the Creative Commons Attribution 4.0 License.
the Creative Commons Attribution 4.0 License.
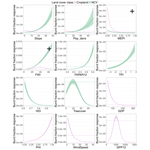
Understanding and simulating cropland and non-cropland burning in Europe using the BASE (Burnt Area Simulator for Europe) model
Matthew Forrest
Jessica Hetzer
Maik Billing
Simon P. K. Bowring
Eric Kosczor
Luke Oberhagemann
Oliver Perkins
Dan Warren
Fátima Arrogante-Funes
Kirsten Thonicke
Thomas Hickler
Fire interacts with many parts of the Earth system. However, its drivers are myriad and complex, interacting differently in different regions depending on prevailing climate regimes, vegetation types, socioeconomic development, and land use and management. Europe is facing strong increases in projected fire weather danger as a consequence of climate change and has experienced extreme fire seasons and events in recent years. Here, we focus on understanding and simulating burnt area across a European study domain using remote sensing data and generalised linear models (GLMs). We first examined fire occurrence across land cover types and found that all non-cropland vegetation (NCV) types (comprising 26 % of burnt area) burnt with similar spatial and temporal patterns, which were very distinct from those in croplands (74 % of burnt area). We then used GLMs to predict cropland and NCV burnt area at km and monthly spatial and temporal resolution, respectively, which together we termed BASE (Burnt Area Simulator for Europe). Compared to satellite burnt area products, BASE effectively captured the general spatial and temporal patterns of burning, explaining 32 % (NCV) and 36 % (cropland) of the deviance, and performed similarly to state-of-the-art global fire models. The most important drivers were fire weather and monthly indices derived from gross primary productivity followed by coarse socioeconomic indicators and vegetation properties. Crucially, we found that the drivers of cropland and NCV burning were very different, highlighting the importance of simulating burning in different land cover types separately. Through the choice of predictor variables, BASE was designed for coupling with dynamic vegetation and Earth system models and thus enabling future projections. The strong model skill of BASE when reproducing seasonal and interannual dynamics of NCV burning and the novel inclusion of cropland burning indicate that BASE is well suited for integration in land surface models. In addition to this, the BASE framework may serve as a basis for further studies using additional predictors to further elucidate drivers of fire in Europe. Through these applications, we suggest BASE may be a useful tool for understanding, and therefore adapting to, the increasing fire risk in Europe.
- Article
(5440 KB) - Full-text XML
-
Supplement
(3298 KB) - BibTeX
- EndNote
Fire is recognised as a fundamental ecological force (McLauchlan et al., 2020); a key component of the Earth system (Archibald et al., 2018; Bowman et al., 2009); and a serious hazard for human health, livelihoods, property, wildlife, and biodiversity (Arrogante-Funes et al., 2024; Bowman et al., 2020; Johnston et al., 2012; Sullivan et al., 2022). It interacts with many components of the Earth system, with notable effects on biogeochemical cycles, surface energy budgets, and vegetation dynamics and composition (Archibald et al., 2018; Bowman et al., 2009). Through these effects, fire alters the chemical composition of the atmosphere and the physical properties of the land surface, thereby influencing regional and global climate (Archibald et al., 2018; Bowman et al., 2009; Jones et al., 2022). Total global burnt area (including fires set deliberately for land management) is decreasing, which is primarily driven by decreases in fire in savanna, grassland, and cropland regions (Andela et al., 2017). However, the frequency of extreme wildfires is increasing (Cunningham et al., 2024) as is forest area loss due to fire (Tyukavina et al., 2022). Many regions are experiencing wildfires of hitherto unrecorded extent and/or severity, e.g. 2019/2020 in Australia (Boer et al., 2020) and 2023 in Canada (Hu et al., 2024). Studies of regional fire dynamics can help resolve these complexities by revealing region-specific processes and drivers whilst also providing results which can inform policy at local, national, and transnational levels. One such region is Europe, which is experiencing unprecedented wildfires (San-Miguel-Ayanz et al., 2023). The already fire-prone region of southern Europe has been experiencing extreme fire seasons with difficult to control fires, for example, in Portugal in 2017 (Turco et al., 2019), Greece in 2018 (Giannaros et al., 2022), and southwestern Europe in 2022 (Rodrigues et al., 2023). In northern and central Europe, regions which were not previously considered fire-prone are now experiencing wildfires (Arnell et al., 2021; Krüger et al., 2023). Even moderate climate change scenarios show large increases in fire danger due to fire weather changes (El Garroussi et al., 2024; Turco et al., 2018). Thus, there is an urgent need to understand and simulate fire occurrence on the European scale.
