the Creative Commons Attribution 4.0 License.
the Creative Commons Attribution 4.0 License.
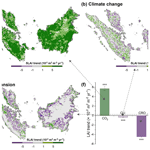
Cropland expansion drives vegetation greenness decline in Southeast Asia
Yuheng Yang
Luri Nurlaila Syahid
Janice Ser Huay Lee
Land-use and land-cover change (LUCC) is a key factor in determining regional vegetation greenness, impacting terrestrial carbon, water, and energy budgets. As a global LUCC hot spot, Southeast Asia has experienced intensive cropland and plantation expansion over the past 50 years; however, the impacts of these changes on the regional greenness have not been elucidated. Here, we harmonized multiple land-cover datasets, and used the satellite-derived leaf area index (LAI) in combination with a machine learning approach to quantify the impacts of LUCC on vegetation greenness in insular Southeast Asia (i.e. Peninsular Malaysia, Sumatra, and Borneo). We found that the regional LAI shows almost no trend (0.04 × 10−2 m2 m−2 yr−1) from 2000 to 2016: the net effect of an increased LAI (+5.71 × 10−2 m2 m−2 yr−1) due to CO2 fertilization is offset by a decreased LAI mainly due to cropland expansion (−4.46 × 10−2 m2 m−2 yr−1). The impact of croplands on greenness in Southeast Asia contrasts with that in India and China. Meanwhile, oil palm expansion and climate change induced only small decreases in the LAI in Southeast Asia (−0.41 × 10−2 and −0.38 × 10−2 m2 m−2 yr−1, respectively). Our research unveils how the LAI changes with different LUCC processes in Southeast Asia and offers a quantitative framework to assess vegetation greenness under different land-use scenarios.
- Article
(5685 KB) - Full-text XML
-
Supplement
(5185 KB) - BibTeX
- EndNote
Terrestrial vegetation plays a pivotal role in regulating ecosystem services, conserving biodiversity, and mitigating climate change impacts. Over recent decades, long-term satellite records of the leaf area index (LAI) have disclosed a notable increase in vegetation greenness on Earth (Chen et al., 2019; Zhu et al., 2016). While elevated atmospheric CO2 concentrations and climate change are regarded as the driving factors for vegetation greening at the global scale (Zhu et al., 2016), land-use and land-cover change (LUCC) can also substantially impact greenness at the regional scale. Previous studies have found that cropland intensification and afforestation are the primary drivers of greening in India and China (Chen et al., 2019; Kuttippurath and Kashyap, 2023). Meanwhile, other studies have reported that deforestation for croplands or pastures serves as a key driver of decreasing greenness in the Amazon (Chen et al., 2019; Querino et al., 2016). Thus, LUCC can either increase or decrease vegetation greenness, depending on the prior land use (e.g. forests and pastures), the subsequent land use (e.g. croplands, pastures, and plantations), and the intensity of these land uses (Wang and Friedl, 2019).
Southeast Asia harbours diverse biodiversity and ecosystems. However, the trends and drivers of regional greenness remain largely underexplored. Previous studies have revealed that CO2 fertilization is a primary driver of the greening trend in Southeast Asia within a global context (Chen et al., 2022; Chen and Guestrin, 2016). Thus, climate change, especially temperature rise, could reduce vegetation growth in the tropics (Piao et al., 2020a) or drive “green-up” in maritime Southeast Asia during El Niño (Satriawan et al., 2024). More importantly, land-use change, particularly deforestation, is the predominant factor causing the decline in greenness in tropical countries like Indonesia (Chen et al., 2019; Piao et al., 2020a). However, these prior studies have primarily focused on a global scale and, thus, likely oversimplified regional dynamics, such as complex land-use processes.
Since the 1950s, Southeast Asia has been a global LUCC hot spot (Houghton and Nassikas, 2017), with maritime countries such as Indonesia and Malaysia having the greatest deforestation rates in the world (Harris et al., 2012). An increase in food crops and export-oriented crop production has driven a significant transformation of tropical forests into commodity plantations like oil palms or croplands for food (Fagan et al., 2022; Zeng et al., 2018). Indonesia and Malaysia are the largest producers of palm fruit, with 250×106 and 97×106 t of palm fruit produced in 2020 (FAOSTAT, 2022), respectively. Meanwhile, Indonesia and Malaysia have experienced tree cover losses of approximately 29.4×106 and 8.92×106 ha, respectively, over the past 2 decades, equivalent to 18 % and 30 % of their respective tree cover in 2000 (Global Forest Watch, https://www.globalforestwatch.org/, last access: 28 November 2024).
Despite the substantial LUCC in the past years, we lack a clear understanding of the impacts of LUCC on vegetation greenness in Southeast Asia. This is partly due to the complexity of the recent land-use history of the region. For example, in Indonesia, the dominant LUCC types in the 2000s were the conversion of forests to oil palm plantations and cropland in lowland regions; however, in the 2010s, the conversion of forests to oil palm slowed down, shifting more towards highland croplands and the rotation of plantations (Descals et al., 2021; Xu et al., 2020; Zeng et al., 2018). These LUCC processes can differentially affect vegetation greenness and biogeochemical cycles (Ito and Hajima, 2020), with further feedback on vegetation greenness (Wang and Friedl, 2019). However, current studies on the assessment of LUCC impacts do not often distinguish these individual land-use processes; instead, they categorize the loss of forests under one “deforestation” category (Sitch et al., 2015) or regard plantation as similar to natural forests (Hansen et al., 2013).
In this study, we aim to assess the impact of LUCC on vegetation greenness in Southeast Asia and quantify the contributions of the different LUCC processes to the changes in greenness. We collected and harmonized various types of land-cover datasets (Chini et al., 2021; Hansen et al., 2013; Sulla-Menashe et al., 2019; Xu et al., 2020) to build a detailed land-use history for Southeast Asia from 2000 to 2016. We further used a machine learning approach to quantify the impacts of land uses on the LAI, along with the impacts from climate, CO2 concentrations, stand age, etc. Our machine learning approach, combined with hypothetical scenarios that simulate vegetation greenness without LUCC processes, enables us to isolate the impacts of different land-use changes on the LAI by estimating the difference between scenario-based LAI values.
2.1 Study areas
Depending on the availability of various land-use data, we focused our study on a region including Peninsular Malaysia, Sumatra, and Borneo (Fig. 1) in Southeast Asia, which experience rapid LUCC (Geist and F, 2001; Mao et al., 2023). Since the 1980s, insular Southeast Asia has lost at least 1.0 % of its forests annually (Felbab-Brown, 2013; Miettinen et al., 2011), primarily due to cropland and plantation expansion (Wang et al., 2023; Wicke et al., 2011; Xu et al., 2020). Particularly notable is the expansion of oil palm plantations in Indonesia and Malaysia (Chen et al., 2024; Euler et al., 2016). From the 1990s to the 2010s, the extent of oil palm plantations increased from 1.3×106 to 7.7×106 ha in Indonesia and from 2.1×106 to 5.2×106 ha in Malaysia (Xu et al., 2022). A nation-wide study reported that around 55 %–59 % of oil palm plantation expansion in Malaysia and at least 56 % in Indonesia occurred on lands previously covered by forests during the period from 1990 to 2005 (Koh and Wilcove, 2008; Vijay et al., 2016). In parallel, cropland expansion also drives deforestation in our study area, with approximately 15 % of forest loss in Indonesia attributed to this cause (Austin et al., 2019). Rubber, timber, and other plantations have also resulted in deforestation. A recent study revealed that, between 2001 and 2016, approximately 20 % of rubber plantations in Indonesia and 33 % in Malaysia were established on land previously covered by forests, resulting in a loss of about 1×106 and 0.32×106 ha of forest in these countries, respectively (Wang et al., 2023).
