the Creative Commons Attribution 4.0 License.
the Creative Commons Attribution 4.0 License.
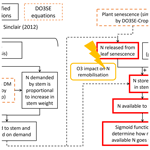
New ozone–nitrogen model shows early senescence onset is the primary cause of ozone-induced reduction in grain quality of wheat
Clare Brewster
Felicity Hayes
Nathan Booth
Sam Bland
Pritha Pande
Samarthia Thankappan
Håkan Pleijel
Lisa Emberson
Ozone (O3) air pollution is well known to adversely affect both the grain and protein yield of wheat, an important staple crop. This study aims to identify and model the key plant processes influencing the effect of O3 on wheat protein. The DO3SE-Crop model was modified in this work to incorporate nitrogen (N) processes, and we parameterised the O3 effect on stem, leaf, and grain N using O3 fumigation datasets spanning 3 years and four O3 treatments. These modifications mean that the newly developed DO3SE-CropN model is the first crop model to include O3 effects on N processes, making it a valuable tool for understanding O3 effects on wheat quality. Our results show that the new model captures the O3 effect on grain N concentrations and the anthesis leaf and stem concentrations well, with an R2 of 0.6 for the increase in grain N concentration and an R2 of 0.3 for the decrease in grain N content under O3 exposure. However, the O3 effect on harvest leaf and stem N is exaggerated. Overestimations of harvest leaf N range from ∼20 % to 120 %, while overestimations of harvest stem N range from ∼40 % to 120 %. Further, a sensitivity analysis revealed that, irrespective of O3 treatment, early senescence onset (simulated as being ∼13 d earlier in the treatment with very high O3 vs. the low-O3 treatment) was the primary plant process affecting grain N. This finding has implications for the breeding of stay-green cultivars for maintaining yield, as well as quality, under O3 exposure. This modelling study therefore demonstrates the capability of the DO3SE-CropN model to simulate processes by which O3 affects N content and, thereby, determines that senescence onset is the main driver of O3 reductions in grain protein yield. The implication of the sensitivity analysis is that breeders should focus their efforts on stay-green cultivars that do not experience a protein penalty when developing O3-tolerant lines, to maintain both wheat yield and nutritional quality under O3 exposure. This work supports the second phase of the Tropospheric Ozone Assessment Report (TOAR) by investigating the impacts of tropospheric O3 on wheat, with a focus on wheat quality impacts that will subsequently affect human nutrition.
- Article
(3513 KB) - Full-text XML
-
Supplement
(709 KB) - BibTeX
- EndNote
The first phase of the Tropospheric Ozone Assessment Report (TOAR; https://igacproject.org/activities/TOAR/TOAR-I, last access: 4 September 2024) built the world's largest database of surface ozone (O3) metrics to identify the global distribution of the pollutant and trends in O3 concentrations over time. The second phase of TOAR (https://igacproject.org/activities/TOAR/TOAR-II, last access: 4 September 2024), to which this paper contributes, has a broader scope, with one of the additional aims being to investigate the impact of tropospheric O3 on human health and vegetation. The present work will address these goals by developing impact assessment methods that consider the interaction between O3 and nitrogen (N) in crops. As N is a key component of protein, the effects of O3 on N have the potential to impact crop quality and, as a result, human nutrition. Areas for further investigation to provide deeper understanding of O3–N interactions are identified, and recommendations for future work to mitigate the negative effects of O3 on both crop yield and protein are discussed.
1.1 The importance of wheat for nutrition and the threat of O3
The Food and Agricultural Organisation (FAO) of the United Nations projects that staple cereals will play a critical role in ensuring food security, particularly in Central and West Asia and North Africa where wheat provides at least 40 % and 47 % of dietary calories and protein respectively, compared with ∼20 % of dietary calories and protein globally (FAO, 2017). There is a large body of evidence, including work from the first phase of TOAR, suggesting that current ambient O3 concentrations in key wheat-growing locations are causing substantial productivity losses of equal importance to other, more well-known, biotic and abiotic stresses (Emberson et al., 2009; Mills et al., 2018a, c). Globally, wheat production is reduced by ∼7 %, although in some regions with high ambient O3 concentrations, such as northern India, the yield loss is much greater (>15 %) (Mills et al., 2018c). There is also a growing body of literature showing that O3 affects the nutritional quality of the wheat grain and reduces the protein yield (Broberg et al., 2015; Piikki et al., 2008; A. Yadav et al., 2019; Yadav et al., 2020). Over the next century, increases in the global population and economic growth as well as changes to climate and land use will increase emissions of O3 precursors (NOx and volatile organic compounds) (Fowler et al., 2008). For Shared Socioeconomic Pathways (SSP) scenario 3-70 (often termed the “business-as-usual” scenario), projections show that O3 concentrations will increase in most locations globally, particularly South and East Asia, South America, Africa, and the Middle East (Szopa et al., 2021). Alterations to local meteorology and carbon dioxide (CO2) concentrations under climate change will also influence O3 production (Fu and Tian, 2019), with Zanis et al. (2022) finding that climatic conditions will likely increase O3 production in regions close to emissions sources. Understanding the O3 impact on the supply and nutritional quality of wheat grown in regions where O3 concentrations are high or predicted to increase is crucial (FAO et al., 2020). This will help us understand and address the threats posed by O3 pollution with respect to the ability of future wheat production to meet increasing demand and nutrient requirements (Mills et al., 2018b; Shiferaw et al., 2013).
1.2 The impact of O3 on wheat quality and the mechanisms by which damage occurs
Reactive oxygen species (ROS), formed by O3 entering the leaves through the stomata, trigger a series of reactions that reduce grain yield and quality (Broberg et al., 2015; Emberson et al., 2018). The uptake and remobilisation of nutrients under O3 exposure is affected less severely by O3 than the O3-induced reduction in dry matter (DM). This results in a decrease in the nutrient yield of the grains (grams of nutrient per square metre) but an increase in the nutrient concentration (grams of nutrient per gram of DM) (Broberg et al., 2015; Wang and Frei, 2011). Broberg et al. (2015) further found that an increased grain protein concentration caused the baking properties, quantified by the Zeleny value, Hagberg falling number, and dry and wet gluten content, to be positively affected by O3. In some wheat studies, where O3 concentrations are very high, the grain protein concentration is decreased, potentially as a result of N being used for antioxidant production and defence against O3 (Baqasi et al., 2018; Fatima et al., 2018; Mishra et al., 2013; D. S. Yadav et al., 2019, 2020).
The main mechanism by which O3 reduces wheat yields and impacts quality is through accelerated senescence (Emberson et al., 2018). Wheat cultivars with delayed senescence, stay-green cultivars, have previously been trialled for their potential to offset yield reductions under environmental stressors such as heat and drought (Kamal et al., 2019). However, accelerated senescence typically reduces protein remobilisation and reduces wheat quality (Havé et al., 2017; Sultana et al., 2021). Understanding the mechanisms by which O3 damages crop yield and influences crop quality is crucial for the breeding of O3-tolerant cultivars. Section 2.2.1 provides more detail and a mechanistic description of how wheat yields and protein are affected by O3.
1.3 The current status of crop modelling with regards to N and O3
The current understanding of the effect of O3 pollution on wheat nutritional quality has been inferred from experimental studies (Broberg et al., 2015, 2021; Feng et al., 2008; Mills et al., 2011). However, experimental work is time-consuming and costly, and it can be difficult to control all of the variables involved. Crop models use environmental inputs to simulate crop growth, for a range of conditions and stressors, in far less time, using fewer resources than required for experimental investigation (Chenu et al., 2017). Developing crop models with experimental data, which provide insights into plant growth processes, allows for the investigation of realistic plant responses to individual and multiple stressors.
It is possible to simulate the O3 effect on grain protein by incorporating N processes into an existing crop model considering O3 damage and by using a simple conversion factor (e.g. Mariotti et al., 2008) to convert N to protein. Many models consider N dynamics in wheat (e.g. APSIM-Nwheat and CERES-Wheat) (see Table S1 in the Supplement), and others have incorporated O3 damage (e.g. LINTULLC2, WOFOST, APSIM, and DO3SE-Crop) (Nguyen et al., 2024; Xu et al., 2023). Some models, such as APSIM, can simulate both O3 effects on yield and grain N, which could be used to calculate grain protein and, hence, provide a measure of grain quality variation under O3 exposure. However, to our knowledge, no simulations have yet been performed on the O3 effect on grain N. Further, these models do not yet include the mechanisms that relate O3 to grain N. Currently, no model simulates the reduced remobilisation of N under O3 exposure from the stem and leaf to the grain, an important determiner of wheat protein under O3 exposure (Brewster, 2023; Brewster et al., 2024; Broberg et al., 2017, 2021; Chang-Espino et al., 2021).
1.4 Objectives
This study aims to develop and use the DO3SE-Crop model to investigate the impact of O3 on the wheat grain N content via the following objectives:
-
identifying the key mechanisms necessary to model N in crops and the influence of O3 on these mechanisms;
-
developing an N module that can be incorporated into the existing O3 deposition and crop growth model, DO3SE-Crop, to simulate how grain N (and hence protein) is affected by O3 exposure (DO3SE-CropN);
-
using the developed DO3SE-CropN model to perform a sensitivity analysis to determine which of the O3 damage mechanisms (senescence onset, senescence rate/end, and remobilisation of N) affects grain quality the most.
2.1 Overview of the DO3SE-Crop model
The DO3SE-Crop model is used to estimate O3 deposition to a plant canopy and the impacts (biomass and yield loss) caused by stomatal O3 uptake (Emberson et al., 2018). The crop phenology is estimated based on thermal time sums. Photosynthesis is simulated at the leaf level, based on a modified version of the biochemical Farquhar model (Farquhar et al., 1980), and scaled to the canopy level by splitting the canopy into equally sized layers of the cumulative leaf area index (LAI). The photosynthetic products from each layer are summed to give the net primary productivity (NPP). The NPP is allocated to the root, stem, leaf, or grain based on the plant's developmental stage using the approach of Osborne et al. (2015). O3 transfer from the atmosphere to the leaf is estimated by a resistance scheme incorporating aerodynamic, boundary layer, and surface resistances above and within the canopy (Pande et al., 2024a). The instantaneous impact of stomatal O3 flux on photosynthesis and the impacts of accumulated O3 flux on senescence, once the cumulative flux exceeds a cultivar specific threshold, are estimated based on the approach of Ewert and Porter (2000) and modified by Pande et al. (2024a). Further details of the DO3SE-Crop model along with a mathematical description can be found in Pande et al. (2024a). In this study, version 4.39.11 of the DO3SE-Crop model was used (Bland, 2024).
2.2 Development of the N module
2.2.1 Identification of which N processes to model
The key plant processes influenced by N and O3 were identified to guide decisions on which processes to include in the N module for DO3SE-Crop (Fig. 1). Figure 1 provides an overview of processes already included in DO3SE-Crop, those to be added in the new N module, and those which are excluded. In brief, DO3SE-Crop includes an instantaneous short-term effect of O3 on carboxylation efficiency which subsequently affects photosynthesis. The leaf has the capacity to recover from this O3 damage overnight, although recovery ability decreases with age. Additionally, we simulate a long-term effect of O3 on accelerating senescence. Details of the DO3SE-Crop model and associated O3 damage processes are given by Pande et al. (2024a). Figure 1 is separated into numbered sections which are explained in the subsequent text:
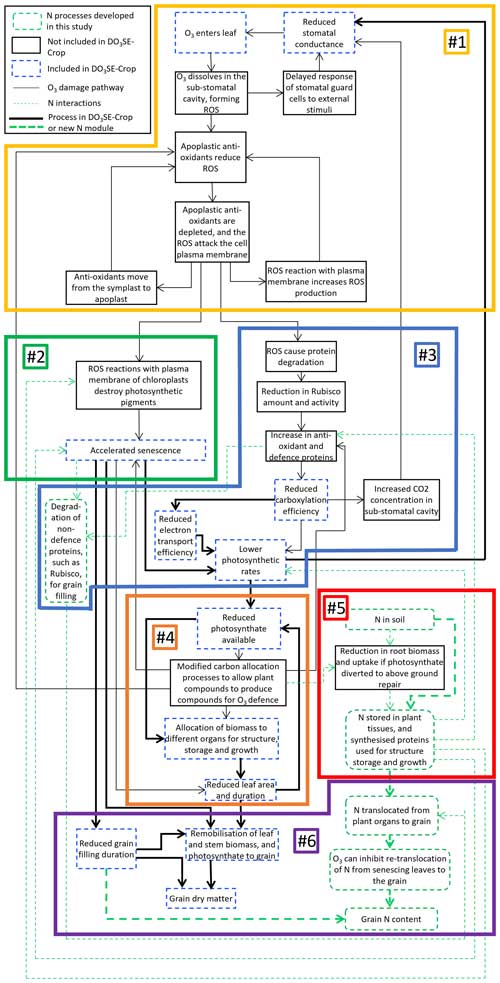
Figure 1A flow chart of the mechanisms by which O3 causes damage to both grain yields and grain N (or protein) in wheat. The different colours and line styles outlining the individual boxes represent whether the process is included in the DO3SE-Crop model already (dashed blue outline), not included in the DO3SE-Crop model (solid black outline), or included in the N module developed for DO3SE-Crop in this study (dashed green outline, rounded boxes). The solid black connector lines represent interactions between the O3 damage processes, while the dashed green connector lines represent where N processes interact with these. The thinner lines represent interactions that are not included in the DO3SE-Crop model or the new N module, whereas thicker lines represent the interactions that are included. The figure has been divided into six numbered sections for which the mechanisms are described individually in Sect. 2.2.1.