However, whilst the basic physical prerequisites of fire occurrence can be summarised fairly simply as a sufficient amount of spatially continuous, suitably aerated, dry fuel and an ignition source, understanding where and when these conditions are fulfilled and how large the resulting fires become is rather more complex. Meteorological conditions (“fire weather”) at the time of a fire affect its rate of spread, and conditions in antecedent days affect the moisture content of both live and dead fuels. Vegetation, the primary fuel source, varies tremendously across the planet, resulting in large heterogeneity in fuel conditions in terms of both fuel moisture and physical flammability characteristics (i.e. leafy vs. woody, dead vs. live fuel, and fuel particle dimensions). Human activity and infrastructure account for the majority of fire ignitions (responsible for 96 % of burnt area in Europe; Dijkstra et al., 2022), but lightning and other natural ignitions also occur. Humans may start fires for a myriad of reasons (including negligence and arson) which vary depending on land use type and cultural practices, but humans also work to suppress fires (Millington et al., 2022). Legislation and law enforcement also play a role if fire practices are allowed to manage the landscape or how well firefighting techniques are funded and can be applied. Land use also affects the vegetation and hence fuel conditions and introduces barriers to fire spread into the landscape. Topography affects rate of spread and can also introduce barriers to fire spread. In summary, we find a plethora of factors affecting fire occurrence and expect them to function differently depending on the local vegetation, socioeconomic development, and human activity.
Two modelling approaches have typically been used to study fire occurrence from an Earth system perspective or on large scales. Process-based fire models coupled to land surface and dynamic global vegetation models (DGVMs) have been used for studying fire dynamics by simulating biophysical mechanisms and some socioeconomic factors across a range of complexities (Hantson et al., 2016). Complementary approaches using correlative methods have been developed using either statistical models (such as generalised linear models, GLMs; for example, Bistinas et al., 2014; Haas et al., 2022) or machine learning models (typically random forests; Forkel et al., 2017; Kuhn-Régnier et al., 2021; Mukunga et al., 2023). These approaches typically use a larger set of input variables, include more socioeconomic variables, and use observed vegetation. Both types of models are usually applied at a global level and so are inherently focussed on matching the global pattern of burnt area. This global pattern is dominated by grass fires in the tropics, particularly Africa, and the fire-enabled DGVMs do a reasonable job simulating this (Hantson et al., 2020). However, they have notable regional discrepancies and in particular overpredict burnt area in the extra tropics (see Fig. 4 and Table 2 in Hantson et al., 2020) likely because their global focus leaves them unable to resolve regionally specific processes or phenomena. On the other hand, national- and sub-national-scale studies are inherently limited in the range of environmental and socioeconomic conditions that they encompass and hence in their broader applicability. Thus, there is a need to develop models focussed on intermediate (i.e. continental) scales (Boulanger et al., 2018; Keeping et al., 2024; Turner et al., 2011) where there is large variation (and hence applicability), but the patterns are not overpowered by the tropical savannas, and the model is sensitive to regionally important phenomena.
