to investigate the applicability and appropriateness of ecohydrological and/or hydrological models for northern peatland restoration. Two literature searches were conducted using the entire Web of Science database in September 2022 and August 2023. Of the final 211 papers included in the review, models and their applications were categorized according to this review's research interests in seven distinct categories aggregating the papers' research themes and model outputs. Restoration site context was added by identifying 229 unique study site locations from the full database, which were catalogued and analysed against raster data for the Köppen–Geiger climate classification scheme. A majority of northern peatland sites were in temperate oceanic zones or humid continental zones that experienced snow. Over one in five models from the full database of papers were unnamed and likely intended for single use. Key themes emerging from topics covered by papers in the database included the following: modelling restoration development from a bog growth perspective, the prioritization of modelling greenhouse gas (GHG) emissions dynamics as a part of policymaking, the importance of spatial connectivity within or alongside process-based models to represent heterogeneous systems, and the increased prevalence of remote sensing and machine learning techniques to predict restoration progress with little physical site intervention. Models are presented according to their application to peatlands or broader ecosystem and organized from most to least complex. This review provides valuable context for the application of ecohydrological models in determining strategies for peatland restoration and evaluating post-intervention development over time.
- Article
(2408 KB) - Full-text XML
-
Supplement
(724 KB) - BibTeX
- EndNote
- Included in Encyclopedia of Geosciences
Peatlands play a vital role in global carbon (C) storage and climate regulation. However, their millennia-long cooling influence is now undermined through human activity, not least the active degradation of extensive areas of peatland and subsequently the effects of climate change (Helbig et al., 2022). In response, northern peatland restoration and rehabilitation activity has increased significantly with large-scale projects in industrial and governmental spheres. Restoration projects and related research often occur in “bursts” with typical spans of 4–5 years based on funding availability (e.g. the European Union LIFE projects) or collaborations between academic institutions and other organizations. However, peatland ecosystems take decades to millennia to develop, and, as such, their impact cannot be perfectly extrapolated from the shorter spans within which these projects are carried out (Bacon et al., 2017).
Restoration plans will need to vary in their responses according to the type of peatland degradation that has occurred. Examples of activities having contributed to peatland degradation include drainage for livestock agriculture, especially in northwestern (NW) Europe, and the creation of oil palm plantations in tropical regions such as Indonesia. Another example is peat harvesting for fuel or horticulture (where mining can extend down to the mineral soil in places), along with manual cutting along bog margins, which makes site-by-site hydrology extremely variable, especially where not well monitored. Frequently practised restoration strategies arising from peatland research and industrial action include the following: (1) inundation, achieved by efforts such as drain blocking, bunding, or cessation of pumping, which is favoured in its simplicity as a direct rewetting approach; (2) topsoil removal, which is achieved by removing the top layer of degrading soil (often less than 30 cm) and vegetation to mitigate nutrient export and/or minimize nutrient availability for the formation of new biomass; and (3) slow rewetting, which is a more controlled and progressive alternative to spontaneous inundation of long-term drained peatlands or costly topsoil removal, which diverge from standard restoration practices (Zak and McInnes, 2022).
When the natural hydrological functions of peatlands are disturbed, the ecosystems react sensitively, with consequences for whole-catchment hydrology, soil properties, water quality, and biodiversity (Dettmann et al., 2014). Environmental impacts of degraded or restored bogs (in their biodiversity, nutrient transport, or C emissions) also depend on site hydrology (Strack et al., 2022). There is an ecological link in the net impact of hydrology on climate change mitigation (as C storage or C sinks), which is a primary goal of restoration, alongside efforts to improve regional and nationwide biodiversity; from policy and management perspectives, peatlands are more often considered nature-based solutions to climate issues based on greenhouse gas (GHG) emissions, which have concomitant “co-benefits” for biodiversity and water quality (Strack et al., 2022). To reflect this, ecohydrological models include interactions between climate, hydrology, and landscape characteristics, producing outputs describing soil moisture, water level and flow, soil nutrient quantities, sediment transport, or vegetation community patterning or growth (Acharya et al., 2017). From this, the key hydrological functions of peatlands can be linked with climate-change-related GHG outputs or environmental protection-related water quality outputs, of which the former is a more prevalent (but no more important) topic in research than the latter according to a dynamic topic modelling study (Yang et al., 2023), especially considering the perceived urgency of peatland restoration as a response to mitigating climate change (Glenk et al., 2021).
Modelling peatland restoration is useful in its flexibility and in giving the potential to calibrate against different cases of rehabilitation and revegetation. Spatial hydrological assessments using models like MODFLOW (e.g. Brandyk et al., 2016), SIMGRO (e.g. Jaenicke et al., 2010; Povilaitis and Querner, 2008), SAGA (e.g. Ikkala et al., 2022), FLUSH (e.g. Haahti et al., 2016), TOPMODEL (e.g. Goudarzi et al., 2021), DigiBog_Hydro (e.g. Putra et al., 2022), or unnamed numerical algorithms (e.g. Kennedy and Price, 2004; Luscombe et al., 2016) provide the basis for creating a restoration plan, which often prioritizes raising the water table as the key engineering goal with outputs confined to groundwater head/flow, surface water level, or catchment runoff and discharge. Yet, despite decades of research, models of this kind rarely address the entirety of restoring peatlands (i.e. the degradation, mid-restoration, and long-term impact stages) in a complete, ecohydrological manner.
1.1 State-of-the-art models available to date
A variety of hydrological modelling approaches can be used in a northern peatland restoration context, including conceptual models; empirical models; physical (i.e. process-based/numerical) models (Lana-Renault et al., 2020); and, more recently, machine learning (ML) models (Shen et al., 2021). These models are not always ecohydrological in nature but can be manipulated to operate to this end.
The theoretical Bog Growth Model (BGM) provides a basis for twenty-first century conceptual models of peatland development (Clymo, 1978). The primary limitation of the BGM is a lack of accounting for cross-scale coupling of hydrological and ecological processes to improve insight into long-term peatland structure development; this is addressed in the conceptual model providing the basis for DigiBog (Belyea and Baird, 2006). Though, at the time of DigiBog's publication, feedback across temporal and spatial scales could not be properly incorporated, linkages between WTD (water table depth) and seven ecohydrological feedbacks have also been presented as a conceptual model for peatland functioning (Waddington et al., 2014). Net-positive feedback related to afforestation/shrubification of the landscape and soil-specific yield, and net-negative feedback related to Sphagnum moss surface resistance and productivity, peat deformation and decomposition, and groundwater transmissivity (Waddington et al., 2014). While connections to ecological processes are improved, some feedback still lacks process-level and/or transdisciplinary, ecohydrological understanding, especially where the ecological focus on Sphagnum's specific impact on hydrology is concerned (Waddington et al., 2014). More site-specific hydrological conceptual models have since been developed after extensive site-based monitoring to inform further predictions of restoration or climate change impacts (e.g. Lhosmot et al., 2021).
Models of peatland dynamics can be specific to climate-policy-related outputs rather than provide a full picture of hydrology and ecology. Empirically based statistical GHG estimation models have been developed for C budgeting, including a decision support tool (DST) to produce daily, monthly, or annual GHG budgets and a statistical model (SET) to annually calculate GHG budgets (van der Snoek et al., 2023) and a site-specific multiple-regression model to demonstrate responses between soil respiration, soil temperature, and WTD in a restoration context (Swails et al., 2022).
Process-based hydrological and ecological models can describe the functioning of specific peatlands or regions when accompanied by site-based monitoring and parameterization, especially in the case of GHG-flux estimation (Mozafari et al., 2023). The process-based DigiBog model (Baird et al., 2012; Morris et al., 2012) combines C accumulation with a hydrology submodule (Young et al., 2017). However, the C-accumulation submodule accounts only for deep-peat modification, meaning that there is a discrepancy between rapid near-surface C accumulation and overall C sinks in a peatland (Young et al., 2019). A newer paired hydrological and peat accumulation model is MPeat (Mahdiyasa et al., 2022), which aims to improve peat mechanical processes in modelling and has not yet been applied in a restoration context.
Recent studies also use ML to either replace or supplement process-based models, as site-specific knowledge for hydrology, topography, and soil properties makes process-based modelling difficult in large-scale applications (Koch et al., 2023). Active development of new ML models is occurring with increased specificity as well, such as being able to describe the distribution of fires in tropical peatlands for testing the potential impact of management and restoration scenarios (Horton et al., 2022).
Recent efforts have been made to identify available models for peatland dynamics (Mozafari et al., 2023), though the review made little explicit reference to peatland restoration. Additionally, a scientometric review was completed to identify papers that examine the restoration of degraded peatland in the current body of literature but does not include a focus on modelling (Apori et al., 2022).
1.2 Knowledge gaps
Restoring site hydrology (i.e. raising WTD) is often considered by engineers and policymakers the landmark for restoring an ecosystem. Desirable ecohydrological model outputs will depend upon (and likely go beyond) detailed site hydrology which, in the case of restoration, may create distinct zones of varying hydraulic behaviour contingent upon the scale of degradation or the restoration technique applied. Additionally, process-based models have infrequently been calibrated to describe degraded scenarios or a transition from a degraded to a restored scenario.
Modellers may be required to understand how and when habitats will change, how much new peat may accumulate long term, and the subsequent C-cycle implications of such evolutions, which are difficult to find in any single modelling exercise in the literature to date. There are very few state-of-the-art, process-based, ecohydrological models available to date which have been employed in a peatland restoration application (Mozafari et al., 2023). The potential application of existing models to peatland restoration (considering more than single-state scenarios) has, to date, not been explored systematically. Engineers, planners, researchers, and policymakers would benefit from being more informed about what models currently exist not only to describe peatlands but also to describe peatland restoration, which would be favoured over developing one's own model tailored to a single restoration scenario or location.
Therefore, the aim of this paper is to identify, describe, and categorize current process-based modelling uses on peatlands in order to investigate the applicability and appropriateness of ecohydrological and/or hydrological models for northern peatland restoration. This will unite ecohydrological and restoration modelling interests by interpreting the relevance of different models' output(s) to peatland restoration projects, with the expected goal to predict the success of restoration measures undertaken in northern peatlands. Hence, the exploratory nature of this review is scoping rather than systematic.
2.1 Literature searches: September 2022 and August 2023
A literature search was conducted using the entire Web of Science database in September 2022 and updated for the 2022–2023 period with a second search in August 2023. The following search string was employed in an advanced search: TS = (peat* AND ((model* AND hydrolog*) OR (model* AND GHG))). Note that there remains a significant knowledge gap in water quality modelling (Yang et al., 2023), such that GHG modelling was emphasized in this review, and no equivalent search term was applied for water quality. It was anticipated that the term “hydrolog*” would be sufficient to encompass water quality and nutrient transport modelling if these papers existed in the literature.
The combined search yielded 1116 results. These references were downloaded in .ris format and collated in the free, open-source reference management software, Zotero (Corporation for Digital Scholarship, George Mason University). The papers were screened three times in alphabetical order, with each iteration eliminating inappropriate titles and reducing the batch size.
While some papers in the search overlapped with the previous search, regular comparisons with the existing database were heeded to avoid double counting. This addition to the full dataset is shown in Table S3 in the Supplement.
2.2 Determination of appropriate models
Models were considered for their potential application in an ecohydrological manner, despite differences in the actual processes incorporated in individual model codes and whether or not they are strictly called ecohydrological (e.g. a purely hydrological model being shown to operate well in a peatland context). As it may be valuable to investigate long-term bog growth for systems so fundamentally changed by mining or degradation that returning to a “former” habitat is not likely, modelling organic matter decomposition and emission over the span of decades or centuries is pertinent for consideration beyond current hydrological and C dynamics. It is unlikely that any single model will be able to encapsulate all these interests for simulating a restored bog or other northern peatland region. Therefore, it was valuable to document which modelling options exist on local to global scales, as well as in different dimensions of space, time, or conceptual structure, and how these models operate in case some can be combined or used in tandem.
A first pass considered all paper titles and occasionally portions of paper abstracts for clarity. A second pass was made where the method sections of each article were read in full. This was done to identify which model interfaces are used and discard those papers that do not name any models or whose models' functions do not match the scope of this review. Criteria for the rejection of a number of articles fell into two themes: too much specificity or a lack of connection with hydrology (Table 1).