2.2 Identification of the greening trend
The LAI indicates the total amount of one-sided leaf area per unit of ground surface area (Chen and Black, 1992; Watson, 1947) and often serves as a measure of vegetation greenness (Zhu et al., 2016). In this study, we use the GLOBMAP LAI dataset, which provides a global record of vegetation cover with a 500 m resolution and is one of the main LAI datasets used for global greenness studies (Piao et al., 2020b; Zhu et al., 2016). We used the GLOBMAP LAI (version 3) dataset post-2001, which was generated based on the Moderate Resolution Imaging Spectroradiometer (MODIS) surface reflectance (Liu et al., 2012), with an advanced algorithm to consider canopy clumping, making it particularly suitable for dense canopies in the tropics (Fang et al., 2019). To assess the trend in the LAI for individual pixels, we utilized the Mann–Kendall test, a non-parametric statistical method that can effectively identify consistent upward or downward trends over time (Mann, 1945). This test provides the pixel-by-pixel magnitude (β) and statistical significance (p values) of the greening trends.
2.3 Mapping different land-use transitions
In our study area, we considered natural forests, oil palms, and croplands as the major land-use types, as they together accounted for over 90 % of the land cover. To delineate the annual changes in all of these land-use types from 2001 to 2016, we harmonized various land-use datasets, which were provided at different spatial resolutions (Table S1), into a unified dataset on a 500 m grid. This harmonization was essential to make land-use changes across various datasets compatible with each other and to match the spatial resolution of the land-use data to the LAI dataset. As shown in Figs. 1 and S1, the workflow for harmonizing multiple land-cover datasets involved the following steps:
-
We first determined the annual percentages of forested (A %) and non-forested areas (B %) within each 500 m grid cell by aggregating the mean of the annual 30 m resolution Global Forest Change (GFC; v1.11) maps (Hansen et al., 2013), using the “reduceResolution()” function in Google Earth Engine (https://developers.google.com/earth-engine/guides/resample, last access: 28 November 2024).
-
Within the forested fraction of each grid cell, we estimated the proportion of oil palm (OP) plantations (A1 %) using an openly available dataset that covers the OP distribution across Malaysia and Indonesia from 2001 to 2016 (Xu et al., 2020). To estimate the proportion of OP, we calculated the frequency of oil palm pixels in each 500 m × 500 m window.
-
After accounting for the OP area, the remaining forested area in each grid was further categorized into the evergreen broadleaf forest (EBF) (A2 %) and other forest types (i.e. deciduous broadleaf forest, coniferous forest, mixed forest, etc.), based on the ratio of EBF to the total forested area provided by the MODIS Land Cover Type Product (MCD12Q1 v6.1) (Sulla-Menashe and Friedl, 2018).
-
Within the non-forested fraction of each grid cell, we used the latest version of the Land-Use Harmonization (LUH2) dataset (Hurtt et al., 2020) to estimate the percentage of cropland (CRO) (B1 %) and other non-forest land uses (i.e. pasture, grass, etc.). In this analysis, we assumed that the fraction of each land-use type in the LUH2 dataset on a 0.25° grid is applicable to the 500 m grid cells within each 0.25° grid cell. To avoid biases when downscaling land-cover data from a coarse to fine resolution, we also tested our results by upscaling all datasets to 0.25° (Figs. S2, S3).
In the end, we obtained detailed information for the EBF, OP, CRO, and Other (including other forests and non-forest vegetated areas) land-use types, at a 500 m spatial resolution. We grouped other forests and other non-forest vegetated areas together, as they represented a minor proportion (less than 5 %) of the land surface (Table S2) and exhibited minimal changes during the study period.
2.4 Extreme gradient boosting model
Tree-based machine learning models, such as extreme gradient boosting (XGBoost), have been widely used in predicting and analysing ecosystem dynamics (Green et al., 2022; Yuan et al., 2019). Compared with neural networks, which often function like “black boxes”, tree-based models offer greater interpretability and are particularly effective on tabular-style datasets (Lundberg et al., 2020). XGBoost is an ensemble learning algorithm that iteratively constructs multiple decision trees and has proven to be effective for both classification and regression tasks (Chen and Guestrin, 2016; Yan et al., 2020). This algorithm employs shrinkage techniques and performs multithreaded calculations to minimize overfitting (Meng et al., 2021).
In our study, we applied the XGBoost algorithm (Chen and Guestrin, 2016) to model the spatiotemporal variations in the mean annual LAI using climatic and LUCC factors as inputs. These factors include the fractions of EBF, OP, CRO, and Other land uses; EBF-stand and OP-stand ages (Besnard et al., 2021; Danylo et al., 2021); CO2 concentrations (https://gml.noaa.gov/ccgg/trends/, last access: 28 November 2024); and climatic variables (Table S3). The climatic variables in our study include the mean annual temperature (MAT), mean annual precipitation (MAP), wind speed (WS), shortwave radiation (RAD), and relative humidity (RH). These gridded climatic variables were obtained from the European Centre for Medium-Range Weather Forecasts (ECMWF) reanalysis product v5 – Land (https://cds.climate.copernicus.eu/datasets/reanalysis-era5-land?tab=overview, last access: 28 November 2024). We aggregated the original hourly data to the annual time step using an annual average.
To fine-tune the parameters of our XGBoost model for LAI prediction, we randomly split the data into training and testing sets using an 80 % : 20 % ratio. We then utilized the GridSearchCV method to test different parameter combinations (i.e. varying numbers of trees from 150 to 400, tree depths from 5 to 15, and learning rates between 0.01 and 0.1) and determined the best parameter combinations through cross-validation (Pedregosa et al., 2011).
2.5 Shapley additive explanations
We utilized the TreeExplainer-based SHapley Additive exPlanations (SHAP) framework to interpret the individual and interactive contributions of LUCC and other factors (including climate variables, CO2 concentration, and stand ages) to the LAI variations in our XGBoost model. The SHAP methodology, which is based on the concept of Shapley values in cooperative game theory, offers an insightful interpretation of factor importance (Lundberg and Lee, 2017). It provides detailed, instance-specific explanations, termed SHAP values, to quantify the impact of each factor on the model predictions (Lundberg et al., 2020; Yang et al., 2021).
In the SHAP framework, the value for a given factor i in a particular sample x is computed as the average marginal contribution of that factor across all possible combinations. This is mathematically represented as follows:
where N is the set of all factors, S is a subset of factors, and f is the prediction model. This formula quantifies the contribution of factor i by comparing the prediction with and without the factor, averaging over all possible subsets of factors.
The SHAP value indicates the magnitude and direction of the impact of a factor on prediction in the specific sample. To be specific, the magnitude (absolute value) of a SHAP value indicates the importance of a factor. Larger absolute SHAP values mean that the factor has a greater impact on the model's output. The sign of a SHAP value (positive or negative) shows the direction of the impact. A positive SHAP value indicates that the factor positively affects the model's output (e.g. increases LAI), whereas a negative SHAP value suggests a negative impact (e.g. decreases LAI). By aggregating the mean SHAP values of all samples, we can also derive global factor importance, which offers a holistic view of variables affecting annual LAI variations.
Furthermore, SHAP values aid in the interpretation of the interactive effects of two or more factors in machine learning models (Lundberg and Lee, 2017). The interactive effect is defined as the change in prediction when the joint contribution of two or more factors is considered, by subtracting the individual contributions made by each factor. The interactive effects of ith and jth factors are expressed as follows:
We utilized the XGBoost and scikit-learn packages in Python 3.11.0 to develop and train the XGBoost model (Chen and Guestrin, 2016; Pedregosa et al., 2011). Then, we employed the TreeExplainer function from the shap package (Lundberg and Lee, 2017) to interpret the impact of factors on LAI predictions.
2.6 Simulation scenarios
To quantify and compare the impacts of specific LUCC processes, climate change, and elevated CO2 concentrations on vegetation greenness changes, we adopted the scenario simulation framework from several factorial attribution analyses (Sitch et al., 2015). Specifically, we first estimated the LAI trend under five hypothetical scenarios (S1–S5) using the established XGBoost model. The equations utilized are as follows:
Here, represents the simulated LAI for the ith grid at year of t under scenario Sn; βLAIi,Sn indicates the LAI trend over the study period; and XGBoost stands for the established model for LAI prediction using the CO2 concentration (CO2), climate variable (CLI), and land-cover types, such as the fraction of evergreen broadleaf forest (f_EBF), cropland (f_CRO), oil palm (f_OP), other land uses (f_Other) (see Sect. 2.4).