Concerning fire regimes on the pan-European scale, recent studies have described and quantified fire regimes in Europe (Galizia et al., 2021), estimated the fractions of anthropogenic vs. lightning ignitions and their contribution to burnt area (Dijkstra et al., 2022), investigated drivers of large to extreme fire occurrence in terms of individual events (Ochoa et al., 2024), and examined the compounding effects of fire and other hazards (Sutanto et al., 2020). Other research has focussed on relating burnt area to fire weather variables in different regions of southern Europe, such as districts of Portugal (Carvalho et al., 2008), NUTS3 subregions (Turco et al., 2018), and Mediterranean countries (Amatulli et al., 2013). There have also been a number of DGVM studies which used global fire models to project future changes in burnt area in Europe but which do not specifically focus on the driving factors and have only limited regional adaptation for Europe (Dury et al., 2011; Migliavacca et al., 2013; Wu et al., 2015; Khabarov et al., 2016). We are not aware of any study examining drivers of burnt area which simultaneously (i) considers specifically the pan-European scale, (ii) considers drivers beyond fire-weather-related variables, and (iii) operates at a gridded resolution for integration with DGVMs and which is necessary for including highly heterogeneous topographic, socioeconomic, and vegetative factors. Furthermore, Europe has a diverse array of land cover types, and these have not been distinguished between in previous studies. This is of particular importance given recent advances in satellite observation of burnt areas which indicate occurrences of fire in croplands that are higher than previously estimated (Hall et al., 2024; Roteta et al., 2019). Cropland burning as an explicit process is almost entirely neglected in fire-enabled DGVMs and the land surface models used in Earth system models (ESMs); we are aware of only one such model in which it is simulated (Li et al., 2013), one in which it is prescribed from remote sensing data (Rabin et al., 2018) and one in which fires in croplands are simulated in the same manner as fires in grasslands (Burton et al., 2019).
Here we seek to fill this knowledge gap by disentangling the drivers of fire occurrence across a European study domain (defined here as 27 countries of the European Union with the United Kingdom and six Balkan candidate countries). This study's aims are twofold: (i) to gain insight into drivers of fire activity across land cover types in Europe and (ii) to encapsulate this knowledge into a new fire model that can be embedded into a DGVM. To fulfil these aims, we chose to use GLMs. As a correlative method, GLMs have the advantage over process-based models that they are highly data-driven and therefore can tease out process understanding rather than only embodying existing knowledge. But also, compared to more complex correlative techniques (for example random forests), GLMs can be described by a handful of coefficients and so can easily be embedded into other models. As a preamble to developing the GLMs, we first examined fire and land cover data to determine which broad land cover categories should be simulated. We then fitted GLMs to determine which environmental and socioeconomic variables can explain fire behaviour in Europe and produce parsimonious predictive models.
2.1 Datasets
This study relied solely on gridded datasets, with the common spatial resolution determined by a state-of-the-art climate dataset with 0.07(03135)°, which corresponds to approximately 9×9 km, derived from ERA5-Land (Muñoz-Sabater et al., 2021). This dataset was selected in order to provide comparatively fine spatial resolution and compatibility with the FirEUrisk Assessment System (Chuvieco et al., 2023) and is available until 2014. For clarity, we refer to elements of the target 9 km grid as grid cells and elements of higher-resolution grids as pixels. Unless otherwise noted, all data processing was done using R (R Core Team, 2024) and the terra package (Hijmans, 2023).
2.1.1 Fire occurrence and land cover combination
Central to this analysis was the combination of ESA FireCCI51 (Lizundia-Loiola et al., 2020) and ESA Land Cover CCI (ESA, 2017) datasets, which we used to quantify fire occurrence in different land cover types (LCTs) in two different ways: burnt area, BA (ha), and burnt fraction, BF (unitless), on a monthly basis. We also calculated the fraction of a grid cell covered by an LCT, LF (unitless). To combine these products, we first performed nearest-neighbour regridding to bring the 300 m land cover data on to the 250 m grid of the burnt area data. Then, for a given LCT, month, and 9 km grid cell, we calculated BPLCT, the number of burnt pixels in the grid cell (from the FireCCI51 pixel product land cover layer), and TPLCT, the total number of pixels of that LCT (from the regridded Land Cover CCI product). We also calculated the total area of the 9 km grid cell, A (ha), and the number of 250 m pixels within that 9 km grid cell, TP.
We calculated burnt fraction (unitless) by
Land cover type fraction (unitless) was calculated by
And, finally, burnt area (ha) was calculated by
Note that this method accounts for the variation in grid cell size with latitude but not the (far smaller) variation in pixel size within a 9 km grid cell.
