the Creative Commons Attribution 4.0 License.
the Creative Commons Attribution 4.0 License.
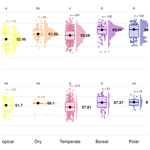
Leaf habit drives leaf nutrient resorption globally alongside nutrient availability and climate
Gabriela Sophia
Silvia Caldararu
Benjamin David Stocker
Sönke Zaehle
Nutrient resorption from senescing leaves can significantly affect ecosystem nutrient cycling, making it an essential process to better understand long-term plant productivity under environmental change that affects the balance between nutrient availability and demand. Although it is known that nutrient resorption rates vary strongly between different species and across environmental gradients, the underlying driving factors are insufficiently quantified. Here, we present an analysis of globally distributed observations of leaf nutrient resorption to investigate the factors driving resorption efficiencies for nitrogen (NRE) and phosphorus (PRE). Our results show that leaf structure and habit, together with indicators of nutrient availability, are the two most important factors driving spatial variation in NRE. Overall, we find higher NRE in deciduous plants (65.2 % ± 12.4 %, n=400) than in evergreen plants (57.9 % ± 11.4 %, n=551), likely associated with a higher share of metabolic N in leaves of deciduous plants. Tropical regions show the lowest resorption for N (NRE: 52.4 % ± 12.1 %), and tundra ecosystems in polar regions show the highest (NRE: 69.6 % ± 12.8 %). At the same time, the PRE is lowest in temperate regions (57.8 % ± 13.6 %) and highest in boreal regions (67.3 % ± 13.6 %). Soil clay content, N and P atmospheric deposition (globally available proxies for soil fertility), and mean annual precipitation (MAP) play an important role in this pattern. The statistical relationships developed in this analysis indicate the important role of leaf habit and type for nutrient cycling and guide improved representations of plant-internal nutrient recycling and nutrient conservation strategies in vegetation models.
- Article
(6294 KB) - Full-text XML
- BibTeX
- EndNote
Nutrient cycling plays an important role in shaping the global distribution of terrestrial primary productivity (LeBauer et al., 2008; Zaehle, 2013; Du et al., 2020). Nitrogen (N) and phosphorus (P) are the main limiting nutrients for plant growth. N is needed to maintain and produce essential proteins for the biosynthesis, while P is an element of genetic material and plays a major role in the regeneration of the main receptor of carbon (C) assimilation and in the production of energy that conducts many processes in living cells (Chapin, 1980; Güsewell, 2004). The anthropogenic increase in atmospheric CO2 since the beginning of industrialization has the potential to enhance the terrestrial carbon sink through increasing plant photosynthetic rates, a process known as CO2 fertilization (Bazzaz, 1990). A potential limitation to the fertilization effect is progressive nutrient limitation to growth (Luo et al., 2004) and associated plant strategies to deal with such limitations. Thus, understanding the ways in which nutrients circulate in ecosystems and are acquired, lost and conserved by plants is essential for simulating plant response to global changes.
Nutrient resorption – defined here as the translocation of nutrients from senescing leaves to temporary storage tissues – is a plant strategy for nutrient conservation (Killingbeck, 1996; Kobe et al., 2005). It allows plants to directly reuse nutrients, decreasing the dependence on soil nutrient availability and the competition for these nutrients with other plants and microbes, especially in nutrient-limited environments (Aerts, 1996; Aerts and Chapin, 1999). The question that arises is then why do plants not all resorb the entirety of leaf nutrients to be more efficient. The fact that they do not achieve their maximum resorption capacity implies the existence of costs and limitations to resorption. A quantitative understanding of nutrient resorption can yield insights into plant strategies to cope with nutrient limitation (Aerts and Chapin, 1999; Chapin et al., 2011). This is because the resorption process influences most other ecosystem processes that determine plant growth, as it directly affects litter quality and therefore soil organic matter decomposition and has indirect consequences for plant nutrient uptake, carbon cycling and finally plant competition (Killingbeck, 1996; Berg and McClaugherty, 2014). The average fraction of leaf nutrients resorbed before abscission is estimated to be ∼ 62 % for N and ∼ 65 % for P (Vergutz et al., 2012). Cleveland et al. (2013) estimated that this corresponds to 31 % of a plant's annual demand for N and 40 % of the annual demand for P but with large geographical and species variations.
However, despite advances in recent years, the drivers behind nutrient resorption and its variation are still unclear: first, soil fertility has long been assumed to be a key driver of variations in nutrient resorption, with increased resorption in infertile soils as the plant's main strategy for nutrient conservation (Aerts and Chapin, 1999). This interpretation has also provided a basis for modelling dynamic resorption efficiency by accounting for nutrient availability in global vegetation models (Fisher et al., 2010; Lawrence et al., 2019). Nonetheless, there is diverging evidence established at different geographic scales, showing positive correlations (Aerts and Chapin, 1999), negative correlations (Yuan and Chen, 2015; Xu et al., 2021), and even a lack of correlation between soil fertility and resorption efficiency (Vergutz et al., 2012). Second, climate factors are also considered to be important drivers of resorption, but the evidence is equally conflicting: on the one hand, Yuan and Chen (2009) and Yan et al. (2018) suggested nitrogen resorption efficiency (NRE) is decreasing with mean annual temperature (MAT) and precipitation (MAP), with the opposite trend for phosphorus resorption efficiency (PRE), arguing that colder regions tend to be more N-limited, while P limitation is observed more commonly in warmer environments. From low to high latitudes globally, the role of N in limiting productivity tends to increase as the availability of N is mainly determined by temperature-limited processes such as biological N fixation and mineralization of soil organic matter (Cleveland et al., 2013; Fay et al., 2015; Deng et al., 2018), but the presence of N fixers in tropical forests introduces complexity to the pattern of nutrient limitation between tropical and temperate zones (Hedin et al., 2009). Nevertheless, the limited availability of P in the tropics due to highly weathered soils distinguishes low- to mid-latitude environments (Elser et al., 2007). On the other hand, Vergutz et al. (2012) and Xu et al. (2021) showed that NRE and PRE are both increasing with decreasing MAT and MAP toward higher latitudes.
A third set of studies suggests plant functional types (PFTs), leaf stoichiometry and plant nutrient demand as drivers of nutrient resorption (Reed et al., 2012; Han et al., 2013; Tang et al., 2013; Brant and Chen, 2015; Du et al., 2020; Chen et al., 2021; Sun et al., 2023). When greater nutrient resorption in evergreen species is found, it is assumed to be a conservation strategy, given their comparatively low leaf nutrient content, slow growth rate and predominant occurrence in nutrient-limited biomes (Killingbeck, 1996; Yan et al., 2018; Xu et al., 2021). The same argument has been used to interpret differences between broad-leaved and needle-leaved plants, in which nutrient resorption is generally observed to be higher in needle-leaved as a strategy to acclimatize and survive in resource-limited environments (Aerts and Chapin, 1999; Yuan et al., 2005; Yan et al., 2018; Xu et al., 2021). Previous studies have suggested that shrub species generally display higher nutrient resorption rates compared to trees, due to their smaller leaves with shorter life cycles and the need to optimize nutrient use in resource-limited environments (Killingbeck, 1996; Yuan and Chen, 2009; Yan et al., 2018; Xu et al., 2021). However, Brant and Chen (2015) suggest that deciduous plants are more dependent on nutrient resorption as their investment in green leaf nutrients is higher to maintain their fast growth through high physiological activity during the growing season. Plants with a slow growth strategy, such as evergreens and needle-leaved trees, have lower photosynthetic nutrient use efficiency due to a higher allocation of C and N to leaf structural rather than metabolic compounds (Reich and Flores-Moreno, 2017). Onoda et al. (2017) empirically support this by showing that a greater allocation of nutrients to structural compounds is associated with decreased specific leaf area (SLA) and increased diffusive limitation to photosynthesis. Thus, variations in leaf traits and construction costs could contribute to differences in resorption between PFTs. Nevertheless, Drenovsky et al. (2010, 2019) suggested that resorption variability is influenced by an interplay of the discussed drivers, which include soil properties, climatic conditions and plant characteristics. Estiarte et al. (2023) support that leaf biochemistry of plants determines the first limitation to nutrient resorption, with a secondary regulation in resorption by environmental conditions, while the costs of leaf ageing remain consistent.
The divergence of observed patterns highlights the need for further investigation into the main drivers of variations in nutrient resorption, distinguishing the influence of plant types, soil and climatic conditions. In this study, we present a meta-analysis that combines version 5.0 of the TRY Plant Trait Database (Kattge et al., 2020) with different ancillary datasets for climate and soil factors to investigate global patterns of resorption efficiencies for N and P. We aim to extend woody species observations for nutrient resorption and investigate the factors that explain observed patterns along three main axes: climate, soil fertility and leaf properties.
2.1 Data collection
We assembled the dataset from the TRY Plant Trait Database (https://www.try-db.org, last access: 14 March 2022, Kattge et al., 2020, version 5.0) containing field measurements of paired leaf and litter mass-based tissue N and P concentrations (Nmass, leaf, Pmass, leaf, Nmass, litter, Pmass, litter) to derive the fractional nutrient resorption (described in Sect. 2.2) and plant functional traits recorded in parallel from the same species and same location to consider as biological predictors variables (Table 1). As additional predictors of nutrient resorption, we combined it with climate and soil input data (Table 2). We processed the data using R statistical software (version 4.0.4), keeping the data at species level. To manipulate the extracted functional traits, we used the package {rtry} (Lam et al., 2022), developed to support the preprocessing of the TRY Plant Trait Database (version 1.0.0), and the {tidyverse} package (Wickham et al., 2019) with its dependencies (version 1.3.2). The data processing followed the quality control according to the published protocol of TRY (Kattge et al., 2011, 2020).
As predictors, we used a set of climate variables, N and P deposition, vegetation type-related variables, and soil data (Table 2) with a spatial resolution of 0.5° × 0.5° to match those of the lowest-resolution dataset (P deposition). Soil fertility was represented here by N and P deposition and other soil characteristics that globally correlate with nutrient availability, such as total soil P and soil texture. MAT, MAP and seasonal temperature amplitude were derived from the global climate database WorldClim (Fick and Hijmans, 2017). We extracted the Köppen climate classification to represent different climate zones from the TRY Plant Trait Database and filled data gaps using the {Kgc} R package (Bryant et al., 2017), which provides the Köppen climate classification for each latitude and longitude. We calculated mean annual evapotranspiration (ET) and growing season length (GSL) from FLUXCOM (Jung et al., 2011), in which GSL was based on the seasonal phasing of gross primary productivity (GPP) considering the time period between 20 % and 80 % of the maximum GPP in an average year for the period 2002–2015. Total soil P concentrations were derived from Yang et al. (2013); soil clay content and soil pH were extracted from the Harmonized World Soil Database (HWSD; Wieder et al., 2014). We used atmospheric N deposition values from CESM-CMIP6 (Hegglin et al., 2016) taking the year 2010 as a reference, summing the emissions and making the annual mean; P deposition was extracted from Brahney et al. (2015) and Chien et al. (2016). The N deposition data are interpolated to annual from decadal time slices and derived from initialized CAM runs; therefore, the information contained is representative of large-scale features. For consistency with P deposition, where we only have a decadal mean estimate, we chose not to include the trend information. All variables used as predictors of global N and P resorption are described in Table 2.