Table 1Details summarizing common topics in papers removed from the dataset prior to analysis. DNDC stands for DeNitrification–DeComposition.
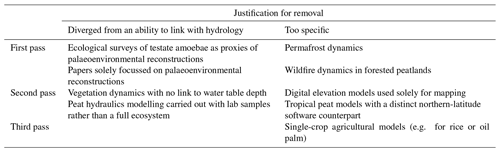
This review focusses on “northern” peatlands, existing above 50–40° N (with some emphasis placed on NW Europe, given this is a region of interest for the authors), as they are distinct enough in climate and land-use contexts from “tropical” peatlands such that modelling in these categories is generally known to diverge in the literature (Tarnocai and Stolbovoy, 2006). Some tropical peatland hydrology studies were retained that employ restoration-related modelling, which may be useful to identify, despite differences in climate and ecosystem characteristics.
A third and final pass was made during which pertinent information from the full articles was gathered to fill a summary table for each paper. The table, created for each model category, included the following subheadings: (1) sole focus on hydrology (Pure Hydrology); (2) focus on greenhouse gases and connected biochemistry (GHG Dynamics), with the ideal to prioritize process-based models, which went beyond calculating net ecosystem exchange or global warming potential as sole outputs; (3) description of long-term peat accumulation projections or reconstructions (Peat Accumulation); (4) regional or national scaled-up models involving northern peatlands looking beyond a site-specific scale (Global Models); (5) multiple models from previous categories used in tandem or in sequence (Model Combinations); and (6) models integrating or coupling processes included in previous categories (Coupled Models). Pertinent information from the second literature search was added to a separate table with identical headers to the original database except for an additional column “category” linking the papers to the previously generated model categories (five papers appeared relevant but did not neatly fit into any of the existing model categories; their sole focus covered restoration-related remote sensing topics and were categorized as (7) “Remote sensing” to reflect this). Studies performed by the same first author(s) on the same or similar site(s) were regarded together as a “suite” during analysis but remained separate for subsequent tables and figures.
A final total of 211 relevant papers were retained in the database. The database was manually reviewed for key models of interest and their relative frequency within the full set (in which 224 models are identified for the 211 papers in the database). Considerations for making this selection were as follows.
-
If coupled model packages already exist that appear flexible enough to apply to a northern peatland context, these should be prioritized, especially considering the FAIR (findable, accessible, interoperable, and reusable) accessibility guidelines, which can improve research communication and future application of models beyond academia (Mozafari et al., 2023).
-
As demonstrated in Table 2, just over one-fifth of the total database consisted of unnamed, often numerical models that were handmade for specific research needs and rarely used more than once or twice. They are therefore less likely to contribute significantly to the sharing of modelling methods because their accessibility is limited to the creator. Additionally, the consolidation of approaches improves confidence in modelling, making the use of unnamed models less beneficial, along with less commonly used models serving similar purposes to more commonly used ones.
-
Focussing on the ecological side of ecohydrological modelling became pertinent because of the wealth of background already present for pure hydrological models and this review's emphasis upon moving beyond hydrology as the priority for restoration targets.
Table 2Relative frequencies of named and unnamed models in this review.
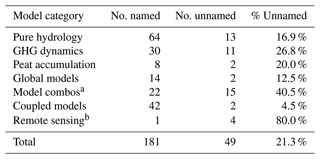
a 11 of 13 papers in this category list two models per paper. A total of 23 distinct models are counted here based on the information available from the papers.
b Remote sensing papers featured prominently from a second post hoc literature search; most are categorized in other categories if possible. However, five papers remained distinct in their objectives and were given their own category.
The full dataset, including each paper's DOI, first author, year published, model(s) used, and a brief description of the research scope, is summarized completely in Tables S1 and S3 in the Supplement. Within the 211 recorded papers, 229 unique study site locations were identified, catalogued, and analysed against raster data for the Köppen–Geiger climate classification scheme (Tables S2 and S4 in the Supplement): developed by Wladimir Köppen and Rudolf Geiger and published by Köppen in 1936, the system has since become an established multidisciplinary standard for describing the climate of a region (Rubel et al., 2017). This was done in order to evaluate the potential prevalence/preference for the use of different models depending on the climate conditions of sites on which they were tested. Additionally, data types and durations used in some papers were documented as the review progressed to provide a fuller picture of the potential utilization of key models.
Post hoc analysis of shortlisted models occurred to explore what information will aid potential modellers in determining either the suitability of a single model for their purposes or the possible compatibility of combining existing codes or running models in tandem. This centred around general model specifications and information about data inputs as discussed above. Observations and conclusions made based on data solely from the second literature search will be designated as such.
The following observations were made for papers collated within the seven model categories.
Pure Hydrology. A total of 77 papers were catalogued for this category, making it the largest (approximately 36.5 % of the full database). MODFLOW featured here prominently, with 15 papers using the model, which is around 19.5 % of all models in this category. Only six papers across the entire category (7.8 %) were published in 2022 or 2023, three of which came from the second literature search. A total of two of the four water-quality-related papers are featured in this category (Sutton and Price, 2022; Nieminen et al., 2018).
GHG Dynamics. A total of 41 papers were found for this category, which makes up approximately 19 % of the full database. Additionally, it is possible that some models in this category are in fact “integrated” or “coupled” (i.e. they include hydrological or other processes, as well as the necessary biochemistry), but the only outputs are GHG-flux related. A total of 10 papers across the category (25 %) were published in 2022 or 2023, 9 of which came from the second literature search.
Peat Accumulation. A total of 10 papers were found for this category, making it the smallest (approximately 5 % of the full database). The majority of models use historical climate reconstructions as the basis of the model. The papers in this section also had an average publication year of 2009, making this category “older” than the others with average ages between 2014 and 2016. No new peat accumulation topics were identified in the second literature search except for one paper classified as a Model Combination, which incorporates peat accumulation.
Global Models. A total of 16 papers were found for this category, which makes up approximately 7.5 % of the full database. While it was not expected for many of these papers to be relevant to the current study, because individual countries may appear as only 5–10 cells within a large grid and individual peatland sites may be indistinguishable, it may still be important to account for the models used here, especially those which represent a “northern peatland” condition. Only one paper in this category had been published since 2021; all others were published in 2020 or earlier.
Model Combinations. A total of 19 papers were found for this category, which makes up approximately 9 % of the full database. Model Combinations either present outputs not encapsulated by a single model or include the processes from two or more models to yield a more robust output. There may be more combinations among the other categories, which are not presented outright, but these papers were considered to be unique because of the combinations they employ. Eight papers across the category (42 %) were published in 2022 or 2023, six of which came from the second literature search; the other two of four water-quality-related papers are featured in this category (Xu et al., 2020; Bernard-Jannin et al., 2018).
Coupled/Integrated Models. The second-largest category (approx. 20.5 % of the full database) consists of coupled models housed within the same interface or code, with 43 total items. Some “suites” of papers from the same authors occur here where model development can be tracked, or multiple outputs are analysed for the same sites and published separately over a number of years.
Remote sensing. Finally, an emerging trend regarding models that include a remote sensing aspect was found in the past year (2022–2023): almost one in three (929) papers from the second literature search included remote sensing data or was based on a remote sensing classification model. Five of these papers (Ball et al., 2023; Dabrowska-Zielinska et al., 2022; Dadap et al., 2022; Jussila et al., 2023; Puertas Orozco et al., 2023) presented their approach and results as distinctly remote sensing oriented and were placed in their own remote sensing category for post hoc discussion. These take up the remaining 2.5 % of the total database.
A total of 13 of the 31 Köppen–Geiger classifications were represented by the peatlands modelled in this review (Fig. 1). Coupled models were applied in the largest quantities for the three most abundant classifications (Dfc, Dfb, and Cfb, in that order). It was only in the Cfb region where every identified model category is applied; the Dfb region did not host any remote sensing research, and the Dfc region did not host any peat accumulation research. Note that Dfc (subarctic climate), Cfb (warm-summer temperate oceanic), and Cfc (cool-summer temperate oceanic) classifications describe most of NW Europe, and Dfb (warm-summer humid continental climate) primarily describes Canadian sites, which are continental rather than coastal, experiencing more snow. A GIS map layout plotting the coordinate locations of individual study sites is included in Fig. 2. For the three least commonly studied climate region classifications (Cwb, Csa, and Dfa), models used fell into the Coupled Models and GHG Dynamics applications, especially with a focus on methane emissions (Wania et al., 2010; Walter et al., 1996).
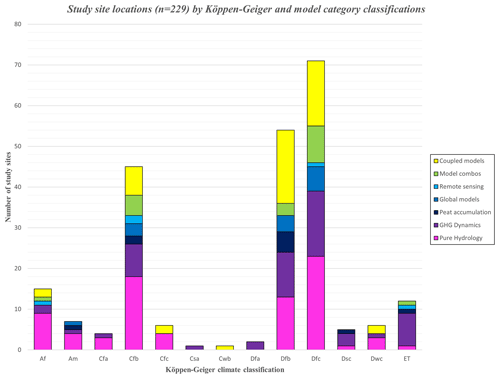
Figure 1Study site locations by Köppen–Geiger climate regions. Much of NW Europe, a region of interest for this review's authors, is classified entirely as Cfb. For a full breakdown of climate region classifications and their abbreviations, see Kottek et al. (2006).
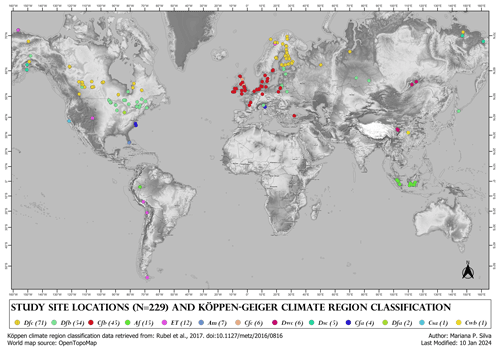
Figure 2Individual site locations for the 229 sites identified in papers from this review, with their corresponding Köppen–Geiger climate region classifications. Map generated using QGIS. Base map © OpenTopoMap.
A total of 17 papers did not use physical study sites, many of which were global or regional studies scaling up data from a wealth of site-specific research. Three papers (Bechtold et al., 2020; Qiu et al., 2018, 2019) catalogued too many sites to document meaningfully (these were also housed within the Global Models category).
The following process-based models had the highest frequency of use within and across model categories (Table 3): MODFLOW (Pure Hydrology); DigiBog, including DigiBog_Hydro (Peat Accumulation, Pure Hydrology, and Coupled Models); CoupModel (Coupled Models); ecosys (Coupled Models and GHG Dynamics); McGill Wetland Model (MWM), including CLASS3W-MWM (GHG Dynamics); PEAT-CLSM (Global Models); and LPJ, including LPJ-GUESS and LPJ-WHyMe (GHG Dynamics). No model was used more than twice in either the Model Combinations or the Peat Accumulation categories. Note that MODFLOW, a household name in hydrological modelling for decades, was not used in Model Combinations or for any applications beyond Pure Hydrology in this review; other Pure Hydrology models, which were used in Model Combinations, include PERSiST and TOPMODEL.
Table 3Names of models recorded in the database, condensed to show only those appearing more than once within a category or once in a category where it exists more than once in another. Models in bold are discussed further in this review's discussion.