Burnt fraction was used as the target variable for model fitting, and mean burnt fraction (averaged across grid cells) was used for comparing temporal patterns of fire occurrence between LCTs. Burnt area was used for comparing predicted fire occurrence to the observed patterns in terms of both agreement metrics and visualisation and comparing the overall amounts of burnt area present in the study area.
2.1.2 Fire weather and wind speed
To capture fire weather, we used an adapted version of the Canadian Forest Fire Weather Index (FWI) (Van Wagner, 1987) that considers the total daily precipitation combined with the daily temperature, relative humidity, and wind speed at noon. Here we calculated it using the implementation of the Canadian Forest Fire Danger Rating System in the R package cffdrs, which calculates the FWI and all subindices (Wang et al., 2017b). The climate variables required were taken from a version of the ERA5-Land climate dataset, which was produced by regridding the original triangular–cubic–octahedral (TCo1279) operational grid from the reanalysis simulations (Muñoz-Sabater et al., 2021) to a regular 9 km grid (∼0.07°) across Europe (Chuvieco et al., 2023) in order to maintain a higher spatial resolution than the standard 0.1° resolution. We used accumulated daily precipitation (in mm), the noon values were approximated using the maximum daily temperature (in °C), the minimum relative humidity (in %) and the daily mean wind speed (in km h−1) by the approach of Hetzer et al. (2024). Monthly averages were calculated from the daily FWI values. We also considered the monthly mean and maximum of wind speed from this climate dataset as candidate predictors.
2.1.3 Gross primary productivity and derived quantities
We considered gross primary productivity (GPP) and quantities derived from it as potential predictors for fuel accumulation and ecosystem state. The monthly version of the Global OCO-2 SIF GPP product (GOSIF, Li and Xiao, 2019) was regridded from its native 0.05° resolution to the target grid using average resampling. From these monthly values, we calculated the sum of the antecedent 12 months (GPP12) following Kuhn-Régnier et al. (2021) to quantify fuel buildup. We also derived two indices to quantify ecosystem state and post-harvest timing (only for use in the cropland burning model). We define the monthly ecosystem productivity index (MEPI) as this month's GPPm divided by the maximum of the 13 previous months (including this month); i.e. the following applies:
MEPI therefore ranges between 0 and 1. High values indicate that photosynthesis is occurring at close to its maximum rate, and so the ecosystem is in an unstressed state with full leaf expansion – i.e. high proportions of live fuel and high live fuel moisture content and thus low expected flammability. Low values imply either a dormant state (i.e. leaves senesced and higher dead fuel proportion) or a stressed state, which we expect to correspond to higher flammability. Using the 13-month maximum accounts for the overall productivity of a grid cell in a manner which is insensitive to the length of the growing season (unlike the annual mean).
We defined the post-harvest index (PHI) by
The logic behind PHI is that crop residue burning is likely to happen when productivity for the previous 3 months has been high relative to the annual maximum. Such a situation indicates a productive growth period for the crops after which point the crops can be harvested, creating an opportunity for residue burning. Note that we expect the opposite response for PHI compared to MEPI, with high values of PHI indicating a higher likelihood of fire occurrence but low values of MEPI indicating higher likelihood.
2.1.4 Fraction of absorbed photosynthetically active radiation
The fraction of absorbed photosynthetically active radiation (FAPAR) is a proxy for live leaf biomass and can be used to quantify fine fuel buildup and availability (Forkel et al., 2017; Knorr et al., 2016; Kuhn-Régnier et al., 2021). Here we used the FAPAR 1km v2 product by the Copernicus Global Land Service (CGLS), which is derived from SPOT-VEGETATION and PROBA-V data (European Commission Directorate-General Joint Research Centre, 2020). It is originally provided at 1 km resolution globally but was aggregated and regridded to the 9 km target grid using pixel averaging and bilinear interpolation. It covers the analysis period in 10 d steps until June 2020. For each time step, the final consolidation product RT6 was used.