2.2 Data derivation
We defined nutrient resorption efficiency (NuRE) as the amount of nutrient resorbed during leaf senescence calculated as
where Nugreen and Nusenesced are nutrient (N or P) concentrations in dry green and senesced leaves (mg g), respectively; MLCF (unitless) is the mass loss correction factor during senescence to account for the loss of leaf mass when senescence occurs. Omitting MLCF overestimates nutrient concentration in senescent leaves and underestimates resorption values (Zhang et al., 2022). Zhang et al. (2022) showed a significant overall improvement when considering MLCF, where both averages of N and P resorption increased by ∼ 9 %, particularly for cases with low resorption efficiencies. In the present study, not considering the MLCF also underestimates the actual nutrient resorption efficiency when comparing the fraction of resorption of four subdatasets from the final global dataset (Appendix A).
We calculated MLCF as the ratio between the dry mass of senesced and green leaves (Van Heerwaarden et al., 2003a), where it was not directly available as percentage leaf mass loss (LML) in the data. We derived average values of MLCF per plant type from the nutrient resorption dataset to fill missing values: 0.712 for deciduous, 0.766 for evergreen, 0.69 for conifers and 0.75 for woody lianas, respectively. To fill in MLCF values for the remaining leaf nutrient and litter data from TRY, we associated these means of MLCF with leaf habit, leaf type and growth form information available on each species. For that, evergreen needle-leaved trees were associated with conifers MLCF, deciduous trees and/or shrubs with deciduous woody MLCF and evergreen trees and/or shrubs with evergreen woody MLCF, respectively. We grouped climbers and lianas with shrubs. Initially, 107 observations for NRE and 76 observations for PRE were derived from site-level MLCF data. We increased these numbers by 847 for NRE and 378 for PRE when applying the mean MLCF per PFT. In total we extracted data from 131 sites for NRE and 74 for PRE (Fig. 1), with more than one entry per site, giving a total of 954 and 454 data points for NRE and PRE species level, respectively. Temperate biomes were most strongly represented in the dataset (518 entries), followed by tropical (180), boreal (103), polar (102) and dry ecosystems (65).
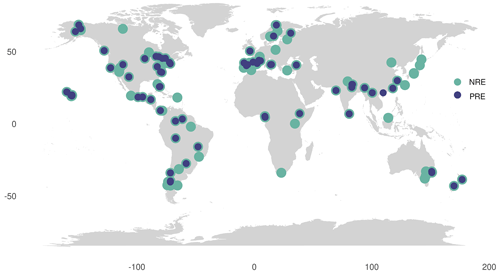
Figure 1Global distribution of data for nitrogen resorption efficiency (NRE) and phosphorus resorption efficiency (PRE). Data include observations from 131 sites for NRE (green circles) and 74 sites for PRE (blue circles). Each site may have multiple entries, resulting in a total of 954 NRE data points and 454 PRE data points at the species level.
2.3 Statistical analysis
As the nutrient resorption data did not conform to a normal distribution (Shapiro–Wilk test), we used the nonparametric Kruskal–Wallis one-way analysis of variance (ANOVA) to examine differences in NRE and PRE among different climate zones and the Mann–Whitney–Wilcoxon test to evaluate differences between leaf habit, leaf type and growth form (deciduous vs. evergreen plants, broad-leaved vs. needle-leaved, shrubs vs. trees), using the {ggstatsplot} R package (Patil, 2021). We applied Pearson correlation and linear regression to analyse the relationship between nutrient resorption and the predictors described in Table 2. For MAP and N deposition, we performed a log transformation prior to conducting the analysis to have the distribution close to the normal. To find the best set of predictors of the variance in NRE and PRE, we used multimodel inference (MMI; Burnham and Anderson, 2002) using Akaike's information criterion (AIC) and estimated the relative importance (RI) of each explanatory variable. Different from setting only a single model based on AIC, multimodel inference accounts for uncertainties in the model performance and in the considered parameters. This approach involves modelling and evaluating all possible combinations of a predetermined set of predictors. The evaluation is typically conducted using a criterion, such as AIC or the Bayesian information criterion (BIC), which favours simpler models and allows for a comprehensive examination of all possible models and their respective performances. By synthesizing the estimated coefficients of predictors across these models, MMI enables inference regarding the overall importance of specific predictors. Before applying MMI, we used generalized linear mixed-effect models to fit different models after removing drivers described in Table 2 that showed the following: (1) high collinearity between them (R≥0.7; Fig. S5), (2) non-significant correlation with NRE (soil P) and PRE (MAP and SLA) (Fig. S5), and (3) a threshold of variance inflation factor (VIF) higher than 10 (James et al., 2013). Specifically, temperature amplitude, GSL and ET were not considered due to their high correlation with MAT and MAP and due to high VIF. Based on ecological interactions, we fitted the model considering interactions between climate variables MAT and MAP, as well as between plant characteristics such as leaf structure, leaf habit and leaf type (SLA : LeafHabit : LeafType). We accounted for species identity as a random factor in the mixed-effect models to test whether intrinsic intra-specific variability plays a role. Environmental and biotic factors have strong shared effects in linear mixed models and therefore are not assessed separately in this study. If the ratio between the sample size and the number of parameters considered was higher than 40, we fitted the model using restricted maximum likelihood (REML) and AICc (corrected for small sample sizes) to avoid bias. We selected the model with the lowest AIC and applied it to the “dredge” function implemented in the multimodal inference package {MuMIn} (Bartoń K, 2023), which generated a full submodel set. A set of best-performing models for NRE and PRE was selected using a cut-off of ΔAIC <2, and based on these top models, the best model parameters were generated. Using the {MuMIn} package, we also calculated the relative importance of each predictor through the sum of the Akaike weights across all models in which the respective parameter was being considered, with a cut-off of 0.8 to distinguish between important and unimportant predictors (Terrer et al., 2016). The marginal and conditional R2 values for the fitted mixed models were 0.23 and 0.98 for NRE and 0.29 and 0.48 for PRE respectively. Therefore, fixed and random effects explain 98 % of the variance in NRE and 48 % in PRE, with fixed effects alone explaining 23 % for NRE and 29 % for PRE. We performed all statistical analyses using the p value <0.05 as statistically significant.
3.1 Global patterns of nutrient resorption between different climate zones
The global median of nutrient resorption is 60.0 % for N ± 12.3 % of standard deviation (n=954) and 61.2 % for P ± 13.6 % (n=454), respectively. We find differences for both NRE and PRE between the climate zones (Fig. 2). Tropical regions show the lowest resorption for N (NRE: 52.4 % ± 12.1 %), and tundra ecosystems in polar regions show the highest (NRE: 69.6 % ± 12.8 %) (Fig. 2a). PRE in temperate regions shows the lowest values (57.8 % ± 13.6 %). PRE increases towards higher latitudes with a significant difference in P resorption from temperate to boreal regions (67.3 % ± 13.6 %) (Fig. 2b). In contrast to NRE, the difference in PRE between tropical and other climate zones, as well as polar regions, is not statistically significant (P>0.05). NRE in dry regions (61.6 % ± 9.7 %) is statistically different from tropical and polar regions, while for PRE, the difference is not significant between climate zones. However, the sample for this zone is substantially smaller. Details of the minimum, maximum and median values can be found in Table B1.
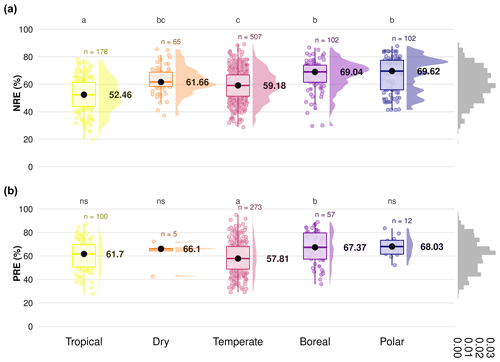
Figure 2Difference in nitrogen resorption efficiency (NRE %) and phosphorus resorption efficiency (PRE %) among climate gradients from tropical to polar zones based on the Köppen climate classification. Panels display NRE (a) and PRE (b), with boxplots showing the median (black dots), interquartile range and outliers, indicating data spread and variability. The side distributions show the overall data distribution for each climate zone. Different letters indicate statistically significant differences in nutrient resorption efficiency between climate zones. “ns” indicates no significant difference. “n” represents the number of observations per climate zone. The grey distribution on the right of each panel represents the overall distribution of NRE and PRE values across all observations.
3.2 Patterns of nutrient resorption between plant functional types
We explore the variation of nutrient resorption between plant functional groups. Deciduous woody plants have a significantly higher NRE (65.2 % ± 12.4 %, n=400) than evergreens (57.9 % ± 11.4 %, n=551) (P<0.001) (Fig. 3a), and shrubs have a significantly higher NRE (63.1 % ± 12.4 %, n=230) than trees (59.2 % ± 12.1 %, n=724) (P<0.001) (Fig. 3c). Conversely, there is no significant difference in NRE between broad-leaved (59.8 % ± 12.5 %, n=841) and needle-leaved plants (61.8 % ± 9.9 %, n=103) (P>0.05) (Fig. 3b). PRE does not differ significantly between deciduous (60.0 % ± 12.8 %, n=220) and evergreen plants (61.7 % ± 14.4 %, n=231) (P=0.4) (Fig. 3d) or between shrubs (64.4 % ± 13.5 %, n=59) and trees (61.1 % ± 13.6 %, n=395) (P=0.2) (Fig. 3f). However, PRE differs significantly between leaf types, with needle-leaved plants showing higher resorption (72.2 % ± 9.2 %, n=45) than broad-leaved plants (59.6 % ± 13.5 %, n=404) (P<0.001) (Fig. 3e). Details of the minimum, maximum and median values can be found in Table B2.
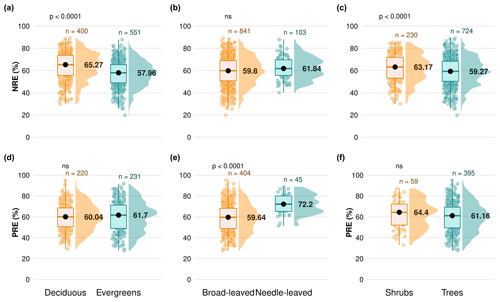
Figure 3Differences in nitrogen resorption efficiency (NRE %) and phosphorus resorption efficiency (PRE %) between plant functional types (PFTs) on a global scale. Panels display NRE (a, b, c) and PRE (d, e, f) for different PFT comparisons: deciduous vs. evergreen species (a, d), broad-leaved vs. needle-leaved species (b, e) and shrubs vs. trees (c, f). Boxplots depict median (black dots), interquartile range and outliers, indicating data spread and variability. The side distributions show the overall data distribution for each PFT. “n” represents the number of observations. p values indicate the significance of differences in nutrient resorption efficiency between PFTs. “ns” indicates no significant difference.