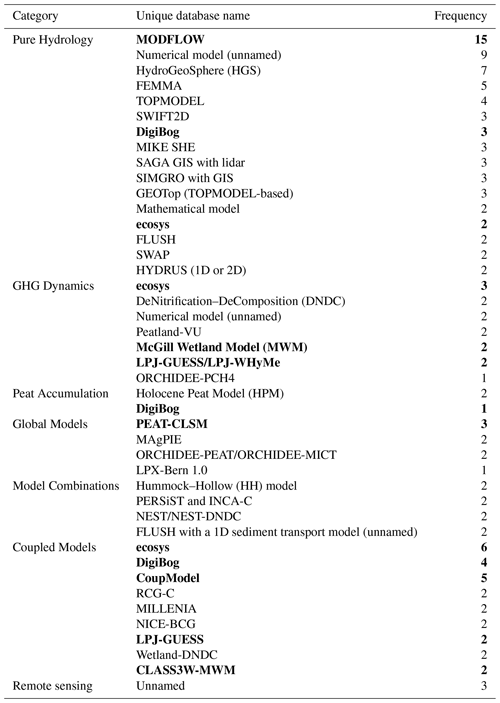
The three models that were used the most across the board, based on the frequency of their use on distinct site locations, were LPJ, MODFLOW, and ecosys, in that order (Table 4). Note that no recent (2022–2023) papers used models highlighted in Table 4 except for CoupModel. For each of the models, the focus of the climate region differs. Papers using DigiBog concentrated their focus on theory (“no location listed”) and UK sites (Cfb), given that the model was developed by researchers from the UK. LPJ and ecosys focus heavily on Df regions, where 5 of 14 ecosys study locations (36 %) are within the Dfb region, and 7 of 16 LPJ study locations (44 %) are within the Dfc region, mirroring the general observation that more peatland modelling research occurred in these regions across all studies (Fig. 1). The more deterministic of the most-used models, incorporating the largest number of processes representing living organisms (vegetation growth, microbial processes, etc.) in peatlands, were MWM, CoupModel, and ecosys (described by Grant et al., 2017, as “process-rich”).
Table 4Instances of use for most frequently used models, organized by the Köppen–Geiger climate region classification. “Instances” may occur more than once within the same paper for different sites; here, they are recorded as separate counts. Bolded values emphasize the more frequently used models across all climate regions (bottom) and the more frequently studied climate regions across all model types (right).
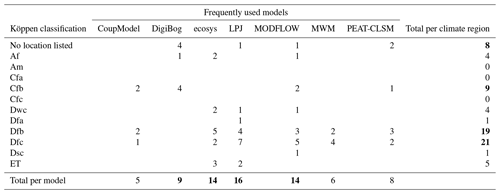
The highest dimension attempted by simulations outside of Pure Hydrology occurs only once in this review for 3D (ecosys: Grant et al., 2017) and once for pseudo 3D (DigiBog_Hydro: Putra et al., 2022). Examples of 3D modelling more common with Pure Hydrology include a MODFLOW model from Sutton and Price (2022), which also includes hydrochemical transport, and a GEOTop model from Zi et al. (2016).
The models arising in this review's database that are featured the most are discussed here, along with models which may have appeared less often, to present an overview of current model options and identify trends in order of process complexity. Note that “process complexity” here is interpreted as the degree to which a process is difficult to observe, understand, or explain (McDonnell et al., 2007). This is different from simply capturing the number of processes (especially when attributing and discretizing equations for such) in different models, but these properties are often connected.
4.1 Peatland-specific models: most to least complex
Within past decades, numerical models have been formulated and refined to represent peatland-specific complexities, especially considering changes occurring in past designations of “acrotelm/catotelm” layers (Clymo, 1978), incorporating characteristics interacting with hydrological boundary conditions (such as bog shape) and addressing feedback specific to peat decomposition (Morris et al., 2011).
McGill Wetland Model. This process-based terrestrial ecosystem model has been developed since 2008 with multiple versions of different names. The foundation is in vascular plant energy exchange using plant functional types (PFTs) and considering photosynthesis, conductance, and respiration decomposition of vegetation (St-Hilaire et al., 2010). CLASS3W-MWM, a coupled version of the McGill Wetland Model (MWM), simulates GHG dynamics across multiple climate scenario projections (Wu and Roulet, 2014). The 1D model represents C fluxes as net ecosystem production (NEP) for representative single-year batches across a century, and it was able to support fen–bog transitions from circa 2000 to 2100, including imposed climate change scenarios, to predict resiliency. The threshold between MWM-modelled C sinks/sources was shown in the balance between gross primary production and decomposition, concluding that the soil C response of bogs to climate change is determined chiefly by vegetation production and decomposition in the acrotelm (Wu and Roulet, 2014).
In the vulnerable years at the start of bog restoration, models may need to represent vegetation growth and associated ecohydrological changes with greater detail than what is demonstrated with older versions of MWM, but this has been addressed in two newer versions of the model: MWMmic and MWMmic_NP (Shao et al., 2022a, b). With the addition of a multi-layer cohort structure, peat decomposability now decreases with peat depth (Shao et al., 2022a), allowing for the modelling of degraded, formerly deep-peat layers to decompose and release nutrients distinctively from ideal, less consolidated “natural” peat cases. Further, vegetation growth and competition (moss and shrubs) have been linked with hydrology and nutrient availability in newer versions (Shao et al., 2022b), improving the ecohydrological character of the model. MWM is not publicly available, and it is not stipulated how to request access.
Peatland-VU and PVN. Peatland-VU and its contemporary, Peatland-VU-NUCOM (PVN), were developed to model site-scale peatland GHG emissions and dynamic vegetation (Lippmann et al., 2023). They present themselves similarly to the CoupModel discussed below (both are 1D) except abridged to a peatland context and with a focus on emissions outputs. Parameters do not focus on complex hydrology (beyond requiring WTD input data), and vegetation is organized into PFTs with variable relative biomass quantities depending on competition for light relative to parameter-defined growth requirements. The models are free and available as Fortran and C++ source code on Zenodo (https://www.bitbucket.org/tlippmann/pvn_public, last access: 27 February 2024).
DNDC and Wetland-DNDC. Wetland-DNDC (DeNitrification–DeComposition) appeared in the Coupled Models category twice, and the core DNDC model appeared in the GHG Dynamics category twice. Wetland-DNDC has been superseded by Forest-DNDC (Gilhespy et al., 2014), which is more often used in boreal forested peatlands (Kim et al., 2016), though Wetland-DNDC is still occasionally being used (e.g. Mikhalchuk et al., 2022). The models focus on C and nitrogen (N) cycling with an original focus on agriculture with uses in natural contexts (Webster et al., 2013). The primary focus is on biochemical processes, requiring common soil chemical, hydrological, and climate inputs, as well as vegetation variables in the form of “crops”. Wetland-DNDC and Forest-DNDC consider wetland restoration as a major management practice and have been parameterized to represent this; however, the models are not physically based and require numerous parameters for microbially mediated chemical transformations.
LPJ-WHyMe. Originating from the Lund–Potsdam–Jena model (LPJ), this version simulates wetland hydrology and methane dynamics for global, regional, or site-scale vegetation modelling (Wania et al., 2010). It was developed specifically for understanding permafrost dynamics on northern peatlands and then expanded to consider methane emissions. The model uses PFTs as the conceptual basis for vegetation growth, with a simplified focus on wetland vegetation and a separate category just for Sphagnum moss. The model code (Fortran 77) is available by request through the Oak Ridge National Laboratory. The model takes in meteorological information, soil type, land and ocean mask maps, and parameter values as well as text files for relevant climate, vegetation, methane, and soil variables. The model appeared less often than LPJ-GUESS (discussed below), and, while they have a shared conceptual basis, they have since diverged. Recent works not captured in this review have used LPJ-WHyMe in non-wetland or partial wetland scenarios (Huang et al., 2024; Sun et al., 2020; Sun and Mu, 2022), possibly due to its capabilities in permafrost dynamics.
ELM-SPRUCE. The ELM-SPRUCE model was developed based on the Energy Exascale Earth System Model (E3SM) and Community Land Model (CLM) for use in Oak Ridge National Laboratory's SPRUCE experiment on peatlands in Minnesota, USA (https://mnspruce.ornl.gov/, last access: 6 March 2024). It is specific to the boreal peatland ecosystem on which it was developed, with a particular focus on improving spatial methane dynamics to be integrated back into the E3SM global model as an ultimate goal (Yuan et al., 2021).
PEAT-CLSM. The PEAT-CLSM model is a northern peatland-specific land surface hydrology model developed on top of NASA's Catchment Land Surface Model (CLSM), using a TOPMODEL approach (discussed below), where microtopography as hummocks and hollows is emphasized, along with peatland-specific vegetation (especially in surface water ponds), and peat soil hydraulic properties are incorporated as parameters (Bechtold et al., 2020). While the peatland-specific improvements are simple to conceptualize, computational units on the global scale (as catchments, which can be discretized into grid cells) introduce complexity. The model is available as sections of NASA's CLSM catchment.F90 code that was modified to become PEAT-CLSM modules (https://osf.io/e58ym/, last access: 6 March 2024).
Modelling hummocks and hollows. Several papers have produced numerical models specifically to capture dynamics in hummock–hollow patterning (including ridge–slough dynamics in the Florida Everglades). These were largely unnamed (Couwenberg, 2005; Eppinga et al., 2009; Heffernan et al., 2013; Kaplan et al., 2012), with two named models arising. One is HOHUM (Nungesser, 2003), though it has not been expanded upon or applied in the literature since its inception, and the other is Hummock–Hollow (HH; Cresto Aleina et al., 2015, 2016), which integrates the relationship between microtopography and emissions fluxes, for which the theory has been cited in papers using the ELM-SPRUCE (Ricciuto et al., 2021) and ORCHIDEE (Yao et al., 2022) models but is not directly applied in new scenarios.
MPeat and MPeat2D. MPeat is similar to HPM and DigiBog but includes peat mechanical processes within a 1D peat column. It introduces more complex relationships for bulk density, active porosity, and hydraulic conductivity, and it also includes Young's modulus as an additional variable (Mahdiyasa et al., 2021). A newer version, MPeat2D, has expanded on the spatial capabilities of the model and has added an ecological submodule but with limited application due to its recent development (Mahdiyasa et al., 2023). It is free and available as MATLAB code (https://zenodo.org/records/10050891, last access: 6 March 2024).
Holocene Peat Model (HPM). Most prominently used for reconstructions of peatlands in past climates, HPM was applied to learn more about the nature of peat accumulation against C-dated peat cores (Quillet et al., 2013; Liu et al., 2018). It is 1D (where it is meant to model the centre of an ombrotrophic peatland) and uses 12 PFTs from which productivity and decomposition are balanced to develop rates of peat accumulation, along with some peat physical properties such as variable bulk density. The model was used in a comparison with MPeat, which was previously discussed (Mahdiyasa et al., 2021), and it has not been made publicly available except in a modified Arctic permafrost version (Treat et al., 2021) (https://zenodo.org/records/4647666, last access: 6 March 2024).
DigiBog and DigiBog_Hydro. The peat accumulation model DigiBog is particularly concise when considering ecosystem functioning, choosing to represent the plant growth process through the relative rates of litterfall and decay to the atmosphere (Morris et al., 2012). The 2D application of DigiBog for a blanket peatland has been used to investigate the impacts of peatland drains and drain blocking (Young et al., 2017; Fig. 3). In some DigiBog simulations, the water table can be fixed or forced before evaluating bog response, allowing for WTD predictions to vary based on human interventions (Young et al., 2017). It should be noted that a constant bulk density is used in DigiBog.
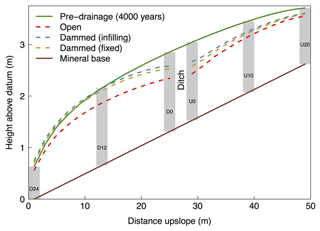
Figure 3Predicted peatland surface height before and after (300 years) ditch drainage and damming imposed upon a blanket bog transect, modelled using DigiBog by Young et al. (2017). Peat accumulation is modelled here alongside hydrology for the annotated peat columns (e.g. D24, D12); columns are identified using their position upslope (U) or downslope (D) of the ditch and the distance (m) of their edges that are nearest the ditch (e.g. column U10 occurs 10 m upslope of the ditch).
DigiBog_Hydro has connectivity with GIS for determining peatland-specific hydrology in a 2D plane, with existing applications to a restoration context (Putra et al., 2022). The required inputs for DigiBog_Hydro are entered into a GUI, so no coding knowledge is required unless further modifications are attempted. Additionally, the user manual is put together well and freely available. DigiBog_Hydro is a submodel of DigiBog and thus neglects some of the peat accumulation elements of the full DigiBog model (and similarly assumes a constant bulk density), though it can provide a starting point to obtain a hydrological “snapshot” for a site area before (or concurrently with) connecting to peat accumulation or ecosystem dynamics over time. Both models are free, where DigiBog is publicly available and DigiBog_Hydro is available by request (https://water.leeds.ac.uk/our-missions/mission-1/digibog/resources/, last access: 6 March 2024).