- 1
Reactive oxygen species (ROS), which are formed when O3 diffuses through leaf stomata, trigger a series of physiological and stress responses in the plant that lead to accelerated senescence and a reduced photosynthetic rate (Emberson et al., 2018). ROS delay stomatal response to external stimuli, reducing stomatal conductance (Dai et al., 2019; Paoletti and Grulke, 2010). ROS are detoxified by apoplastic antioxidants, but an excess of ROS may overwhelm the antioxidant response, damaging the cell plasma membrane (Emberson et al., 2018; Fatima et al., 2019).
- 2
ROS destroy photosynthetic pigments (Emberson et al., 2018). Degradation of photosynthetic pigments by ROS accelerates crop senescence, during which Rubisco, comprising 50 % of soluble leaf protein, is broken down to release N for remobilisation to other parts of the plant (Emberson et al., 2018; Feller and Fischer, 1994).
- 3
Degradation of Rubisco by ROS leads to reduced carboxylation efficiency (Emberson et al., 2018). Together with a reduced electron transport efficiency, photosynthetic rate is reduced (Emberson et al., 2018; Rai and Agrawal, 2012). There is an increase in antioxidant and defence proteins triggered by elevated-O3 conditions (Cho et al., 2011; Fatima et al., 2018; Sarkar et al., 2010).
- 4
O3-induced accelerated senescence reduces the green-leaf area for photosynthesis, further decreasing carbon assimilation (Emberson et al., 2018). Diminished photosynthesis leads to lesser photosynthate production (Emberson et al., 2018). More photosynthate is used in respiration and for antioxidant production to target ROS (Emberson et al., 2018; Khanna-Chopra, 2012). Under O3 stress, annual crops, such as wheat, prioritise allocation of assimilates to flowers and seeds, reducing the availability for leaves, stems, and roots (Emberson et al., 2018).
- 5
N taken up by the plant is used to produce all proteins (Lawlor, 2002). Root biomass, and subsequently nutrient uptake, is reduced under stress conditions, as assimilate allocation to repair above-ground O3 damage is prioritised over export to the roots (Emberson et al., 2018; Pandey et al., 2018). While O3 can induce senescence and reduce photosynthesis, a higher leaf N can delay the onset of senescence and increase the photosynthetic rate (Brewster et al., 2024; Martre et al., 2006; Nehe et al., 2020; Pilbeam, 2010). On the other hand, N deficiency can damage the structure and function of the chloroplasts which could exacerbate O3 impacts on senescence and the photosynthetic rate (Kang et al., 2023). Brewster et al. (2024) found that an increase in residual leaf and stem N occurs, potentially as a result of O3 toxicity.
- 6
Wheat yields are decreased due to reduced photosynthesis and grain-filling duration (Broberg et al., 2015; Emberson et al., 2018). Wheat grain N comprises N taken up post-anthesis and N remobilised from the leaves and stem when senescence begins (Barraclough et al., 2014; Gaju et al., 2014; Havé et al., 2017; Nehe et al., 2020). Hence, any damage mechanism which affects grain-filling duration, influences the final N content of the wheat grains. Additionally, as Rubisco is a key source of N for grains, once senescence begins, reductions to Rubisco will impact the amount of N that is available to grains (Feller and Fischer, 1994). Brewster et al. (2024) and Chang-Espino et al. (2021) have found evidence of an additional, unknown process, independent of accelerated senescence, that reduces the remobilisation of N under O3 exposure.
Generally, grain protein concentrations increase under elevated-O3 conditions, due to a smaller decrease in N uptake and re-translocation relative to the O3-induced decrease in grain DM (Broberg et al., 2015, 2019; Piikki et al., 2008; Triboi and Triboi-Blondel, 2002; Wang and Frei, 2011). However, per metre square of crop, the starch and grain protein yield is reduced (Broberg et al., 2015; Feng et al., 2008; Gelang et al., 2000). Some wheat cultivars have shown a decrease in the grain protein concentration under O3 exposure (Baqasi et al., 2018; Mishra et al., 2013; A. Yadav et al., 2019). This could be because leaf proteins are converted to enzymatic antioxidants to defend against O3-induced damage, resulting in less protein being available for translocation to the grains (Fatima et al., 2018; Sarkar et al., 2010; D. S. Yadav et al., 2019).
2.2.2 Assessment of existing crop models that include N
Table S1 summarises and discusses the similarities between models that simulate plant N dynamics. Most models simulated leaf and stem N by fulfilling the required N demanded by the respective parts from the N uptake pool, with N demand based on a defined minimum and maximum for that organ. The maximum and minimum N concentrations can be set as constants or defined using the phenological stage of the plant, which in turn is based on the accumulation of thermal time or a temperature sum based on a scheme by van Keulen and Seligman (1987). Most crop models define a labile pool of N available to be translocated to the grain and consisting of N available from post-anthesis uptake, non-structural stem N, and N released from leaf senescence. The N released from leaf senescence is calculated in proportion to the decrease in carbon of green-leaf area, as N remobilisation is proportional to carbon remobilisation (Havé et al., 2017). Most of the crop models simulated grain N by calculating and fulfilling an N demand or by simulating a rate at which the grains fill with N.
2.2.3 Modifications to the existing DO3SE-Crop model for this study
Prior applications of the DO3SE-Crop model have assumed that the last 33 % of the mature-leaf lifespan is when leaf senescence occurs (e.g. Pande et al., 2024a). In some of these applications, multiple leaf populations were considered. Given the limitations of available data in parameterising the model for multiple leaf populations, only one leaf population is considered in the present study. As a result, the fraction of the mature-leaf lifespan that is senescence needed to be modified to instead simulate the fraction of the canopy mature-leaf lifespan that is senescence. Recent work by Brewster et al. (2024) has shown that the fourth leaves can begin to senesce even before anthesis. Given the importance of senescence in determining N remobilisation (Gaju et al., 2014; Nehe et al., 2020), work by Brewster et al. (2024) was used to re-parameterise the onset of the rapid phase of leaf senescence as the last 75 % of the canopy level mature-leaf lifespan for the Skyfall cultivar in DO3SE-Crop.
2.2.4 The DO3SE-Crop N module for wheat
Based on Sects. 2.2.1 and 2.2.2, we identified the following key processes for inclusion in the N module: soil N uptake, partitioning of N uptake between the leaf and stem, remobilisation of N in the leaf and stem to the grains, grain filling with N, and O3 effects on grain N. At the present modelling stage, we do not include any processes relating to usage of N for antioxidant production or utilisation of photosynthate for above-ground repair due to the lack of data for parameterisation. Full details of equations, sources of equations, and model parameterisations are available in Appendix A. Briefly, the key processes are as follows:
- (a)
Soil N uptake. Pre-anthesis, daily N uptake from the soil is proportional to the increase in the LAI and stem mass that day, along with any N deficit that has accumulated over the plant's life, following the work of Soltani and Sinclair (2012). Post-anthesis, we use the formulation from SiriusQuality (Martre et al., 2006) that links post-anthesis N uptake with the capacity of the stem to store N. Pre- and post-anthesis, we define a maximum N uptake which cannot be exceeded. As we did not have data to calibrate for the effects of N stress, the present model assumes optimal soil N availability.
- (b)
N partitioning. Pre-anthesis, N uptake is allocated to the leaf and stem in accordance with the increase in the LAI or stem mass that day, as commonly used by other crop modellers (Sect. 2.2.2). The specific equations used closely follow those of Soltani and Sinclair (2012).
- (c)
N remobilisation. After anthesis, N remobilisation from the stem to the grains begins. N is released from senescing leaves in accordance with the decrease in the LAI that day. Released N is stored in the stem where it is available to the grain. The combination of N released from leaf senescence and non-structural stem N creates the labile pool of N for grain filling.
- (d)
Grain N. The N in the labile pool can be transferred to the grain or remain as part of the stem. In contrast to other crop models, the proportion of labile N transferred to the grain each day follows a sigmoid function. The sigmoid was chosen as it uses only two extra parameters (αN and βN); this allows the start and rate of grain fill with N to be customised without the addition of much complexity. The fraction leaving the labile pool increases as the plant develops.
where Nto_grain represents the amount of N leaving the labile pool (Nlabile) to the grains and DVI represents the development index of the plant in DO3SE-Crop (Pande et al., 2024a). The Nto_grain profile for different parameterisations of αN and βN is shown in Appendix A (Fig. A3).
- (e)
Direct effect of O3 on grain N. The fraction of N remaining in the leaf and straw increases with O3 exposure (Broberg et al., 2017, 2021). Additionally, in the work of Brewster et al. (2024), the same effect is observed, as a lower proportion of N stored in these parts at anthesis is moved to the grains. Few data are available on this effect, so we used all available existing data from Broberg et al. (2017) and Brewster et al. (2024) to produce a linear regression of the percentage of N remaining in the leaf and stem at harvest as a function of M12 (the common metric for the two studies – defined as the 12 h mean O3 concentration during daylight hours; Guarin et al., 2019). The results of this can be seen in Fig. 2.
The minimum allowed leaf and stem N concentrations were varied to optimise the grain nitrogen percentage (hereafter N%) and harvest leaf and stem N% simulations, whilst making sure that the percentage of N remaining in the leaf and stem was within the 95 % confidence interval (CI) of the remobilisation regression. The form of the regressions representing the minimum leaf and stem N concentrations under O3 exposure are given in Eqs. (2) and (3) respectively.
where [Nleaf,min] is the minimum leaf N concentration in grams of nitrogen per unit LAI, [Nstem,min] is the minimum stem N concentration in grams of nitrogen per gram of stem DM, and [O3,M12] is the concentration of O3 using the M12 metric in parts per billion. The parameterisation for Eqs. (2) and (3) is given in Table 1. Further details of the process by which the best parameters were obtained is given in Sect. A4.
- (f)
Indirect effect of O3 on grain N. In the DO3SE-Crop model, O3 accelerates the onset and rate of senescence (Pande et al., 2024a). In this N module, remobilisation of N from senescing leaves occurs once senescence begins. Further, no N is remobilised from the leaves once senescence is complete. DO3SE-Crop also simulates the impact of O3 on the rate of photosynthesis and, consequently, biomass production and leaf area expansion (Pande et al., 2024a). In this N module, leaf area determines accumulation of leaf N and stem biomass determines the accumulation of stem N, providing an indirect link between O3 damage in the DO3SE-Crop model and the newly developed N module.
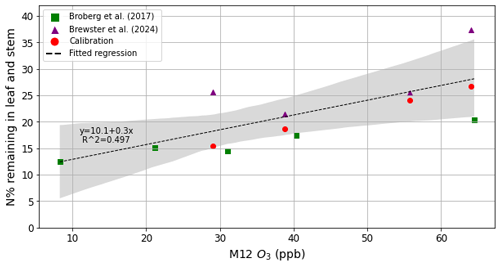
Figure 2The percentage of N remaining in the leaf and stem as a function of M12 for studies by Broberg et al. (2017) (square green markers) and supplementary data obtained from Brewster et al. (2024) (triangular purple markers). The grey area represents the 95 % CI of the fitted regression (dashed linear line), and the R2 of the regression is given in the figure. Overlaid are circular red markers showing the effect of the calibrated mleaf, cleaf, mstem, and cstem on overall remobilisation.
Table 1The calibrated values for the newly developed regressions describing how the minimum leaf and stem N concentrations vary under differing O3 concentrations. All parameters are unitless.
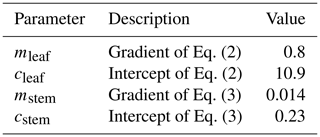
In combination, the N and DO3SE-Crop processes are integrated to form the DO3SE-CropN model, as shown in Fig. 3. Simply, N uptake is partitioned in accordance with demand from the leaf and stem. The N available to the grain comes from senesced leaf area, post anthesis N uptake, and non-structural stem N. The amount of N that is transferred to the grain from this available pool is calculated using a sigmoid function. The fraction of N that is available to the grain from the leaf and stem is modified in accordance with daily O3 concentrations. Further details of the equations used and processes involved are given in Appendix A. In this study, version 1.0 of the N module was used, and the corresponding code can be found at Cook (2024).
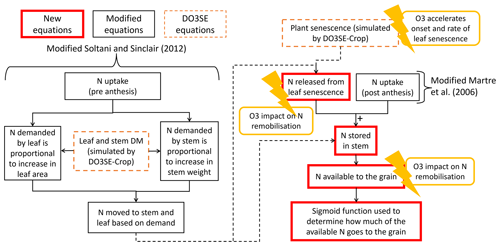
Figure 3Simplified overview of the DO3SE-CropN module. Boxes with red outlines show the processes developed in this study. Boxes with orange outlines indicate where the N module takes outputs from the DO3SE-Crop model. Boxes with black outlines indicate where equations were taken from the existing literature and modified for the current study. The lightning bolts represent the locations where O3 impacts plant N processes in the newly developed DO3SE-CropN model.
Experimental data have been collated and gap filled (using the AgMIP Ozone gap-filling methodology; Methodology for gap-filling and standardisation of data for AgMIP Ozone V8, 2024) to calibrate and evaluate the DO3SE-Crop model and newly developed N module. Further details of the gap-filling methods can be found in the Supplement.