We next explore how climate zones affect NRE and PRE within plant functional groups. NRE tends to increase from tropical to boreal climates (Fig. 4a) – a pattern seen among deciduous and evergreen woody plants, among shrubs and trees, and among broad-leaved but not needle-leaved plants. Also, PRE increases from temperate to boreal and polar climates but declines from the tropics to temperate climates in evergreens (Fig. 4b). Apart from the overall tendency, we observe a few statistical deviations from the general pattern that emerges across all plants pooled: NRE is significantly lower in polar regions compared to boreal forests for evergreens (NRE: 56.0 % ± 13.4 %; NRE: 70.5 % ± 10.8 %) and compared to needle-leaved plants (NRE: 56.0 % ± 11.5 %; NRE: 51.5 % ± 7.3 %) (P<0.001); PRE shows the same pattern deviation between these regions, but the pattern is not statistically significant (P>0.05). Also, we do not observe lower NRE for tropical regions in needle-leaved plants because the only observation of this plant type is in this climate zone. Details of the minimum, maximum and median values can be found in Table B3.
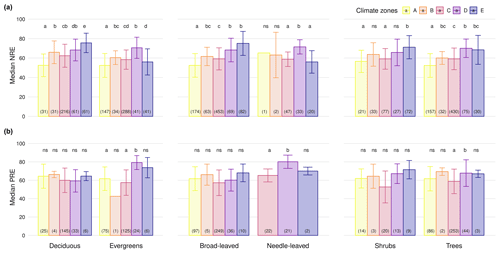
Figure 4Median nitrogen resorption efficiency (NRE %) and phosphorus resorption efficiency (PRE %) across different plant functional types (PFTs) and climate zones. Panels display median NRE (a) and PRE (b) for the following PFTs: deciduous vs. evergreen species, broad-leaved vs. needle-leaved species and shrubs vs. trees. Each bar represents a climate zone (A: tropical; B: dry; C: temperate; D: boreal; E: polar) based on the Köppen classification, with colour-coded legends. Error bars indicate variability. Numbers in parentheses denote the number of observations, and letters above bars indicate statistically significant differences between climate zones within each PFT. “ns” indicates no significant difference).
3.3 Main drivers of nutrient resorption
We investigate the main drivers of variation in nutrient resorption, considering biological, climatic and soil factors and using data from all PFTs and climate zones pooled. Dredge model averaging based on a set of best-performing models with corrected AIC (see Sect. 2.3) shows that the best model for NRE includes soil clay content, N deposition, MAP and growth form (Table 3). The best combination of predictors of the PRE model includes N deposition, leaf type and MAT (Table 3). Sums of Akaike weights indicate that the order of importance of predictors of NRE is N deposition (RI 0.99), MAP (RI 0.99) and leaf habit (RI 0.98), followed by soil clay content (RI 0.97), growth form (RI 0.93) and leaf type (RI 0.87) (Fig. 5a). For PRE, the order is P deposition (RI 0.99), leaf type (RI 0.99) and N deposition (RI 0.94), followed by leaf habit (RI 0.89) (Fig. 5b). The criteria to fit the model selecting and/or excluding predictors and interactions for the multimodel inference can be found in Sect. 2.3. Correlations between all variables, as well as linear relationships to the regression slope between nutrient resorption and all possible predictors, can be found in Figs. C1, C2 and C3.
Through an extensive global dataset of leaf nutrient resorption and a multifactorial analysis, we show that leaf habit and type are a strong driver of the spatial variation in nutrient resorption, with thicker, longer-lived leaves having lower resorption efficiencies. Climate and soil-availability-related factors also emerge as strong drivers, in which we discuss a secondary regulation related to environmental conditions in space and time. Our study covers significantly more woody species observations for nutrient resorption, especially for N, than previous studies (Yuan and Chen, 2009; Yan et al., 2018; Xu et al., 2021). We also account for variations in the mass loss of senescing leaves by deriving the MLCF when leaf mass loss or leaf dry mass was available and then apply the calculated average MLCF to the missing data rather than using a single average of MLCF from the literature per PFT (Yan et al., 2018; Xu et al., 2021), which may lead to a more correct estimate of nutrient resorption (see Sect. 2.2).
4.1 Nutrient resorption limited by leaf structure
The structural properties of leaves limit the efficiency of resorption along geographic and climatic ranges. We find that the global median for NRE is significantly higher in deciduous than evergreen plants and is higher in shrubs than trees (discussed at the end of this section) (Fig. 3a, c). This finding is in contrast to previous global studies that found decreasing nutrient resorption with increasing green leaf nutrient content, implying that deciduous species, which generally have higher leaf N content than evergreen species, have lower resorption (Yan et al., 2018; Xu et al., 2021). Nevertheless, our finding is in agreement with Vergutz et al. (2012), who reported that deciduous woody species had higher NRE than evergreen woody species and found no significant differences for PRE.
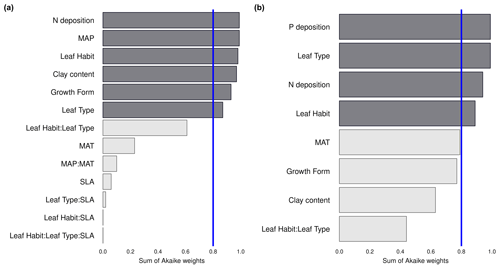
Figure 5Importance of the abiotic and biotic predictors on nitrogen resorption efficiency (NRE; a) and phosphorus resorption efficiency (PRE; b). The relative importance (RI) of each predictor is calculated through the sum of the Akaike weights derived from the multimodal inference selection, using corrected Akaike's information criteria. The blue line marks the threshold for important predictors (RI > 0.8). Interactions between predictors are denoted by colons. MAP: mean annual precipitation. MAT: mean annual temperature. SLA: specific leaf area.
We find that leaf habit is a strong driver of variation in resorption for both nutrients (Table 3; Fig. 5). Figure 3a shows that leaf habit is associated with clearly different median NRE values for evergreen and deciduous species, while the relationship of the average resorption is less clear for PRE (Fig. 3d). This is likely the consequence of a dominance of evergreen species in the tropics in our dataset, but we cannot conclude that the lower number of data for PRE is also a driver of this pattern. The inconsistencies of patterns and significance in P resorption can be related to high biochemical divergence in leaf P fractions compared to N, leading to varied mobilization paths (Estiarte et al., 2023). The breakdown of proteins is the main way N moves around, as 75 %–80 % of N is allocated in proteins, while P mobilization involves many different catabolic pathways that lead to a wider variety in P dynamics in leaves during leaf development (Estiarte et al., 2023).
We observe no statistical difference between leaf types for NRE (Fig. 3). The higher PRE in needle-leaved than broad-leaved plants (Fig. 3e) is likely a species effect since almost all needle-leaved plant observations for PRE are plants of the same family, Pinaceae. Nevertheless, leaf type is also a strong driver of variance in NRE and PRE (Table 3; Fig. 5). This finding goes together with the view of thicker, longer-lived leaves – such as evergreens and needle-leaved plants – having lower resorption efficiencies. One possible explanation for this global leaf habit and type pattern is that thicker leaves from evergreens plants, i.e. those with low SLA, have more N allocated to structural leaf compartments, which means it is harder to break down and resorb nutrients back, leading to less resorption. This is different to deciduous plants, in which leaves are characterized by a higher SLA and a larger N investment into metabolic compounds (Onoda et al., 2017). Although SLA is not directly selected in the statistical model, our results implicitly contain the effects of SLA on nutrient resorption through the strong and known relationship between SLA and leaf type and habit (Fig. C5).
The leaf economics spectrum (LES) distinguishes “fast” and “slow” economic strategies found globally and existing independent of climate (Wright et al., 2004). A rapid return on investments, or “fast” economic strategy, is typically associated with deciduous plants and achieved through a combination of traits such as shorter leaf longevity, higher nutrient concentrations and thinner leaves (high SLA), resulting in higher gas exchange rates per unit mass / area (Reich et al., 1992, 1997; Wright et al., 2004). Conversely, a slow return on investments is associated with the opposite set of traits and typically found in evergreen plants (Reich et al., 1992, 1997; Wright et al., 2004). The low SLA of long-lived leaves is associated with low photosynthetic N-use efficiency but with nutrient investment spread over a longer period. The low photosynthetic N-use efficiency can be attributed to a higher proportion of C and N being allocated to structural rather than metabolic components of the leaf (Reich et al., 2017), which aligns with the theory on leaf carbon optimization proposed by Kikuzawa (1995) and posits that shorter leaf longevity is associated with higher photosynthetic rates or lower costs of leaf construction.
Here, we find that plants with a conservative nutrient resorption strategy are located at the non-conservative end of the LES, that is, in the “fast” economic strategy. The discussion that revolves around the LES is determined by a combination of trade-offs between investments in structural and metabolic components, as well as trade-offs over time in the expected returns on those investments (Reich et al., 2017). The non-transferable and possibly transferable nutrients depend on where they are located in the cell and their biochemistry (Estiarte et al., 2023). Metabolic fractions are considered to be fully accessible for resorption, while structural fractions have been considered non-degradable (Estiarte et al., 2023). Wang et al. (2023) bring the worldwide pattern of high leaf lifespan (LLS) in plants with low SLA as a natural selection response to maximize carbon gain during leaf development, with variations in SLA in deciduous and evergreen species being determined by microclimate conditions. This pattern scales up from the organ level to a broader perspective that encompasses the trade-off between growth and survival at the plant level (Kikuzawa and Lechowicz, 2011). We find higher NRE in shrubs than trees, as observed in previous studies (Yuan and Chen, 2009; Yan et al., 2018; Xu et al., 2021), which is also reflected in the identification of plant growth form as one of the main driving factors of NRE in the multimodel inference analysis (Table 3; Fig. 5a). Compared to trees, shrubs typically have smaller leaves and shorter leaf lifespans. With that they need to be more resourceful with the nutrients available and prioritize nutrient resorption as a way to optimize nutrient usage for growth.
Resorption is an internal plant process that aims to maintain the balance of soil–plant interactions in the acquisition and conservation of nutrients, considering which process is less costly for the plant. The efficiency in nutrient use by plants is determined mainly by the nutrient residence time in the plant, which can be accessed through the leaf longevity maintaining the nutrients or through resorption before leaf abscission (Veneklaas, 2022). Our results support the concept that nutrient resorption is mainly driven by the share of metabolic vs. total leaf N and P, which co-varies with SLA (proxy for construction costs).
Therefore, higher resorption in deciduous trees may be an important conservation strategy as this process is less energetically costly than new growth. Brant and Chen (2015) discuss the dependence of deciduous trees on nutrient resorption efficiency, as their investment in green leaf nutrients is higher to keep fast physiological activity during growing season, or the entire nutrient economy is compromised. With that, we can argue that leaf longevity may be an important strategy for evergreen plants to conserve their lower leaf nutrient content, as the nutrient residence time is higher in evergreens. These plants retain nutrients for as long as possible, because once the nutrients are transferred to the soil through litterfall, they are partially lost from the system.
4.2 Effects of climate factors
Our global dataset shows that NRE significantly increases from tropical to polar zones (Fig. 2a), while PRE is lowest in temperate zones and significantly increases toward the poles (Fig. 2b). This suggests that the resorption of both nutrients is governed to some extent by a comparable dependency on climate, possibly related to slowed soil organic matter decomposition at lower temperatures, which reduces the net rate of mineralization and, in turn, limits the availability of nutrients for plant uptake from the soil (Sharma and Kumar, 2023). MAT emerges as one of the main drivers of PRE but not of NRE (Table 3). This result may be the outcome of the overall distribution of deciduous and evergreen species across climate zones, suggesting that global variations in N and P resorption along climatic gradients may arise primarily from global patterns in deciduous vs. evergreen and needle-leaved vs. broad-leaved plants. This statement is important in the context of projecting nutrient cycling under altered climate and indicates limited responses in resorption to temporal changes in climate at decadal timescales – before the global distribution of leaf habit and type changes as a result of shifts in species composition.