4.2 General models: most to least complex
ecosys. The output capabilities of the fully coupled mathematical ecohydrological model ecosys overlap with many other models of interest. Its extreme process richness requires explicit understanding of the theory governing plant substrate and microbial populations, which acquire, transform, and exchange resources (as energy, water, C, N, and phosphorus (P)), and the computational power for simulations in 1D, 2D, or 3D, with multiple canopy and soil layers and with sub-hourly time steps (Grant, 2013). The model is free with documentation available by request (https://github.com/jinyun1tang/ECOSYS, last access: 6 March 2024). The complexity of ecosys does not inherently make the model a “better” choice; in fact, the model overestimated and underestimated seasonal GHG fluxes similarly to less complex models across multiple land cover types, perhaps calling into question the necessity of such detail if outputs are not significantly more accurate or precise (Sulman et al., 2012). For the purposes of evaluating ecohydrology, however, ecosys matches the performance from the ecological process side while also simulating WTD, unlike other models reported by Sulman et al. (2012) which do not have hydrological outputs.
Earlier ecosys models were applied on a bog (Dimitrov et al., 2010) and later on a peatland transition zone (Dimitrov et al., 2014), focussing solely on hydrological outputs. Models did not display very high accuracy (R2 between 0.40 and 0.56) for monitoring WTD and water content in the intact bog but were even less accurate without including a macropore flow process (R2 between 0.27 and 0.41). Model applications have since expanded to fully coupled ecohydrology in both peatland and non-peatland contexts. ecosys has also been used for coupled ecohydrological modelling in a Canadian boreal fen (Mezbahuddin et al., 2017), controlling lateral flow between a chosen number of cells, while including microbial activity and carbon dioxide (CO2) fluxes (which can be reduced to gross primary production (GPP) or NEP) and simulating WTDs within the plane with decent accuracy (R2 between 0.68 and 0.84 for CO2 fluxes).
MODFLOW, MODFLOW-SURFACT, and MODPATH. A 3D finite-difference groundwater flow model with added vadose-zone capabilities, MODFLOW is well known for being developed by the USGS and frequently used and improved upon. Indeed, the most recent release of MODFLOW 6 does include an unsaturated zone flow package but still focusses on groundwater transport (https://water.usgs.gov/water-resources/software/MODFLOW-6/, last access: 6 March 2024). Input and output parameters are too numerous to list, and the model requires knowledge of finite-difference cell-based discretization concepts; within the field of pure hydrological modelling, there may be a prerequisite for knowledge in computational modelling, but MODFLOW's demands may not combine best with general ecosystem process modelling. MODPATH demonstrates water quality connectivity, though none of the papers using MODPATH on peatlands in this review simulate water quality (Reeve et al., 2000, 2001a, b, 2009; Reeve and Gracz, 2008). Older versions of MODFLOW, such as MODFLOW-SURFACT, may prove simpler to use for peatlands where the vadose zone is more critical than groundwater flow (Sutton and Price, 2022).
ORCHIDEE. The global land surface model ORCHIDEE consists of a trunk version for global applications of water–energy C budgets as well as branches for more detailed simulations such as high-latitude peatland C dynamics (Largeron et al., 2018; Qiu et al., 2019; Kwon et al., 2022). The model is free and available as Fortran code (https://orchidee.ipsl.fr/you-orchidee/, last access: 6 March 2024) though with extensive computing power and data inputs required which are too numerous to name here.
CoupModel. This 1D, fully integrated process-based model can simultaneously simulate WTD and CO2 fluxes based on water, soil organic and inorganic, and canopy and/or groundcover vegetation energy processes (e.g. Kasimir et al., 2018; He et al., 2023a, b). Like ecosys, CoupModel requires a rigorous understanding of manifold chemical and energy transformation processes; however, CoupModel is housed within a browser user interface (UI), rather than requiring coding knowledge, where visualization of the soil column and water and energy fluxes is simple but available to the user. The model provides numerous “switch” specifications for selecting if certain processes should be considered and in what manner (e.g. choosing to include snowmelt processes or soil freezing and thawing or neither) to determine the ultimate number of parameters necessary in a given simulation. While default parameters exist, along with some soil and vegetation categories based on a past simulation database, the total number of parameters is still extensive and requires site-based knowledge for calibration. The UI of the model is free and available by request on the CoupModel website (https://www.coupmodel.com/downloading-of-model, last access: 18 April 2024).
Soil and Water Assessment Tool (SWAT). As an ecosystem-based model, SWAT has been adapted for uses ranging from farmland nutrient tracing to cold wetland watershed organic cycling (Kalcic et al., 2015). It is 2D and formed on the basis of hydrologic response units (HRUs), which are user-defined areas of similar land use, soil type, and topographical characteristics. The watershed conceptualization is configured via spatial objects, differentiating HRUs and collecting them into a “landscape unit”, with a “routing unit” connecting delineated aquifer(s), channel(s), and reservoir(s) in the landscape. The model requires upwards of 30 input files; a pre-existing database provides groups for plants, generic land covers, and a number of associated parameters, and little to no pre-processing or post-processing is required outside of the interface, which is similar to CoupModel. SWAT executables as command-line code, as well as GIS-integrated interfaces ArcSWAT and QSWAT, are publicly available on the SWAT website (https://swat.tamu.edu/, last access: 6 March 2024).
While it did not feature as often in frequency in a peatland context, the SWAT model modified by Melaku et al. (2022) adds a wetland-specific emissions subroutine, which estimates groundwater table, CO2 emissions, and net ecosystem exchange spatially and temporally. The number of currently incorporated HRUs limits the resolution available for classifying different kinds of wetlands, which may pose a challenge for demonstrating heterogeneity in site-scale modelling (Melaku et al., 2022).
LPJ-GUESS. LPJ-GUESS is a dynamic global vegetation model designed for a global to regional scale incorporating plant physiology of “individuals” within a stand (as PFTs) as well as population dynamics and soil biogeochemistry. It was originally developed for bioclimatic zones where entire countries may be represented by a single set of PFTs (Smith et al., 2001). The model code (C++) is publicly available (https://web.nateko.lu.se/lpj-guess/download.html, last access: 7 March 2024). Dissolved organic carbon (DOC) and “water routing” modules have also been added to the model; the simulated DOC transport through a watershed (excluding gaseous C fluxes) demonstrated higher DOC quantities in peatlands (bog and fen) than in mineral soils (Tang et al., 2018). However, there is no indication of the consideration of peat growth or microtopography, though litter and soil organic matter turnover is an included process.
SIMGRO. The mechanistic distributed SIMGRO model is a combination of the MetaSWAP land surface and unsaturated zone and the MODFLOW phreatic zone models, housed in a GIS interface. The model is not publicly available, and it is not clearly stipulated if downloads can be requested (https://www.wur.nl/en/research-results/research-institutes/environmental-research/facilities-tools/software-models-and-databases/simgro.htm, last access: 6 March 2024). SIMGRO is able to generate a 3D spatial representation of WTD rise pre-restoration and post-restoration across an entire domed peatland area (Fig. 4), though with no connection to carbon cycling or peat accumulation processes (Jaenicke et al., 2010). The simulated hydrology was related to C storage by providing an index (developed by Couwenberg et al. in 2009) for C stored in a peatland of a given area per centimetre of groundwater rise. While this model does not feature prominently in this review or within recent northern peatland applications, SIMGRO remains one of the few models in this review which specifically carries out a multidimensional representation of peatland restoration.
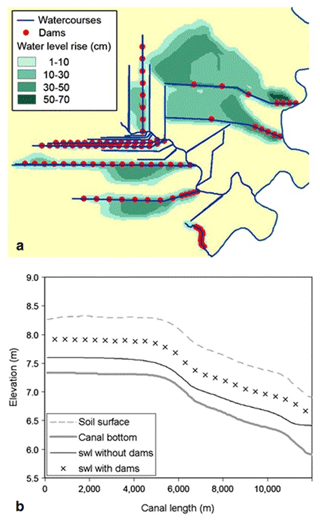
Figure 4GIS-based predictions of water level rise for a tropical peatland using SIMGRO (Jaenicke et al., 2010). (a) Plan view of groundwater level rise after dam construction. (b) Surface water level rise (relative to soil surface elevation) in a single canal after dam construction.
GEOTop. GEOTop, a contemporary of TOPMODEL, is a watershed-distributed hydrological model which couples water and energy balances. It is free and available as Fortran code through a web repository (https://github.com/geotopmodel/geotop, last access: 6 March 2024). Inputs include elevation as a digital terrain model, soil-type mapping, land-use mapping, and hourly meteorological time-series data. The representation of vegetation growth as energy flux (i.e. net radiation, sensible, latent, and ground heat fluxes) calls for inputs of land cover maps with fixed vegetation zones and solving the energy equation in 1D despite solving the water balance in 3D (Zi et al., 2016).
TOPMODEL. TOPMODEL is a topographically based model describing the dynamics of surface and subsurface saturated areas using simplified storage–discharge relationships in steady state. A grid cell size of ≤50 m is recommended so that it can be flexible for smaller catchments and yield a good idea of water transport and its connectivity with water quality. Hydrological and topographic inputs are all that are required for TOPMODEL, though outputs are only in the form of a hydrograph and sensitivity analysis; therefore, it remains distinct from any ecological processes. It is free and available online as Fortran code (https://cran.r-project.org/web/packages/topmodel/index.html, last access: 26 February 2024). It was used in 2006 in tandem with InTEC 3.0 as a simple hydrological input to model GHGs on a multi-year, national scale on peatlands (Ju et al., 2006), and it has been used more recently in more pure hydrological scenarios (Beven et al., 2021).
Modelling products (Visual MODFLOW© and HydroGeoSphere). Visual MODFLOW has simplified much of the set-up work and visualization by framing MODFLOW (including the newest version) in a GUI for groundwater flow and solute transport only (used in Brust et al., 2017; see specifications at https://www.waterloohydrogeologic.com/products/visual-modflow-flex/; last access: 8 March 2024). However, this is a modelling product and exists behind a paywall, diverging from FAIR modelling ideals. HydroGeoSphere (HGS™) is fully integrated 3D finite-element software carrying out similar modelling ends to MODFLOW except with a node and element mesh rather than with cells (https://www.aquanty.com/hydrogeosphere, last access: 20 June 2024). HGS™ has been used alongside a global sensitivity analysis technique to reduce the number of parameters required to model groundwater–surface water interactions in aapa mires (Jaros et al., 2019). While the physically based model produces spatially intuitive results, it may require separate software for visualization on top of being a product behind a paywall, again diverging from FAIR modelling ideals.
HYDRUS. Being housed in a GUI (Windows application), behind a paywall, the HYDRUS program numerically solves the Richards equation for variably saturated flow, which is most commonly used in pure hydrology applications (e.g. Hokanson et al., 2021) with abundant applications in non-peatland-specific scenarios. Available in 1D, 2D, and 3D, the software is often used in peatland solute transport applications (Šimůnek et al., 2024). Vegetation is modelled as crops in the program insofar as to inform water and solute uptake through the root zone rather than considering changes in vegetation dynamics (Šimůnek et al., 2024). The application of HYDRUS to peatlands can be done in scenarios of controlled vegetation and topography to investigate other properties of the peatland's functioning.
PERSiST and INCA-C. This model combination was used in two separate papers captured in this review (Xu et al., 2020; de Wit et al., 2016), with precedents for this combination made by Futter et al. in 2007, 2009, and 2014. The combination captures carbon cycling with INCA-C and watershed hydrology with PERSiST, both models requiring very few measured data inputs: a total of three daily time-series datasets, with additional calibration and validation site measurements required for soil carbon or DOC (Xu et al., 2020). INCA-C conceptualizes the carbon cycle by simulated transformations between C pools and fluxes between water pools, organized into organic soils, mineral soils, and open-water categories; PERSiST is a derivative rainfall-runoff model with specific focus on solute transport (Futter et al., 2014). R2 values ranged in evaluation periods from 0.44 to 0.78 for simulated discharge and from 0.29 to 0.69 for DOC (Xu et al., 2020), with an even better performance of 0.85 in an earlier study (de Wit et al., 2016). Considering that key hydrological outputs had decent accuracy with significantly less computation required, taking a less process-complex approach may be beneficial in cases where desired outputs are few. However, this approach hinders the ability to investigate the results' implications for other processes in the natural system not parameterized in the model (e.g. the DOC quantities simulated here cannot inform as much about vegetation dynamics or decomposition).