For different scenarios, we adjusted the input variables according to specific assumptions to progressively incorporate different factors. For S1, we assumed that only the CO2 concentration varied from 2001 to 2016, while climate and land-use variables (i.e. CLI, f_EBF, f_CRO, f_OP, and f_Other) remained constant at their 2001 values. For S2, CO2 and climate change over time, with land uses remaining unchanged at their 2001 values. S3 to S5 sequentially considered different land-use processes: S3 involved changes from EBF to CRO using time-varying CO2, climate, and CRO fractions, while keeping OP and other land-use types constant post-2001; S4 included conversions from EBF to both CRO and OP using time-varying CO2, climate, CRO and OP fractions, while other land uses remained unchanged post-2001; and S5 encompassed all LUCC changes, with all variables including CO2, climate, and all LUCC types varying over time.
We then quantified the impacts of each factor on vegetation greening based on differences in LAI trends between scenarios as follows:
Here, Drivern measures the impact of the nth driver on LAI trends. The drivers include CO2, climate change, CRO expansion, OP expansion, and Other LUCC. Notably, Driver1 quantifies the impact of CO2, equal to βLAIi,S1. In addition, our estimation of CRO or OP expansion assumed that the increased areas of CRO or OP since 2001 came from EBF, given that CRO and OP expansion mostly resulted from deforestation in Indonesia and Malaysia (Numata et al., 2022; Wagner et al., 2022).
3.1 Land-use changes and greening trends
From 2001 to 2016, the extent of forest in our study area decreased annually by 1.29 %, leading to an EBF reduction from 73.41 % to 53.09 % of the study area. Notably, approximately 25.54 % of the region experienced rapid deforestation, with a forest loss rate exceeding 2 % of the land surface per year. This deforestation rate was especially pronounced in the eastern parts of Sumatra and along the western and southern edges of Borneo (Fig. 2b).
In the deforested areas, we observed the widespread expansion of CRO and OP plantations. The CRO area increased at a rate of 0.63 % yr−1, resulting in an increase in CRO area from 14.45 % to 24.56 % of the region (i.e. 20.76 × 104 to 35.28 × 104 km2) between 2000 and 2016. Meanwhile, the expansion of OP proceeded at a pace of 0.48 % yr−1, resulting in a nearly tripled extent of oil plantations over the past decade (i.e. from 3.91 % in 2001 to 12.05 % in 2016; Fig. 2a). In central Sumatra, the southern edge of Borneo, and the southern part of Peninsula Malaysia, OP showed the largest increase, partly at the expense of decreases in CRO (Fig. 2c).
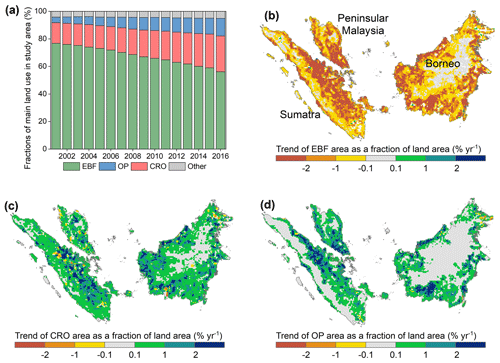
Figure 2Land-use composition and its changes in the study area from 2001 to 2016: (a) the changes in the fractions of main land uses, including evergreen broadleaf forest (EBF), oil palm (OP), cropland (CRO), and Other land uses over the study period; (b–d) spatial distribution of the trend in EBF, CRO, and OP as a fraction of land area.
The regional average LAI exhibited a non-significant upward trend over the study period, with a slope of 0.04 × 10−2 m2 m−2 yr−1 (Fig. 3a). Using another LAI dataset (MODIS LAI), we also captured an increasing trend (Fig. S4). According to Galán-Acedo et al. (2021), areas with over 70 % forest loss are categorized as having high to severe deforestation, whereas areas with less than 70 % forest loss are classified as undergoing low to intermediate deforestation. We found that, for our study area, the non-significant upward trend in the LAI was due to the net effect of a rapid LAI increase ( × 10−2 m2 m−2 yr−1, p<0.05) in areas with low to intermediate deforestation and a significant LAI decline ( × 10−2 m2 m−2 yr−1, p<0.001) in areas with high to severe deforestation (Fig. 3a). Across the study area, 58.50 % of the region showed a significant decreasing LAI trend; these areas were mostly regions with pronounced forest loss (Figs. 2b, 3b).
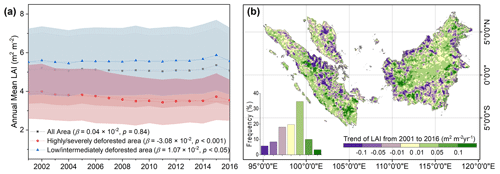
Figure 3Trends in the LAI in the study area from 2001 to 2016. Panel (a) shows the regional average LAI trend, the LAI trends in regions with high to severe deforestation, and the LAI trends in regions with low to intermediate deforestation. The classification of the deforestation level is taken from Galán-Acedo et al. (2021): areas experiencing more than 70 % forest loss are classified as high or severe deforestation, whereas those with less than 70 % loss are classified as low or intermediate deforestation. Panel (b) presents the spatial pattern in the LAI trend, with the inset histogram showing the frequency (%) of the pixel-wise LAI trend in the study area.
3.2 Drivers of changes in the LAI
To understand the variations in vegetation greenness in Southeast Asia, we established an XGBoost model to quantify the relationship between vegetation greenness and land uses, climate variables, CO2 concentrations, and stand ages. The XGBoost model showed high explanatory power (i.e. 98 % accuracy for calibration and 93 % accuracy for validation), underscoring the model's reliability for analysing the determinants of LAI variability (Fig. 4). Using different splitting ratios for calibration and validation dataset, we obtained similar model performance (Fig. S5).
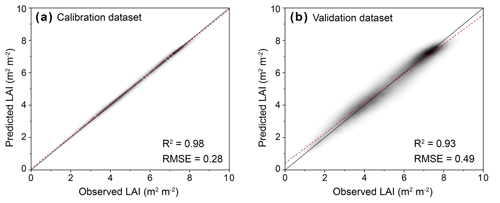
Figure 4Comparison of observed and predicted LAI values with the XGBoost model for the (a) calibration and (b) validation datasets.
Based on the built XGBoost model, we evaluated the contributions of each factor to the LAI in the SHAP framework (Fig. 5; see Sect. 2). Our results reveal that the fraction of EBF (f_EBF) in each grid holds the greatest mean absolute SHAP value (1.28), indicating that f_EBF had the largest impact on the LAI (Fig. 5b) and that this impact was positive (Figs. 5a, S6a).
The average impact of the fraction of OP (f_OP) on the LAI ranked the second largest (0.21) and had a similar positive impact on the LAI to f_EBF (Figs. 5a, b; S6b). In contrast, the impact of CRO and other land uses on the LAI was found to be negative (Figs. 5a; S6c, d). A higher fraction of CRO (f_CRO) led to a larger negative SHAP value. We further explored the impacts of the interactions of land-use types (i.e. f_CRO, f_OP, and f_EBF) on the LAI. The results demonstrated that, in areas with low f_EBF, an increase in f_OP enhanced the LAI, whereas f_CRO induced LAI decreases. Meanwhile, in areas with high f_EBF, the impacts of both f_OP and f_CRO on the LAI were markedly reduced, suggesting a dominant role of f_EBF in greenness in the region (Fig. 5c, d).
Apart from the impacts of land uses on the LAI, we also found that an elevated CO2 concentration substantially increased the LAI, with a mean SHAP value of 0.18. In contrast to an elevated CO2 concentration, the MAT was negatively related to the LAI, with a smaller mean SHAP value of 0.08 (Figs. 5b, S6e). Other climate variables have limited impacts on the LAI. It is noteworthy that the stand ages of both EBF (Age_EBF) and OP (Age_OP) positively impact the LAI. Specifically, Age_EBF has a greater impact than that of Age_OP, as indicated by a higher absolute mean SHAP value for Age_EBF (0.11) compared with Age_OP (0.08). While we found that Age_EBF continuously contributed to LAI increases (Fig. S6k), Age_OP increased the LAI at a younger age (less than 12 years) and then decreased the LAI afterward (Fig. S6l).