MAP emerges as an important driver of NRE (Table 3; Fig. 5). One explanation is that low MAP leads to low soil moisture, constraining nutrient mobility and increasing the carbon cost for plants to take up nutrients (Gill and Finzi, 2016). Therefore, together with limited N-resorption mobility in leaf tissues discussed above (Estiarte and Peñuelas, 2015), soil moisture constrains N mobilization during the mineralization process (Thamdrup, 2012). Liu et al. (2017) analysed the relation between soil N mineralization and temperature sensitivity on a global scale and showed largest N-mineralization rates at tropical latitudes and a general poleward decrease. We can observe a similar pattern of NRE with latitude (Fig. C4). Deng et al. (2018) observed a negative relationship between NRE and mineralization rate, which suggests a reciprocal causal relationship where systems emerge exhibiting both simultaneously low mineralization and high resorption rates. The strong link we find here between NRE and leaf habit and leaf type – traits that are immutable within a given species – indicates that the variations we observe in resorption might be a possible reflection of species composition, with a direct consequence for N cycling. It suggests that a positive feedback mechanism exists that leads ecosystems to be characterized by high resorption and a slower soil cycling or vice versa (Phillips et al., 2013). For example, species adapted to low soil N are favoured in N-limited environments, but they also produce low-N litter that decreases mineralization and further favours their competitiveness (Chapin et al., 2011).
In addition, we find a negative correlation between resorption and GSL (Figs. C1 and C2). Plant strategies in regions with short growing seasons (e.g. high latitudes or seasonally dry subtropical regions) are focused on nutrient conservation to maximize growth during the favourable period, despite nutrient availability. In very cold and seasonal environments, as seen in grassy tundra vegetation, soil nutrients are often not available concurrently with plant demand (Lacroix et al., 2022), implying that it may be more advantageous for plants to retain their nutrients. While we did not include GSL in the multimodel inference analysis due to its high collinearity with MAT, this aspect is partially reflected in leaf habit.
When we separate the global patterns for different climate zones and PFTs, our results show that the major climatic pattern is consistent across the growth forms and leaf types and leaf habit (Fig. 4), in which NRE and PRE increase towards higher latitudes and PRE shows a minimum at mid-latitudes. Our findings support that maximum NRE and PRE may be firstly constrained by leaf properties, with secondary effects from climate and soil texture (discussed below). Estiarte et al. (2023) suggest that a plant's leaf biochemistry (biochemical and subcellular fractions of N and P) is the primary factor in limiting nutrient resorption, followed by secondary regulation related to environmental conditions in space and time. They present that resorption efficiency declines when soil nutrient availability rises, as plant uptake becomes less costly in more fertile soil. However, the expenses linked to ageing leaves remain constant (Estiarte et al., 2023).
4.3 Effect of soil nutrient availability
N and P deposition and clay content emerge as important predictors of both PRE and NRE (Table 3; Fig. 5). This likely reflects the influence of soil N and P availability for NRE and PRE. Clay content is an important factor determining the nutrient retention capacity and cation exchange capacity in soils (Chapin et al., 2011). Chronic N deposition has increased soil N availability (Galloway et al., 2004) and leaf nutrient content (Chapin et al., 2011) over the 20th century and likely affected plant-internal recycling and resorption as indicated by our spatial results. In a fertilization experiment, higher P input had a negative effect on both NRE and PRE (Yuan and Chen, 2015), suggesting that increased P deposition may reduce the plant-internal recycling and thus resorption. The cycling and accessibility of soil P are influenced by N deposition (Marklein and Houlton, 2012) through various mechanisms, including changes in plant P-use strategies (Dalling et al., 2016; Wu et al., 2020a). Higher N deposition tends to reduce total soil P content (Sardans et al., 2016), so plants would need to increase PRE to compensate for the high soil N : P stoichiometry and P limitation. Jonard et al. (2015) suggested that forest ecosystems are becoming less efficient at recycling P due to excessive N input and climatic stress. This observation likely contributes to our finding that N and P depositions emerge as a stronger driver in a negative correlation with PRE (Figs. 5 and C2; Table 3). The lack of effect by total soil P on NRE and PRE may result from the fact that this variable does not represent the actual fraction of P available for plant uptake. Nevertheless, N deposition has a strong positive effect on NRE (Fig. 5; Table 3) – contrary to expectations (Aerts and Chapin, 1999; Yuan and Chen, 2015; Fisher et al., 2010). This indicates that the influence of N deposition might be via effects on SLA, whereby increasing N deposition increases the fraction of non-structurally bound N and therefore increases the fraction of N that can be resorbed. This effect, corrected for covariant factors such as leaf type and growth form, overlaps with the negative effect of soil clay content on NRE and PRE, which suggests that resorption decreases with nutrient availability in clay-rich soils. Our results raise an important point on the correlation of leaf nutrient resorption and nutrient limitation, showing that the relationships are complex and driven by multiple interacting and seemingly opposing factors.
Another soil factor we find to be important for nutrient resorption is the clay content (Table 3). Clay minerals are formed during soil weathering and have a high surface area that influences the soil's water retention capacity and a negative charge that enables nutrient retention and exchange with plant roots (Chapin et al., 2011). High-latitude soils that are younger and experience slow rates of chemical weathering usually have low clay content and, therefore, less potential for mineral nutrient storage, which may affect their availability for plant uptake (Chapin et al., 2011). As a result, plants in these environments need to invest more in resorption. Thus, together with MAP and MAT, soil clay content is also closely related to soil nutrient supply on a global scale, which is reflected in its role as driving resorption (Table 3; Fig. 5), as well as in the negative correlation between clay content and nutrient resorption (Figs. C1 and C2). The important effect of leaf properties on nutrient resorption, along with climate, soil texture and soil fertility (as previously suggested by Aerts and Chapin, 1999; Yuan and Chen, 2015; Xu et al., 2021), may indicate that biological and environmental factors are interconnected, as it is also influenced by multiple elements such as litter quality, precipitation, parent materials and soil texture. For example, P availability is geologically and pedologically limited in warm environments, which means it is mainly determined by soil parent materials (Augusto et al., 2017); therefore, soil texture becomes an important factor of P limitation in tropical regions. Also, the role of P deposition in relation to plant demand is high for tropical forests (Van Langenhove et al., 2020) but low worldwide (Cleveland et al., 2013). PRE in the tropics does not differ statistically from other climate zones, although we observe an increase of PRE from mid-latitudes to low latitudes (Figs. B1b and C4), which may indicate data limitation for PRE. The combination of plant properties with an underlying soil and climate control as driving factors of resorption variation is also supported by Drenovsky et al. (2010, 2019), who suggested a combination of soil properties, climatic factors and plant morphology to explain changes in nutrient resorption.
4.4 Data uncertainties and implications
Our study contributes to the existing research on nutrient resorption by using a comprehensive approach to deriving resorption values from the TRY Plant Trait Database. However, we encounter limitations in this derivation due to a lack or limited quality of data. The absence of co-located nutrient measurements in leaf and litter led to a shortage of suitable data pairs, mainly for PRE, in which the robustness of the model selection raised concerns about its reliability. In addition, it is not possible to assess the entire temporal aspect of data collection, which increases intraspecific variability. For NRE, 645 of a total of 954 observations are from the same growing season, as we have collection information for green leaves and litter samples whether they were picked from the plant, recently fallen or from litterfall traps cleared every week. Consequently, for approximately 30 % of the data, we cannot confirm that the leaf and litter measurements are from the same growing season and legitimately from the same individual. This is indeed one of the greatest limitations in assessing reliable nutrient resorption values. Nevertheless, it remains the accepted – and only – method for evaluating resorption on a broad scale.
While our approach of accounting for the MLCF improves estimates of resorption (Appendix A), we could not estimate the MLCF for all data pairs or fill all gaps using average functional type characteristics due to the lack of trait attributes in the TRY Plant Trait Database. These two factors reduce the number of data points available for statistical analysis using the multi-model inference. Furthermore, although we recognize the importance of leaf lifespan (LLS), it is not possible to analyse the relationship between resorption and LLS due to the few measurements of this functional trait. Nevertheless, applying the available statistical methods to analyse the drivers behind NRE and PRE, we find consistent patterns for the key gradients of climate, soil and PFTs, which are informative for other studies despite the remaining unexplained variance. In addition, we find that even within species of the same family, the distribution of NRE values is nearly as wide as the distribution for PFTs. This coordination in the observed spread likely reflects a substantial contribution from environmental variability, which would be interesting for further analysis if more data were available. In order to improve the depth of resorption investigation, we encourage researchers in fieldwork to perform concurrent measurements of litter nutrient content as well as leaf and litter dry mass.
The statistical analysis of dredge multi-model inference depends on the specific factors used in the analysis. We removed highly collinear variables and tested the impact of different combinations of factors. Although changing the factors affects the exact number of data points used in each multi-model inference, the overall identification of important and less important factors of NRE and PRE remains robust, especially for PFTs. However, ensuring that our analysis is as global as possible, the statistical dredge model analysis can consequently be influenced by temperate-region bias, which is an inherent limitation we cannot fully mitigate but one that is present in any global meta-analysis of this kind.
By quantifying these trends that we have found, we can delve deeper into ecosystem models by improving model parameterization and developing a dynamic nutrient resorption concept. Studies that utilize data to infer nutrient cycling frequently simplify resorption by making general assumptions (Finzi et al., 2007; Cleveland et al., 2013) or simply representing this process as a fixed value of 50 % (Vergutz et al., 2012; Zaehle et al., 2014), which may cause inaccuracies in their findings on nutrient cycling. The flow of recycling nutrients in land surface models is a factor that determines how strong the soil nutrient availability controls plant production. N resorption and N uptake in the FUN model (Fisher et al., 2010), for example, are defined by the relative acquisition cost of the two sources. Fisher et al. (2010) discuss that the cost of resorption assumes a constant based on global observations, but it may require a clearer connection to leaf physiology. Here, we provide a start for a statistical model that can connect resorption and plant properties and restrict how much plants could actually resorb nutrients, as well as the dataset to test the predictions of a physiological model. In addition, environmental drivers that have been shown to influence the overall patterns, such as soil texture and climate, could be considered to influence the resorption efficiency after primary leaf physiology limitation. Such information is essential when estimating how it can constrain carbon assimilation in the face of global changes (Galloway et al., 2008) and, therefore, essential to predict future plant growth and the capacity of the forest to act as a carbon sink (Thornton et al., 2007; Arora et al., 2022).
Our analysis of the global plant trait database indicates that variations of NRE and PRE are driven by the combination of plant properties with an additional soil and climate control. Systematic variations of NRE across leaf habit and type indicate that these traits are linked to plant nutrient use and conservation strategies and that leaf structure plays an important role in determining the proportion of nutrients that can be resorbed. Different metrics of soil fertility and soil-related variables influence NRE and PRE together with climatic variables and leaf structure and habit. Clay content, N and P deposition have a strong influence with a negative relationship – possibly an expression of its role in nutrient retention – as well as MAP. These trends provide a target to benchmark the simulation of nutrient recycling in global nutrient-enabled models. A focus on considering the links between leaf structure and nutrient resorption efficiency should enable a more realistic consideration of ecological and environmental controls on nutrient cycling and limitation than the current state of the art. The importance of intrinsic plant properties raises important questions about the flexibility of leaf resorption under future changes in climate, CO2 concentrations and atmospheric deposition.