4.3 Purely data-driven models
This review did not focus on statistical or empirical models in favour of comparing the complexity of process-based models and the potential for representing peatland restoration from a bottom-up, theory-based approach.
However, it is notable that machine learning models applied to peatland restoration, while not having a foundation in key environmental processes on the ground, featured with increasing frequency. Models with direct links to spatial imagery software can more easily incorporate already-existing satellite data or mapping data to hopefully reduce process complexity. The five papers which stood apart from the pre-existing analysis' model categories included outputs centred around predictions of soil moisture, vegetation communities and their extents, and resulting gross primary productivity estimates; while these outputs have hydrological and ecological themes, they do not directly incorporate hydrological or ecological processes and, rather, favour using the existing body of research, along with ML predictions, to form conclusions about the nature of peatland ecosystems with little to no physical intervention. Four other recent (2022–2023) papers gathered in the Model Combinations and GHG Dynamics categories also featured satellite data with ML classification, though these were treated as data inputs to other models rather than being the end goal of the research.
The challenge posed in bringing together similar types of ML code for this type of review is a lack of an official title for these programs apart from well-known algorithms like Random Forest (e.g. Kou et al., 2022; Rissanen et al., 2023; Ross et al., 2023). Until now, there has not been much consolidation of data or codes used for remote sensing projects. As the field of ecohydrological modelling progresses, the connection with spatial imagery may become more prominent and favoured not only as a way to incorporate more data into models when site-level instrumentation proves too difficult to orchestrate but also as a standalone technique for predicting hydrological and ecological changes and especially for enacting regional or nationwide policy decisions.
4.4 Synthesis
The most complex models presented are generally less specific to peatlands and are fully coupled (e.g. ecosys), which requires a large input of data and knowledge to achieve the flexibility to model as many physical or chemical outputs in as many landscapes as possible. However, general ecosystem models (most often applied for GHG Dynamics or Global Models on peatlands) may be just as complex as some pure hydrological models, where each model may generate entirely separate outputs with few common inputs. Challenges with many general ecosystem process-based models remain in that simulating changes in peat near-surface characteristics (i.e. key features such as bulk density, macroporosity, and vegetation) over time due to restoration is not integrated and may need to be forced, and these programs do not account for peat volume change in the long term. A key trend in applicability of many of the GHG Dynamics and peatland-specific models (e.g. MWM, PVN, and some applications of CoupModel) is the representation of whole peatland ecosystems with 1D points where studies may have broadly compared bog and fen ecosystems, climate scenarios, etc.
There is a considerable lack of recent focus upon peat accumulation modelling as demonstrated by this review, especially by the absence of this category in recent (2022–2023) papers; furthermore, where peat accumulation modelling was studied, these simulations existed mostly in Cfb and Dfb regions with few other sites. A limited representation of climate regions here does not necessarily imply a lacking global picture of peat accumulation research; however, research on the palaeoecological peat accumulation rate that contributes to scientific understanding about worldwide peat accumulation is not considered in this review. It is only observed that the simulation of worldwide peat accumulation (especially for future projections) is scarce. In studies using peat accumulation models (e.g. HPM, MPeat, DigiBog), changes in soil characteristics (especially where bare peat rehabilitation is concerned) over time have not been investigated. Though this transition in hydraulic behaviour has been studied in laboratory-scale models (e.g. Gauthier et al., 2018) and in the field (e.g. Lehan et al., 2022), it has yet to be demonstrated by the process-based models in this review, such that the representation of bare peat rehabilitation may still pose a challenge for modelling. These peat accumulation models also do not include biogeochemical changes associated with the fen–bog transition. These changes occur along geologic timescales when the water table becomes consistently high enough to cease meaningful interactions with groundwater (Wu and Roulet, 2014) or more quickly due to sudden drops in WTD (e.g. volcanic impacts, Loisel and Bunsen, 2020, and intensive peatland rehabilitation works, Malloy and Price, 2014). However, this would appear as a mere fraction of typical spin-up and simulation timescales.
When modelling degraded and rehabilitating landscapes, for which there is little precedent, further field measurements and model modifications will be needed to model the dynamics of new vegetation communities arising in these conditions. He et al. (2023a) recently modelled GHG emissions coupled with hydrological and chemical impacts of an actively extracted peatland using CoupModel, establishing a precedent for this interface. A large number of models (especially coupled ecohydrological models) use PFTs to categorize vegetation parameters more simply, being either fixed to the locations initialized or dynamic and competing for resources throughout the simulations. Additionally, setting up land-use boundaries for HRUs may be a useful way to delineate restoration techniques within a bog site or to examine interactions with nearby agricultural lands; however, significant assumptions would need to be made in creating such classifications.
Given that many restoration actions primarily control the water table, such as cell bunding or weir-managed ditches, it is a valuable application of many of the models simulating peatlands to focus on WTD as both an output and a driving variable. Also, the use of general ecosystem models on peatlands often has an overarching goal of future incorporation into global climate models (e.g. Sulman et al., 2012), which deviates from the goals of field-based peatland restoration. However, organizing a process-based ecosystem modelling system to analyse C fluxes in restored/rehabilitated peatlands is of interest, especially during the period of time required to reach some form of ecosystem stability. If specific metrics are being developed to characterize restoring sites, such as estimating short-term methane emissions resulting from rewetting or longer-term vegetation community extents to incorporate in land-use change projections, it may be advantageous to maintain a “base” model with a site-level hydrological focus and subsequently vary a connected ecological model element relative to a desired output (i.e. Model Combinations like in Bernard-Jannin et al., 2018; Booth et al., 2022; Wilson et al., 2022). Compared to using a fully coupled model outlining all possible site-level process interactions, this approach may not require as many data or as much parameter calibration, time, and power to compute results.
The application of ecohydrological process-based models is a promising field for exploring strategies for peatland restoration and evaluating post-intervention development over time. Modelling can contribute to the combined responsibilities of restoring peatland sites effectively and tracking subsequent environmental impacts; this review highlights a number of models with existing applications to peatland contexts and the potential for application to peatland restoration, though it is not exhaustive.
While this review has stressed the sphere of GHG emissions of modelling peatland environments ecohydrologically, the vegetation community and water/soil nutrient outputs from ecohydrological models may be of additional value. It is probable that more process-rich, general models such as ecosys or CoupModel have the flexibility to produce outputs with implications for biodiversity or water quality as well, given that foundational biogeochemical processes are present. Water quality did not appear as a prominent area of research where, if modelling for a particular “end” had an environmental focus, it favoured GHG emissions (partly caused by the specified search string where GHG modelling was sought out specifically); an additional review into water quality modelling, as it relates to ecohydrology, would be valuable. Predicting long-term peat accumulation (such as with DigiBog) may be less compatible with models producing other desired outputs. It may still be valuable to develop peat depth projections as a result of current restoration efforts, though this may need to remain a separate and supplementary focus to the more prominent ecohydrological component. Additionally, the introduction of vegetation on a previously bare landscape is (understandably) not included in most studies reviewed and may need to be forced to mimic a restoration “event” over a period of time. Expansion upon existing models is active and ongoing in the field, for example with increasing the number of dimensions in MPeat2D or adding new processes in MWMmic_NP. Finally, while the majority of this review has discussed process-based models, statistical (especially regression) models and ML provide additional ways to conceptualize and predict peatland processes; as requirements for spatial connectivity prove useful for policymaking or engineering design, newer research appears to diverge from a site-by-site focus while still remaining distinct from scaled-up global models.
Where current restoration efforts may focus primarily on raising WTD as a proxy for “successful” rewetting, here it may be argued that evaluating the success of peatland restoration, especially for former industrial extraction sites, must include the monitoring and projections for emissions, nutrient loading, and other ecosystem changes without assuming that improved hydrology tends to improve environmental impact. As such, there may be additional management options required for peatland restoration in the future, which could alter the demands of ecohydrological modelling and go beyond the considerations from this review.
The authors confirm that the data supporting the findings of this study are available within the article and its Supplement.
The supplement related to this article is available online at: https://doi.org/10.5194/bg-21-3143-2024-supplement.
MPS conducted the literature searches and all subsequent analysis. LG provided advice throughout the review process. MGH proofread manuscript drafts to prepare for submission.
The contact author has declared that none of the authors has any competing interests.
Publisher's note: Copernicus Publications remains neutral with regard to jurisdictional claims made in the text, published maps, institutional affiliations, or any other geographical representation in this paper. While Copernicus Publications makes every effort to include appropriate place names, the final responsibility lies with the authors.
We would like to thank Siya Shao for their contributions with regard to MWM advancements. We would also like to thank the reviewers for their valuable comments and criticisms.
This research has been supported by the Environmental Protection Agency (grant no. 17783, project code 2021-NE-1036).
This paper was edited by Paul Stoy and reviewed by two anonymous referees.
Acharya, S., Kaplan, D. A., Jawitz, J. W., and Cohen, M. J.: Doing ecohydrology backward: Inferring wetland flow and hydroperiod from landscape patterns, Water Resour. Res., 53, 5742–5755, https://doi.org/10.1002/2017WR020516, 2017.
Apori, S. O., Mcmillan, D., Giltrap, M., and Tian, F.: Mapping the restoration of degraded peatland as a research area: A scientometric review, Front. Environ. Sci., 10, 942788, https://doi.org/10.3389/fenvs.2022.942788, 2022.
Bacon, K. L., Baird, A. J., Blundell, A., Bourgault, M.-A., Chapman, P. J., Dargie, G., Dooling, G. P., Gee, C., Holden, J., Kelly, T., McKendrick-Smith, K. A., Morris, P. J., Noble, A., Palmer, S. M., Quillet, A., Swindles, G. T., Watson, E. J., and Young, D. M.: Questioning ten common assumptions about peatlands, Mires Peat, 19, 1–23, https://doi.org/10.19189/MaP.2016.OMB.253, 2017.
Baird, A. J., Morris, P. J., and Belyea, L. R.: The DigiBog peatland development model 1: rationale, conceptual model, and hydrological basis, Ecohydrology, 5, 242–255, https://doi.org/10.1002/eco.230, 2012.
Ball, J., Gimona, A., Cowie, N., Hancock, M., Klein, D., Donaldson-Selby, G., and Artz, R. R. E.: Assessing the Potential of using Sentinel-1 and 2 or high-resolution aerial imagery data with Machine Learning and Data Science Techniques to Model Peatland Restoration Progress – a Northern Scotland case study, Int. J. Remote Sens., 44, 2885–2911, https://doi.org/10.1080/01431161.2023.2209916, 2023.
Bechtold, M., De Lannoy, G. J. M., Reichle, R. H., Roose, D., Balliston, N., Burdun, I., Devito, K., Kurbatova, J., Strack, M., and Zarov, E. A.: Improved groundwater table and L-band brightness temperature estimates for Northern Hemisphere peatlands using new model physics and SMOS observations in a global data assimilation framework, Remote Sens. Environ., 246, 111805, https://doi.org/10.1016/j.rse.2020.111805, 2020.
Belyea, L. R. and Baird, A. J.: Beyond “the limits to peat bog growth”: Cross-scale feedback in peatland development, Ecol. Monogr., 76, 299–322, https://doi.org/10.1890/0012-9615(2006)076[0299:BTLTPB]2.0.CO;2, 2006.
Bernard-Jannin, L., Binet, S., Gogo, S., Leroy, F., Défarge, C., Jozja, N., Zocatelli, R., Perdereau, L., and Laggoun-Défarge, F.: Hydrological control of dissolved organic carbon dynamics in a rehabilitated Sphagnum-dominated peatland: a water-table based modelling approach, Hydrol. Earth Syst. Sci., 22, 4907–4920, https://doi.org/10.5194/hess-22-4907-2018, 2018.
Beven, K. J., Kirkby, M. J., Freer, J. E., and Lamb, R.: A history of TOPMODEL, Hydrol. Earth Syst. Sci., 25, 527–549, https://doi.org/10.5194/hess-25-527-2021, 2021.