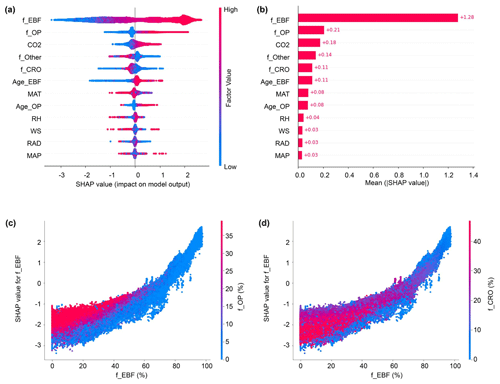
Figure 5The impact of factors on LAI. In panel (a), beeswarm plots show the SHAP values of each LAI factor for each sample. The SHAP value indicates the magnitude and direction of the impact on LAI (see Sect. 2). Each dot represents an individual sample, with the colour indicating the relative values of the specific factor. Panel (b) presents a bar plot of the mean absolute SHAP values of each LAI factor. Panel (c) shows the interaction of f_OP and f_EBF on the LAI, whereas panel (d) presents the interaction of f_CRO and f_EBF on the LAI. The definitions of the abbreviations used for each factor are available in Table S3.
3.3 Impacts of LUCC on greening
Through scenario-based prediction, we quantified the impacts of LUCC, elevated CO2 concentration, and climate change on the greening trend (Figs. 6f, S8). Compared with the observed greening trend for the study area (i.e. 0.04 × 10−2 m2 m−2 yr−1), we found that the greening trend increased to 5.71 × 10−2 m2 m−2 yr−1 under scenario S1, which simulated the effect of elevated CO2 alone, with the climate and LUCC values remaining constant. This result suggests that elevated CO2 had a clear positive impact on the LAI in Southeast Asia. Climate change showed a small negative impact (i.e. mostly due to rising temperature) on the LAI. We found that both CRO expansion and OP expansion decreased the LAI trend, with the trend dramatically dropping by −4.46 × 10−2 m2 m−2 yr−1 under the impact of CRO expansion and by −0.41 × 10−2 m2 m−2 yr−1 under the impact of OP expansion. These results highlight that CRO expansion was the primary reason for the decrease in vegetation greenness, counteracting the greening trend caused by elevated CO2 in our study area. In contrast, OP expansion only contributed to a small decline in greenness.
We further examined the spatial variations in the impacts of each factor on greening by quantifying the differences in greening trends under different scenarios at the pixel level. Across the study area, LUCC imposed a negative impact on LAI trends (Fig. 6c, d, e). Consistent with regional average values, we found that the changes from EBF to CRO had a more pronounced negative impact on the greening trend than the conversion of EBF to OP (Fig. 6c, d). In some regions, such as the southern edges of Sumatra and Borneo, OP enhanced regional greening (Fig. 6d). Meanwhile, elevated CO2 concentrations consistently had a positive impact on greening across the region (Fig. 6a), and climate change showed an overall negative but highly heterogeneous impact on LAI trends (Fig. 6b). From a spatial perspective, we found that elevated CO2 dominated the increase in the LAI in most areas, accounting for 62.10 % of the study area, while CRO expansion was the primary driver of LAI decreases in other regions (26.33 %), especially coastal areas (Fig. S7).
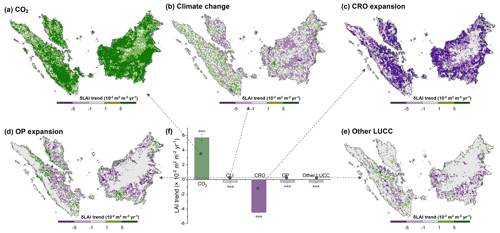
Figure 6The spatial distribution of the pixel-wise impacts of each factor on the greening trends (δLAI trend). Positive values mean that the factors considered increase the LAI trend, whereas negative values mean the inverse. We show the spatial patterns of contribution from (a) elevated CO2 concentrations, (b) climate change, (c) expansion of cropland (CRO), (d) expansion of oil palm (OP), and (e) Other land-use changes. Panel (f) shows the average impact of each factor, with the error bars indicating 1 standard deviation. The symbol “” denotes a statistically significant difference in LAI trends at the p<0.001 level.
In this study, we analysed the typical LUCC processes in Peninsular Malaysia, Sumatra, and Borneo as well as their impacts on greenness over the past 2 decades. We found a significant decline in EBF coverage, from 73.41 % to 53.09 %, predominantly due to CRO and OP plantation expansion. Meanwhile, we did not find a significant trend in the LAI in our study area, as the increases in regional greenness due to elevated CO2 were offset by the decreases in regional greenness caused by CRO and OP expansion. Notably, the negative effect of CRO expansion (−4.46 × 10−2 m2 m−2 yr−1) was more pronounced than that of OP expansion (−0.41 × 10−2 m2 m−2 yr−1), indicating a dominant role of CRO expansion on greenness decline in our study area.
4.1 Strong negative impact of cropland expansion on greenness in Southeast Asia
Our results demonstrate that CRO expansion in Southeast Asia contributed negatively to vegetation greenness. This is the opposite of existing reports on the greening trend in China and India, where CRO expansion is suggested to be the main reason for the net increase in the LAI (Chen et al., 2019; Kuttippurath and Kashyap, 2023). This disparity may be partly attributed to the original land-use types before CRO expansion. In Southeast Asia, CRO expansion mainly occurs at the expense of natural forests (Potapov et al., 2022; Zeng et al., 2018), and crops often have less-dense canopies than natural forests (Asner et al., 2005; Foley et al., 2005; Pocock et al., 2010). In contrast, the increase in the LAI in China and India primarily resulted from the intensification of croplands, rather than their expansion; moreover, where expansion did occur, it predominantly took place on lands that were previously bare or sparsely vegetated (Chen et al., 2019).
We also note that agricultural practices in Indonesia and Malaysia are generally less intensive compared with those in India and China, partly due to the less-advanced agricultural technologies deployed in the region (X. Liu et al., 2021). For example, in China and India, intensive agricultural practices, including precision fertilization and advanced irrigation systems, such as drip irrigation in China and spray irrigation in India, are widely adopted to enhance crop growth (Cui et al., 2022; Wang et al., 2013). In addition, the development of specialized crop varieties, such as hybrid rice in China and climate-smart drought-tolerant rice varieties in India (Panda et al., 2021; Zhang et al., 2022), also facilitated plant growth and, consequently, regional greening (Zhao et al., 2021; J. Zhao et al., 2023). In contrast, Indonesia and Malaysia predominantly depend on rainfed irrigation and traditional farming methods, such as the “subak” terraced rice fields in Indonesia, leading to lower crop intensity. Less-intensive practices are less likely to create a dense canopy and high biomass in croplands (Takeshima, 2019).
4.2 Minor contribution of oil palm to regional greenness
Our study observed a small negative impact of OP expansion on greenness. This aligns with studies reporting that OP has LAI values comparable to, or only slightly lower than, native forests (Propastin, 2009; Rusli and Majid, 2014; Vernimmen et al., 2007). Therefore, OP expansion, although a major driver of land-use change in Southeast Asia, was not a main driver of greenness decline in the study region.
Meanwhile, we also found that the OP stand age influences the LAI, as the LAI of OP increases with stand age when OP is young but then decreases after a threshold stand age is reached. This observation agrees with previous studies that reported a plateau and decrease in the oil palm LAI after the age of 10 (Xu et al., 2021) and a plateau and decrease in the oil palm yield after the age of 8–9 (Park et al., 2023). The stand age of most OP plantations in Southeast Asia is approaching maturity (over half were planted before 2009) (Danylo et al., 2021). In response, industrial and smallholder plantations have undergone or are starting to undergo the process of replanting (Danylo et al., 2021; Numata et al., 2022), although replanting in smallholder plantations is often delayed as farmers face more financial constraints (J. Zhao et al., 2023). Hence, we expect to see a more complex influence of OP on the LAI trend depending on the management practices of different types of plantations.
In comparison with CRO, our findings indicate that the impact of OP expansion on vegetation greenness decline is relatively minor (Fig. 6). This can be attributed to the generally higher biomass of OP compared with typical crops, like rice and maize, in Indonesia and Malaysia. In addition, we assumed that the CRO or OP expansion since 2001 came from EBF in our study. While CRO and OP expansion indeed mostly resulted from deforestation in Indonesia and Malaysia (Numata et al., 2022; Wagner et al., 2022), the expansion of CRO and OP can also come from other land uses (i.e. grasslands or pastures). We suspect that transitions from these land uses to CRO and OP might result in a smaller negative impact on vegetation greenness, considering that grasslands and shrubs have a lower LAI than EBF.