We assembled the global dataset from the gap-filled version of the TRY Plant Trait Database (https://www.try-db.org, last access: 14 March 2022, Kattge et al., 2020, version 5.0) containing field measurements of paired leaf and litter-mass-based tissue N and P concentrations (Nmass, leaf, Pmass, leaf, Nmass, litter, Pmass, litter) to derive the fractional nutrient resorption (described in Sect. 2.1).
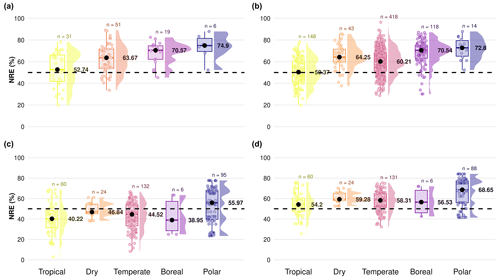
Figure A1Difference in nitrogen resorption efficiency (NRE %) among climate gradients from tropical to polar zones based on the Köppen climate classification, comparing four subdatasets to understand the importance of mass loss correction factor (MLCF) in the formula to derive nutrient resorption values: (a) nutrient resorption values derived directly from the nutrient resorption dataset with MLCF calculated from leaf dry mass or leaf mass loss measurements, (b) nutrient resorption values derived directly from the nutrient resorption dataset but with missing MLCF filled by the mean for each plant functional type (PFT), (c) nutrient resorption values derived from TRY traits with no MLCF in the formula and (d) nutrient resorption values derived from TRY traits but with missing MLCF filled by the mean for each PFT. Boxplots depict median (black dots), interquartile range and outliers, indicating data spread and variability. The side distributions show the overall data distribution for each climate zone. The dashed line indicates the overall mean NRE of 50 % used in most land surface models. “n” represents the number of observations per climate zone.
In order to understand the importance of considering MLCF in the formula to derive reliable nutrient resorption values, we compared four subdatasets from the final global dataset:
- a.
We derived nutrient resorption from the nutrient resorption database, in which MLCF was calculated directly from leaf dry mass or leaf mass loss measurements.
- b.
For the second dataset we derived nutrient resorption from the nutrient resorption database as well, but we filled the missing values of MLCF using the mean for each plant functional type (PFT): 0.712 for deciduous, 0.766 for evergreen, 0.69 for conifers and 0.75 for woody lianas, respectively.
- c.
For the third dataset we derived nutrient resorption using leaf nutrient and litter data from TRY traits, in which we did not include MLCF in the formula, calculated as
- d.
For the fourth dataset we derived nutrient resorption using leaf nutrient and litter data from TRY, but here we filled MLCF with the mean per PFT calculated before, in which we associated these means with leaf habit, leaf type and growth form information. For that, evergreen needle-leaved trees received conifer MLCF, deciduous trees and/or shrubs received deciduous woody MLCF, and evergreen trees and/or shrubs received evergreen woody MLCF, respectively.
Figure A1 shows nitrogen resorption efficiency (NRE) between different climate zones, where we can see underestimated values of resorption only when we do not consider MLCF in the formula (Fig. A1c), with values around or lower than 50 % of N resorption. We can see more reliable resorption values around 60 % when considering MLCF in the formula (Fig. A1a, b, d). When applying the mean of MLCF for the table deriving NRE from TRY traits (Fig. A1d), we are able to reproduce a similar pattern compared to the resorption database imported from TRY (Fig. A1a). Figure A2 shows the distribution of NRE for each subset described before, where we can see a clear difference in data distribution only when we do not consider MLCF in the formula (Fig. A2c). For our final dataset, we then considered the dataset (Fig. A2b) and (Fig. A2d) together, in which the most reliable data are for nutrient resorption as it provides more data points for resorption and considers MLCF in the formula.
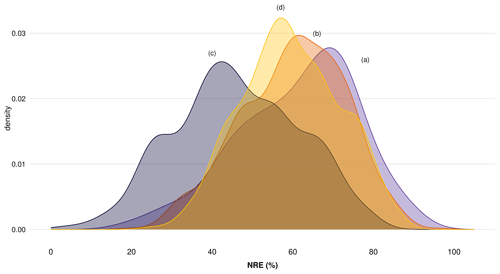
Figure A2Distribution of nitrogen resorption efficiency (NRE %) comparing four subdatasets to understand the importance of mass loss correction factor (MLCF) in the formula to derive nutrient resorption values: (a) nutrient resorption values derived directly from the nutrient resorption dataset with MLCF calculated from leaf dry mass or leaf mass loss measurements, (b) nutrient resorption values derived directly from the nutrient resorption dataset but with missing MLCF filled by the mean for each plant functional type (PFT), (c) nutrient resorption values derived from TRY traits with no MLCF in the formula and (d) nutrient resorption values derived from TRY traits but with missing MLCF filled by the mean for each PFT.
Table B1Summary of nitrogen resorption efficiency (NRE %) and phosphorus resorption efficiency (PRE %) in different climate zones. For each relationship, the number of observations (N), minimum (Min), maximum (Max), median and standard deviation (SD) were reported. Letters under “Significance” show the statistical comparison between each climate zone. “ns” indicates no significant difference.
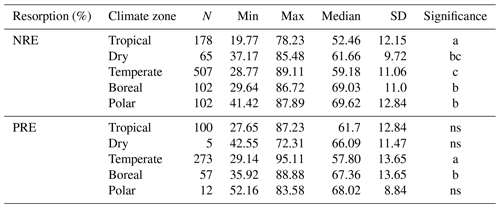
Table B2Summary of nitrogen resorption efficiency (NRE %) and phosphorus resorption efficiency (PRE %) in different plant functional types (PFTs). For each relationship, the number of observations (N), minimum (Min), maximum (Max), median, p value and standard deviation (SD) were reported. p value < 0.05 indicates statistical significance.
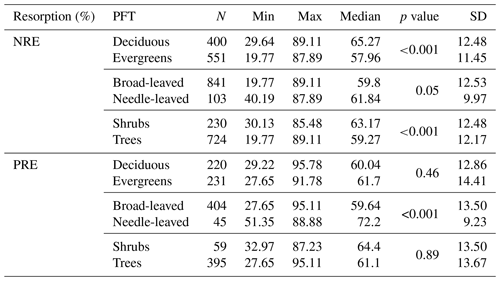
Table B3Summary of nitrogen resorption efficiency (NRE %) and phosphorus resorption efficiency (PRE %) in different plant functional types (PFT) separated in different climate zones. For each relationship, the number of observations (N), minimum (Min), maximum (Max), median and standard deviation (SD) were reported. Letters under “Significance” show the statistical comparison between each climate zone. “ns” indicates no significant difference.
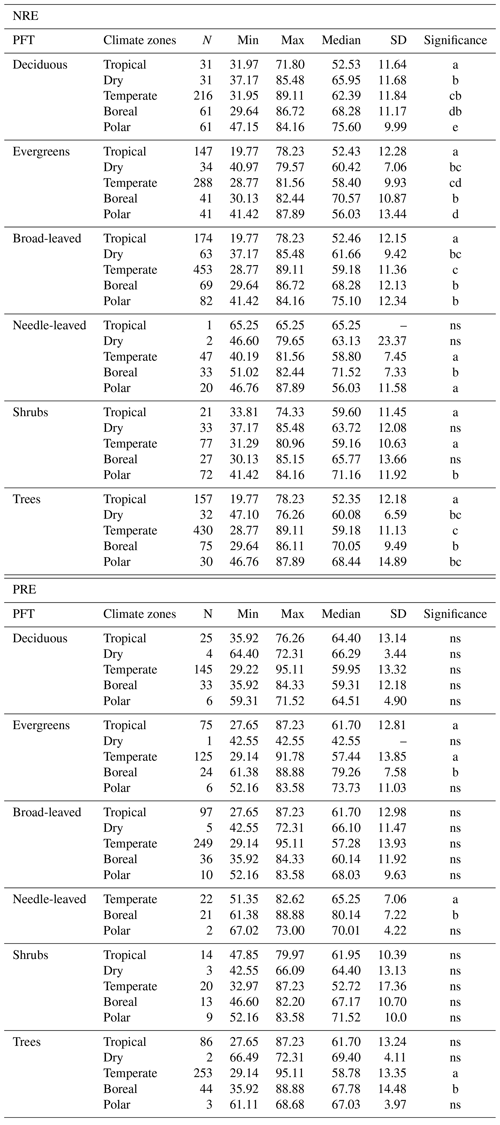
PFTs do not appear in the correlation matrix shown in Figs. C1, C2 and C3, as PFTs constitute a categorical variable. However, we explore the implication of SLA on nutrient resorption based on the strong and known relationship between SLA and PFTs in our dataset (Fig. C5), which derives from the leaf economics spectrum (LES) theory.
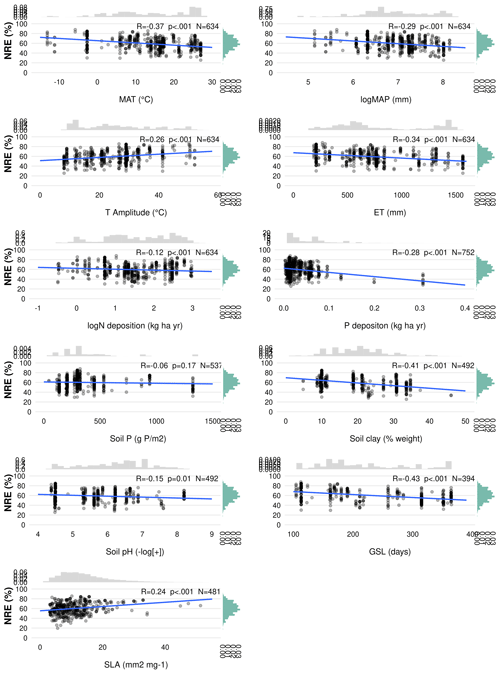
Figure C1Linear regression of nitrogen resorption efficiency (NRE %) with all possible predictor variables. Environmental predictors: mean annual temperature (MAT), mean annual precipitation (MAP), evapotranspiration (ET), temperature amplitude (T amplitude), nitrogen deposition (N deposition), phosphorus deposition (P deposition), total soil P (soil P), soil clay fraction (Soil Clay) and soil pH. Biological predictors: growing season length (GSL) and specific leaf area (SLA). R denotes Pearson correlation. p<0.05 is the statistical significance. N indicates the number of observations. The distribution on the right of the correlation represents the overall distribution of NRE values for each predictor.