3.1 Experimental datasets
Data from the Centre for Ecology & Hydrology, Bangor, from the years 2015, 2016, and 2021 were used to calibrate the N module for DO3SE-Crop. For each year, the Skyfall wheat cultivar was grown in solar domes under four O3 treatment conditions, with median O3 concentrations ranging from 29 to 61.1 ppb. Across the 3 years, wheat was planted between 23 February and 15 April and harvested between 11 August and 17 August. O3 fumigation occurred between stem elongation (GS30) and harvest (mid-August) (Brewster et al., 2024; Broberg et al., 2023; Osborne et al., 2019, and additional data as detailed in the “Data availability” section). In this study, only treatments in which the plants were well watered and experienced no N or temperature stress were used. No grain data were used for the year 2021, as the plants did not put on any grain (see Brewster et al., 2024, for further detail). A tabulation of data available from each year can be found in Table S2. Further details of the experimental set-up for all years can be found in Brewster et al. (2024), Broberg et al. (2023), and Osborne et al. (2019), and additional data as detailed in the “Data availability” section were used for model development.
3.2 Model calibration and evaluation
Model calibration was performed in stages, with the calibrated parameters indicated in parentheses. Definitions of DO3SE-Crop parameters, along with the value they were calibrated to and the units, are given in Table S3. These parameters are as follows:
-
phenology (Tb, To, Tm, TTemr, TTflag,emr, TTastart, TTamid, and TTharv);
-
photosynthesis and respiration (Vcmax,25, kN, Jmax,25, m, Rdcoeff, and Rg);
-
DM allocation and yield and the O3 effect on yield and senescence (αroot, βroot, αleaf, βleaf, αstem, βstem, Ω, τ, Eg, γ3, γ4, γ5, and CLsO3);
-
N allocation and the O3 effect on N remobilisation (mleaf, cleaf, mstem, and cstem; Sect. 2.2.4, Table 1).
This sequential calibration prevents later adjustments caused by the interdependencies between parameters at different calibration stages. It was necessary to calibrate O3 effects on yield at the same time as the DM allocation and yield parameters, as O3 still influences yield, even in the low-O3 treatments. Vcmax,25 and Jmax,25 had been measured and, thus, were fixed to their experimentally measured values to limit the numbers of parameters to calibrate. Further, data on photosynthesis under different light concentrations allowed the determination of the rate of dark respiration; hence, we fixed the parameter controlling dark respiration in DO3SE-Crop. A combination of genetic algorithm and manual calibration was then used to calibrate chosen parameters to achieve the desired output variable. The genetic algorithm is not always the most suitable for model calibration, as it can give parameterisations that maximise the R2 but do not make sense physiologically. In cases where unrealistic parameterisations were given, a manual “by-eye” calibration process was also used. By varying one parameter at a time to understand its effect on a desired output, realistic parameterisations were chosen. For all calibrations, the R2 and RMSE values were used to assess the fit between observed data and simulations. Generally, 50 % of the combined data for all years for the low-O3 and very high O3 treatments were used in the calibration, with the remaining data used in the evaluation along with 100 % of the medium- and high-O3 treatment data for all years. A tabulation of the parameters calibrated for as well as the values they were calibrated to is given in Table S3 along with further details of the calibration process. Parameterisations relating to the newly developed N module are discussed and presented along with their equations in Appendix A.
The model was evaluated by calculating the RMSE and R2 values of the linear regression between observed and simulated values of phenology dates, grain DM, stem and leaf N, and grain N%. More emphasis is placed on the relative O3 impact on yield and quality than simulations of absolute values, as the aim of the study was to develop a model that can capture relative O3 impacts on crop quality.
3.3 Sensitivity analysis
Sensitivity analyses are used to determine the proportion of variance in a desired model output attributed to a variation in the model input (Saltelli et al., 2008). In this study, we use a sensitivity analysis to identify and rank the sensitivity of grain N to different plant processes simulated by DO3SE-Crop and the new N module. We identified three key mechanisms that can influence grain quality in the crop model: senescence onset, the end/duration of senescence, and the O3 interruption of N remobilisation of the leaf and stem. A preliminary sensitivity analysis was conducted to identify the key parameters in DO3SE-Crop influencing these processes. After reduction, four parameters which contribute the greatest to the variance of output variables representing these processes were identified for the sensitivity analysis (see Table 2). We use an extended Fourier amplitude sensitivity analysis (eFAST) to explain the variation in a chosen output variable attributed to varying selected input variables over a given range (Saltelli et al., 2008). The eFAST method is a commonly used method for sensitivity analyses and has previously been used by crop modellers to improve calibration (Silvestro et al., 2017; Vazquez-Cruz et al., 2014). The benefit of eFAST over other forms of sensitivity analysis is that it allows the interactions between model parameters to be quantified, it can sample the entire parameter space, and it is robust for non-linear relationships (Cariboni et al., 2007; Saltelli et al., 2008). These benefits make it a useful tool for complex systems such as crop models, where interacting, non-linear processes are common (Cariboni et al., 2007; Saltelli et al., 2008). The Python library SALib was used for all sensitivity analyses (Herman and Usher, 2019). The first sensitivity index, S1, quantifies the uncertainty in the output variable that is attributed to varying only that parameter. The total sensitivity index, ST, quantifies the uncertainty in the output variable that is attributed to varying a chosen parameter in combination with the other selected parameters (Saltelli et al., 2008). The range of values for the sensitivity analysis were taken from the theoretical maximum and minimum in the DO3SE-Crop model for those mathematical equations. The ranges for γ4 and γ5 were determined using the breakpoint method, as described by Pande et al. (2024b). For the leaf and stem remobilisation equations, the minimum gradient is zero, as this assumes no O3 effect on remobilisation for that plant part, and the maximum gradient was calculated by assuming that the other plant part has had as close to zero O3 impact on remobilisation as is mathematically possible in the equation formulation.
4.1 End-of-season grain DM and N% in grain, leaf, and stem
Figure 4 shows the evaluation of the grain DM as well as the grain, leaf, and stem N% simulations. Leaf and stem N data were only available in 2021; hence, the leaf and stem plots only use data from 2021. Additionally, for 2021, the plant did not put on any grain (the reason for which is unknown; Brewster et al., 2024); therefore, it was not possible to use the grain DM or grain N data for that year. However, as this was the only year of data for which stem and leaf N% measurements were available and the plants developed and flowered normally, the decision was made to proceed with these data for stem and leaf parameterisation. For 2016, the model captured the grain DM and the grain N% more precisely than for the year 2015. In 2015, the underestimate of grain DM resulted in an overestimation of grain N%. The stem and leaf N% at anthesis is more accurately simulated than at harvest. Harvest leaf N is overestimated by between 20 % and 120 %, while harvest stem N overestimations range from ∼40 % to 120 %. However, in both the stem and the leaf, N concentrations are overestimated at both anthesis and harvest, despite the calibration showing that the remobilisation of N from the leaf and stem under the differing O3 concentrations was well captured (see Fig. 2). The R2 values (calculated using scikit-learn, developed by Pedregosa et al., 2011) for grain DM and grain N% are negative, implying that the model simulations are worse than using the mean of the observed data (sklearn.metrics.r2_score; scikit-learn developers, 2024).
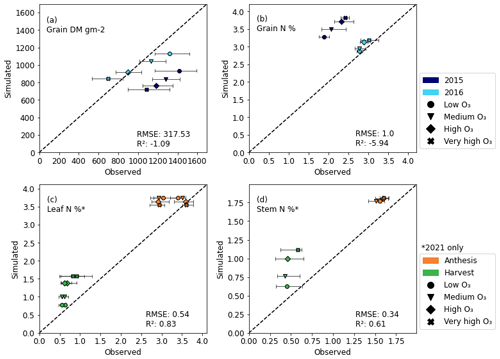
Figure 4Output of the DO3SE-Crop grain dry matter simulations at harvest (a). Output of the newly developed N module simulations of grain N% at harvest (b), leaf N% at anthesis and harvest (c), and stem N% at anthesis and harvest (d). The simulations shown are for the evaluation datasets only. The evaluation data available contained grain dry matter and N% for 2015 and 2016, whereas the plant did not put on any grain for 2021, so the latter data were not used for evaluation. Leaf and stem N% data were only available for 2021. The RMSE and R2 values of the observed vs. simulated data (not including error bars) are shown in the plots. The error bars represent the maximum and minimum of the experimental data, excluding outliers, for comparison with simulations. Panel (c) contains data for both the flag and second leaf. DO3SE-Crop and the new N module do not discriminate between these, so simulations of leaf N% for the flag and second leaf are the same.
The relative yield (RY) loss of the 2015 simulations is more well simulated than the 2016 simulations (Fig. 5). However, the R2 value is 0, meaning that the simulations work equally well as when using the mean of the observed data (sklearn.metrics.r2_score; scikit-learn developers, 2024). When considering grain quality, the increase in grain N% that occurs as O3 concentrations increase is simulated very effectively with an R2 value of 0.6 and an RMSE of 4.9 %. The percent decrease in the grain N content (grain N content measured in grams of N per square metre of crop) is not simulated as well as the change in the grain N concentration, as seen from the lower R2 value (0.3) and greater RMSE value (14.4). Further, Fig. 5c shows that the model had trouble capturing the large differences in the grain N content that occurred in 2016, compared with the much smaller differences in 2015.
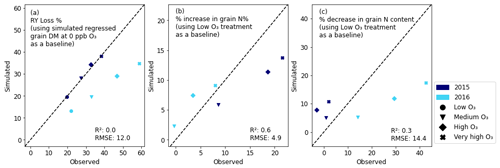
Figure 5Relative plots of the evaluation data: (a) the relative yield (RY) loss of the grain DM when using the grain DM at 0 ppb (obtained by regressing the simulated and observed yields) as a baseline; (b) the percent increase in grain N%; and (c) the percent decrease in grain N content (g m−2) when using the low-O3 treatment as a baseline. The RMSE and R2 values of the observed vs. simulated data (not including error bars) are shown in the plots.
4.2 Seasonal profiles of grain DM; grain N content; and N% in the grain, leaf, and stem
The profile of grain DM accumulation over time for all treatments is shown in Fig. 6 for 2015 and 2016; the corresponding profile for all years is given in Fig. S5 in the Supplement. From the profiles, we see that the accumulation of grain DM is initially slow; then, at days 200 and 192 for 2015 and 2016 respectively, there is a rapid increase. From the plots, we can see that the O3 effect on grain DM accumulation begins around 5–10 d post-anthesis.
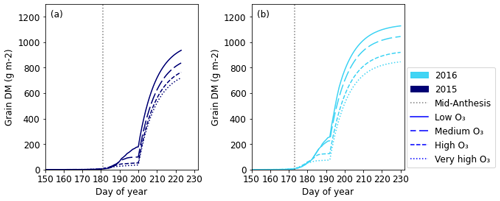
Figure 6The profile of simulated grain DM for 2015 (a) and 2016 (b). Mid-anthesis is indicated on the graph using a vertical dotted line, and the different line styles on the plot represent the simulations for the different O3 concentrations.
Figure 7 shows the change in simulated grain N% and grain N content as a function of time under the different O3 concentrations. As O3 concentrations increase, the absolute grain N content (in g m−2) (Fig. 7c and d) decreases for both years. Figure 7a and b appear to show a very sharp increase in grain N% as the grain starts filling with N after anthesis; then, after approximately 20 d, the N concentration starts to decrease. This rapid increase is due to a difference in the accumulation rates of grain DM and N in the model and is not representative of a plant process (see Fig. S1). Due to the large spike in initial N concentrations, it is difficult to see the effect of O3 on the end N concentrations. Therefore, the end profiles of the grain N concentrations are enlarged in the inset of Fig. 7 (Fig. 7a* and b*). Once magnified, it is possible to see the increase in grain N% with increasing O3 concentrations.
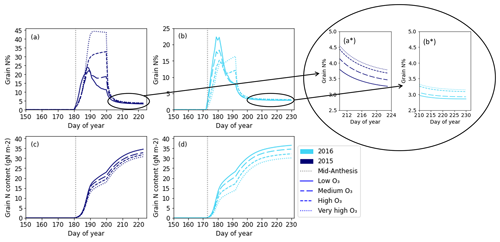
Figure 7The simulated grain N% for 2015 (a) and 2016 (b) and the simulated grain N content in grams per square metre for 2015 (c) and 2016 (d). The different line styles represent the different O3 concentrations. Mid-anthesis is indicated on the graph using a dashed vertical line for each year. The end points of panels (a) and (b) have been enlarged and are represented in subpanels (a*) and (b*) respectively so that differences at the end points can be distinguished.
Figure 8 shows the seasonal profile of leaf and stem N content and N% under differing O3 concentrations. Simulations of leaf and stem N% (Fig. 8a and b) are relatively constant at their target N concentration (see Appendix A for the target N explanation) until anthesis, as the model assumes no limitations to soil N uptake. The leaf and stem N contents increase in line with increasing biomass. Post-anthesis, the stem begins to transfer N to the grain; thus, the N concentration and content in the stem decrease (Fig. 8b and d). The remobilisation of N from the stem to the grain continues provided that the stem N concentration does not decrease below the minimum (Fig. 8b and d). The levelling off of the stem N% in Fig. 8b shows that the minimum stem N concentration for that O3 treatment has been reached. At higher O3 concentrations, the stem remobilises less N to the grains, and the final concentration of N in the stem is greater (Fig. 8b and d). Initially, leaf N% and content decreases faster in the simulated wheat plants experiencing greater O3 concentrations, as senescence begins earlier in these treatments (Fig. 8a and c). Then, at around 20 d after mid-anthesis, the O3 effect on remobilisation takes over, and the leaf N% and content are greater under increased O3 concentrations, due to the O3 inhibition of N remobilisation.