4.3 Other LUCC impacts on greenness in Southeast Asia
While our study examined the two most prominent processes of LUCC in Southeast Asia (EBF to OP and EBF to CRO), there are other types of land-use change that we analysed together under the category of “Other” land-use types. The changes in these land-use types are also relevant to deforestation, and they include other plantations, such as rubber (Wang et al., 2023); agroforestry plantations, such as cocoa and coffee (Panda et al., 2021); and grasslands or pastures (Austin et al., 2019). In total, the Other category accounted for 4.70 % of the study area in 2016, much less than the three main land-use types that we studied (51.06 % for EBF, 25.01 % for CRO, and 12.09 % for OP in 2016), and has experienced minor changes since 2001.
Meanwhile, we found that the overall impact of these other LUCC types on greenness was likely small (Fig. 6f). The small impact is contingent on their smaller extent compared with EBF, CRO, and OP (see above). It may also result from the offset of positive and negative impacts from individual other LUCC processes on greenness. For example, rubber plantations exhibit a higher LAI than natural forests (Wang et al., 2022), whereas agroforestry and other plantations generally have a lower LAI than natural forests, therefore leading to different trends in the LAI after deforestation.
4.4 Impact of climate change and CO2 concentrations on regional greenness
CO2 fertilization effects appear to be the primary drivers of greening trends observed in global studies (Chen et al., 2022; Ewert, 2004; Zhu et al., 2016). Our research also confirmed the substantial contribution of rising CO2 levels to the greening of vegetation in Southeast Asia. The impact of temperature on greenness in Southeast Asia was negative, in contrast to the positive effects noted in cold climate zones, such as the Qinghai–Tibet Plateau (Yang et al., 2024; R. Zhao et al., 2023; Zhong et al., 2019) and Arctic areas (Forbes et al., 2010). We suspect that the negative effect of temperature implies that temperature may have exceeded the optimal point for plant growth in parts of Southeast Asia. This aligns with several studies suggesting that ecosystem functions in the tropics are approaching a temperature tipping point (Doughty et al., 2023; Meir et al., 2015; Wu et al., 2019). Additionally, temperature rise might exacerbate the incidence of pests and diseases in tropical forests, negatively impacting plant health and productivity (Ghini et al., 2011). These factors might jointly contribute to the observed decline in greenness with increasing temperatures in Southeast Asia.
Our study closely examined the impacts of LUCC on vegetation greenness in part of Southeast Asia. We found that there was no significant trend in vegetation greenness in the study area, which is attributed to the net effect of negative impacts of LUCC on LAI and positive influence of elevated CO2 on the LAI. Among various LUCC processes, we found that cropland expansion was the primary reason for LAI decrease, whereas oil palm expansion had a small impact on the LAI trends. These results shed light on the interplay between greenness and land-use changes and provide valuable insight into our future studies on terrestrial carbon, water, and energy budgets in the land-use-change-intensive region of Southeast Asia.
Preprocessing and post-processing scripts are available from https://doi.org/10.5281/zenodo.14259860 (Zhao, 2024).
The GLOBMAP LAI dataset is available from https://doi.org/10.5281/zenodo.4700264 (R. Liu et al., 2021). Climatic variables, including temperature, precipitation, wind speed, shortwave downward radiation, and humidity, can be obtained from the ERA5 model website (https://doi.org/10.24381/cds.e2161bac, Muñoz Sabater, 2019). The forest age and plantation age distribution maps are available from https://doi.org/10.17871/ForestAgeBGI.2021 (Carvalhais, 2021). CO2 concentrations can be obtained from https://gml.noaa.gov/ccgg/trends/ (Lan, 2024).
The supplement related to this article is available online at: https://doi.org/10.5194/bg-21-5393-2024-supplement.
XL and RZ conceptualized the study, created the figures, and wrote and prepared the original manuscript draft. RZ and YY contributed to the data curation, methodology, and software development. RZ, XL, and YY performed the formal analysis. XL acquired the funds. LNS, CC, and JSHL were responsible for the project administration and the supervision of the research planning. RZ and YY contributed to software development. All authors contributed to the writing process, with respect to the review, editing, and validation.
The contact author has declared that none of the authors has any competing interests.
Publisher's note: Copernicus Publications remains neutral with regard to jurisdictional claims made in the text, published maps, institutional affiliations, or any other geographical representation in this paper. While Copernicus Publications makes every effort to include appropriate place names, the final responsibility lies with the authors.
Xiangzhong Luo and Ruiying Zhao are supported by a Tier 2 research grant from the Singapore Ministry of Education (grant no. A-8001551-00-00) and a Singapore Energy Centre Core project (grant no. A-8000179-00-00). The authors wish to acknowledge Tin Widyani Satriawan, a PhD student in our group, for her valuable suggestions on refining the manuscript's language.
This research has been supported by the Singapore Ministry of Education (grant no. A-8001551-00-00) and the Singapore Energy Centre (grant no. A-8000179-00-00).
This paper was edited by Sara Vicca and reviewed by two anonymous referees.
Asner, G. P., Knapp, D. E., Broadbent, E. N., Oliveira, P. J. C., Keller, M., and Silva, J. N.: Selective Logging in the Brazilian Amazon, Science, 310, 480–482, https://doi.org/10.1126/science.1118051, 2005.
Austin, K. G., Schwantes, A., Gu, Y., and Kasibhatla, P. S.: What causes deforestation in Indonesia?, Environ. Res. Lett., 14, 24007, https://doi.org/10.1088/1748-9326/aaf6db, 2019.
Besnard, S., Koirala, S., Santoro, M., Weber, U., Nelson, J., Gütter, J., Herault, B., Kassi, J., N'Guessan, A., Neigh, C., Poulter, B., Zhang, T., and Carvalhais, N.: Mapping global forest age from forest inventories, biomass and climate data, Earth Syst. Sci. Data, 13, 4881–4896, https://doi.org/10.5194/essd-13-4881-2021, 2021.
Carvalhais, B.: Global 1km forest age datasets, Max Planck Institute for Biogeochemistry [data set], https://doi.org/10.17871/ForestAgeBGI.2021, 2021.
Chen, C., Park, T., Wang, X., Piao, S., Xu, B., Chaturvedi, R. K., Fuchs, R., Brovkin, V., Ciais, P., Fensholt, R., Tømmervik, H., Bala, G., Zhu, Z., Nemani, R. R., and Myneni, R. B.: China and India lead in greening of the world through land-use management, Nat. Sustain., 2, 122–129, https://doi.org/10.1038/s41893-019-0220-7, 2019.
Chen, C., Riley, W. J., Prentice, I. C., and Keenan, T. F.: CO2 fertilization of terrestrial photosynthesis inferred from site to global scales, P. Natl. Acad. Sci. USA, 119, e21156271, https://doi.org/10.1073/pnas.2115627119, 2022.
Chen, J. M. and Black, T. A.: Defining leaf area index for non-flat leaves, Plant Cell Environ., 15, 421–429, https://doi.org/10.1111/j.1365-3040.1992.tb00992.x, 1992.
Chen, S., Woodcock, C., Dong, L., Tarrio, K., Mohammadi, D., and Olofsson, P.: Review of drivers of forest degradation and deforestation in Southeast Asia, Remote Sens. Appl. Soc. Environ., 33, 101129, https://doi.org/10.1016/j.rsase.2023.101129, 2024.
Chen, T., and Guestrin, C.: XGBoost: A Scalable Tree Boosting System, in: Proceedings of the 22nd acm sigkdd international conference on knowledge discovery and data mining, ACM, Ithaca, 785–794, 2016.
Chini, L., Hurtt, G., Sahajpal, R., Frolking, S., Klein Goldewijk, K., Sitch, S., Ganzenmüller, R., Ma, L., Ott, L., Pongratz, J., and Poulter, B.: Land-use harmonization datasets for annual global carbon budgets, Earth Syst. Sci. Data, 13, 4175–4189, https://doi.org/10.5194/essd-13-4175-2021, 2021.