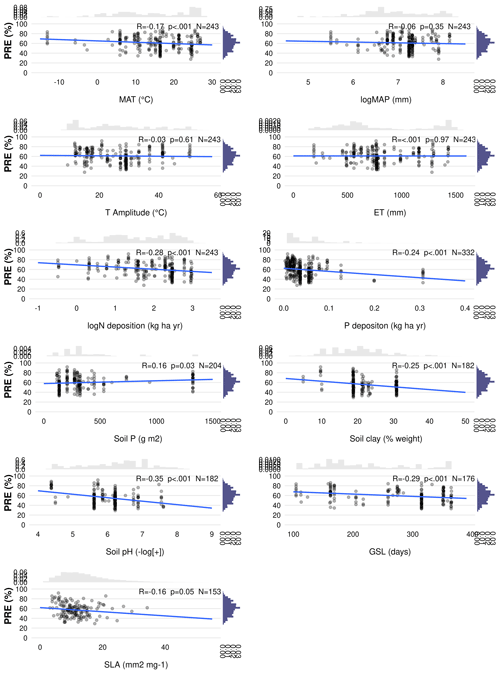
Figure C2Linear regression of phosphorus resorption efficiency (PRE; %) with all possible predictor variables. Environmental predictors: mean annual temperature (MAT), mean annual precipitation (MAP), evapotranspiration (ET), temperature amplitude (T amplitude), nitrogen deposition (N deposition), phosphorus deposition (P deposition), total soil P (soil P), soil clay fraction (Soil Clay), and soil pH. Biological predictors: Growing season length (GSL) and specific leaf area (SLA). R denotes the Pearson correlation. p<0.05 is the statistical significance. N indicates the number of observations. The distribution on the right of the correlation represents the overall distribution of PRE values for each predictor.
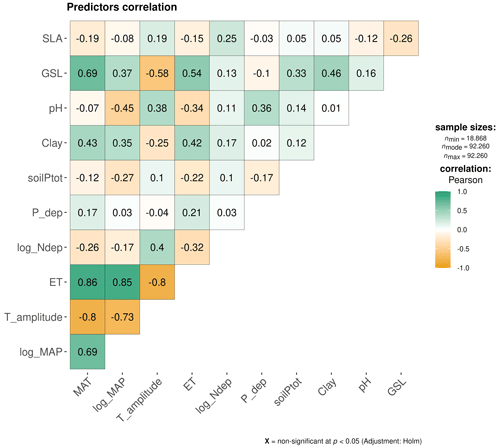
Figure C3Multiple Pearson correlation matrices between all predictors. The colour scale indicates the strength of the correlations, with green representing positive correlations and orange representing negative correlations, with non-significant correlations at p<0.05 indicated by “X”. Environmental predictors: mean annual temperature (MAT), mean annual precipitation (MAP), evapotranspiration (ET), temperature amplitude (T amplitude), nitrogen deposition (N deposition), phosphorus deposition (P deposition), total soil P (soil P), soil clay fraction (Soil clay) and soil pH. Biological predictors: growing season length (GSL) and specific leaf area (SLA).
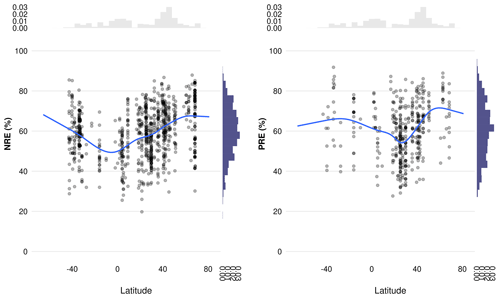
Figure C4Relationship between nitrogen resorption efficiency (NRE %) and phosphorus resorption efficiency (PRE %) with latitude. The scatter plots display individual observations, with the blue lines representing the smoothed regression curves, indicating trends in NRE and PRE across latitudes. Histograms on the top and right margins show the density distributions of latitude and resorption efficiencies, respectively. This visualization highlights the variation in nutrient resorption efficiencies across different latitudinal gradients.
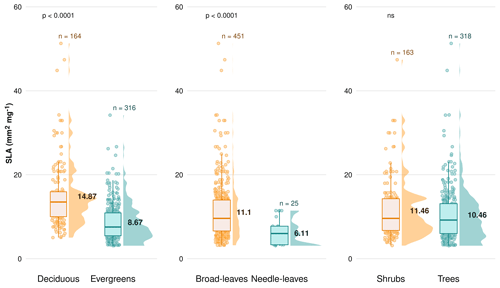
Figure C5Difference in the specific leaf area (SLA; mm2 mg−1) between plant functional types (PFTs) on a global scale, comparing deciduous vs. evergreens, broad-leaved species vs. needle-leaved and shrubs vs. trees. Boxplots depict median, interquartile range and outliers, indicating data spread and variability. The side distributions show the overall data distribution for each PFT. “n” represents the number of observations. p values indicate the significance of differences in SLA between PFTs. “ns” indicates no significant difference.
All data used in this study are publicly available through the TRY Plant Trait Database at https://www.try-db.org/ (TRY Plant Trait Database, 2022).
GS, SC and SZ designed the study. GS performed the analysis. All authors contributed to interpreting the results. GS drafted the manuscript; all authors contributed to writing and editing the manuscript.
At least one of the (co-)authors is a member of the editorial board of Biogeosciences. The peer-review process was guided by an independent editor, and the authors also have no other competing interests to declare.
Publisher’s note: Copernicus Publications remains neutral with regard to jurisdictional claims made in the text, published maps, institutional affiliations, or any other geographical representation in this paper. While Copernicus Publications makes every effort to include appropriate place names, the final responsibility lies with the authors.
We thank our external reviewer, Katrin Fleisher, for her helpful comments on the manuscript.
This work was supported by the European Research Council (ERC) under the European Union's Horizon 2020 research and innovation programme (QUINCY; grant no. 647204). Benjamin David Stocker was funded by the Swiss National Science Foundation (grant no. PCEFP2_181115).
The article processing charges for this open-access publication were covered by the Max Planck Society.
This paper was edited by Erika Buscardo and reviewed by Helena Vallicrosa and one anonymous referee.
Aerts, R.: Nutrient Resorption from Senescing Leaves of Perennials: Are there General Patterns?, J. Ecol., 84, 597–608, https://doi.org/10.2307/2261481, 1996.
Aerts, R. and Chapin, F. S.: The Mineral Nutrition of Wild Plants Revisited: A Re-evaluation of Processes and Patterns, Vol. 30, edited by: Fitter, A. H. and Raffaelli, D. G., Academic Press, 1–67, Advances in Ecological Research, https://doi.org/10.1016/S0065-2504(08)60016-1, 1999.
Arora, V. K., Seiler, C., Wang, L., and Kou-Giesbrecht, S.: Towards an ensemble-based evaluation of land surface models in light of uncertain forcings and observations, EGUsphere [preprint], https://doi.org/10.5194/egusphere-2022-641, 2022.
Augusto, L., Achat, D. L., Jonard, M., Vidal, D., and Ringeval, B.: Soil parent material-A major driver of plant nutrient limitations in terrestrial ecosystems, Glob. Change Biol., 23, 3808–3824, 2017.
Bartoń, K.: MuMIn: multi-model inference, R package version 1.47.5, CRAN (The Comprehensive R Archive Network), https://cran.r-project.org/package=MuMIn (lasta access: 17 January 2024), 2023.
Bazzaz, F. A.: The Response of Natural Ecosystems to the Rising Global CO2 Levels, Annu. Rev. Ecol. Syst., 21, 167–196, 1990.
Berg, B. and McClaugherty, C. A.: Plant Litter, Decomposition, Humus Formation, Carbon Sequestration, Springer Verlag, Springer Berlin, Heidelberg, https://doi.org/10.1007/978-3-642-38821-7, 2014.
Brahney, J., Mahowald, N., Ward, D. S., Ballantyne, A. P., and Neff, J. C.: Is atmospheric phosphorus pollution altering global alpine Lake stoichiometry?, Global Biogeochem. Cy., 29, 1369–1383, 2015.
Brant, A. N. and Chen, H. Y. H.: Patterns and Mechanisms of Nutrient Resorption in Plants, CRC Crit. Rev. Plant Sci., 34, 471–486, 2015.
Bryant, C., Wheeler, N. R., Rubel, F., and French, R. H.: kgc: Koeppen-Geiger Climatic Zones, R package version 1.0.0.2, CRAN (The Comprehensive R Archive Network), https://cran.r-project.org/package=kgc (lasta access: 16 January 2024), 2017.
Burnham, K. P. and Anderson, D. R.: Model Selection and Inference, Springer New York, 20 pp., https://doi.org/10.1007/b97636, 2002.
Chapin, F. S.: The Mineral Nutrition of Wild Plants, Annu. Rev. Ecol. Syst., 11, 233–260, 1980.
Chapin, F. S., Matson, P. A., and Vitousek, P. M.: Principles of Terrestrial Ecosystem Ecology, Springer, New York, NY, https://doi.org/10.1007/978-1-4419-9504-9, 2011.
Chen, H., Reed, S. C., Lü, X., Xiao, K., Wang, K., and Li, D.: Coexistence of multiple leaf nutrient resorption strategies in a single ecosystem, Sci. Total Environ., 772, 144951, https://doi.org/10.1016/j.scitotenv.2021.144951, 2021.
Chien, C.-T., Mackey, K. R. M., Dutkiewicz, S., Mahowald, N. M., Prospero, J. M., and Paytan, A.: Effects of African dust deposition on phytoplankton in the western tropical Atlantic Ocean off Barbados, Global Biogeochem. Cy., 30, 716–734, 2016.
Cleveland, C. C., Houlton, B. Z., Smith, W. K., Marklein, A. R., Reed, S. C., Parton, W., Del Grosso, S. J., and Running, S. W.: Patterns of new versus recycled primary production in the terrestrial biosphere, P. Natl. Acad. Sci. USA, 110, 12733–12737, 2013.
Dalling, J. W., Heineman, K., Lopez, O. R., Wright, S. J., and Turner, B. L.: Nutrient Availability in Tropical Rain Forests: The Paradigm of Phosphorus Limitation, in: Tropical Tree Physiology: Adaptations and Responses in a Changing Environment, edited by: Goldstein, G. and Santiago, L. S., Springer International Publishing, Cham, 261–273, https://doi.org/10.1007/978-3-319-27422-5_12, 2016.
Deng, M., Liu, L., Jiang, L., Liu, W., Wang, X., Li, S., Yang, S., and Wang, B.: Ecosystem scale trade-off in nitrogen acquisition pathways, Nat. Ecol. Evol., 2, 1724–1734, 2018.
Drenovsky, R. E., James, J. J., and Richards, J. H.: Variation in nutrient resorption by desert shrubs, J. Arid Environ., 74, 1564–1568, 2010.
Drenovsky, R. E., Pietrasiak, N., and Short, T. H.: Global temporal patterns in plant nutrient resorption plasticity, Glob. Ecol. Biogeogr., 28, 728–743, 2019.
Du, E., Terrer, C., Pellegrini, A. F. A., Ahlström, A., van Lissa, C. J., Zhao, X., Xia, N., Wu, X., and Jackson, R. B.: Global patterns of terrestrial nitrogen and phosphorus limitation, Nat. Geosci., 13, 221–226, https://doi.org/10.1038/s41561-019-0530-4, 2020.
Elser, J. J., Bracken, M. E. S., Cleland, E. E., Gruner, D. S., Harpole, W. S., Hillebrand, H., Ngai, J. T., Seabloom, E. W., Shurin, J. B., and Smith, J. E.: Global analysis of nitrogen and phosphorus limitation of primary producers in freshwater, marine and terrestrial ecosystems, Ecol. Lett., 10, 1135–1142, 2007.
Estiarte, M. and Peñuelas, J.: Alteration of the phenology of leaf senescence and fall in winter deciduous species by climate change: effects on nutrient proficiency, Glob. Change Biol., 21, 1005–1017, 2015.