Booth, E. G., Loheide, S. P., and Bart, D.: Fen ecohydrologic trajectories in response to groundwater drawdown with an edaphic feedback, Ecohydrology, 15, e2471, https://doi.org/10.1002/eco.2471, 2022.
Brandyk, A., Majewski, G., Kiczko, A., Boczon, A., Wrobel, M., and Porretta-Tomaszewska, P.: Ground Water Modelling for the Restoration of Carex Communities on a Sandy River Terrace, Sustainability, 8, 1324, https://doi.org/10.3390/su8121324, 2016.
Brust, K., Krebs, M., Wahren, A., Gaudig, G., and Joosten, H.: The water balance of a Sphagnum farming site in north-west Germany, Mires Peat, 20, 1–12, https://doi.org/10.19189/MaP.2017.OMB.301, 2017.
Clymo, R. S.: A Model of Peat Bog Growth, in: Production Ecology of British Moors and Montane Grasslands, vol. 27, edited by: Heal, O. W. and Perkins, D. F., Springer-Verlag, Berlin, Heidelberg, 187–223, https://doi.org/10.1007/978-3-642-66760-2_9, 1978.
Couwenberg, J.: A simulation model of mire patterning – revisited, Ecography, 28, 653–661, https://doi.org/10.1111/j.2005.0906-7590.04265.x, 2005.
Cresto Aleina, F., Runkle, B. R. K., Kleinen, T., Kutzbach, L., Schneider, J., and Brovkin, V.: Modeling micro-topographic controls on boreal peatland hydrology and methane fluxes, Biogeosciences, 12, 5689–5704, https://doi.org/10.5194/bg-12-5689-2015, 2015.
Cresto Aleina, F., Runkle, B. R. K., Brücher, T., Kleinen, T., and Brovkin, V.: Upscaling methane emission hotspots in boreal peatlands, Geosci. Model Dev., 9, 915–926, https://doi.org/10.5194/gmd-9-915-2016, 2016.
Dabrowska-Zielinska, K., Misiura, K., Malinska, A., Gurdak, R., Grzybowski, P., Bartold, M., and Kluczek, M.: Spatiotemporal estimation of gross primary production for terrestrial wetlands using satellite and field data, Remote Sensing Applications-Society and Environment, 27, 100786, https://doi.org/10.1016/j.rsase.2022.100786, 2022.
Dadap, N. C., Cobb, A. R., Hoyt, A. M., Harvey, C. F., Feldman, A. F., Im, E.-S., and Konings, A. G.: Climate change-induced peatland drying in Southeast Asia, Environ. Res. Lett., 17, 074026, https://doi.org/10.1088/1748-9326/ac7969, 2022.
de Wit, H. A., Ledesma, J. L. J., and Futter, M. N.: Aquatic DOC export from subarctic Atlantic blanket bog in Norway is controlled by seasalt deposition, temperature and precipitation, Biogeochemistry, 127, 305–321, https://doi.org/10.1007/s10533-016-0182-z, 2016.
Dettmann, U., Bechtold, M., Frahm, E., and Tiemeyer, B.: On the applicability of unimodal and bimodal van Genuchten–Mualem based models to peat and other organic soils under evaporation conditions, J. Hydrol., 515, 103–115, https://doi.org/10.1016/j.jhydrol.2014.04.047, 2014.
Dimitrov, D. D., Grant, R. F., Lafleur, P. M., and Humphreys, E. R.: Modeling the effects of hydrology on ecosystem respiration at Mer Bleue bog, J. Geophys. Res.-Biogeo., 115, G04043, https://doi.org/10.1029/2010JG001312, 2010.
Dimitrov, D. D., Bhatti, J. S., and Grant, R. F.: The transition zones (ecotone) between boreal forests and peatlands: Modelling water table along a transition zone between upland black spruce forest and poor forested fen in central Saskatchewan, Ecol. Model., 274, 57–70, https://doi.org/10.1016/j.ecolmodel.2013.11.030, 2014.
Eppinga, M. B., de Ruiter, P. C., Wassen, M. J., and Rietkerk, M.: Nutrients and Hydrology Indicate the Driving Mechanisms of Peatland Surface Patterning, Am. Nat., 173, 803–818, https://doi.org/10.1086/598487, 2009.
Futter, M. N., Erlandsson, M. A., Butterfield, D., Whitehead, P. G., Oni, S. K., and Wade, A. J.: PERSiST: a flexible rainfall-runoff modelling toolkit for use with the INCA family of models, Hydrol. Earth Syst. Sci., 18, 855–873, https://doi.org/10.5194/hess-18-855-2014, 2014.
Gauthier, T. J., McCarter, C. P. R., and Price, J. S.: The effect of compression on Sphagnum hydrophysical properties: Implications for increasing hydrological connectivity in restored cutover peatlands, Ecohydrology, 11, e2020, https://doi.org/10.1002/eco.2020, 2018.
Gilhespy, S. L., Anthony, S., Cardenas, L., Chadwick, D., Del Prado, A., Li, C., Misselbrook, T., Rees, R. M., Salas, W., Sanz-Cobena, A., Smith, P., Tilston, E. L., Topp, C. F. E., Vetter, S., and Yeluripati, J. B.: First 20 years of DNDC (DeNitrification DeComposition): Model evolution, Ecol. Model., 292, 51–62, https://doi.org/10.1016/j.ecolmodel.2014.09.004, 2014.
Glenk, K., Faccioli, M., Martin-Ortega, J., Schulze, C., and Potts, J.: The opportunity cost of delaying climate action: Peatland restoration and resilience to climate change, Global Environ. Chang., 70, 102323, https://doi.org/10.1016/j.gloenvcha.2021.102323, 2021.
Goudarzi, S., Milledge, D. G., Holden, J., Evans, M. G., Allott, T. E. H., Shuttleworth, E. L., Pilkington, M., and Walker, J.: Blanket Peat Restoration: Numerical Study of the Underlying Processes Delivering Natural Flood Management Benefits, Water Resour. Res., 57, e2020WR029209, https://doi.org/10.1029/2020WR029209, 2021.
Grant, R. F.: Modelling changes in nitrogen cycling to sustain increases in forest productivity under elevated atmospheric CO2 and contrasting site conditions, Biogeosciences, 10, 7703–7721, https://doi.org/10.5194/bg-10-7703-2013, 2013.
Grant, R. F., Mekonnen, Z. A., Riley, W. J., Arora, B., and Torn, M. S.: Mathematical Modelling of Arctic Polygonal Tundra with Ecosys: 2. Microtopography Determines How CO2 and CH4 Exchange Responds to Changes in Temperature and Precipitation, J. Geophys. Res.-Biogeo., 122, 3174–3187, https://doi.org/10.1002/2017JG004037, 2017.
Haahti, K., Warsta, L., Kokkonen, T., Younis, B. A., and Koivusalo, H.: Distributed hydrological modeling with channel network flow of a forestry drained peatland site, Water Resour. Res., 52, 246–263, https://doi.org/10.1002/2015WR018038, 2016.
He, H., Clark, L., Lai, O. Y., Kendall, R., Strachan, I., and Roulet, N. T.: Simulating Soil Atmosphere Exchanges and CO2 Fluxes for an Ongoing Peat Extraction Site, Ecosystems, 26, 1335–1348, https://doi.org/10.1007/s10021-023-00836-2, 2023a.
He, H., Moore, T., Humphreys, E. R., Lafleur, P. M., and Roulet, N. T.: Water level variation at a beaver pond significantly impacts net CO2 uptake of a continental bog, Hydrol. Earth Syst. Sci., 27, 213–227, https://doi.org/10.5194/hess-27-213-2023, 2023b.
Heffernan, J. B., Watts, D. L., and Cohen, M. J.: Discharge Competence and Pattern Formation in Peatlands: A Meta-Ecosystem Model of the Everglades Ridge-Slough Landscape, PLOS ONE, 8, e64174, https://doi.org/10.1371/journal.pone.0064174, 2013.
Helbig, M., Živković, T., Alekseychik, P., Aurela, M., El-Madany, T. S., Euskirchen, E. S., Flanagan, L. B., Griffis, T. J., Hanson, P. J., Hattakka, J., Helfter, C., Hirano, T., Humphreys, E. R., Kiely, G., Kolka, R. K., Laurila, T., Leahy, P. G., Lohila, A., Mammarella, I., Nilsson, M. B., Panov, A., Parmentier, F. J. W., Peichl, M., Rinne, J., Roman, D. T., Sonnentag, O., Tuittila, E.-S., Ueyama, M., Vesala, T., Vestin, P., Weldon, S., Weslien, P., and Zaehle, S.: Warming response of peatland CO2 sink is sensitive to seasonality in warming trends, Nat. Clim. Change, 12, 743–749, https://doi.org/10.1038/s41558-022-01428-z, 2022.
Hokanson, K. J., Thompson, C., Devito, K., and Mendoza, C. A.: Hummock-scale controls on groundwater recharge rates and the potential for developing local groundwater flow systems in water-limited environments, J. Hydrol., 603, 126894, https://doi.org/10.1016/j.jhydrol.2021.126894, 2021.
Horton, A. J., Lehtinen, J., and Kummu, M.: Targeted land management strategies could halve peatland fire occurrences in Central Kalimantan, Indonesia, Commun. Earth Environ., 3, 204, https://doi.org/10.1038/s43247-022-00534-2, 2022.
Huang, R., Chen, X., Hu, Q., Jiang, S., and Dong, J.: Impacts of altitudinal ecohydrological dynamic changes on water balance under warming climate in a watershed of the Qilian Mountains, China, Sci. Total Environ., 908, 168070, https://doi.org/10.1016/j.scitotenv.2023.168070, 2024.
Ikkala, L., Ronkanen, A.-K., Ilmonen, J., Simila, M., Rehell, S., Kumpula, T., Pakkila, L., Klove, B., and Marttila, H.: Unmanned Aircraft System (UAS) Structure-From-Motion (SfM) for Monitoring the Changed Flow Paths and Wetness in Minerotrophic Peatland Restoration, Remote Sens.-Basel, 14, 3169, https://doi.org/10.3390/rs14133169, 2022.
Jaenicke, J., Wosten, H., Budiman, A., and Siegert, F.: Planning hydrological restoration of peatlands in Indonesia to mitigate carbon dioxide emissions, Mitig. Adapt. Strat. Gl., 15, 223–239, https://doi.org/10.1007/s11027-010-9214-5, 2010.
Jaros, A., Rossi, P. M., Ronkanen, A.-K., and Klove, B.: Parameterisation of an integrated groundwater-surface water model for hydrological analysis of boreal aapa mire wetlands, J. Hydrol., 575, 175–191, https://doi.org/10.1016/j.jhydrol.2019.04.094, 2019.
Ju, W., Chen, J., Black, T., Barr, A., Mccaughey, H., and Roulet, N.: Hydrological effects on carbon cycles of Canada's forests and wetlands, Tellus B, 58, 16–30, https://doi.org/10.1111/j.1600-0889.2005.00168.x, 2006.
Jussila, T., Heikkinen, R. K., Anttila, S., Aapala, K., Kervinen, M., Aalto, J., and Vihervaara, P.: Quantifying wetness variability in aapa mires with Sentinel-2: towards improved monitoring of an EU priority habitat, Remote Sens. Ecol. Conserv., 10, 172–187, https://doi.org/10.1002/rse2.363, 2023.
Kalcic, M. M., Chaubey, I., and Frankenberger, J.: Defining Soil and Water Assessment Tool (SWAT) hydrologic response units (HRUs) by field boundaries, Int. J. Agr. Biol. Eng., 8, 69–80, https://doi.org/10.3965/j.ijabe.20150803.951, 2015.
Kaplan, D. A., Paudel, R., Cohen, M. J., and Jawitz, J. W.: Orientation matters: Patch anisotropy controls discharge competence and hydroperiod in a patterned peatland, Geophys. Res. Lett., 39, L17401, https://doi.org/10.1029/2012GL052754, 2012.
Kasimir, A., He, H., Coria, J., and Norden, A.: Land use of drained peatlands: Greenhouse gas fluxes, plant production, and economics, Global Change Biol., 24, 3302–3316, https://doi.org/10.1111/gcb.13931, 2018.
Kennedy, G. and Price, J.: Simulating soil water dynamics in a cutover bog, Water Resour. Res., 40, W12410, https://doi.org/10.1029/2004WR003099, 2004.