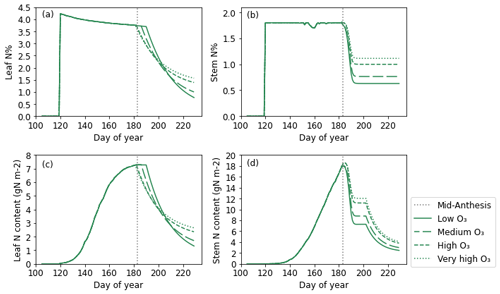
Figure 8The simulated (a) leaf and (b) stem N% values along with the simulated absolute N content in grams per square metre for the (c) leaf and (d) stem. Mid-anthesis is indicated on the graph using a vertical dotted line, and the different line styles on the plot represent the simulations for the different O3 concentrations. These plots are for the 2021 simulations only.
4.3 Sensitivity analysis results
Figure 9a and b illustrate the results of the sensitivity analysis study and show that more than 60 % of the variance in both grain N content and grain N% in simulations of all O3 treatments is explained by varying the parameter controlling the onset of leaf senescence. Absolute grain N content (g m−2) is more sensitive to variations in senescence onset than grain N%, and grain N% is more sensitive to variations in senescence end than grain N content. A threshold of ST>0.1 was used by Silvestro et al. (2017) to identify influential parameters in their sensitivity analysis. In this study, senescence onset and senescence end were found to be the only influential parameters on grain N content and grain N%. The effect of varying the leaf and stem remobilisation accounts for less than 2 % of the variance in both grain N% and grain N content for all O3 simulations and can be considered uninfluential. The interactions between the parameters were close to zero for the grain N%, as shown by a small difference between S1 and ST, whereas a stronger interaction was seen when considering absolute grain N as the output. The negligible ST terms for leaf and stem remobilisation imply that the larger ST observed for senescence onset in Fig. 9a must be due to the interaction between senescence onset and end. Onset of leaf senescence was simulated as occurring 15, 11, and 12 d earlier in the very high vs. low treatments for 2015, 2016, and 2021 respectively.
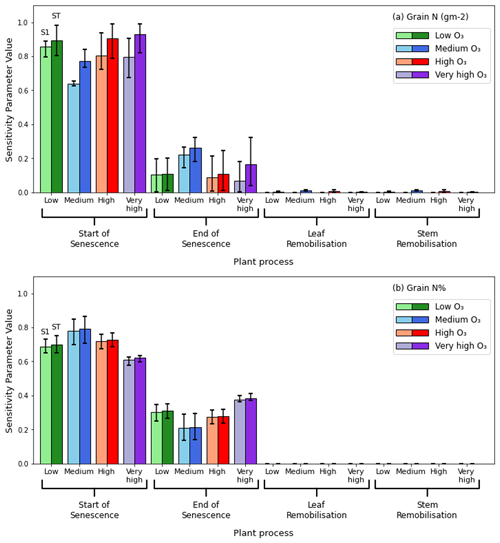
Figure 9Results of the sensitivity analysis. Lighter bars represent the first sensitivity index, S1, and the darker bars represent the total sensitivity index, ST. The different colours represent the different O3 treatments and, for clarity, are also indicated on the x axis. Braces group together the results of the sensitivity analysis for a particular plant process. The single model parameter chosen to represent each plant process in this analysis is described in Sect. 3.3. This graph shows the averaged results for the 3 different years. The coloured bars represent the mean value of the sensitivity index for all 3 years, while the error bars represent the maximum and minimum values for that sensitivity index achieved in the runs for the 3 years. Panel (a) shows the results of the sensitivity analysis when considering the absolute grain N content (in g N m−2) as the output parameter. Panel (b) shows the results of the sensitivity analysis when considering the percentage of N in the grain as the output parameter (100 g N g DM−1).
5.1 Evaluation of grain DM simulations
5.1.1 Grain DM simulations at harvest
The relative yield (RY) loss of the wheat in 2015 was more accurately simulated than in 2016, despite the grain DM being more well simulated in 2016 than in 2015 (Figs. 4a and 5a). The variability in whether grain DM or RY loss is more accurately simulated occurs because it is not possible to calibrate grain DM independently of the O3 effect on yield loss, due to overnight O3 concentrations of >20 ppb in the solar domes, which give a pollutant effect on yield even in the lowest-O3 treatment. Subsequently, there is a trade-off between achieving a greater accuracy in either grain DM or RY loss simulations, as the current model construct was not able to capture both at the same time. In this study, the decision was made to give priority to greater accuracy on the relative O3 effect on yield so that we could better test our simulations of the relative effect of O3 on grain quality. Further, the splitting of data for calibration and evaluation was randomised. The randomisation resulted in the calibration simulations for 2015 having a slightly lower grain DM; hence, the grain DM for 2015 in the evaluation simulations was underestimated.
5.1.2 Seasonal profile of grain DM accumulation
Grain DM accumulation profiles follow an approximate sigmoid shape, with a “bump” in the grain DM profile occurring when the stem begins to allocate DM to the grain (Fig. 6a and b and Fig. S5). Under higher O3 concentrations, grain DM is reduced ∼5–10 d after mid-anthesis. While profiles of grain DM accumulation have not yet been studied for O3, they have been studied for other stressors, such as drought. Given that both drought stress and O3 damage are mediated by ROS, we can expect their effects on the seasonal profiles of DM and N to be similar, where the stresses occur continuously throughout the growing season (Emberson et al., 2018; Khanna-Chopra, 2012). Liu et al. (2020) found that drought-stressed wheat initially had a higher grain weight than well-watered wheat 7 d after anthesis; however, by 28 d, well-watered wheat had surpassed drought-stressed wheat with respect to weight. Similarly, Zhang et al. (2015) found that the grain weight of drought-stressed wheat was higher 12–20 d after anthesis; however, by 36 d, it had been surpassed by well-watered wheat. In maize, Guo et al. (2021) found a decrease in kernel fresh weight under drought stress 10 d after anthesis, with the effect consistent to the end of the study, as in the present grain DM profiles for wheat. However, Liu et al. (2020) and Zhang et al. (2015), who did study wheat, did not observe a consistently lower grain DM for the drought-stressed wheat, as observed in this study (Fig. 6). Nevertheless, their profiles were based on individual grains (Liu et al., 2020) and the thousand-grain weight of the four main spikes (Zhang et al., 2015). Neither of those values take into consideration the reduced grain number per plant that occurs under drought stress (Ben Mariem et al., 2021). Reduced grain numbers are also observed under O3 stress (Broberg et al., 2015). Therefore, a consistently lower grain DM profile when presented as the grain DM per square metre (as in this study) may be expected for increasing levels of stress.
Together, these data suggest that differences in grain DM accumulation under drought stress are evident as early as 7–10 d post-anthesis. Therefore, it is not unreasonable for our simulations to show a decrease in grain DM accumulation in O3-exposed wheat 5–10 d after mid-anthesis. Further, considering the grain DM per square metre, we may expect to see a consistently lower grain DM for plants experiencing greater O3 stress.
5.2 Evaluation of grain N simulations
5.2.1 Grain N simulations at harvest
Harvest grain N% is simulated well for 2016, when the grain DM is more closely captured (Fig. 4a and b). However, in 2015, when the grain DM is underestimated, the grain N% is overestimated. The overestimation of grain N% is reduced when the observed, rather than simulated, grain DM values are used to calculate grain N% (data not shown). This suggests that the newly developed N module can simulate the absolute grain N% under differing O3 treatments accurately, provided that the grain DM is simulated well.
The model more successfully captured the increase in the grain N% (100 g N g DM−1) under elevated-O3 conditions compared with the decrease in the grain N content (g N m−2) (Fig. 5b and c). The model was calibrated to grain N%, as the response of grain N% to O3 was more consistent between years than grain N content. Between the 2 years of data, there were large differences in the grain N content of the wheat at harvest, the reason for which is unclear, although it could partially be due to the differences in grain DM between the 2 years. As the grain N content was so different between the 2015 and 2016 datasets for all treatments, the model struggled to match both years when simulating the decrease in the grain N content. Additionally, the interdependence between grain DM and N meant that, in the calibration, changing one of them subsequently changed grain N%, making the calibration process more difficult (see Fig. S1). If the wheat in 2021 had put on grain, the grain N measurements would have been invaluable in determining which of the years (2015 or 2016) had the more common response of grain N to O3 for the Skyfall cultivar. Having at least three datasets for model calibration allows outliers to be more easily identified, and more physiologically representative data selected for calibration.
5.2.2 Seasonal profile of grain N accumulation
The seasonal grain N content profiles (Fig. 7c and d) match well with profiles seen in experimental work (Bertheloot et al., 2008; Nagarajan et al., 1999) and that can be constructed from available wheat N data (see Fig. S2). There is a bump in our simulated grain N content (Fig. 7c and d) which matches that of our simulated grain DM profile (Fig. 6a and b). The bump occurs because available N for grain is increased as stem DM decreases (due to remobilisation). It is not thought that this bump represents the actual rate by which the grain fills with N; rather, it is believed that it occurs due to the complex interconnections between leaf senescence, leaf N remobilisation, stem N remobilisation, and required N for the grains in the model.
The seasonal profiles of grain N content (Fig. 7c and d) show that O3 effects are distinguishable ∼10 d after mid-anthesis, with the model simulating lower rates of accumulation of grain N content when wheat is exposed to higher concentrations of O3. To our knowledge, no studies describe how the seasonal profile of grain N content accumulation varies under differing O3 concentrations. However, again using the fact that both O3 and water stress are mediated by ROS (Emberson et al., 2018; Khanna-Chopra, 2012), the response of grain N accumulation under water stress, compared with a well-watered treatment, could be comparable to grain N accumulation under different O3 concentrations. Nagarajan et al. (1999) found varying responses with respect to grain N accumulation between cultivars for water-stressed wheat, with some showing similar profiles to the control treatment and others experiencing a negative impact on both total N and the rate of accumulation compared with the control.
In a study measuring N accumulation with and without irrigation, Panozzo and Eagles (1999) found that the irrigated wheat accumulated N more slowly per grain, but it continued accumulating for longer and ultimately had a higher N content per grain. In the current study, our simulations show that the grains accumulated a lower N content under O3 exposure, which occurs due to the explicit modelling of a reduced remobilisation rate under higher O3 concentrations (Fig. 7c and d and Eqs. 2 and 3).
Seasonal profiles of grain N% (Fig. 7a and b) under different O3 treatments are less consistent than the grain N content profiles. Initially, grain N% increases rapidly, then levels off and decreases. Using data from Nagarajan et al. (1999), who measured grain N and carbon (C) of wheat cultivars at three time points post-anthesis under water-stressed and control conditions, we constructed time profiles of grain N% (see the Supplement, Fig. S4). The constructed profiles generally show an initial increase in grain N% that tends to decrease or level off, as in the present study. Panozzo and Eagles (1999) measured grain weight and N for seven time points post-anthesis, again allowing a profile of grain N% under dry and irrigated treatments to be constructed. The profiles show that the wheat grain N% decreases from 2.5 % and levels off to 2 % approximately 21 d after anthesis, matching the shape of our grain N% profile, although our peak grain N% is far too large at 15 %–45 % (Panozzo and Eagles, 1999). In our study, the reason for the large peak in grain N% is that N accumulation occurs more rapidly after anthesis than grain DM, leading to a greater concentration of grain N (see Fig. S1). If the initial rate of grain fill with N was slower or grain DM accumulation was faster, our grain N% profiles would likely match the shape and magnitude of those constructed by Panozzo and Eagles (1999) or Nagarajan et al. (1999). It would be helpful to have measurements of grain N content and DM for multiple time points after anthesis under varying O3 treatment conditions, to develop a temporal understanding of the grain N% response to O3 for model parameterisation.
In 2015, grain N% is already higher in the elevated-O3 treatments around 10 d after anthesis. In contrast, in 2016, grain N% is initially higher in the lower-O3 treatments, and then, around 20 d after mid-anthesis, the elevated-O3 treatments show a higher grain N% (Fig. 7a and b). The difference in the response of grain N% is due to the differences in the simulated rates of grain N and grain DM accumulation between the years. In the grain N% profiles constructed from Panozzo and Eagles (1999) (see Fig. S3), the irrigated (non-stressed) wheat had a lower final grain N% than the dry (stressed) treatment, and the difference was clear to see from >10 d post-anthesis. Toward harvest, the effect of increased O3 concentrations on increasing the grain N% is seen approximately 20 d after anthesis, when the initial sharp increase levels off and the concentrations reach more reasonable values.