Estiarte, M., Campioli, M., Mayol, M., and Peñuelas, J.: Variability and limits of nitrogen and phosphorus resorption during foliar senescence, Plant Commun., 4, 100503, https://doi.org/10.1016/j.xplc.2022.100503, 2023.
Fay, P. A., Prober, S. M., Harpole, W. S., Knops, J. M. H., Bakker, J. D., Borer, E. T., Lind, E. M., MacDougall, A. S., Seabloom, E. W., Wragg, P. D., Adler, P. B., Blumenthal, D. M., Buckley, Y. M., Chu, C., Cleland, E. E., Collins, S. L., Davies, K. F., Du, G., Feng, X., Firn, J., Gruner, D. S., Hagenah, N., Hautier, Y., Heckman, R. W., Jin, V. L., Kirkman, K. P., Klein, J., Ladwig, L. M., Li, Q., McCulley, R. L., Melbourne, B. A., Mitchell, C. E., Moore, J. L., Morgan, J. W., Risch, A. C., Schütz, M., Stevens, C. J., Wedin, D. A., and Yang, L. H.: Grassland productivity limited by multiple nutrients, Nat. Plants, 1, 15080, https://doi.org/10.1038/nplants.2015.80, 2015.
Fick, S. E. and Hijmans, R. J.: WorldClim 2: new 1-km spatial resolution climate surfaces for global land areas, Int. J. Climatol., 37, 4302–4315, 2017.
Finzi, A. C., Norby, R. J., Calfapietra, C., Gallet-Budynek, A., Gielen, B., Holmes, W. E., Hoosbeek, M. R., Iversen, C. M., Jackson, R. B., Kubiske, M. E., Ledford, J., Liberloo, M., Oren, R., Polle, A., Pritchard, S., Zak, D. R., Schlesinger, W. H., and Ceulemans, R.: Increases in nitrogen uptake rather than nitrogen-use efficiency support higher rates of temperate forest productivity under elevated CO2, P. Natl. Acad. Sci. USA, 104, 14014–14019, 2007.
Fisher, J. B., Sitch, S., Malhi, Y., Fisher, R. A., Huntingford, C., and Tan, S.-Y.: Carbon cost of plant nitrogen acquisition: A mechanistic, globally applicable model of plant nitrogen uptake, retranslocation, and fixation, Global Biogeochem. Cy., 24, GB1014, https://doi.org/10.1029/2009gb003621, 2010.
Galloway, J. N., Dentener, F. J., Capone, D. G., Boyer, E. W., Howarth, R. W., Seitzinger, S. P., Asner, G. P., Cleveland, C. C., Green, P. A., Holland, E. A., Karl, D. M., Michaels, A. F., Porter, J. H., Townsend, A. R., and Vöosmarty, C. J.: Nitrogen Cycles: Past, Present, and Future, Biogeochemistry, 70, 153–226, 2004.
Galloway, J. N., Townsend, A. R., Erisman, J. W., Bekunda, M., Cai, Z., Freney, J. R., Martinelli, L. A., Seitzinger, S. P., and Sutton, M. A.: Transformation of the nitrogen cycle: recent trends, questions, and potential solutions, Science, 320, 889–892, 2008.
Gill, A. L. and Finzi, A. C.: Belowground carbon flux links biogeochemical cycles and resource-use efficiency at the global scale, Ecol. Lett., 19, 1419–1428, 2016.
Güsewell, S.: N : P ratios in terrestrial plants: variation and functional significance, New Phytol., 164, 243–266, 2004.
Han, W., Tang, L., Chen, Y., and Fang, J.: Relationship between the relative limitation and resorption efficiency of nitrogen vs phosphorus in woody plants, PLoS One, 8, e83366, https://doi.org/10.1371/journal.pone.0083366, 2013.
Hedin, L. O., Brookshire, E. N. J., Menge, D. N. L., and Barron, A. R.: The Nitrogen Paradox in Tropical Forest Ecosystems, Annu. Rev. Ecol. Evol. Syst., 40, 613–635, 2009.
Hegglin, M., Kinnison, D., and Lamarque, J.-F.: CCMI nitrogen surface fluxes in support of CMIP6 – version 2.0, Earth System Grid Federation (ESGF)/input4MIPs, https://doi.org/10.22033/ESGF/input4MIPs.1125, 2016.
James, G., Witten, D., Hastie, T., and Tibshirani, R.: An Introduction to Statistical Learning: with Applications in R, Springer, New York, ISBN 978-1-4614-7138-7, 2013.
Jonard, M., Fürst, A., Verstraeten, A., Thimonier, A., Timmermann, V., Potočić, N., Waldner, P., Benham, S., Hansen, K., Merilä, P., Ponette, Q., de la Cruz, A. C., Roskams, P., Nicolas, M., Croisé, L., Ingerslev, M., Matteucci, G., Decinti, B., Bascietto, M., and Rautio, P.: Tree mineral nutrition is deteriorating in Europe, Glob. Chang.e Biol., 21, 418–430, 2015.
Jung, M., Reichstein, M., Margolis, H. A., Cescatti, A., Richardson, A. D., Arain, M. A., Arneth, A., Bernhofer, C., Bonal, D., Chen, J., Gianelle, D., Gobron, N., Kiely, G., Kutsch, W., Lasslop, G., Law, B. E., Lindroth, A., Merbold, L., Montagnani, L., Moors, E. J., Papale, D., Sottocornola, M., Vaccari, F., and Williams, C.: Global patterns of land-atmosphere fluxes of carbon dioxide, latent heat, and sensible heat derived from eddy covariance, satellite, and meteorological observations, J. Geophys. Res., 116, G00J07, https://doi.org/10.1029/2010jg001566, 2011.
Kattge, J., Díaz, S., Lavorel, S., Prentice, I. C., Leadley, P., Bönisch, G., Garnier, E., Westoby, M., Reich, P. B., Wright, I. J., Cornelissen, J. H. C., Violle, C., Harrison, S. P., Van Bodegom, P. M., Reichstein, M., Enquist, B. J., Soudzilovskaia, N. A., Ackerly, D. D., Anand, M., Atkin, O., Bahn, M., Baker, T. R., Baldocchi, D., Bekker, R., Blanco, C. C., Blonder, B., Bond, W. J., Bradstock, R., Bunker, D. E., Casanoves, F., Cavender-Bares, J., Chambers, J. Q., Chapin, F. S. III, Chave, J., Coomes, D., Cornwell, W. K., Craine, J. M., Dobrin, B. H., Duarte, L., Durka, W., Elser, J., Esser, G., Estiarte, M., Fagan, W. F., Fang, J., Fernández-Méndez, F., Fidelis, A., Finegan, B., Flores, O., Ford, H., Frank, D., Freschet, G. T., Fyllas, N. M., Gallagher, R. V., Green, W. A., Gutierrez, A. G., Hickler, T., Higgins, S. I., Hodgson, J. G., Jalili, A., Jansen, S., Joly, C. A., Kerkhoff, A. J., Kirkup, D., Kitajima, K., Kleyer, M., Klotz, S., Knops, J. M. H., Kramer, K., Kühn, I., Kurokawa, H., Laughlin, D., Lee, T. D., Leishman, M., Lens, F., Lenz, T., Lewis, S. L., Lloyd, J., Llusià, J., Louault, F., Ma, S., Mahecha, M. D., Manning, P., Massad, T., Medlyn, B. E., Messier, J., Moles, A. T., Müller, S. C., Nadrowski, K., Naeem, S., Niinemets, Ü., Nöllert, S., Nüske, A., Ogaya, R., Oleksyn, J., Onipchenko, V. G., Onoda, Y., Ordoñez, J., Overbeck, G., Ozinga, W. A., Penuelas, J., Pérez-Harguindeguy, N., Pillar, V. D., Pla, L., Poorter, H., Poschlod, P., Purschke, O., Qi, Y., Reich, M., Römermann, C., Sack, L., Salgado-Negret, B., Sardans, J., Shiodera, S., Shipley, B., Siefert, A., Sosinski, E. E., Soussana, J. F., Swaine, E., Swenson, N., Thompson, K., Thornton, P., Waldram, M., Weiher, E., White, M., Wright, S. J., Yguel, B., Zaehle, S., Zanne, A. E., and Wirth, C.: TRY – a global database of plant traits, Glob. Change Biol., 17, 2905–2935, 2011.
Kattge, J., Bönisch, G., Díaz, S., et al.: TRY plant trait database – enhanced coverage and open access, Glob. Change Biol., 26, 119–188, 2020.
Kikuzawa, K.: Leaf phenology as an optimal strategy for carbon gain in plants, Can. J. Bot., 73, 158–163, https://doi.org/10.1139/b95-019, 1995.
Kikuzawa, K. and Lechowicz, M. J.: Ecology of leaf longevity, 2011th Edn., Springer, Tokyo, Japan, 147 pp., https://doi.org/10.1007/978-4-431-53918-6, 2011.
Killingbeck, K. T.: Nutrients in senesced leaves: Keys to the search for potential resorption and resorption proficiency, Ecology, 77, 1716–1727, 1996.
Kobe, R. K., Lepczyk, C. A., and Iyer, M.: Resorption efficiency decreases with increasing green leaf nutrients in a global data set, Ecology, 86, 2780–2792, 2005.
Lacroix, F., Zaehle, S., Caldararu, S., Schaller, J., Stimmler, P., Holl, D., Kutzbach, L., and Goeckede, M.: Decoupling of permafrost thaw and vegetation growth could mean both ongoing nutrient limitation and an emergent source of N2O in high latitudes, Earth Space Sci. Open Arch., 1–43, https://doi.org/10.1002/essoar.10510605.1, 2022.
Lam, O. H. Y., Tautenhahn, S., Walther, G., Boenisch, G., Baddam, P., and Kattge, J.: The ‘rtry’ R package for preprocessing plant trait data, EGU General Assembly 2022, Vienna, Austria, 23–27 May 2022, EGU22-13251, https://doi.org/10.5194/egusphere-egu22-13251, 2022.
Lawrence, D. M., Fisher, R. A., Koven, C. D., Oleson, K. W., Swenson, S. C., Bonan, G., Collier, N., Ghimire, B., van Kampenhout, L., Kennedy, D., Kluzek, E., Lawrence, P. J., Li, F., Li, H., Lombardozzi, D., Riley, W. J., Sacks, W. J., Shi, M., Vertenstein, M., Wieder, W. R., Xu, C., Ali, A. A., Badger, A. M., Bisht, G., van den Broeke, M., Brunke, M. A., Burns, S. P., Buzan, J., Clark, M., Craig, A., Dahlin, K., Drewniak, B., Fisher, J. B., Flanner, M., Fox, A. M., Gentine, P., Hoffman, F., Keppel-Aleks, G., Knox, R., Kumar, S., Lenaerts, J., Leung, L. R., Lipscomb, W. H., Lu, Y., Pandey, A., Pelletier, J. D., Perket, J., Randerson, J. T., Ricciuto, D. M., Sanderson, B. M., Slater, A., Subin, Z. M., Tang, J., Thomas, R. Q., Val Martin, M., and Zeng, X.: The community land model version 5: Description of new features, benchmarking, and impact of forcing uncertainty, J. Adv. Model. Earth Syst., 11, 4245–4287, 2019.
LeBauer, D. S. and Treseder, K. K.: Nitrogen limitation of net primary productivity in terrestrial ecosystems is globally distributed, Ecology, 89, 371–379, 2008.