Kim, Y., Roulet, N. T., Li, C., Frolking, S., Strachan, I. B., Peng, C., Teodoru, C. R., Prairie, Y. T., and Tremblay, A.: Simulating carbon dioxide exchange in boreal ecosystems flooded by reservoirs, Ecol. Model., 327, 1–17, https://doi.org/10.1016/j.ecolmodel.2016.01.006, 2016.
Koch, J., Elsgaard, L., Greve, M. H., Gyldenkærne, S., Hermansen, C., Levin, G., Wu, S., and Stisen, S.: Water-table-driven greenhouse gas emission estimates guide peatland restoration at national scale, Biogeosciences, 20, 2387–2403, https://doi.org/10.5194/bg-20-2387-2023, 2023.
Kottek, M., Grieser, J., Beck, C., Rudolf, B., and Rubel, F.: World map of the Köppen-Geiger climate classification updated, Metz, 15, 259–263, https://doi.org/10.1127/0941-2948/2006/0130, 2006.
Kou, D., Virtanen, T., Treat, C. C., Tuovinen, J.-P., Rasanen, A., Juutinen, S., Mikola, J., Aurela, M., Heiskanen, L., Heikkila, M., Weckstrom, J., Juselius, T., Piilo, S. R., Deng, J., Zhang, Y., Chaudhary, N., Huang, C., Valiranta, M., Biasi, C., Liu, X., Guo, M., Zhuang, Q., Korhola, A., and Shurpali, N. J.: Peatland Heterogeneity Impacts on Regional Carbon Flux and Its Radiative Effect Within a Boreal Landscape, J. Geophys. Res.-Biogeo., 127, e2021JG006774, https://doi.org/10.1029/2021JG006774, 2022.
Kwon, M. J., Ballantyne, A., Ciais, P., Qiu, C., Salmon, E., Raoult, N., Guenet, B., Gockede, M., Euskirchen, E. S., Nykanen, H., Schuur, E. A. G., Turetsky, M. R., Dieleman, C. M., Kane, E. S., and Zona, D.: Lowering water table reduces carbon sink strength and carbon stocks in northern peatlands, Global Change Biol., 28, 6752–6770, https://doi.org/10.1111/gcb.16394, 2022.
Lana-Renault, N., Morán-Tejeda, E., Moreno de las Heras, M., Lorenzo-Lacruz, J., and López-Moreno, N.: Land-use change and impacts, in: Water Resources in the Mediterranean Region, Elsevier, 257–296, https://doi.org/10.1016/B978-0-12-818086-0.00010-8, 2020.
Largeron, C., Krinner, G., Ciais, P., and Brutel-Vuilmet, C.: Implementing northern peatlands in a global land surface model: description and evaluation in the ORCHIDEE high-latitude version model (ORC-HL-PEAT), Geosci. Model Dev., 11, 3279–3297, https://doi.org/10.5194/gmd-11-3279-2018, 2018.
Lehan, K., McCarter, C. P. R., Moore, P. A., and Waddington, J. M.: Effect of stockpiling time on donor-peat hydrophysical properties: Implications for peatland restoration, Ecol. Eng., 182, 106701, https://doi.org/10.1016/j.ecoleng.2022.106701, 2022.
Lhosmot, A., Collin, L., Magnon, G., Steinmann, M., Bertrand, C., Stefani, V., Toussaint, M., and Bertrand, G.: Restoration and meteorological variability highlight nested water supplies in middle altitude/latitude peatlands: Towards a hydrological conceptual model of the Frasne peatland, Jura Mountains, France, Ecohydrology, 14, e2315, https://doi.org/10.1002/eco.2315, 2021.
Lippmann, T. J. R., van der Velde, Y., Heijmans, M. M. P. D., Dolman, H., Hendriks, D. M. D., and van Huissteden, K.: Peatland-VU-NUCOM (PVN 1.0): using dynamic plant functional types to model peatland vegetation, CH4, and CO2 emissions, Geosci. Model Dev., 16, 6773–6804, https://doi.org/10.5194/gmd-16-6773-2023, 2023.
Liu, X., Chen, H., Zhu, Q., Wu, J., Frolking, S., Zhu, D., Wang, M., Wu, N., Peng, C., and He, Y.: Holocene peatland development and carbon stock of Zoige peatlands, Tibetan Plateau: a modeling approach, J. Soil. Sediment., 18, 2032–2043, https://doi.org/10.1007/s11368-018-1960-0, 2018.
Loisel, J. and Bunsen, M.: Abrupt Fen-Bog Transition Across Southern Patagonia: Timing, Causes, and Impacts on Carbon Sequestration, Frontiers in Ecology and Evolution, 8, 273, https://doi.org/10.3389/fevo.2020.00273, 2020.
Luscombe, D. J., Anderson, K., Grand-Clement, E., Gatis, N., Ashe, J., Benaud, P., Smith, D., and Brazier, R. E.: How does drainage alter the hydrology of shallow degraded peatlands across multiple spatial scales?, J. Hydrol., 541, 1329–1339, https://doi.org/10.1016/j.jhydrol.2016.08.037, 2016.
Mahdiyasa, A. W., Large, D. J., Muljadi, B. P., Icardi, M., and Triantafyllou, S.: MPeat-A fully coupled mechanical-ecohydrological model of peatland development, Ecohydrology, 15, e2361, https://doi.org/10.1002/eco.2361, 2022.
Mahdiyasa, A. W., Large, D. J., Icardi, M., and Muljadi, B. P.: MPeat2D – A fully coupled mechanical-ecohydrological model of peatland development in two dimensions, EGUsphere [preprint], https://doi.org/10.5194/egusphere-2023-2535, 2023.
Malloy, S. and Price, J. S.: Fen restoration on a bog harvested down to sedge peat: A hydrological assessment, Ecol. Eng., 64, 151–160, https://doi.org/10.1016/j.ecoleng.2013.12.015, 2014.
McDonnell, J. J., Sivapalan, M., Vaché, K., Dunn, S., Grant, G., Haggerty, R., Hinz, C., Hooper, R., Kirchner, J., Roderick, M. L., Selker, J., and Weiler, M.: Moving beyond heterogeneity and process complexity: A new vision for watershed hydrology, Water Resour. Res., 43, 2006WR005467, https://doi.org/10.1029/2006WR005467, 2007.
Melaku, N. D., Wang, J., and Meshesha, T. W.: Modeling the Dynamics of Carbon Dioxide Emission and Ecosystem Exchange Using a Modified SWAT Hydrologic Model in Cold Wetlands, Water, 14, 1458, https://doi.org/10.3390/w14091458, 2022.
Mezbahuddin, M., Grant, R. F., and Flanagan, L. B.: Coupled eco-hydrology and biogeochemistry algorithms enable the simulation of water table depth effects on boreal peatland net CO2 exchange, Biogeosciences, 14, 5507–5531, https://doi.org/10.5194/bg-14-5507-2017, 2017.
Mikhalchuk, A., Borilo, L., Burnashova, E., Kharanzhevskaya, Y., Akerman, E., Chistyakova, N., Kirpotin, S. N., Pokrovsky, O. S., and Vorobyev, S.: Assessment of Greenhouse Gas Emissions into the Atmosphere from the Northern Peatlands Using the Wetland-DNDC Simulation Model: A Case Study of the Great Vasyugan Mire, Western Siberia, Atmosphere, 13, 2053, https://doi.org/10.3390/atmos13122053, 2022.
Morris, P. J., Waddington, J. M., Benscoter, B. W., and Turetsky, M. R.: Conceptual frameworks in peatland ecohydrology: looking beyond the two-layered (acrotelm-catotelm) model, Ecohydrology, 4, 1–11, https://doi.org/10.1002/eco.191, 2011.
Morris, P. J., Baird, A. J., and Belyea, L. R.: The DigiBog peatland development model 2: ecohydrological simulations in 2D, Ecohydrology, 5, 256–268, https://doi.org/10.1002/eco.229, 2012.
Mozafari, B., Bruen, M., Donohue, S., Renou-Wilson, F., and O'Loughlin, F.: Peatland dynamics: A review of process-based models and approaches, Sci. Total Environ., 877, 162890, https://doi.org/10.1016/j.scitotenv.2023.162890, 2023.
Nieminen, M., Palviainen, M., Sarkkola, S., Lauren, A., Marttila, H., and Finer, L.: A synthesis of the impacts of ditch network maintenance on the quantity and quality of runoff from drained boreal peatland forests, Ambio, 47, 523–534, https://doi.org/10.1007/s13280-017-0966-y, 2018.
Nungesser, M.: Modelling microtopography in boreal peatlands: hummocks and hollows, Ecol. Model., 165, 175–207, https://doi.org/10.1016/S0304-3800(03)00067-X, 2003.
Povilaitis, A. and Querner, E. P.: Possibilities to Restore Natural Water Regime in the Zuvintas Lake and Surrounding Wetlands – Modelling Analysis Approach, J. Environ. Eng. Landsc., 16, 105–112, https://doi.org/10.3846/1648-6897.2008.16.105-112, 2008.
Puertas Orozco, O. L., Paz Cardenas, M., Barria Meneses, J., Lizama Sanchez, T., and Jimenez Nunez, H.: Detection of long-term changes by multispectral analysis in the high-altitude Andean wetlands vegetation's: Michincha case study, 1985–2019, Rev. Geogr. Norte Gd., 84, 177–200, https://doi.org/10.4067/S0718-34022023000100177, 2023.
Putra, S. S., Baird, A. J., and Holden, J.: Modelling the performance of bunds and ditch dams in the hydrological restoration of tropical peatlands, Hydrol. Process., 36, e14470, https://doi.org/10.1002/hyp.14470, 2022.
Qiu, C., Zhu, D., Ciais, P., Guenet, B., Krinner, G., Peng, S., Aurela, M., Bernhofer, C., Brümmer, C., Bret-Harte, S., Chu, H., Chen, J., Desai, A. R., Dušek, J., Euskirchen, E. S., Fortuniak, K., Flanagan, L. B., Friborg, T., Grygoruk, M., Gogo, S., Grünwald, T., Hansen, B. U., Holl, D., Humphreys, E., Hurkuck, M., Kiely, G., Klatt, J., Kutzbach, L., Largeron, C., Laggoun-Défarge, F., Lund, M., Lafleur, P. M., Li, X., Mammarella, I., Merbold, L., Nilsson, M. B., Olejnik, J., Ottosson-Löfvenius, M., Oechel, W., Parmentier, F.-J. W., Peichl, M., Pirk, N., Peltola, O., Pawlak, W., Rasse, D., Rinne, J., Shaver, G., Schmid, H. P., Sottocornola, M., Steinbrecher, R., Sachs, T., Urbaniak, M., Zona, D., and Ziemblinska, K.: ORCHIDEE-PEAT (revision 4596), a model for northern peatland CO2, water, and energy fluxes on daily to annual scales, Geosci. Model Dev., 11, 497–519, https://doi.org/10.5194/gmd-11-497-2018, 2018.
Qiu, C., Zhu, D., Ciais, P., Guenet, B., Peng, S., Krinner, G., Tootchi, A., Ducharne, A., and Hastie, A.: Modelling northern peatland area and carbon dynamics since the Holocene with the ORCHIDEE-PEAT land surface model (SVN r5488), Geosci. Model Dev., 12, 2961–2982, https://doi.org/10.5194/gmd-12-2961-2019, 2019.
Quillet, A., Garneau, M., and Frolking, S.: Sobol' sensitivity analysis of the Holocene Peat Model: What drives carbon accumulation in peatlands?, J. Geophys. Res.-Biogeos., 118, 203–214, https://doi.org/10.1029/2012JG002092, 2013.
Reeve, A., Siegel, D., and Glaser, P.: Simulating vertical flow in large peatlands, J. Hydrol., 227, 207–217, https://doi.org/10.1016/S0022-1694(99)00183-3, 2000.
Reeve, A., Siegel, D., and Glaser, P.: Simulating dispersive mixing in large peatlands, J. Hydrol., 242, 103–114, https://doi.org/10.1016/S0022-1694(00)00386-3, 2001a.