Cui, M., Qian, J., and Cui, L.: Developing precision agriculture through creating information processing capability in rural China, J. Rural Stud., 92, 237–252, https://doi.org/10.1016/j.jrurstud.2022.04.002, 2022.
Danylo, O., Pirker, J., Lemoine, G., Ceccherini, G., See, L., McCallum, I., Hadi, Kraxner, F., Achard, F., and Fritz, S.: A map of the extent and year of detection of oil palm plantations in Indonesia, Malaysia and Thailand, Sci. Data, 8, 96, https://doi.org/10.1038/s41597-021-00867-1, 2021.
Descals, A., Wich, S., Meijaard, E., Gaveau, D. L. A., Peedell, S., and Szantoi, Z.: High-resolution global map of smallholder and industrial closed-canopy oil palm plantations, Earth Syst. Sci. Data, 13, 1211–1231, https://doi.org/10.5194/essd-13-1211-2021, 2021.
Doughty, C. E., Keany, J. M., Wiebe, B. C., Rey-Sanchez, C., Carter, K. R., Middleby, K. B., Cheesman, A. W., Goulden, M. L., Da Rocha, H. R., Miller, S. D., Malhi, Y., Fauset, S., Gloor, E., Slot, M., Oliveras Menor, I., Crous, K. Y., Goldsmith, G. R., and Fisher, J. B.: Tropical forests are approaching critical temperature thresholds, Nature, 621, 105–111, https://doi.org/10.1038/s41586-023-06391-z, 2023.
Euler, M., Schwarze, S., Siregar, H., and Qaim, M.: Oil Palm Expansion among Smallholder Farmers in Sumatra, Indonesia, J. Agric. Econ., 67, 658–676, https://doi.org/10.1111/1477-9552.12163, 2016.
Ewert, F.: Modelling Plant Responses to Elevated CO2: How Important is Leaf Area Index?, Ann. Bot., 93, 619–627, https://doi.org/10.1093/aob/mch101, 2004.
Fagan, M. E., Kim, D., Settle, W., Ferry, L., Drew, J., Carlson, H., Slaughter, J., Schaferbien, J., Tyukavina, A., Harris, N. L., Goldman, E., and Ordway, E. M.: The expansion of tree plantations across tropical biomes, Nat. Sustain., 5, 681–688, https://doi.org/10.1038/s41893-022-00904-w, 2022.
Fang, H., Baret, F., Plummer, S., and Schaepman Strub, G.: An Overview of Global Leaf Area Index (LAI): Methods, Products, Validation, and Applications, Rev. Geophys., 57, 739–799, https://doi.org/10.1029/2018RG000608, 2019.
FAOSTAT: Crops and livestock products database, Food and Agriculture Organization of the United Nations, https://www.fao.org/faostat/en/{#}data/QCL2022 (last access: 28 November 2024), 2022.
Felbab-Brown, V.: An Atlas of Trafficking in Southeast Asia: the jagged edge: Illegal logging in Southeast Asia, IB Tauris & Co. Ltd. New York, ISBN 9780857721808, 2013.
Foley, J. A., DeFries, R., Asner, G. P., Barford, C., Bonan, G., Carpenter, S. R., Chapin, F. S., Coe, M. T., Daily, G. C., Gibbs, H. K., Helkowski, J. H., Holloway, T., Howard, E. A., Kucharik, C. J., Monfreda, C., Patz, J. A., Prentice, I. C., Ramankutty, N., and Snyder, P. K.: Global Consequences of Land Use, Science, 309, 570–574, https://doi.org/10.1126/science.1111772, 2005.
Forbes, B. C., Fauria, M. M., and Zetterberg, P.: Russian Arctic warming and “greening” are closely tracked by tundra shrub willows, Glob. Change Biol., 16, 1542–1554, https://doi.org/10.1111/j.1365-2486.2009.02047.x, 2010.
Galán-Acedo, C., Spaan, D., Bicca-Marques, J. C., de Azevedo, R. B., Villalobos, F., and Rosete-Vergés, F.: Regional deforestation drives the impact of forest cover and matrix quality on primate species richness, Biol. Conserv., 263, 109338, https://doi.org/10.1016/j.biocon.2021.109338, 2021.
Geist, H. J., and Lambin, E. F.: What Drives Tropical Deforestation, LUCC Report Series, 4, 116, 2001.
Ghini, R., Bettiol, W., and Hamada, E.: Diseases in tropical and plantation crops as affected by climate changes: current knowledge and perspectives, Plant Pathol., 60, 122–132, https://doi.org/10.1111/j.1365-3059.2010.02403.x, 2011.
Green, J. K., Ballantyne, A., Abramoff, R., Gentine, P., Makowski, D., and Ciais, P.: Surface temperatures reveal the patterns of vegetation water stress and their environmental drivers across the tropical Americas, Glob. Change Biol., 28, 2940–2955, https://doi.org/10.1111/gcb.16139, 2022.
Hansen, M. C., Potapov, P. V., Moore, R., Hancher, M., Turubanova, S. A., Tyukavina, A., Thau, D., Stehman, S. V., Goetz, S. J., Loveland, T. R., Kommareddy, A., Egorov, A., Chini, L., Justice, C. O., and Townshend, J. R. G.: High-Resolution Global Maps of 21st-Century Forest Cover Change, Science, 342, 850–853, https://doi.org/10.1126/science.1244693, 2013.
Harris, N. L., Brown, S., Hagen, S. C., Saatchi, S. S., Petrova, S., Salas, W., Hansen, M. C., Potapov, P. V., and Lotsch, A.: Baseline map of carbon emissions from deforestation in tropical regions, Science, 336, 1573–1576, https://doi.org/10.1126/science.1217962, 2012.
Houghton, R. A. and Nassikas, A. A.: Global and regional fluxes of carbon from land use and land cover change 1850–2015, Global Biogeochem. Cy., 31, 456–472, https://doi.org/10.1002/2016GB005546, 2017.
Hurtt, G. C., Chini, L., Sahajpal, R., Frolking, S., Bodirsky, B. L., Calvin, K., Doelman, J. C., Fisk, J., Fujimori, S., Klein Goldewijk, K., Hasegawa, T., Havlik, P., Heinimann, A., Humpenöder, F., Jungclaus, J., Kaplan, J. O., Kennedy, J., Krisztin, T., Lawrence, D., Lawrence, P., Ma, L., Mertz, O., Pongratz, J., Popp, A., Poulter, B., Riahi, K., Shevliakova, E., Stehfest, E., Thornton, P., Tubiello, F. N., van Vuuren, D. P., and Zhang, X.: Harmonization of global land use change and management for the period 850–2100 (LUH2) for CMIP6, Geosci. Model Dev., 13, 5425–5464, https://doi.org/10.5194/gmd-13-5425-2020, 2020.
Ito, A. and Hajima, T.: Biogeophysical and biogeochemical impacts of land-use change simulated by MIROC-ES2L, Prog. Earth Planet. Sci., 7, 54, https://doi.org/10.1186/s40645-020-00372-w, 2020.
Koh, L. P. and Wilcove, D. S.: Is oil palm agriculture really destroying tropical biodiversity?, Conserv. Lett., 1, 60–64, https://doi.org/10.1111/j.1755-263X.2008.00011.x, 2008.
Kuttippurath, J. and Kashyap, R.: Greening of India: Forests or Croplands?, Appl. Geogr., 161, 103115, https://doi.org/10.1016/j.apgeog.2023.103115, 2023.
Lan, X.: Atmospheric CO2 trends, NOAA Global Monitoring Laboratory and Scripps Institution of Oceanography, https://gml.noaa.gov/ccgg/trends/ (last access: 2 December 2024), 2024.
Liu, R., Liu, Y., and Chen, J.: GLOBMAP global Leaf Area Index since 1981 (Version 3.0), Zenodo [data set], https://doi.org/10.5281/zenodo.4700264, 2021.
Liu, X., Zheng, J., Yu, L., Hao, P., Chen, B., Xin, Q., Fu, H., and Gong, P.: Annual dynamic dataset of global cropping intensity from 2001 to 2019, Sci. Data, 8, 283, https://doi.org/10.1038/s41597-021-01065-9, 2021.