Liu, Y., Wang, C., He, N., Wen, X., Gao, Y., Li, S., Niu, S., Butterbach-Bahl, K., Luo, Y., and Yu, G.: A global synthesis of the rate and temperature sensitivity of soil nitrogen mineralization: latitudinal patterns and mechanisms, Glob. Change Biol., 23, 455–464, 2017.
Luo, Y., Su, B., Currie, W. S., Dukes, J. S., Finzi, A., Hartwig, U., Hungate, B., McMurtrie, R. E., Oren, R., Parton, W. J., Pataki, D. E., Shaw, R. M., Zak, D. R., and Field, C. B.: Progressive Nitrogen Limitation of Ecosystem Responses to Rising Atmospheric Carbon Dioxide, Bioscience, 54, 731–739, 2004.
Marklein, A. R. and Houlton, B. Z.: Nitrogen inputs accelerate phosphorus cycling rates across a wide variety of terrestrial ecosystems, New Phytol., 193, 696–704, 2012.
Onoda, Y., Wright, I. J., Evans, J. R., Hikosaka, K., Kitajima, K., Niinemets, Ü., Poorter, H., Tosens, T., and Westoby, M.: Physiological and structural tradeoffs underlying the leaf economics spectrum, New Phytol., 214, 1447–1463, 2017.
Patil, I.: Visualizations with statistical details: The “ggstatsplot” approach, J. Open Source Softw., 6, 3167, https://doi.org/10.21105/joss.03167, 2021.
Phillips, R. P., Brzostek, E., and Midgley, M. G.: The mycorrhizal-associated nutrient economy: a new framework for predicting carbon-nutrient couplings in temperate forests, New Phytol., 199, 41–51, 2013.
Reed, S. C., Townsend, A. R., Davidson, E. A., and Cleveland, C. C.: Stoichiometric patterns in foliar nutrient resorption across multiple scales, New Phytol., 196, 173–180, 2012.
Reich, P. B. and Flores-Moreno, H.: Peeking beneath the hood of the leaf economics spectrum, New Phytol., 214, 1395–1397, 2017.
Reich, P. B., Walters, M. B., and Ellsworth, D. S.: Leaf Life-Span in Relation to Leaf, Plant, and Stand Characteristics among Diverse Ecosystems, Ecol. Monogr., 62, 365–392, 1992.
Reich, P. B., Walters, M. B., and Ellsworth, D. S.: From tropics to tundra: global convergence in plant functioning, P. Natl. Acad. Sci. USA, 94, 13730–13734, 1997.
Sardans, J., Alonso, R., Janssens, I. A., Carnicer, J., Vereseglou, S., Rillig, M. C., Fernández-Martínez, M., Sanders, T. G. M., and Peñuelas, J.: Foliar and soil concentrations and stoichiometry of nitrogen and phosphorous across E uropean P inus sylvestris forests: relationships with climate, N deposition and tree growth, Funct. Ecol., 30, 676–689, 2016.
Sharma, P. K. and Kumar, S.: Soil Temperature and Plant Growth, in: Soil Physical Environment and Plant Growth: Evaluation and Management, edited by: Sharma, P. K. and Kumar, S., Springer International Publishing, Cham, 175–204, 2023.
Sun, X., Li, D., Lü, X., Fang, Y., Ma, Z., Wang, Z., Chu, C., Li, M., and Chen, H.: Widespread controls of leaf nutrient resorption by nutrient limitation and stoichiometry, Funct. Ecol., 37, 1653–1662, 2023.
Tang, L., Han, W., Chen, Y., and Fang, J.: Resorption proficiency and efficiency of leaf nutrients in woody plants in eastern China, J. Plant Ecol., 6, 408–417, 2013.
Terrer, C., Vicca, S., Hungate, B. A., Phillips, R. P., and Prentice, I. C.: Mycorrhizal association as a primary control of the CO2 fertilization effect, Science, 353, 72–74, 2016.
Thamdrup, B.: New Pathways and Processes in the Global Nitrogen Cycle, Annu. Rev. Ecol. Evol. Syst., 43, 407–428, 2012.
Thornton, P. E., Lamarque, J.-F., Rosenbloom, N. A., and Mahowald, N. M.: Influence of carbon-nitrogen cycle coupling on land model response to CO2 fertilization and climate variability, Global Biogeochem. Cy., 21, GB4018, https://doi.org/10.1029/2006gb002868, 2007.
TRY Plant Trait Database: TRY – a global database of plant traits, TRY Plant Trait Database [data set], https://www.try-db.org/ (last access: 14 March 2022), 2022.
Van Heerwaarden, L. M., Toet, S., and Aerts, R.: Current measures of nutrient resorption efficiency lead to a substantial underestimation of real resorption efficiency: facts and solutions, Oikos, 101, 664–669, 2003.
Van Langenhove, L., Verryckt, L. T., Bréchet, L., Courtois, E. A., Stahl, C., Hofhansl, F., Bauters, M., Sardans, J., Boeckx, P., Fransen, E., Peñuelas, J., and Janssens, I. A.: Atmospheric deposition of elements and its relevance for nutrient budgets of tropical forests, Biogeochemistry, 149, 175–193, 2020.
Veneklaas, E. J.: Phosphorus resorption and tissue longevity of roots and leaves – importance for phosphorus use efficiency and ecosystem phosphorus cycles, Plant Soil, 476, 627–637, 2022.
Vergutz, L., Manzoni, S., Porporato, A., Novais, R. F., and Jackson, R. B.: Global resorption efficiencies and concentrations of carbon and nutrients in leaves of terrestrial plants, Ecol. Monogr., 82, 205–220, 2012.
Wang, H., Prentice, I. C., Wright, I. J., Warton, D. I., Qiao, S., Xu, X., Zhou, J., Kikuzawa, K., and Stenseth, N. C.: Leaf economics fundamentals explained by optimality principles, Sci. Adv., 9, eadd5667, https://doi.org/10.1126/sciadv.add5667, 2023.
Wickham, H., Averick, M., Bryan, J., Chang, W., McGowan, L., François, R., Grolemund, G., Hayes, A., Henry, L., Hester, J., Kuhn, M., Pedersen, T., Miller, E., Bache, S., Müller, K., Ooms, J., Robinson, D., Seidel, D., Spinu, V., Takahashi, K., Vaughan, D., Wilke, C., Woo, K., and Yutani, H.: Welcome to the tidyverse, J. Open Source Softw., 4, 1686, https://doi.org/10.21105/joss.01686, 2019.
Wieder, W.: Regridded Harmonized World Soil Database v1.2, ORNL DAAC (Oak Ridge National Laboratory Distributed Active Archive Center), https://doi.org/10.3334/ORNLDAAC/1247, 2014.
Wright, I. J., Reich, P. B., Westoby, M., Ackerly, D. D., Baruch, Z., Bongers, F., Cavender-Bares, J., Chapin, T., Cornelissen, J. H. C., Diemer, M., Flexas, J., Garnier, E., Groom, P. K., Gulias, J., Hikosaka, K., Lamont, B. B., Lee, T., Lee, W., Lusk, C., Midgley, J. J., Navas, M.-L., Niinemets, U., Oleksyn, J., Osada, N., Poorter, H., Poot, P., Prior, L., Pyankov, V. I., Roumet, C., Thomas, S. C., Tjoelker, M. G., Veneklaas, E. J., and Villar, R.: The worldwide leaf economics spectrum, Nature, 428, 821–827, 2004.
Wu, H., Xiang, W., Ouyang, S., Xiao, W., Li, S., Chen, L., Lei, P., Deng, X., Zeng, Y., Zeng, L., and Peng, C.: Tree growth rate and soil nutrient status determine the shift in nutrient-use strategy of Chinese fir plantations along a chronosequence, Foest Ecol. Manag., 460, 117896, https://doi.org/10.1016/j.foreco.2020.117896, 2020.
Xu, M., Zhu, Y., Zhang, S., Feng, Y., Zhang, W., and Han, X.: Global scaling the leaf nitrogen and phosphorus resorption of woody species: Revisiting some commonly held views, Sci. Total Environ., 788, 147807, https://doi.org/10.1016/j.scitotenv.2021.147807, 2021.
Yan, T., Zhu, J., and Yang, K.: Leaf nitrogen and phosphorus resorption of woody species in response to climatic conditions and soil nutrients: a meta-analysis, J. Forest Res., 29, 905–913, https://doi.org/10.1007/s11676-017-0519-z, 2018.
Yang, X., Post, W. M., Thornton, P. E., and Jain, A.: The distribution of soil phosphorus for global biogeochemical modeling, Biogeosciences, 10, 2525–2537, https://doi.org/10.5194/bg-10-2525-2013, 2013.
Yuan, Z. Y. and Chen, H. Y. H.: Global-scale patterns of nutrient resorption associated with latitude, temperature and precipitation, Glob. Ecol. Biogeogr., 18, 11–18, 2009.
Yuan, Z. Y. and Chen, H. Y. H.: Negative effects of fertilization on plant nutrient resorption, Ecology, 96, 373–380, 2015.
Yuan, Z.-Y., Li, L.-H., Han, X.-G., Huang, J.-H., Jiang, G.-M., Wan, S.-Q., Zhang, W.-H., and Chen, Q.-S.: Nitrogen resorption from senescing leaves in 28 plant species in a semi-arid region of northern China, J. Arid Environ., 63, 191–202, 2005.
Zaehle, S.: Terrestrial nitrogen-carbon cycle interactions at the global scale, Philos. T. R. Soc. Lond. B, 368, 20130125, https://doi.org/10.1098/rstb.2013.0125, 2013.
Zaehle, S., Medlyn, B. E., De Kauwe, M. G., Walker, A. P., Dietze, M. C., Hickler, T., Luo, Y., Wang, Y.-P., El-Masri, B., Thornton, P., Jain, A., Wang, S., Warlind, D., Weng, E., Parton, W., Iversen, C. M., Gallet-Budynek, A., McCarthy, H., Finzi, A., Hanson, P. J., Prentice, I. C., Oren, R., and Norby, R. J.: Evaluation of 11 terrestrial carbon-nitrogen cycle models against observations from two temperate Free-Air CO2 Enrichment studies, New Phytol., 202, 803–822, 2014.
Zhang, M., Luo, Y., Meng, Q., and Han, W.: Correction of leaf nutrient resorption efficiency on the mass basis, J. Plant Ecol., 15, 1125–1132, 2022.
- Abstract
- Introduction
- Methods
- Results
- Discussion
- Conclusions
- Appendix A: Sensitivity study of the importance of MLCF
- Appendix B: Global patterns of nutrient resorption efficiency for N and P by PFTs and climate zones
- Appendix C: Linear regressions of nutrient resorption with environmental and biological factors
- Data availability
- Author contributions
- Competing interests
- Disclaimer
- Acknowledgements
- Financial support
- Review statement
- References
- Abstract
- Introduction
- Methods
- Results
- Discussion
- Conclusions
- Appendix A: Sensitivity study of the importance of MLCF
- Appendix B: Global patterns of nutrient resorption efficiency for N and P by PFTs and climate zones
- Appendix C: Linear regressions of nutrient resorption with environmental and biological factors
- Data availability
- Author contributions
- Competing interests
- Disclaimer
- Acknowledgements
- Financial support
- Review statement
- References