2.1.5 Tree cover
The degree of tree cover affects fuel load and composition, local wind speed, and fuel moisture (due to subcanopy microclimates). A recent global study indicated that tree cover has a negative effect on burnt area (Haas et al., 2022). For maximum precision, we used the global 30 m Landsat tree canopy version 4 product (Sexton et al., 2013), which was processed to 9 km resolution by simple averaging. We took the mean of the layers for 2000, 2005, 2010, and 2015 to smooth out occasional anomalous values seen in the individual layers.
2.1.6 Population density
The presence of humans has long been recognised as affecting fire occurrence and population density and is widely used in global fire models (Hantson et al., 2016; Rabin et al., 2017) and empirical studies of global fire patterns (Bistinas et al., 2014; Haas et al., 2022). For this study, population density was acquired from the HYDE v3.2 database baseline version (Klein Goldewijk et al., 2017). This was converted from ASCII to netCDF format using GDAL (Rouault et al., 2024). Annual maps were created by linearly interpolating between the 5-yearly population density estimates, performed using Climate Data Operators (Schulzweida, 2023) and remapped to this study's ∼9 km spatial resolution.
2.1.7 Human development index and gross domestic product
Both the human development index (HDI) and gross domestic product (GDP) have been used as socioeconomic indicators to represent human effects on fire regimes. Li et al. (2013) implemented a suppression of both non-cropland and cropland fires with increasing GDP per capita in a global fire model. More recently, Chuvieco et al. (2021) used HDI in an analysis to explain variability in burnt area and found that increasing HDI dampens burnt area interannual variability. Here both HDI and GDP per capita were taken from the datasets from Kummu et al. (2018) and regridded to the target 9 km resolution by simple averaging. This dataset introduced a small data gap in Northern Macedonia.
2.1.8 Topographic variables
The interactions between terrain and fire spread can be highly complex and variable (Sharples, 2009). On the one hand, rough terrain can be expected to increase fire size by increasing fire spread rate on a local scale on slopes (e.g. Rothermel et al., 1972) and by limiting access to firefighters. On the other hand, it may reduce fire size by introducing barriers to fire spread. In their study at 0.5° resolution, Haas et al. (2022) found that the vector ruggedness measure, a measure of terrain roughness, had a negative effect on burnt area in a grid cell. In contrast, the topographic position index (TPI), which quantifies the relative proportions of hill tops to valley floors, was found to have a slightly positive effect. Here we extracted a set of terrain variables from the Geomorpho90 dataset, which is based on the 90 m resolution MERIT digital elevation model (Amatulli et al., 2020). We masked out pixels with more than 50 % urban or permanent water bodies based on version 3 of the Copernicus Land Cover dataset (Buchhorn et al., 2020) as such pixels are not expected to burn and influence fire behaviour. We then aggregated these to the target grid by calculating the median of pixels using Google Earth Engine (Gorelick et al., 2017). We found that at our target resolution of ∼9 km, all the terrain variables fell into two groups of strongly correlated variables (data not shown). From these groups, we picked slope and TPI because of their relative simplicity of interpretation and for comparability with other studies.
2.2 Analysis of fire occurrence by land cover types
Before performing the main task of building GLM models, we first grouped land cover types based on their relative contributions to the total burnt area and their spatiotemporal patterns of burning. We therefore examined fire occurrence in land cover types using the ESA Land Cover CCI dataset. We first of all separated cropland from non-cropland areas to form two main land cover types and then divided these types into subtypes. For croplands, we considered the following subtypes: herbaceous croplands, woody croplands, and mosaic croplands. For non-croplands we considered grasslands, shrublands, woodlands, natural mosaics, and sparse vegetation (see Table S1 in the Supplement for more details).
We then compared the mean annual burnt area in each of our aggregated classes to determine their relative contributions to fire occurrence in Europe, indicating which classes are most important to simulate. To determine how we might group the subtypes, we examined the spatial patterns of burnt area, and the interannual variability and season cycle of mean grid cell burnt fraction. Based on this, we concluded that it would be sufficient to build separate models for only two land cover types: croplands (excluding woody and mosaic cropland types) and non-cropland vegetation (hereafter NCV).