5.3 Evaluation of stem and leaf N simulations
5.3.1 Stem and leaf N simulations at anthesis and harvest
The anthesis leaf and stem N% values are captured well by the model, but the effect of O3 on harvest leaf and stem N% is exaggerated (Fig. 4c and d). Although the differences between harvest leaf and stem N% were non-significant (Brewster et al., 2024), there appears to be a slight decrease in final leaf and stem N% under the medium-O3 treatment and a subsequent increase for O3 concentrations that were high or very high. A potential reason for the decrease under medium O3 concentrations could be an effect called hormesis, where a stressor initially induces a greater productivity in the plant and then, past a given threshold, has a negative effect (Agathokleous et al., 2019). While it could be argued that hormesis is present for the data of Brewster et al. (2024) in Fig. 2 of this study, there are only four O3 treatments; thus, it is not possible to parameterise the minimum point of the hormetic response. In contrast, Broberg et al. (2017) had five O3 treatments but did not observe a hormetic response; instead, they found the response to be linear. If future experimental work looks at the O3 impact on N remobilisation from the leaf and stem to the grain, it would be helpful to place a greater emphasis on O3 treatments between 30 and 60 ppb to determine a potential turning point at which higher O3 concentrations start to limit N remobilisation. This would enable parameterisation of a non-linear hormetic response for N remobilisation in wheat under O3 exposure.
5.3.2 Seasonal profiles of stem and leaf N accumulation
Our simulations of leaf and stem N content and N% over time (Fig. 8) show that they reach a peak before anthesis and decrease after anthesis, which is also shown by the stem and leaf N profiles constructed from available experimental data (see Fig. S2). The levelling off of the stem N content (Fig. 8d) profile at approximately 190 d is a result of the model construct in that no N was required for the grains from the stem at that point.
Currently, there are no data in the literature describing the effect of O3 on the leaf or stem N status in crop plants over the course of the growing season to compare with the present study (Fig. 8). However, one study by Bielenberg et al. (2002) did measure the variation in the stem and leaf N content over time in hybrid poplar exposed to elevated-O3 conditions. In plants receiving the same N treatment, increased O3 generally reduced the N content of the leaves and stem at each measurement point. Overall, the temporal profiles of the N content had the same shape regardless of O3 treatment (Bielenberg et al., 2002). In our study, the stem N content was greater at every stage post-anthesis for greater O3 concentrations due to the reduced remobilisation of nutrients under O3 exposure (Brewster et al., 2024). By contrast, the leaf N content was initially reduced in wheat experiencing greater O3 concentrations due to accelerated senescence, but the effect of the reduced remobilisation eventually outweighed the senescence effect, leading to a greater N content in O3-stressed wheat at harvest.
5.4 Suggestions for improving the representation of plant growth in DO3SE-CropN
It has been suggested that the reduced remobilisation of leaf and stem N under O3 exposure occurs as a result of reduced N use efficiency, as O3-induced accelerated senescence shortens the grain-filling period (Broberg et al., 2017, 2021). However, Brewster et al. (2024) found an increase in the residual flag leaf N concentration under O3 exposure, despite not finding a difference in senescence onset, suggesting that the accelerated senescence and subsequent reduced N remobilisation efficiency is not the only factor increasing the residual N concentration in plant parts. Some researchers have suggested that the increase occurs as defence proteins accumulate (Brewster et al., 2024; Sarkar et al., 2010). In the present study, we simulate the accelerated senescence that occurs under O3 exposure, and we model the reduced remobilisation using simple linear equations (Eqs. 2 and 3). Future work to understand the mechanism underpinning the increase in residual N in the stem and leaf would help refine the model to more accurately simulate plant processes.
Brewster et al. (2024) found that N fertilisation reduced the N left in the leaf and stem at harvest under O3 exposure, delaying senescence and protecting chlorophyll against O3 damage. The current model construct does not simulate this effect and assumes the plant receives optimum N. No N stress or extra fertilisation was considered. Future iterations of this model incorporating soil N processes could use the work of Brewster et al. (2024) to include N fertilisation and model the ameliorative effect of variable N fertilisation on O3 damage. Additionally, this first iteration of the model does not include any feedback of plant N status on photosynthetic or growth processes. Future research may want to consider the feedbacks between leaf N levels and photosynthetic rate, with higher leaf N increasing the photosynthetic rate, which could offset O3-induced reductions (Pilbeam, 2010). Researchers may also want to consider the influence of a higher leaf N in potentially delaying O3-induced early senescence onset (Martre et al., 2006; Nehe et al., 2020).
5.5 Sensitivity analysis results
The sensitivity analysis (Fig. 9a and b) showed that the effect of varying leaf and stem remobilisation contributed little, if at all, to variations in the grain N content or grain N% under the differing O3 treatments. Further, the most influential parameter on grain N content and grain N%, was senescence onset; this does not change regardless of the O3 treatment simulated. These results align with existing literature, which shows that a shorter duration for grain fill leads to less time for nutrient remobilisation, subsequently impacting grain quality (Havé et al., 2017). Therefore, it is expected that the senescence parameters would have a large influence on grain N. Further, as the onset of leaf senescence is the beginning of leaf N remobilisation to the grain, it has a larger influence on the grain N content and concentration than the end of leaf senescence (Havé et al., 2017). Therefore, for the Skyfall cultivar, under the O3 conditions simulated, senescence onset has greater influence on grain quality than O3 interruptions to remobilisation from an unspecified process. In our sensitivity analysis we observed a difference in the magnitude of S1 (the uncertainty in the output variable that is attributed to varying only that parameter) and ST (the uncertainty in the output variable that is attributed to varying a chosen parameter in combination with the other selected parameters) (Saltelli et al., 2008) between the different O3 treatments. The non-linear response is unsurprising given the complex and interconnected nature of crop modelling. However, it is not possible to determine whether this response is typical of wheat, as the present study considers data for only one cultivar and one location. If future work investigates multiple growing locations and wheat varieties and uncovers a similar response with respect to the sensitivity indices for the differing O3 concentrations, the underlying crop modelling processes could be investigated to determine the reason for this effect.
5.6 Implications of sensitivity analysis results for growers
The sensitivity analysis results pose interesting questions regarding the importance of cultivars having an earlier or delayed senescence for maximising grain protein under O3 exposure. Generally, a delayed onset of leaf senescence decreases the grain protein content, as there is a delay to the start of N remobilisation from the leaves to the grain (Havé et al., 2017; Sultana et al., 2021). A delayed senescence also decreases the grain protein percentage, due to a reduction in the grain N content and an increased length of photosynthetic activity that increases grain yield (Bogard et al., 2011; Nehe et al., 2020; Sultana et al., 2021). However, this is not always the case, as there are several interacting effects from the environment (e.g. temperature, drought, O3, or pathogens), N application, and gene expression (Bogard et al., 2011; Gaju et al., 2014; Nehe et al., 2020; Sultana et al., 2021; Zhao et al., 2015). Stay-green cultivars have previously been identified as allowing plants to maintain their photosynthetic capacity under heat and drought stress conditions (Kamal et al., 2019). As O3 damage is mediated by ROS, similar to the damage from heat and drought stress (Khanna-Chopra, 2012), it is expected that stay-green cultivars will provide a similar increased yield for O3-exposed wheat by delaying the stress-induced early senescence onset. The impact of using stay-green cultivars on wheat grain protein under O3 stress conditions is yet to be investigated. However, a decrease in the grain protein content under O3 exposure may be due to the delayed onset of remobilisation (Havé et al., 2017; Sultana et al., 2021). Zhao et al. (2015) genetically modified wheat plants to investigate the response of senescence, grain yield, and grain N% when a senescence-delaying gene was over-expressed. In the genetically modified wheat, the grain yields were similar to the control, but the grain N% was increased (Zhao et al., 2015). Therefore, stay-green wheat cultivars that do not experience a grain protein penalty should be considered by breeders and investigated with respect to their suitability under differing O3 concentrations.
5.7 Potential model applications
Simulations of the crop N content can be easily converted into protein through the use of a simple conversion factor or via linear regressions if N or water stress conditions are present that have not been previously accounted for in the simulation (Liu et al., 2018; Mariotti et al., 2008; Tkachuk, 1969). Therefore, the grain protein percentage can easily be obtained from grain N%. Further, by using the protein percentage, amino acid concentrations can be simulated using regressions developed by Liu et al. (2019), allowing the model to be extended to simulate protein quality. In addition, building on the work of Broberg et al. (2015), it would also be possible to link changes in N content under O3 exposure to changes in other grain mineral contents, such as zinc, magnesium, and starch. Such relations would be simple to integrate given that the model already simulates O3 effects on N and that there seem to be similarities between the effect of O3 on N and the effect of O3 on other minerals (Broberg et al., 2015). These improvements would increase the nutritional relevance of the model.
The DO3SE-Crop model takes inputs of temperature, photosynthetic photon flux density, CO2 concentration, and precipitation. There is also a built-in soil moisture module that can simulate the effect of water stress on stomatal O3 flux (Büker et al., 2012). Because of these features, the DO3SE model is an ideal candidate for simulating the combined effects of O3 pollution and climate change on crop yields. With the newly developed N module, this would allow the user to determine how O3 pollution and climate change effects may interact to affect crop yield, quantity, and quality and, in turn, dietary nutrition.
In summary, this study identified the key mechanisms for modelling N in wheat as soil N uptake, partitioning of N taken up from the soil between the leaf and stem, remobilisation of N from the leaves and stem to the grain, and the impact of O3 on N remobilisation. Using these key processes, a new model was developed that can be used in combination with the existing O3 deposition crop model, DO3SE-Crop, to simulate the O3 impact on wheat N. The newly developed model is the first to simulate the effect of O3 on N in any plant species. After evaluation, a sensitivity analysis was applied to the model to identify the key plant process that affects grain quality under O3 exposure. It was found that O3-induced early senescence onset was the key plant process affecting grain quality under O3 exposure, regardless of the O3 treatment. We recommend that breeders focussing on stay-green cultivars aim to develop cultivars that do not suffer a protein penalty. If such cultivars can maintain their yield and quality under abiotic stresses, they may also be tolerant to O3 in terms of both yield and crop quality; testing this would be beneficial to further understand the implications of O3 on global food and nutritional security.
Detailed below are the equations and references for the N module of the DO3SE-Crop N module. We found the approach of Wang and Engel (2002) to writing up their crop model to be very effective; thus, we take inspiration from their approach and (1) present a description of the processes and references in the text and (2) tabulate the specific equations with their corresponding references in each section. The values of parameters used in the module and their corresponding sources are tabulated also. For a full mathematical description of the phenology, photosynthesis, carbon allocation, and O3 damage processes of DO3SE-Crop, the reader is referred to Pande et al. (2024a).
At the current stage of development, the N module is not integrated within DO3SE-Crop. It requires the output file from a DO3SE-Crop run in order to perform the N simulations.
A1 N uptake
Pre-anthesis, a maximum N uptake (NUPpre,max, in ) is defined (Soltani and Sinclair, 2012). The actual N uptake by the crop pre-anthesis (NUPpre, in ) is calculated using the work of Soltani and Sinclair (2012). Daily N uptake is the sum of the N associated with the increase in the LAI that day (LAIgrowth, in m2 d−1); the N associated with the increase in stem dry matter that day (DWstem_grth, in ); and the N deficit, which is the difference between the target stem N concentration and the current stem N concentration (Nstem,deficit, in ).
Here, [Nstem,target] is the target N concentration of the stem and [Nleaf,target] is the target N concentration of the leaf (Soltani and Sinclair, 2012). The Nstem,deficit is defined as the difference between the target stem N for its mass and its current N content (Nstem):
where DWstem is the dry weight of the stem (in g m−2). If NUPpre>NUPpre,max, we set NUPpre=NUPpre,max; otherwise, the crop takes up N equal to NUPpre.
Post-anthesis, N uptake is a function associated with the capacity of the stem to hold N. The basis for this equation is taken from SiriusQuality (Martre et al., 2006). The potential N uptake post anthesis (NUPpost,pot, in ) is calculated as follows:
where NUPpost,max is the maximum N uptake post-anthesis, Tgrainfill is the thermal time (in degree days) between the end of grain filling (i.e. harvest in the present model) and the start of anthesis, and T is the current thermal time (in degree days) since anthesis. Subsequently, the stem's target capacity to hold N is calculated and compared to the current amount of N stored in the stem. If the current stem N, Nstem (in g N m−2), exceeds the target capacity, no N is taken up that day (Eq. A4).
If the current stem N is less than the target capacity, the N taken up is equal to the minimum of the stem's current capacity and the potential uptake.
A2 N partitioning to the stem and leaf
Pre-anthesis, the equations describing how the uptake of N is split between the leaf and stem are based on the work of Soltani and Sinclair (2012), and no N is allocated to the grains at this time. The stem and leaf have a defined target and minimum N concentration that can be calibrated for different wheat cultivars. In Soltani and Sinclair (2012), both the target and minimum stem and leaf N concentrations are constants. In this study, the minimum N concentrations of the leaf and stem are variable based on the O3 concentrations. This allows a reduction in remobilisation of N from the leaf and stem to the grain, as found by Brewster et al. (2024), under higher O3 concentrations. It is easiest to see the structure of the N partitioning code in Fig. A1. The write-up of pre-anthesis N partitioning to the leaves and stem is split into two sections based on whether the stem is experiencing an N deficit (i.e. stem N is less than its minimum).
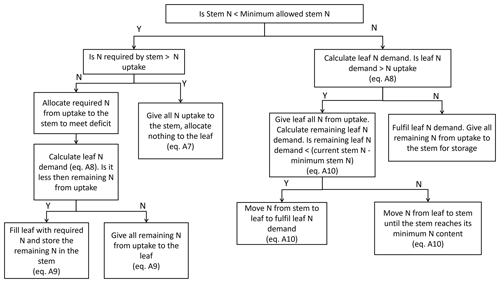
Figure A1Figure showing the allocation of N to the stem and leaf before anthesis. Y and N represent “yes” and “no” respectively. Equation definitions are referenced appropriately. Where equation numbers are not indicated, additional information can be found in the text. Equations and the model structure for N allocation are based on those of Soltani and Sinclair (2012) and modified for the purposes of this study.