Liu, Y., Liu, R., and Chen, J. M.: Retrospective retrieval of long-term consistent global leaf area index (1981–2011) from combined AVHRR and MODIS data, J. Geophys. Res.-Biogeo., 117, G04003, https://doi.org/10.1029/2012JG002084, 2012.
Lundberg, M. and Lee, S.: A Unified Approach to Interpreting Model Predictions, Adv. Neur. In., Long Beach, California, USA, Curran Associates Inc., 4768–4777, ISBN: 9781510860964, 2017.
Lundberg, S. M., Erion, G., Chen, H., DeGrave, A., Prutkin, J. M., Nair, B., Katz, R., Himmelfarb, J., Bansal, N., and Lee, S.: From local explanations to global understanding with explainable AI for trees, Nat. Mach. Intell., 2, 56–67, https://doi.org/10.1038/s42256-019-0138-9, 2020.
Mann, H. B.: Nonparametric Tests Against Trend, Econometrica, 13, 245–259, https://doi.org/10.2307/1907187, 1945.
Mao, F., Li, X., Zhou, G., Huang, Z., Xu, Y., Chen, Q., Yan, M., Sun, J., Xu, C., and Du, H.: Land use and cover in subtropical East Asia and Southeast Asia from 1700 to 2018, Glob. Planet. Change, 226, 104157, https://doi.org/10.1016/j.gloplacha.2023.104157, 2023.
Meir, P., Wood, T. E., Galbraith, D. R., Brando, P. M., Da Costa, A. C. L., Rowland, L., and Ferreira, L. V.: Threshold Responses to Soil Moisture Deficit by Trees and Soil in Tropical Rain Forests: Insights from Field Experiments, Bioscience, 65, 882–892, https://doi.org/10.1093/biosci/biv107, 2015.
Meng, Y., Yang, N., Qian, Z., and Zhang, G.: What Makes an Online Review More Helpful: An Interpretation Framework Using XGBoost and SHAP Values, J. Theor. Appl. Electron. Commer. Res., 16, 466–490, https://doi.org/10.3390/jtaer16030029, 2021.
Miettinen, J., Shi, C., and Liew, S. C.: Deforestation rates in insular Southeast Asia between 2000 and 2010, Glob. Change Biol., 17, 2261–2270, https://doi.org/10.1111/j.1365-2486.2011.02398.x, 2011.
Muñoz Sabater, J.: ERA5-Land hourly data from 1950 to present, Copernicus Climate Change Service (C3S) Climate Data Store (CDS) [data set], https://doi.org/10.24381/cds.e2161bac, 2019.
Numata, I., Elmore, A. J., Cochrane, M. A., Wang, C., Zhao, J., and Zhang, X.: Deforestation, plantation-related land cover dynamics and oil palm age-structure change during 1990–2020 in Riau Province, Indonesia, Environ. Res. Lett., 17, 94024, https://doi.org/10.1088/1748-9326/ac8a61, 2022.
Panda, D., Mishra, S. S., and Behera, P. K.: Drought Tolerance in Rice: Focus on Recent Mechanisms and Approaches, Rice Sci., 28, 119–132, https://doi.org/10.1016/j.rsci.2021.01.002, 2021.
Park, T., Gumma, M. K., Wang, W., Panjala, P., Dubey, S. K., and Nemani, R. R.: Greening of human-dominated ecosystems in India, Commun. Earth Environ., 4, 419, https://doi.org/10.1038/s43247-023-01078-9, 2023.
Pedregosa, F., Varoquaux, G., Gramfort, A., Michel, V., Thirion, B., Grisel, O., Blondel, M., Prettenhofer, P., Weiss, R., Dubourg, V., Vanderplas, J., Passos, A., Cournapeau, D., Brucher, M., Perrot, M., and Duchesnay, É.: Scikit-learn: Machine learning in Python, J. Mach. Learn. Res., 12, 2825–2830 2011.
Piao, S., Wang, X., Park, T., Chen, C., Lian, X., He, Y., Bjerke, J. W., Chen, A., Ciais, P., Tømmervik, H., Nemani, R. R., and Myneni, R. B.: Characteristics, drivers and feedbacks of global greening, Nat. Rev. Earth Environ., 1, 14–27, https://doi.org/10.1038/s43017-019-0001-x, 2020a.
Piao, S., Wang, X., Park, T., Chen, C., Lian, X., He, Y., Bjerke, J. W., Chen, A., Ciais, P., Tømmervik, H., Nemani, R. R., and Myneni, R. B.: Characteristics, drivers and feedbacks of global greening, Nat.e Rev. Earth Environ., 1, 14–27, https://doi.org/10.1038/s43017-019-0001-x, 2020b.
Pocock, M. J. O., Evans, D. M., and Memmott, J.: The impact of farm management on species-specific leaf area index (LAI): Farm-scale data and predictive models, Agr. Ecosyst. Environ., 135, 279–287, https://doi.org/10.1016/j.agee.2009.10.006, 2010.
Potapov, P., Turubanova, S., Hansen, M. C., Tyukavina, A., Zalles, V., Khan, A., Song, X., Pickens, A., Shen, Q., and Cortez, J.: Global maps of cropland extent and change show accelerated cropland expansion in the twenty-first century, Nat. Food, 3, 19–28, https://doi.org/10.1038/s43016-021-00429-z, 2022.
Propastin, P. A.: Spatial non-stationarity and scale-dependency of prediction accuracy in the remote estimation of LAI over a tropical rainforest in Sulawesi, Indonesia, Remote Sens. Environ., 113, 2234–2242, https://doi.org/10.1016/j.rse.2009.06.007, 2009.
Querino, C. A. S., Beneditti, C. A., Machado, N. G., Da Silva, M. J. G., Da Silva Querino, J. K. A., Dos Santos Neto, L. A., and Biudes, M. S.: Spatiotemporal NDVI, LAI, albedo, and surface temperature dynamics in the southwest of the Brazilian Amazon forest, J. Appl. Remote Sens., 10, 26007, https://doi.org/10.1117/1.JRS.10.026007, 2016.
Rusli, N. and Majid, M. R.: Monitoring and mapping leaf area index of rubber and oil palm in small watershed area, IOP C. Ser. Earth Env., 18, 12035–12036, https://doi.org/10.1088/1755-1315/18/1/012036, 2014.
Satriawan, T. W., Luo, X., Tian, J., Ichii, K., Juneng, L., and Kondo, M.: Strong Green-Up of Tropical Asia During the 2015/16 El Niño, Geophys. Res. Lett., 51, e2023GL106955, https://doi.org/10.1029/2023GL106955, 2024.
Sitch, S., Friedlingstein, P., Gruber, N., Jones, S. D., Murray-Tortarolo, G., Ahlström, A., Doney, S. C., Graven, H., Heinze, C., Huntingford, C., Levis, S., Levy, P. E., Lomas, M., Poulter, B., Viovy, N., Zaehle, S., Zeng, N., Arneth, A., Bonan, G., Bopp, L., Canadell, J. G., Chevallier, F., Ciais, P., Ellis, R., Gloor, M., Peylin, P., Piao, S. L., Le Quéré, C., Smith, B., Zhu, Z., and Myneni, R.: Recent trends and drivers of regional sources and sinks of carbon dioxide, Biogeosciences, 12, 653–679, https://doi.org/10.5194/bg-12-653-2015, 2015.
Sulla-Menashe, D., Gray, J. M., Abercrombie, S. P., and Friedl, M. A.: Hierarchical mapping of annual global land cover 2001 to present: The MODIS Collection 6 Land Cover product, Remote Sens. Environ., 222, 183–194, https://doi.org/10.1016/j.rse.2018.12.013, 2019.
Sulla-Menashe, D. and Friedl, M. A.: User guide to collection 6 MODIS land cover (MCD12Q1 and MCD12C1) product, Usgs: Reston, Va, Usa, 1, 18, 2018.
Takeshima, H. J. P. K.: Overview of the agricultural modernization in Southeast Asia, Intl. Food Policy Res. Inst., 1819, 2019.
Vernimmen, R. R. E., Bruijnzeel, L. A., Romdoni, A., and Proctor, J.: Rainfall interception in three contrasting lowland rain forest types in Central Kalimantan, Indonesia, J. Hydrol., 340, 217–232, https://doi.org/10.1016/j.jhydrol.2007.04.009, 2007.