2.3 GLM fitting
We fitted GLMs for the NCV and cropland LCTs using the standard glm function in R over the period of 2002–2014 (determined by the climate dataset). The quasi-binomial family was used to account for the high degree of overdispersion (large amount of zero values) in the data with the logit link function. Note that the use of a “quasi” family precluded the use of some standard GLM tools such a Q–Q plots and information criteria because there is no clear generating model. We also chose not to scale the predictors in order to maintain maximum interpretability of the results, but our testing showed that scaling made no difference to model fit results.
We considered every month and grid cell which had more than 10 % of the LCT present as a data point and used 80 % of the data points (sampled randomly from all grid cell months) for training and kept 20 % for testing. When comparing the normalised mean error (NME; Kelley et al., 2013) between the testing and training, we saw differences of ∼0.002.
2.4 Predictor variable selection
For predictor variable selection, we took an approach that could be summarised as “process-informed trial and error”. This was chosen over automated variable selection methods because we wanted to select and test specific variables to capture specific effects or processes. This means, for example, that at least one variable that is an indicator of fuel availability and one for fire weather must be maintained in the model. As the model was developed, variables were added, substituted, or removed. Interaction terms and different responses (e.g. quadratic terms) were also tested. This required continuous evaluation of model performance and of the responses of individual variables. We also minimised the degree of correlation between predictors by testing only one predictor from any set of highly correlated variables (say FAPAR and GPP or HDI and GDP) at a time (for correlations between predictors, see Fig. S6 in the Supplement). Automated variable selection does not allow for this flexibility nor does it allow informed decision-making anchored in process understanding. We therefore present the outcome of this variable selection fait accompli, but we also present a table of sensitivity of model results, with predictors changed/removed and plots of the effects of removing certain key terms.
2.5 Evaluating model fit and behaviour
The statistical models generated here can be viewed as both a GLM and a simulator of fire occurrence for use in an ESM context and, as such, can be evaluated through these two lenses. When viewed as a GLM, we evaluated the models using deviance explained; we made partial response and residual plots for each predictor on the link scale as is typically done for such analyses using the R visreg package (Breheny and Burchett, 2017), and we calculated variable importance using a SHAP-derived variable importance ranking using the R vip package (Greenwell and Boehmke, 2020). Note that because we did not scale the predictors and did choose to use interaction and polynomial terms, we avoided the use of predictor coefficients or t statistics to compare variable importance. Overall, this form of evaluation looks at the model's ability to predict burnt fraction, with equal weight given to all grid cells in the training dataset (regardless of how much of an LCT is present in the grid cell or what time of year it is) and does not consider details of the spatiotemporal patterns.
We also undertook complementary evaluation of predicted burnt area using methods that are more typically used in an ESM/DGVM context. We plotted the spatial, interannual, and seasonal patterns of burnt area and compared them to the observations. We also calculated the normalised mean error (NME) of the spatial and interannual burnt area distribution and the mean phase difference (MPD) to quantify the seasonal agreement, all following Kelley et al. (2013), compared to the data (over the full datasets, not just the training or testing subsample). We also plotted the predictor responses on the response scale (with all other predictors held at their median values) and compared them between the LCTs to give a more “real-world” idea of how these predictors act. It should be noted that these evaluations were done with burnt area as opposed to burnt fraction, so when the model predictions are aggregated for the spatial temporal plots, they implicitly weight the grid cells' contribution by the fraction of LCT present. This implies that grid cells with less of a LCT present contribute less to the plot.
3.1 Analysis of observed fire occurrence by land cover type
Of the 1.46 Mha yr−1 of burnt area in our European study domain between 2001 and 2020, the majority occurred in croplands, with a mean of 1.09 Mha yr−1 (74 % of total) (Fig. 1a). The majority of this was in herbaceous croplands (0.98 Mha yr−1), with a much smaller contribution from mosaic croplands (0.08 Mha yr−1) and a very small amount in woody croplands (0.01 Mha yr−1). Given that cropland burning comprised three-quarters of total burnt area, we conclude that our study should include cropland burning. Furthermore, since 90 % of this burning happened in herbaceous cropland, we decided to neglect burning in the other crop land cover types and consider only herbaceous cropland with the expectation that this corresponds to the practice of burning crop residues.