Table A2Default and present parameterisations for the N module along with citations corresponding to where the original default value was obtained.
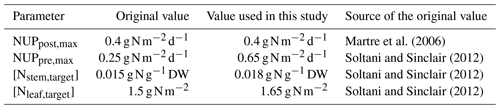
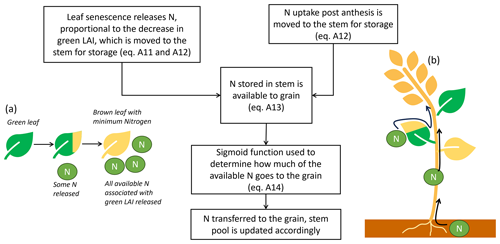
Figure A2The allocation of N to the wheat grains post-anthesis, with equations indicated appropriately. Panel (a) is a visual representation of a leaf releasing N as the leaf area senesces. Panel (b) shows the three locations where N is transferred to the grain from post-anthesis N uptake, N stored in the stem, and senescing leaf area.
Firstly, the current stem N concentration is compared to the minimum allowed stem N concentration, [Nstem,min]. If the current stem N concentration is less than the minimum stem N concentration, then there is a deficit, and the processes of Sect A2.1 are followed. If the current stem N concentration is not less than the minimum stem N concentration, i.e. there is no N deficit, then the processes of Sect A2.2 are followed.
A2.1 If the current stem N concentration is less than the minimum stem N concentration (N deficit)
If the current stem N concentration is less than the minimum concentration, the allocation of N to the stem is prioritised.
where Nreq,stem is the N required by the stem to meet its deficit (in g N m−2). If Nreq,stem is greater than the amount of N taken up that day (NUP, in g N m−2), the N entering the stem pool (Ninto,stem, in g N m−2) is capped at the amount of N taken up.
Equation (A7) has been modified from Soltani and Sinclair (2012), who allowed leaf area to senesce and provide the stem with N if there was not enough to meet the stem's N demand. The senescing of leaf area to provide N was removed in the current iteration of DO3SE-CropN. Currently, there are no interdependencies between the N module and DO3SE-Crop. The DO3SE-Crop model runs, and the N module is then applied to the output of DO3SE-Crop to calculate crop N. It is therefore not possible to senesce leaf area to provide N in the current version of the N module, as the leaf area has already been determined in DO3SE-Crop.
If there is enough N to meet the deficit of the stem, Ninto,stem=Nreq,stem and Nleaving,stem=0. Then, the N required to maintain leaf area growth at its target N concentration (Nreq,leaf, in g N m−2) is calculated using Eq. (A8).
If Nreq,leaf can be fulfilled by the N remaining after maintaining stem growth at the minimum N concentration, N is partitioned to the leaves and any leftover N is partitioned to the stem for storage. If the leftover N cannot fulfil the demand of the leaf, the remaining N from uptake is partitioned to the leaves (Soltani and Sinclair, 2012).
A2.2 If the current stem N concentration is not less than the minimum stem N concentration (no N deficit)
If the stem N is not below its minimum, N is first allocated to the leaves. The N required by the leaves is calculated in accordance with Eq. (A8). If the N required by the leaves is less than the N uptake that day, the N required by the leaves is transferred to them: Ninto,leaf=Nreq,leaf and Nleaving,leaf=0. Subsequently, the remaining N from uptake is transferred to the stem, and Nleaving,stem=0
If the leaves required more N than was taken up by the crop, the extra demand is fulfilled using some of the stem N. The N from the stem is removed only until the stem reaches its minimum concentration.
In Eq. (A10), the minimum function ensures that the stem N does not fall below its minimum. In the scenario that the leaves required more N than taken up by the crop, Nleaving,leaf=0 and Ninto,stem=0.
A3 N partitioning to the grain
After anthesis, the grain begins to fill with N and the model equations change to reflect that the priority is grain fill, not leaf area expansion or growth. After anthesis Ninto,leaf=0 always. As leaf area senesces, N is released:
where LAIyesterday and LAItoday represent the respective values of the leaf area index yesterday and today as calculated in DO3SE-Crop. N released from the leaf and N taken up by the plant post-anthesis are added to the stem N pool for storage, as they would have to travel through the stem to reach the grains (Sanchez-Bragado et al., 2017). The stem N is then updated accordingly:
Not all of the N in the stem is available to be transferred to the grain, as the stem has a minimum N concentration. Therefore, the available stem N (often referred to as the labile pool in other crop models) is calculated as follows:
The fraction of Navailable that is transferred to the grain each day is determined through a sigmoid function. The sigmoid was chosen as it uses only two extra parameters and, thus, allows the start and rate of grain fill with N to be customised without the addition of much complexity. The N in the Navailable pool can be transferred to the grain or can remain as part of the stem. The sigmoid determines the fraction of N going to the grain from Navailable. The fraction increases as the plant develops. Multiplying Navailable by the sigmoid function gives the amount of N transferred to the grain that day. αN and βN are the coefficients that customise the respective onset and rate of grain fill. They can be calibrated to customise grain fill.
Once N has been transferred to the grain, the leaf and stem N pools are decreased according to their N availability to account for this transfer. Figure A2 diagrammatically represents the grain-filling process, while Fig. A3 shows an illustration of the sigmoid function with varying parameterisations to control grain fill.
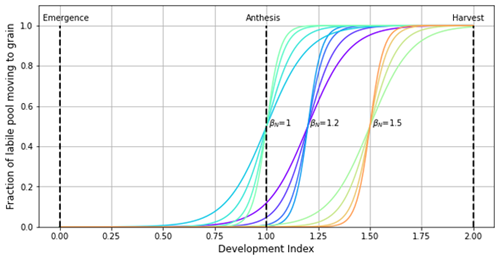
Figure A3Example parameterisations of αN and βN for customising the sigmoid function describing the fraction of labile N moving to the grain. For each value of βN, αN values of 10, 15, 23, and 30 are plotted to show how the rate, start, and end of grain fill can be customised. Although βN=1 has been plotted to illustrate how the shape of the function can be customised, care should be taken if using this value, as it can imply that grain filling with N begins midway between emergence and anthesis for lower values of αN. Sensible parameterisations should be chosen.
A4 Ozone impact on N remobilisation
The effect of O3 on grain N has been described in the main body of this study; however, it will be discussed with relation to the equations and how they link with the previously described model structure here. Broberg et al. (2017) and Brewster et al. (2024) found that the fraction of N in the leaf and stem at harvest, compared with that present at anthesis, increased as O3 concentrations increased. In essence, the remobilisation of N from the leaves and stem to the grains decreased. A regression of the combined data from Broberg et al. (2017) and Brewster et al. (2024) representing remobilisation is shown in Fig. 2 of the main body of the study.
To represent the reduced remobilisation under increased O3 exposure, the remobilisation regression was used to calculate new values of [Nstem,min] and [Nleaf,min] under O3 exposure. The fraction of N remaining in the leaf and stem at harvest that was there at anthesis (fNremob) is approximated by the following:
Equation (A15) assumes the anthesis leaf and stem N concentration is the same as the target N concentration, as the first iteration of the model assumes no N limitation. Additionally, for calibration purposes, the “harvest” N concentration was assumed to be the same as the minimum N concentration. In the model itself, this would not occur, as not all available N will be remobilised due to O3 effects on senescence. However, for calibration purposes, Eq. (A15) is a good approximation. Using Eq. (A15), minimum values of leaf and stem N for the differing O3 treatments were manually altered for each O3 concentration, to estimate values for the N remobilisation (red markers in Fig. 2) that form a linear regression fitting inside the 95 % CI. The leaf and stem minimum N concentrations of each red marker were extracted. The minimum leaf N concentration and the minimum stem N concentration were regressed separately with M12 to give regressions describing how the minimum N concentration in the plant part varies with O3 concentration:
A5 Calculating leaf N concentration
In the DO3SE-Crop model, green leaves are photosynthesising leaves, whereas brown leaves are senesced leaves. In the N module, the N associated with the senesced LAI is released (as described in Sect. 1.3). Leaf area that has senesced will have the minimum leaf N concentration. Green-leaf area that has not senesced will have a higher N concentration. An average leaf N concentration can be calculated by taking the absolute N in both green and brown leaves and dividing it by the total (green + brown) leaf DM.
An open version (version 4.39.11) of the DO3SE-Crop model, as used in the present study, can be found at https://doi.org/10.5281/zenodo.11620482 (Bland, 2024), and version 1.0 of the N module for DO3SE-Crop can be found at https://doi.org/10.5281/zenodo.13771475 (Cook, 2024).
Data from Brewster et al. (2024), Broberg et al. (2023), and Osborne et al. (2019) were used, with additional data from these studies provided by Felicity Hayes (fhay@ceh.ac.uk). Due to data ownership considerations, readers are asked to contact Felicity Hayes directly for access to the required data.
The supplement related to this article is available online at: https://doi.org/10.5194/bg-21-4809-2024-supplement.
JC, LE, FH, ST, CB, and HP: conceptualisation; JC, FH, and CB: data curation; JC: formal analysis; JC, LE, FH, ST, CB, and HP: methodology; JC, SB, PP, NB, and LE: software – N module and DO3SE-Crop, DO3SE-Crop, DO3SE-Crop, DO3SE-Crop, and DO3SE-Crop respectively; LE, FH, and ST: supervision; JC: visualisation and writing – original draft preparation; JC, LE, FH, ST, CB, HP, NB, PP, and SB: writing – review and editing.
The contact author has declared that none of the authors has any competing interests.
Publisher's note: Copernicus Publications remains neutral with regard to jurisdictional claims made in the text, published maps, institutional affiliations, or any other geographical representation in this paper. While Copernicus Publications makes every effort to include appropriate place names, the final responsibility lies with the authors.
This article is part of the special issue “Tropospheric Ozone Assessment Report Phase II (TOAR-II) Community Special Issue (ACP/AMT/BG/GMD inter-journal SI)”. It is a result of the Tropospheric Ozone Assessment Report, Phase II (TOAR-II, 2020–2024).
This research has been supported by the Natural Environment Research Council (grant no. NE/S00713X/1).
This paper was edited by Paul Stoy and reviewed by Stefano Galmarini and one anonymous referee.
Agathokleous, E., Kitao, M., and Calabrese, E. J.: Hormesis: A Compelling Platform for Sophisticated Plant Science, Trends Plant Sci., 24, 318–327, https://doi.org/10.1016/j.tplants.2019.01.004, 2019.
Baqasi, L. A., Qari, H. A., Al Nahhas, N., Badr, R. H., Taia, W. K., El Dakkak, R., and Hassan, I. A.: Effects of low concentrations of ozone (O3) on metabolic and physiological attributes in wheat (Triticum aestivum L.) pants, Biomed. Pharmacol. J., 11, 929–934, https://doi.org/10.13005/bpj/1450, 2018.
Barraclough, P. B., Lopez-Bellido, R., and Hawkesford, M. J.: Genotypic variation in the uptake, partitioning and remobilisation of nitrogen during grain-filling in wheat, Field Crop. Res., 156, 242–248, https://doi.org/10.1016/j.fcr.2013.10.004, 2014.
Ben Mariem, S., Soba, D., Zhou, B., Loladze, I., Morales, F., and Aranjuelo, I.: Climate Change, Crop Yields, and Grain Quality of C3 Cereals: A Meta-Analysis of [CO2], Temperature, and Drought Effects, Plants, 10, 1–19, https://doi.org/10.3390/plants10061052, 2021.
Bertheloot, J., Andrieu, B., Fournier, C., and Martre, P.: A process-based model to simulate nitrogen distribution in wheat (Triticum aestivum) during grain-filling, Funct. Plant Biol., 35, 781–796, https://doi.org/10.1071/FP08064, 2008.
Bielenberg, D. G., Lynch, J. P., and Pell, E. J.: Nitrogen dynamics during O3-induced accelerated senescence in hybrid poplar, Plant Cell Environ., 25, 501–512, https://doi.org/10.1046/j.1365-3040.2002.00828.x, 2002.
Bland, S.: SEI-DO3SE/pyDO3SE-open: V4.39.11 (v4.39.11), Zenodo [code], https://doi.org/10.5281/zenodo.11620482, 2024.
Bogard, M., Jourdan, M., Allard, V., Martre, P., Perretant, M. R., Ravel, C., Heumez, E., Orford, S., Snape, J., Griffiths, S., Gaju, O., Foulkes, J., and Le Gouis, J.: Anthesis date mainly explained correlations between post-anthesis leaf senescence, grain yield, and grain protein concentration in a winter wheat population segregating for flowering time QTLs, J. Exp. Bot., 62, 3621–3636, https://doi.org/10.1093/jxb/err061, 2011.
Brewster, C.: Ground level ozone and wheat: an exploration of effects on yield, interactions with nitrogen, and potential sources of sensitivity and tolerance, 170 pp., 2023.
Brewster, C., Fenner, N., and Hayes, F.: Chronic ozone exposure affects nitrogen remobilization in wheat at key growth stages, Sci. Total Environ., 908, 168288, https://doi.org/10.1016/j.scitotenv.2023.168288, 2024.