Reeve, A., Warzocha, J., Glaser, P., and Siegel, D.: Regional ground-water flow modeling of the Glacial Lake Agassiz Peatlands, Minnesota, J. Hydrol., 243, 91–100, https://doi.org/10.1016/S0022-1694(00)00402-9, 2001b.
Reeve, A. S. and Gracz, M.: Simulating the hydrogeologic setting of peatlands in the Kenai Peninsula Lowlands, Alaska, Wetlands, 28, 92–106, https://doi.org/10.1672/07-71.1, 2008.
Reeve, A. S., Tyczka, Z. D., Comas, X., and Slater, L. D.: The Influence of Permeable Mineral Lenses on Peatland Hydrology, in: Carbon Cycling in Northern Peatlands, Vol. 184, edited by: Baird, A., Belyea, L., Comas, X., Reeve, A., and Slater, L., 289–297, https://doi.org/10.1029/2008GM000825, 2009.
Ricciuto, D. M., Xu, X., Shi, X., Wang, Y., Song, X., Schadt, C. W., Griffiths, N. A., Mao, J., Warren, J. M., Thornton, P. E., Chanton, J., Keller, J. K., Bridgham, S. D., Gutknecht, J., Sebestyen, S. D., Finzi, A., Kolka, R., and Hanson, P. J.: An Integrative Model for Soil Biogeochemistry and Methane Processes: I. Model Structure and Sensitivity Analysis, J. Geophys. Res.-Biogeo., 126, e2019JG005468, https://doi.org/10.1029/2019JG005468, 2021.
Rissanen, A. J., Ojanen, P., Stenberg, L., Larmola, T., Anttila, J., Tuominen, S., Minkkinen, K., Koskinen, M., and Mäkipää, R.: Vegetation impacts ditch methane emissions from boreal forestry-drained peatlands – Moss-free ditches have an order-of-magnitude higher emissions than moss-covered ditches, Front. Environ. Sci., 11, 1121969, https://doi.org/10.3389/fenvs.2023.1121969, 2023.
Ross, A. C., Mendoza, M. M., Drenkhan, F., Montoya, N., Baiker, J. R., Mackay, J. D., Hannah, D. M., and Buytaert, W.: Seasonal water storage and release dynamics of bofedal wetlands in the Central Andes, Hydrol. Process., 37, e14940, https://doi.org/10.1002/hyp.14940, 2023.
Rubel, F., Brugger, K., Haslinger, K., and Auer, I.: The climate of the European Alps: Shift of very high resolution Köppen-Geiger climate zones 1800–2100, Meteorol. Z., 26, 115–125, https://doi.org/10.1127/metz/2016/0816, 2017.
Shao, S., Wu, J., He, H., Moore, T. R., Bubier, J., Larmola, T., Juutinen, S., and Roulet, N. T.: Ericoid mycorrhizal fungi mediate the response of ombrotrophic peatlands to fertilization: a modeling study, New Phytol., 238, 80–95, https://doi.org/10.1111/nph.18555, 2022a.
Shao, S., Wu, J., He, H., and Roulet, N.: Integrating McGill Wetland Model (MWM) with peat cohort tracking and microbial controls, Sci. Total Environ., 806, 151223, https://doi.org/10.1016/j.scitotenv.2021.151223, 2022b.
Shen, C., Chen, X., and Laloy, E.: Editorial: Broadening the Use of Machine Learning in Hydrology, Front. Water, 3, 681023, https://doi.org/10.3389/frwa.2021.681023, 2021.
Šimůnek, J., Brunetti, G., Jacques, D., Van Genuchten, M. Th., and Šejna, M.: Developments and applications of the HYDRUS computer software packages since 2016, Vadose Zone J., e20310, https://doi.org/10.1002/vzj2.20310, 2024.
Smith, B., Prentice, I. C., and Sykes, M. T.: Representation of vegetation dynamics in the modelling of terrestrial ecosystems: comparing two contrasting approaches within European climate space, Global Ecol. Biogeogr., 10, 621–637, https://doi.org/10.1046/j.1466-822X.2001.t01-1-00256.x, 2001.
St-Hilaire, F., Wu, J., Roulet, N. T., Frolking, S., Lafleur, P. M., Humphreys, E. R., and Arora, V.: McGill wetland model: evaluation of a peatland carbon simulator developed for global assessments, Biogeosciences, 7, 3517–3530, https://doi.org/10.5194/bg-7-3517-2010, 2010.
Strack, M., Davidson, S. J., Hirano, T., and Dunn, C.: The Potential of Peatlands as Nature-Based Climate Solutions, Curr. Clim. Change Rep., 8, 71–82, https://doi.org/10.1007/s40641-022-00183-9, 2022.
Sulman, B. N., Desai, A. R., Schroeder, N. M., Ricciuto, D., Barr, A., Richardson, A. D., Flanagan, L. B., Lafleur, P. M., Tian, H., Chen, G., Grant, R. F., Poulter, B., Verbeeck, H., Ciais, P., Ringeval, B., Baker, I. T., Schaefer, K., Luo, Y., and Weng, E.: Impact of hydrological variations on modeling of peatland CO2 fluxes: Results from the North American Carbon Program site synthesis, J. Geophys. Res.-Biogeo., 117, G01031, https://doi.org/10.1029/2011JG001862, 2012.
Sun, G. and Mu, M.: Role of hydrological parameters in the uncertainty in modeled soil organic carbon using a coupled water-carbon cycle model, Ecol. Complex., 50, 100986, https://doi.org/10.1016/j.ecocom.2022.100986, 2022.
Sun, G., Mu, M., and You, Q.: Identification of Key Physical Processes and Improvements for Simulating and Predicting Net Primary Production Over the Tibetan Plateau, J. Geophys. Res.-Atmos., 125, e2020JD033128, https://doi.org/10.1029/2020JD033128, 2020.
Sutton, O. F. and Price, J. S.: Projecting the hydrochemical trajectory of a constructed fen watershed: Implications for long-term wetland function, Sci. Total Environ., 847, 157543–157543, https://doi.org/10.1016/j.scitotenv.2022.157543, 2022.
Swails, E. E., Ardón, M., Krauss, K. W., Peralta, A. L., Emanuel, R. E., Helton, A. M., Morse, J. L., Gutenberg, L., Cormier, N., Shoch, D., Settlemyer, S., Soderholm, E., Boutin, B. P., Peoples, C., and Ward, S.: Response of soil respiration to changes in soil temperature and water table level in drained and restored peatlands of the southeastern United States, Carbon Balance Manage., 17, 18, https://doi.org/10.1186/s13021-022-00219-5, 2022.
Tang, J., Yurova, A. Y., Schurgers, G., Miller, P. A., Olin, S., Smith, B., Siewert, M. B., Olefeldt, D., Pilesjo, P., and Poska, A.: Drivers of dissolved organic carbon export in a subarctic catchment: Importance of microbial decomposition, sorption-desorption, peatland and lateral flow, Sci. Total Environ., 622, 260–274, https://doi.org/10.1016/j.scitotenv.2017.11.252, 2018.
Tarnocai, C. and Stolbovoy, V.: Chapter 2 Northern Peatlands: their characteristics, development and sensitivity to climate change, in: Developments in Earth Surface Processes, vol. 9, Elsevier, 17–51, https://doi.org/10.1016/S0928-2025(06)09002-X, 2006.
Treat, C. C., Jones, M. C., Alder, J., Sannel, A. B. K., Camill, P., and Frolking, S.: Predicted Vulnerability of Carbon in Permafrost Peatlands With Future Climate Change and Permafrost Thaw in Western Canada, J. Geophys. Res.-Biogeo., 126, e2020JG005872, https://doi.org/10.1029/2020JG005872, 2021.
van der Snoek, M., André, L., and Field, C.: Report on model and tool comparison and improvements, Interreg Care-Peat, Mechelen, Belgium, https://vb.nweurope.eu/media/20125/d414_deliverable_report_interreg_care-peat.pdf (last access: 24 June 2024), 2023.
Waddington, J. M., Morris, P. J., Kettridge, N., Granath, G., and Thompson, D. K.: Hydrological feedbacks in northern peatlands, Ecohydrology, 8, 113–127, https://doi.org/10.1002/eco.1493, 2014.
Walter, B., Heimann, M., Shannon, R., and White, J.: A process-based model to derive methane emissions from natural wetlands, Geophys. Res. Lett., 23, 3731–3734, https://doi.org/10.1029/96GL03577, 1996.
Wania, R., Ross, I., and Prentice, I. C.: Implementation and evaluation of a new methane model within a dynamic global vegetation model: LPJ-WHyMe v1.3.1, Geosci. Model Dev., 3, 565–584, https://doi.org/10.5194/gmd-3-565-2010, 2010.
Webster, K. L., McLaughlin, J. W., Kim, Y., Packalen, M. S., and Li, C. S.: Modelling carbon dynamics and response to environmental change along a boreal fen nutrient gradient, Ecol. Model., 248, 148–164, https://doi.org/10.1016/j.ecolmodel.2012.10.004, 2013.
Wilson, D., Mackin, F., Tuovinen, J.-P., Moser, G., Farrell, C., and Renou-Wilson, F.: Carbon and climate implications of rewetting a raised bog in Ireland, Global Change Biol., 28, 6349–6365, https://doi.org/10.1111/gcb.16359, 2022.
Wu, J. and Roulet, N. T.: Climate change reduces the capacity of northern peatlands to absorb the atmospheric carbon dioxide: The different responses of bogs and fens, Global Biogeochem. Cy., 28, 1005–1024, https://doi.org/10.1002/2014GB004845, 2014.
Xu, J., Morris, P. J., Liu, J., Ledesma, J. L. J., and Holden, J.: Increased Dissolved Organic Carbon Concentrations in Peat-Fed UK Water Supplies Under Future Climate and Sulfate Deposition Scenarios, Water Resour. Res., 56, e2019WR025592, https://doi.org/10.1029/2019WR025592, 2020.
Yang, H., Chae, J., Yang, A.-R., Suwignyo, R. A., and Choi, E.: Trends of Peatland Research Based on Topic Modeling: Toward Sustainable Management under Climate Change, Forests, 14, 1818, https://doi.org/10.3390/f14091818, 2023.
Yao, Y., Joetzjer, E., Ciais, P., Viovy, N., Cresto Aleina, F., Chave, J., Sack, L., Bartlett, M., Meir, P., Fisher, R., and Luyssaert, S.: Forest fluxes and mortality response to drought: model description (ORCHIDEE-CAN-NHA r7236) and evaluation at the Caxiuanã drought experiment, Geosci. Model Dev., 15, 7809–7833, https://doi.org/10.5194/gmd-15-7809-2022, 2022.
Young, D. M., Baird, A. J., Morris, P. J., and Holden, J.: Simulating the long-term impacts of drainage and restoration on the ecohydrology of peatlands, Water Resour. Res., 53, 6510–6522, https://doi.org/10.1002/2016WR019898, 2017.
Young, D. M., Baird, A. J., Charman, D. J., Evans, C. D., Gallego-Sala, A. V., Gill, P. J., Hughes, P. D. M., Morris, P. J., and Swindles, G. T.: Misinterpreting carbon accumulation rates in records from near-surface peat, Sci. Rep.-UK, 9, 17939, https://doi.org/10.1038/s41598-019-53879-8, 2019.
Yuan, F., Wang, Y., Ricciuto, D., Shi, X., Yuan, F., Brehme, T., Bridgham, S., Keller, J., Warren, J., Griffiths, N., Sebestyen, S., Hanson, P., Thornton, P., and Xu, X.: Hydrological feedbacks on peatland CH4 emission under warming and elevated CO2: A modeling study, J. Hydrol., 603, 127137, https://doi.org/10.1016/j.jhydrol.2021.127137, 2021.
Zak, D. and McInnes, R. J.: A call for refining the peatland restoration strategy in Europe, J. Appl. Ecol., 59, 2698–2704, https://doi.org/10.1111/1365-2664.14261, 2022.
Zi, T., Kumar, M., Kiely, G., Lewis, C., and Albertson, J.: Simulating the spatio-temporal dynamics of soil erosion, deposition, and yield using a coupled sediment dynamics and 3D distributed hydrologic model, Environ. Modell. Softw., 83, 310–325, https://doi.org/10.1016/j.envsoft.2016.06.004, 2016.
- Article
(2408 KB) - Full-text XML