A further 0.38 Mha yr−1 (26 % of total burnt area) is burnt in non-cropland vegetation (Fig. 1a). The largest contributions to this were from the woodlands and natural mosaics categories (0.13 and 0.12 Mha yr−1, respectively), with smaller contributions from grasslands (0.06 Mha yr−1) and shrublands (0.03 Mha yr−1) and a negligible contribution from sparse vegetation (0.003 Mha yr−1).
Although both NCV and cropland burning are generally confined to southern Europe, the spatial patterns of burnt fraction were rather distinct (Fig. 1b). Cropland burning was concentrated in the Balkans, particularly in Bulgaria and Romania on their respective sides of the Danube and around northern Serbia, and with some further patches in Italy and less intense patches on the Iberian Peninsula. In contrast, NCV burning was most intense in Portugal, with further patches across much of the Mediterranean, including particular hotspots in Sicily, on the Balkan Adriatic coast, and in northern Serbia. Comparing the normalised time series of burnt fraction of cropland and NCV revealed very different interannual variabilities (IAVs; Fig. 1c). The seasonal distribution of the burnt fraction (Fig. 1d) also showed some considerable differences, with a broader summer peak and distinctive October shoulder in the cropland burning. Despite the fact that NCV and cropland burning showed very different spatiotemporal patterns, our analysis of the NCV subtypes (including grasslands, shrublands, and woodlands) revealed remarkably similar distributions (see Figs. S3–S5). From this we concluded that it would be sufficient to build GLMs for only two broad land cover classes to capture the fire patterns in Europe: herbaceous croplands (henceforth just croplands) that experience residue burning and all other non-cropland vegetation (NCV) which primarily experience uncontrolled wildfires, which we refer to as BASE Cropland and BASE NCV, respectively.
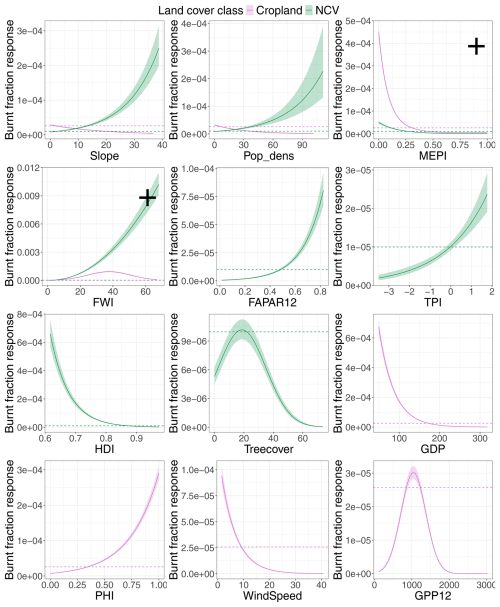
Figure 2Partial responses of burnt area to each predictor variable for the NCV (green line and shaded area) and cropland (purple line and shaded area) burning models. Dashed lines for the NCV and cropland burning model mark the burnt area predicted by the BASE models when all predictors are held at their median values. Pops_dens is the population density, MEPI is the monthly ecosystem productivity index, FWI is the fire weather index, FAPAR12 is the fraction of absorbed photosynthetically active radiation averaged over the last 12 months, HDI is the human development index, GDP is the gross domestic product (per capita), PHI is the post-harvest index, and GPP12 is the gross primary productivity summed over the previous 12 months.
3.2 BASE deviance explained and predictors
The final selection of predictors is shown in Table 1 and the associated regression coefficients in Tables S2 and S3. In terms of raw deviance explained, the fitted GLMs did moderately well, explaining 32.4 % of the deviance of NCV burning and 36.0 % of cropland burning (Tables 2 and 3).
For the BASE NCV, the SHAP-derived importance scores indicated that the most important driver was FWI (log-transformed) closely followed by MEPI (Fig. 2). These relationships were positive and negative, respectively, as would be expected (Fig. 3). HDI (negative response), tree cover (unimodal response), and FAPAR12 (positive response) formed a group each with approximately one-third of the importance, closely followed by topographic slope (positive response). TPI and Pop_dens were of small importance, and both had a positive response. Additionally, we found that including an interaction between FWI and MEPI had a small beneficial effect on the reproduction of the IAV and seasonal cycle (see Sect. S6 in the Supplement for details).