Broberg, M. C., Feng, Z., Xin, Y., and Pleijel, H.: Ozone effects on wheat grain quality – A summary, Environ. Pollut., 197, 203–213, https://doi.org/10.1016/j.envpol.2014.12.009, 2015.
Broberg, M. C., Uddling, J., Mills, G., and Pleijel, H.: Fertilizer efficiency in wheat is reduced by ozone pollution, Sci. Total Environ., 607–608, 876–880, https://doi.org/10.1016/j.scitotenv.2017.07.069, 2017.
Broberg, M. C., Högy, P., Feng, Z., and Pleijel, H.: Effects of elevated CO2 on wheat yield: Non-linear response and relation to site productivity, Agronomy, 9, 1–18, https://doi.org/10.3390/agronomy9050243, 2019.
Broberg, M. C., Xu, Y., Feng, Z., and Pleijel, H.: Harvest index and remobilization of 13 elements during wheat grain filling: Experiences from ozone experiments in China and Sweden, Field Crop. Res., 271, 108259, https://doi.org/10.1016/j.fcr.2021.108259, 2021.
Broberg, M. C., Hayes, F., Harmens, H., Uddling, J., Mills, G., and Pleijel, H.: Effects of ozone, drought and heat stress on wheat yield and grain quality, Agr. Ecosyst. Environ., 352, 108505, https://doi.org/10.1016/j.agee.2023.108505, 2023.
Büker, P., Morrissey, T., Briolat, A., Falk, R., Simpson, D., Tuovinen, J.-P., Alonso, R., Barth, S., Baumgarten, M., Grulke, N., Karlsson, P. E., King, J., Lagergren, F., Matyssek, R., Nunn, A., Ogaya, R., Peñuelas, J., Rhea, L., Schaub, M., Uddling, J., Werner, W., and Emberson, L. D.: DO3SE modelling of soil moisture to determine ozone flux to forest trees, Atmos. Chem. Phys., 12, 5537–5562, https://doi.org/10.5194/acp-12-5537-2012, 2012.
Cariboni, J., Gatelli, D., Liska, R., and Saltelli, A.: The role of sensitivity analysis in ecological modelling, Ecol. Model., 203, 167–182, https://doi.org/10.1016/j.ecolmodel.2005.10.045, 2007.
Chang-Espino, M., González-Fernández, I., Alonso, R., Araus, J. L., and Bermejo-Bermejo, V.: The effect of increased ozone levels on the stable carbon and nitrogen isotopic signature of wheat cultivars and landraces, Atmosphere-Basel, 12, 1–25, https://doi.org/10.3390/atmos12070883, 2021.
Chenu, K., Porter, J. R., Martre, P., Basso, B., Chapman, S. C., Ewert, F., Bindi, M., and Asseng, S.: Contribution of Crop Models to Adaptation in Wheat, Trends Plant Sci., 22, 472–490, https://doi.org/10.1016/j.tplants.2017.02.003, 2017.
Cho, K., Tiwari, S., Agrawal, S. B., Torres, N. L., Agrawal, M., Sarkar, A., Shibato, J., Agrawal, G. K., Kubo, A., and Rakwal, R.: Tropospheric ozone and plants: Absorption, responses, and consequences, Springer, New York, NY, 61–111, https://doi.org/10.1007/978-1-4419-8453-1_3, 2011.
Cook, J.: JoCook1997/DO3SE-CropN: Initial release (v2.0), Zenodo [code], https://doi.org/10.5281/zenodo.13771475, 2024.
Dai, L., Hayes, F., Sharps, K., Harmens, H., and Mills, G.: Nitrogen availability does not affect ozone flux-effect relationships for biomass in birch (Betula pendula) saplings, Sci. Total Environ., 660, 1038–1046, https://doi.org/10.1016/j.scitotenv.2019.01.092, 2019.
Emberson, L. D., Büker, P., Ashmore, M. R., Mills, G., Jackson, L. S., Agrawal, M., Atikuzzaman, M. D., Cinderby, S., Engardt, M., Jamir, C., Kobayashi, K., Oanh, N. T. K., Quadir, Q. F., and Wahid, A.: A comparison of North American and Asian exposure-response data for ozone effects on crop yields, Atmos. Environ., 43, 1945–1953, https://doi.org/10.1016/j.atmosenv.2009.01.005, 2009.
Emberson, L. D., Pleijel, H., Ainsworth, E. A., van den Berg, M., Ren, W., Osborne, S., Mills, G., Pandey, D., Dentener, F., Büker, P., Ewert, F., Koeble, R., and Van Dingenen, R.: Ozone effects on crops and consideration in crop models, Eur. J. Agron., 100, 19–34, https://doi.org/10.1016/j.eja.2018.06.002, 2018.
Ewert, F. and Porter, J. R.: Ozone effects on wheat in relation to CO2: Modelling short-term and long-term responses of leaf photosynthesis and leaf duration, Glob. Change Biol., 6, 735–750, https://doi.org/10.1046/j.1365-2486.2000.00351.x, 2000.
FAO: The future of food and agriculture: trends and challenges, Rome, ISBN 978-92-5-109551-5, 2017.
FAO, IFAD, UNICEF, WFP, and WHO: The State of Food Security and Nutrition in the World 2020. Transforming food systems for affordable healthy diets, FAO, Rome, 320 pp., ISBN 978-92-5-132901-6, 2020.
Farquhar, G. D., Caemmerer, S., and Berry, J. A.: A biochemical model of photosynthetic CO2 assimilation in leaves of C3 species, Planta, 149, 78–90, https://doi.org/10.1007/BF00386231, 1980.
Fatima, A., Singh, A. A., Mukherjee, A., Agrawal, M., and Agrawal, S. B.: Variability in defence mechanism operating in three wheat cultivars having different levels of sensitivity against elevated ozone, Environ. Exp. Bot., 155, 66–78, https://doi.org/10.1016/j.envexpbot.2018.06.015, 2018.
Fatima, A., Singh, A. A., Mukherjee, A., Agrawal, M., and Agrawal, S. B.: Ascorbic acid and thiols as potential biomarkers of ozone tolerance in tropical wheat cultivars, Ecotox. Environ. Safe., 171, 701–708, https://doi.org/10.1016/j.ecoenv.2019.01.030, 2019.
Feller, U. and Fischer, A.: Nitrogen metabolism in senescing leaves, Crit. Rev. Plant Sci., 13, 241–273, https://doi.org/10.1080/07352689409701916, 1994.
Feng, Z., Kobayashi, K., and Ainsworth, E. A.: Impact of elevated ozone concentration on growth, physiology, and yield of wheat (Triticum aestivum L.): A meta-analysis, Glob. Change Biol., 14, 2696–2708, https://doi.org/10.1111/j.1365-2486.2008.01673.x, 2008.
Fowler, D., Amann, M., Anderson, R., Ashmore, M., Cox, P., Depledge, M., Derwent, D., Grennfelt, P., Hewitt, N., Hov, O., Jenkin, M., Kelly, F., Liss, P., Pilling, M., Pyle, J., Slingo, J., and Stevenson, D.: Ground-level ozone in the 21st century: future trends, impacts and policy implications, The Royal Society, ISBN 978-0-85403-713-1, 134 pp., 2008.
Fu, T. M. and Tian, H.: Climate Change Penalty to Ozone Air Quality: Review of Current Understandings and Knowledge Gaps, Curr. Pollut. Reports, 5, 159–171, https://doi.org/10.1007/s40726-019-00115-6, 2019.
Gaju, O., Allard, V., Martre, P., Le Gouis, J., Moreau, D., Bogard, M., Hubbart, S., and Foulkes, M. J.: Nitrogen partitioning and remobilization in relation to leaf senescence, grain yield and grain nitrogen concentration in wheat cultivars, Field Crop. Res., 155, 213–223, https://doi.org/10.1016/j.fcr.2013.09.003, 2014.
Gelang, J., Pleijel, H., Sild, E., Danielsson, H., Younis, S., and Selldén, G.: Rate and duration of grain filling in relation to flag leaf senescence and grain yield in spring wheat (Triticum aestivum) exposed to different concentrations of ozone, Physiol. Plantarum, 110, 366–375, https://doi.org/10.1111/j.1399-3054.2000.1100311.x, 2000.
Guarin, J. R., Kassie, B., Mashaheet, A. M., Burkey, K., and Asseng, S.: Modeling the effects of tropospheric ozone on wheat growth and yield, Eur. J. Agron., 105, 13–23, https://doi.org/10.1016/j.eja.2019.02.004, 2019.
Guo, J., Qu, L., Hu, Y., Lu, W., and Lu, D.: Proteomics reveals the effects of drought stress on the kernel development and starch formation of waxy maize, BMC Plant Biol., 21, 1–14, https://doi.org/10.1186/s12870-021-03214-z, 2021.
Havé, M., Marmagne, A., Chardon, F., and Masclaux-Daubresse, C.: Nitrogen remobilization during leaf senescence: Lessons from Arabidopsis to crops, J. Exp. Bot., 68, 2513–2529, https://doi.org/10.1093/jxb/erw365, 2017.
Herman, J. and Usher, W.: SALib Documentation, https://doi.org/10.21105/joss.00097, 2019.
Kamal, N. M., Gorafi, Y. S. A., Abdelrahman, M., Abdellatef, E., and Tsujimoto, H.: Stay-green trait: A prospective approach for yield potential, and drought and heat stress adaptation in globally important cereals, Int. J. Mol. Sci., 20, 5837, https://doi.org/10.3390/ijms20235837, 2019.
Kang, J., Chu, Y., Ma, G., Zhang, Y., Zhang, X., Wang, M., Lu, H., Wang, L., Kang, G., Ma, D., Xie, Y., and Wang, C.: Physiological mechanisms underlying reduced photosynthesis in wheat leaves grown in the field under conditions of nitrogen and water deficiency, Crop J., 11, 638–650, https://doi.org/10.1016/j.cj.2022.06.010, 2023.
Khanna-Chopra, R.: Leaf senescence and abiotic stresses share reactive oxygen species-mediated chloroplast degradation, Protoplasma, 249, 469–481, https://doi.org/10.1007/s00709-011-0308-z, 2012.
Lawlor, D. W.: Carbon and nitrogen assimilation in relation to yield: Mechanisms are the key to understanding production systems, J. Exp. Bot., 53, 773–787, https://doi.org/10.1093/jxb/53.370.773, 2002.
Liu, J., Feng, H., He, J., Chen, H., and Ding, D.: The effects of nitrogen and water stresses on the nitrogen-to-protein conversion factor of winter wheat, Agr. Water Manage., 210, 217–223, https://doi.org/10.1016/j.agwat.2018.07.042, 2018.
Liu, J., Feng, H., He, J., Chen, H., Ding, D., Luo, X., and Dong, Q.: Modeling wheat nutritional quality with a modified CERES-wheat model, Eur. J. Agron., 109, 125901, https://doi.org/10.1016/j.eja.2019.03.005, 2019.
Liu, Y., Zhang, P., Li, M., Chang, L., Cheng, H., Chai, S., and Yang, D.: Dynamic responses of accumulation and remobilization of water soluble carbohydrates in wheat stem to drought stress, Plant Physiol. Bioch., 155, 262–270, https://doi.org/10.1016/j.plaphy.2020.07.024, 2020.
Mariotti, F., Tomé, D., and Mirand, P. P.: Converting nitrogen into protein – Beyond 6.25 and Jones' factors, Crit. Rev. Food Sci., 48, 177–184, https://doi.org/10.1080/10408390701279749, 2008.
Martre, P., Jamieson, P. D., Semenov, M. A., Zyskowski, R. F., Porter, J. R., and Triboi, E.: Modelling protein content and composition in relation to crop nitrogen dynamics for wheat, Eur. J. Agron., 25, 138–154, https://doi.org/10.1016/j.eja.2006.04.007, 2006.
Mills, G., Pleijel, H., Braun, S., Büker, P., Bermejo, V., Calvo, E., Danielsson, H., Emberson, L., Fernández, I. G., Grünhage, L., Harmens, H., Hayes, F., Karlsson, P. E., and Simpson, D.: New stomatal flux-based critical levels for ozone effects on vegetation, Atmos. Environ., 45, 5064–5068, https://doi.org/10.1016/j.atmosenv.2011.06.009, 2011.
Mills, G., Pleijel, H., Malley, C. S., Sinha, B., Cooper, O. R., Schultz, M. G., Neufeld, H. S., Simpson, D., Sharps, K., Feng, Z., Gerosa, G., Harmens, H., Kobayashi, K., Saxena, P., Paoletti, E., Sinha, V., and Xu, X.: Tropospheric Ozone Assessment Report: Present-day ozone distribution and trends relevant to human health, Elem. Sci. Anthr., 6, 47, https://doi.org/10.1525/elementa.302, 2018a.
Mills, G., Sharps, K., Simpson, D., Pleijel, H., Broberg, M., Uddling, J., Jaramillo, F., Davies, W. J., Dentener, F., Van den Berg, M., Agrawal, M., Agrawal, S. B., Ainsworth, E. A., Büker, P., Emberson, L., Feng, Z., Harmens, H., Hayes, F., Kobayashi, K., Paoletti, E., and Van Dingenen, R.: Ozone pollution will compromise efforts to increase global wheat production, Glob. Change Biol., 24, 3560–3574, https://doi.org/10.1111/gcb.14157, 2018b.