Vijay, V., Pimm, S. L., Jenkins, C. N., Smith, S. J., and Anand, M.: The Impacts of Oil Palm on Recent Deforestation and Biodiversity Loss, PLoS One, 11, e0159668, https://doi.org/10.1371/journal.pone.0159668, 2016.
Wagner, M., Wentz, E. A., and Stuhlmacher, M.: Quantifying oil palm expansion in Southeast Asia from 2000 to 2015: A data fusion approach, J. Land Use Sci., 17, 26–46, https://doi.org/10.1080/1747423X.2021.2020918, 2022.
Wang, J. A. and Friedl, M. A.: The role of land cover change in Arctic-Boreal greening and browning trends, Environ. Res. Lett., 14, 125007, https://doi.org/10.1088/1748-9326/ab5429, 2019.
Wang, L., Zhao, Z., Zhang, K., and Tian, C.: Reclamation and Utilization of Saline Soils in Arid Northwestern China: A Promising Halophyte Drip-Irrigation System, Environ. Sci. Technol., 47, 5518–5519, https://doi.org/10.1021/es4017415, 2013.
Wang, X., Blanken, P. D., Kasemsap, P., Petchprayoon, P., Thaler, P., Nouvellon, Y., Gay, F., Chidthaisong, A., Sanwangsri, M., Chayawat, C., Chantuma, P., Sathornkich, J., Kaewthongrach, R., Satakhun, D., and Phattaralerphong, J.: Carbon and Water Cycling in Two Rubber Plantations and a Natural Forest in Mainland Southeast Asia, J. Geophys. Res.-Biogeo., 127, e2022JG006840, https://doi.org/10.1029/2022JG006840, 2022.
Wang, Y., Hollingsworth, P. M., Zhai, D., West, C. D., Green, J. M. H., Chen, H., Hurni, K., Su, Y., Warren-Thomas, E., Xu, J., and Ahrends, A.: High-resolution maps show that rubber causes substantial deforestation, Nature, 623, 340–346, https://doi.org/10.1038/s41586-023-06642-z, 2023.
Watson, D. J.: Comparative Physiological Studies on the Growth of Field Crops, I. Variation in Net Assimilation Rate and Leaf Area between Species and Varieties and between Years, Ann. Bot., 11, 41–76 1947.
Wicke, B., Sikkema, R., Dornburg, V., and Faaij, A.: Exploring land use changes and the role of palm oil production in Indonesia and Malaysia, Land Use Policy, 28, 193–206, https://doi.org/10.1016/j.landusepol.2010.06.001, 2011.
Wu, T., Qu, C., Li, Y., Li, X., Zhou, G., Liu, S., Chu, G., Meng, Z., Lie, Z., and Liu, J.: Warming effects on leaf nutrients and plant growth in tropical forests, Plant Ecol., 220, 663–674, https://doi.org/10.1007/s11258-019-00943-y, 2019.
Xu, Y., Ciais, P., Yu, L., Li, W., Chen, X., Zhang, H., Yue, C., Kanniah, K., Cracknell, A. P., and Gong, P.: Oil palm modelling in the global land surface model ORCHIDEE-MICT, Geosci. Model Dev., 14, 4573–4592, https://doi.org/10.5194/gmd-14-4573-2021, 2021.
Xu, Y., Yu, L., Ciais, P., Li, W., Santoro, M., Yang, H., and Gong, P.: Recent expansion of oil palm plantations into carbon-rich forests, Nat. Sustain., 5, 574–577, https://doi.org/10.1038/s41893-022-00872-1, 2022.
Xu, Y., Yu, L., Li, W., Ciais, P., Cheng, Y., and Gong, P.: Annual oil palm plantation maps in Malaysia and Indonesia from 2001 to 2016, Earth Syst. Sci. Data, 12, 847–867, https://doi.org/10.5194/essd-12-847-2020, 2020.
Yan, F., Song, K., Liu, Y., Chen, S., and Chen, J.: Predictions and mechanism analyses of the fatigue strength of steel based on machine learning, J. Mater. Sci., 55, 15334–15349, https://doi.org/10.1007/s10853-020-05091-7, 2020.
Yang, C., Chen, M., and Yuan, Q.: The application of XGBoost and SHAP to examining the factors in freight truck-related crashes: An exploratory analysis, Accident Anal. Prev., 158, 106153, https://doi.org/10.1016/j.aap.2021.106153, 2021.
Yang, Y., Xiao, X., Li, M., Dong, Z., and Zhao, R.: A new framework for assessing carbon fluxes in alpine rivers, Catena, 246, 108423, https://doi.org/10.1016/j.catena.2024.108423, 2024.
Yuan, W., Zheng, Y., Piao, S., Ciais, P., Lombardozzi, D., Wang, Y., Ryu, Y., Chen, G., Dong, W., Hu, Z., Jain, A. K., Jiang, C., Kato, E., Li, S., Lienert, S., Liu, S., Nabel, J., Qin, Z., Quine, T., Sitch, S., Smith, W. K., Wang, F., Wu, C., Xiao, Z., and Yang, S.: Increased atmospheric vapor pressure deficit reduces global vegetation growth, Sci. Adv., 5, eaax1396, https://doi.org/10.1126/sciadv.aax1396, 2019.
Zeng, Z., Estes, L., Ziegler, A. D., Chen, A., Searchinger, T., Hua, F., Guan, K., Jintrawet, A., and F. Wood, E.: Highland cropland expansion and forest loss in Southeast Asia in the twenty-first century, Nat. Geosci., 11, 556–562, https://doi.org/10.1038/s41561-018-0166-9, 2018.
Zhang, A., Liu, Y., Wang, F., Kong, D., Bi, J., Zhang, F., Luo, X., Wang, J., Liu, G., Luo, L., and Yu, X.: Molecular Breeding of Water-Saving and Drought-Resistant Rice for Blast and Bacterial Blight Resistance, Plants, 11, 2641, https://doi.org/10.3390/plants11192641, 2022.
Zhao, H., Chang, J., Havlík, P., van Dijk, M., Valin, H., Janssens, C., Ma, L., Bai, Z., Herrero, M., Smith, P., and Obersteiner, M.: China's future food demand and its implications for trade and environment, Nat. Sustain., 4, 1042–1051, https://doi.org/10.1038/s41893-021-00784-6, 2021.
Zhao, J., Elmore, A. J., Lee, J. S. H., Numata, I., Zhang, X., and Cochrane, M. A.: Replanting and yield increase strategies for alleviating the potential decline in palm oil production in Indonesia, Agric. Syst., 210, 103714, https://doi.org/10.1016/j.agsy.2023.103714, 2023.
Zhao, R.: Code for Paper “cropland Expansion Drives Vegetation Greenness Decline in Southeast Asia”, Zenodo [code], https://doi.org/10.5281/zenodo.14259860, 2024.
Zhao, R., Zhang, W., Duan, Z., Chen, S., and Shi, Z.: An improved estimate of soil carbon pool and carbon fluxes in the Qinghai-Tibetan grasslands using data assimilation with an ecosystem biogeochemical model, Geoderma, 430, 116283, https://doi.org/10.1016/j.geoderma.2022.116283, 2023.
Zhong, L., Ma, Y., Xue, Y., and Piao, S.: Climate Change Trends and Impacts on Vegetation Greening Over the Tibetan Plateau, J. Geophys. Res.-Atmos., 124, 7540–7552, https://doi.org/10.1029/2019JD030481, 2019.
Zhu, Z., Piao, S., Myneni, R. B., Huang, M., Zeng, Z., Canadell, J. G., Ciais, P., Sitch, S., Friedlingstein, P., Arneth, A., Cao, C., Cheng, L., Kato, E., Koven, C., Li, Y., Lian, X., Liu, Y., Liu, R., Mao, J., Pan, Y., Peng, S., Peñuelas, J., Poulter, B., Pugh, T. A. M., Stocker, B. D., Viovy, N., Wang, X., Wang, Y., Xiao, Z., Yang, H., Zaehle, S., and Zeng, N.: Greening of the Earth and its drivers, Nat. Clim. Change, 6, 791–795, https://doi.org/10.1038/nclimate3004, 2016.