Mills, G., Sharps, K., Simpson, D., Pleijel, H., Frei, M., Burkey, K., Emberson, L., Uddling, J., Broberg, M., Feng, Z., Kobayashi, K., and Agrawal, M.: Closing the global ozone yield gap: Quantification and cobenefits for multistress tolerance, Glob. Change Biol., 24, 4869–4893, https://doi.org/10.1111/gcb.14381, 2018c.
Mishra, A. K., Rai, R., and Agrawal, S. B.: Individual and interactive effects of elevated carbon dioxide and ozone on tropical wheat (Triticum aestivum L.) cultivars with special emphasis on ROS generation and activation of antioxidant defence system, Indian J. Biochem. Bio., 50, 139–149, 2013.
Nagarajan, S., Rane, J., Maheswari, M., and Gambhir, N.: Effect of Post-Anthesis Water Stress on Accumulation of Dry Matter, Carbon and Nitrogen and Their Partitioning in Wheat Varieties Differing in Drought Tolerance, J. Agron. Crop Sci., 183, 129–136, https://doi.org/10.1046/j.1439-037x.1999.00326.x, 1999.
Nehe, A. S., Misra, S., Murchie, E. H., Chinnathambi, K., Singh Tyagi, B., and Foulkes, M. J.: Nitrogen partitioning and remobilization in relation to leaf senescence, grain yield and protein concentration in Indian wheat cultivars, Field Crop. Res., 251, 107778, https://doi.org/10.1016/j.fcr.2020.107778, 2020.
Nguyen, T. H., Cappelli, G. A., Emberson, L., Ignacio, G. F., Irimescu, A., Francesco, S., Fabrizio, G., Booth, N., Boldeanu, G., Bermejo, V., Bland, S., Frei, M., Ewert, F., and Gaiser, T.: Assessing the spatio-temporal tropospheric ozone and drought impacts on leaf growth and grain yield of wheat across Europe through crop modeling and remote sensing data, Eur. J. Agron., 153, 127052, https://doi.org/10.1016/j.eja.2023.127052, 2024.
Osborne, S., Pandey, D., Mills, G., Hayes, F., Harmens, H., Gillies, D., Büker, P., and Emberson, L.: New insights into leaf physiological responses to ozone for use in crop modelling, Plants, 8, 84, https://doi.org/10.3390/plants8040084, 2019.
Osborne, T., Gornall, J., Hooker, J., Williams, K., Wiltshire, A., Betts, R., and Wheeler, T.: JULES-crop: a parametrisation of crops in the Joint UK Land Environment Simulator, Geosci. Model Dev., 8, 1139–1155, https://doi.org/10.5194/gmd-8-1139-2015, 2015.
Pande, P., Bland, S., Booth, N., Cook, J., Feng, Z., and Emberson, L.: Developing the DO3SE-crop model for Xiaoji, China, EGUsphere [preprint], https://doi.org/10.5194/egusphere-2024-694, 2024a.
Pande, P., Hayes, F., Bland, S., Booth, N., Pleijel, H., and Emberson, L. D.: Ozone dose-response relationships for wheat can be derived using photosynthetic-based stomatal conductance models, Agr. Forest Meteorol., 356, 110150, https://doi.org/10.1016/j.agrformet.2024.110150, 2024b.
Pandey, A. K., Ghosh, A., Agrawal, M., and Agrawal, S. B.: Effect of elevated ozone and varying levels of soil nitrogen in two wheat (Triticum aestivum L.) cultivars: Growth, gas-exchange, antioxidant status, grain yield and quality, Ecotox. Environ. Safe., 158, 59–68, https://doi.org/10.1016/j.ecoenv.2018.04.014, 2018.
Panozzo, J. F. and Eagles, H. A.: Rate and duration of grain filling and grain nitrogen accumulation of wheat cultivars grown in different environments, Aust. J. Agr. Res., 50, 1007–1015, https://doi.org/10.1071/AR98146, 1999.
Paoletti, E. and Grulke, N. E.: Ozone exposure and stomatal sluggishness in different plant physiognomic classes, Environ. Pollut., 158, 2664–2671, https://doi.org/10.1016/j.envpol.2010.04.024, 2010.
Pedregosa, F., Varoquaux, G., Gramfort, A., Michel, V., Thirion, B., Grisel, O., Blondel, M., Prettenhofer, P., Weiss, R., Dubourg, V., Vanderplas, J., Passos, A., Cournapeau, D., Brucher, M., Perrot, M., and Duchesnay, E.: Scikit-learn: Machine Learning in Python Fabian, J. Mach. Learn. Res., 12, 2825–2830, https://doi.org/10.48550/arXiv.1201.0490, 2011.
Piikki, K., De Temmerman, L., Ojanperä, K., Danielsson, H., and Pleijel, H.: The grain quality of spring wheat (Triticum aestivum L.) in relation to elevated ozone uptake and carbon dioxide exposure, Eur. J. Agron., 28, 245–254, https://doi.org/10.1016/j.eja.2007.07.004, 2008.
Pilbeam, D. J.: The Utilization of Nitrogen by Plants: A Whole Plant Perspective, Wiley, New York, 305–351, https://doi.org/10.1002/9781444328608.ch13, 2010.
Rai, R. and Agrawal, M.: Impact of tropospheric ozone on crop plants, P. Natl. A. Sci. India B, 82, 241–257, https://doi.org/10.1007/s40011-012-0032-2, 2012.
Saltelli, A., Ratto, M., Andres, T., Campolongo, F., Cariboni, J., Gatelli, D., Saisana, M., and Tarantola, S.: Global Sensitivity Analysis: The Primer, John Wiley & Sons Ltd, Chichester, https://doi.org/10.1111/j.1751-5823.2008.00062_17.x, 2008.
Sanchez-Bragado, R., Serret, M. D., and Araus, J. L.: The nitrogen contribution of different plant parts to wheat grains: Exploring genotype, water, and nitrogen effects, Front. Plant Sci., 7, 1–14, https://doi.org/10.3389/fpls.2016.01986, 2017.
Sarkar, A., Rakwal, R., Agrawal, S. B., Shibato, J., Ogawa, Y., Yoshida, Y., Kumar Agrawal, G., and Agrawal, M.: Investigating the impact of elevated levels of ozone on tropical wheat using integrated phenotypical, physiological, biochemical, and proteomics approaches, J. Proteome Res., 9, 4565–4584, https://doi.org/10.1021/pr1002824, 2010.
scikit-learn developers: sklearn.metrics.r2_score; scikit-learn 1.3.2 documentation: https://scikit-learn.org/stable/modules/generated/sklearn.metrics.r2_score.html, last access: 17 April 2024.
Shiferaw, B., Smale, M., Braun, H. J., Duveiller, E., Reynolds, M., and Muricho, G.: Crops that feed the world 10. Past successes and future challenges to the role played by wheat in global food security, Food Secur., 5, 291–317, https://doi.org/10.1007/s12571-013-0263-y, 2013.
Silvestro, P. C., Pignatti, S., Yang, H., Yang, G., Pascucci, S., Castaldi, F., and Casa, R.: Sensitivity analysis of the Aquacrop and SAFYE crop models for the assessment of water limited winter wheat yield in regional scale applications, PLoS One, 12, 1–30, https://doi.org/10.1371/journal.pone.0187485, 2017.
Soltani, A. and Sinclair, T. R.: Modeling physiology of crop development, growth and yield, edited by: Soltani, A. and Sinclair, T. R., CAB International, 336 pp., https://doi.org/10.1079/9781845939700.0001, 2012.
Sultana, N., Islam, S., Juhasz, A., and Ma, W.: Wheat leaf senescence and its regulatory gene network, Crop J., 9, 703–717, https://doi.org/10.1016/j.cj.2021.01.004, 2021.
Szopa, S., Naik, V., Adhikary, B., Artaxo, P., Berntsen, T., Collins, W. D., Fuzzi, S., Gallardo, L., Kiendler-Scharr, A., Klimont, Z., Liao, H., Unger, N., and Zanis, P.: Short-lived Climate Forcers, in: Climate Change 2021: The Physical Science Basis. Contribution of Working Group I to the Sixth Assessment Report of the Intergovernmental Panel on Climate Change, edited by: Masson-Delmotte, V., Zhai, P., Pirani, A., Connors, S. L., Péan, C., Berger, S., Caud, N., Chen, Y., Goldfarb, L., Gomis, M. I., Huang, M., Leitzell, K., Lonnoy, E., Matthews, J. B. R., Maycock, T. K., Waterfield, T., Yelekçi, O., Yu, R., and Zhou, B., Cambridge University Press, Cambridge, United Kingdom and New York, USA, 817–922, https://doi.org/10.1017/9781009157896.008, 2021.
Tkachuk, R.: Nitrogen-to-protein conversion factors for cereals and oilseed meals, Cereal Chem., 46, 419–424, 1969.
Triboi, E. and Triboi-Blondel, A. M.: Productivity and grain or seed composition: A new approach to an old problem – Invited paper, Eur. J. Agron., 16, 163–186, https://doi.org/10.1016/S1161-0301(01)00146-0, 2002.
van Keulen, H. and Seligman, N. H.: Simulation of water use, nitrogen nutrition and growth of a spring wheat crop, Pudoc, Wageningen, https://doi.org/10.1017/S0021859600081582, 1987.
Vazquez-Cruz, M. A., Guzman-Cruz, R., Lopez-Cruz, I. L., Cornejo-Perez, O., Torres-Pacheco, I., and Guevara-Gonzalez, R. G.: Global sensitivity analysis by means of EFAST and Sobol' methods and calibration of reduced state-variable TOMGRO model using genetic algorithms, Comput. Electron. Agr., 100, 1–12, https://doi.org/10.1016/j.compag.2013.10.006, 2014.
Wang, E. and Engel, T.: Simulation of growth, water and nitrogen uptake of a wheat crop using the SPASS model, Environ. Modell. Softw., 17, 387–402, https://doi.org/10.1016/S1364-8152(02)00006-3, 2002.
Wang, Y. and Frei, M.: Stressed food – The impact of abiotic environmental stresses on crop quality, Agr. Ecosyst. Environ., 141, 271–286, https://doi.org/10.1016/j.agee.2011.03.017, 2011.
Xu, B., Wang, T., Gao, L., Ma, D., Song, R., Zhao, J., Yang, X., Li, S., Zhuang, B., Li, M., and Xie, M.: Impacts of meteorological factors and ozone variation on crop yields in China concerning carbon neutrality objectives in 2060, Environ. Pollut., 317, 120715, https://doi.org/10.1016/j.envpol.2022.120715, 2023.
Yadav, A., Bhatia, A., Yadav, S., Kumar, V., and Singh, B.: The effects of elevated CO2 and elevated O3 exposure on plant growth, yield and quality of grains of two wheat cultivars grown in north India, Heliyon, 5, e02317, https://doi.org/10.1016/j.heliyon.2019.e02317, 2019.
Yadav, D. S., Rai, R., Mishra, A. K., Chaudhary, N., Mukherjee, A., Agrawal, S. B., and Agrawal, M.: ROS production and its detoxification in early and late sown cultivars of wheat under future O3 concentration, Sci. Total Environ., 659, 200–210, https://doi.org/10.1016/j.scitotenv.2018.12.352, 2019.
Yadav, D. S., Mishra, A. K., Rai, R., Chaudhary, N., Mukherjee, A., Agrawal, S. B., and Agrawal, M.: Responses of an old and a modern Indian wheat cultivar to future O3 level: Physiological, yield and grain quality parameters, Environ. Pollut., 259, 113939, https://doi.org/10.1016/j.envpol.2020.113939, 2020.
Zanis, P., Akritidis, D., Turnock, S., Naik, V., Szopa, S., Georgoulias, A. K., Bauer, S. E., Deushi, M., Horowitz, L. W., Keeble, J., Le Sager, P., O'Connor, F. M., Oshima, N., Tsigaridis, K., and Van Noije, T.: Climate change penalty and benefit on surface ozone: A global perspective based on CMIP6 earth system models, Environ. Res. Lett., 17, 024014, https://doi.org/10.1088/1748-9326/ac4a34, 2022.
Zhang, J., Chen, W., Dell, B., Vergauwen, R., Zhang, X., Mayer, J. E., and Van den Ende, W.: Wheat genotypic variation in dynamic fluxes of WSC components in different stem segments under drought during grain filling, Front. Plant Sci., 6, 1–11, https://doi.org/10.3389/fpls.2015.00624, 2015.
Zhao, D., Derkx, A. P., Liu, D. C., Buchner, P., and Hawkesford, M. J.: Overexpression of a NAC transcription factor delays leaf senescence and increases grain nitrogen concentration in wheat, Plant Biol., 17, 904–913, https://doi.org/10.1111/plb.12296, 2015.
- Abstract
- Introduction
- Model development
- Parameterisation and calibration of DO3SE-Crop and the new N module
- Results
- Discussion
- Conclusions
- Appendix A: DO3SE-CropN
- Code availability
- Data availability
- Author contributions
- Competing interests
- Disclaimer
- Special issue statement
- Financial support
- Review statement
- References
- Supplement
- Abstract
- Introduction
- Model development
- Parameterisation and calibration of DO3SE-Crop and the new N module
- Results
- Discussion
- Conclusions
- Appendix A: DO3SE-CropN
- Code availability
- Data availability
- Author contributions
- Competing interests
- Disclaimer
- Special issue statement
- Financial support
- Review statement
- References
- Supplement