In BASE Cropland, MEPI was the most important determinant variable, with a negative response best represented in quadratic form (Figs. 2 and 3). PHI (positive), FWI (unimodal), GDP (negative), and GPP12 (unimodal) all showed high and similar levels of importance (about two-thirds that of MEPI) and wind speed, population density, and slope showed less importance (all negative). We further note that many predictors produced contrasting responses and different functional forms in the NCV versus the cropland model (Fig. 2).
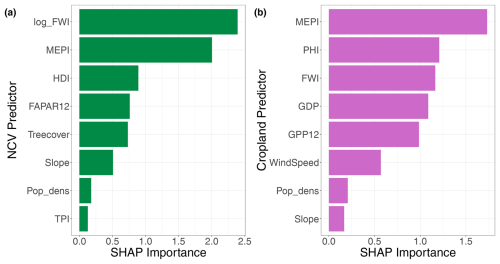
Figure 3SHAP-derived variable importance for (a) the NCV and (b) the cropland models. For variable descriptions, see Table 1. Pops_dens is the population density, MEPI is the monthly ecosystem productivity index, FWI is the fire weather index, FAPAR12 is the fraction of absorbed photosynthetically active radiation averaged over the last 12 months, HDI is the human development index, GDP is the gross domestic product (per capita), PHI is the post-harvest index, and GPP12 is the gross primary productivity summed over the previous 12 months.
Table 2Model skill metrics for best NCV burning model and the differences relative to the model for sensitivity models. Changes above 0.005 are highlighted in italics if they correspond to model improvement and bold if they correspond to model degradation. The numbers in parentheses in column titles refer to the bootstrap null model. Pops_dens is the population density, MEPI is the monthly ecosystem productivity index, FWI is the fire weather index, FAPAR12 is the fraction of absorbed photosynthetically active radiation averaged over the last 12 months, HDI is the human development index, GDP is the gross domestic product (per capita), and GPP12 is the gross primary productivity summed over the previous 12 months.
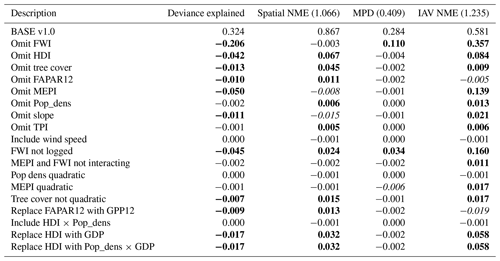
Table 3Model skill metrics for best cropland burning model and the differences relative to the model for sensitivity models. Changes above 0.005 are highlighted in italics if they correspond to model improvement and bold if they correspond to model degradation. The numbers in parentheses in column titles refer to the bootstrap null model. Pops_dens is the population density, MEPI is the monthly ecosystem productivity index, FWI is the fire weather index, HDI is the human development index, GDP is the gross domestic product (per capita), PHI is the post-harvest index, and GPP12 is the gross primary productivity summed over the previous 12 months.
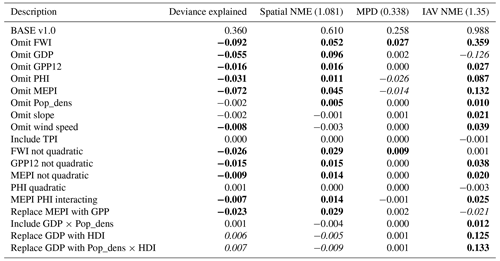
3.3 NCV model performance
The spatial patterns of burning simulated by BASE NCV matched the ESA FireCCI51 data reasonably well (Fig. 4) as evidenced by an NME score of 0.87 (Table 2). Burnt area occurred and was simulated largely in southern Europe on the Iberian Peninsula, in the Balkans, in Italy, and on Mediterranean islands. However there were some regional mismatches. The most striking mismatch is the large overestimation by BASE in Spain and the simultaneous underestimation in Portugal. BASE also overestimated burning in Sardinia and Greece but failed to simulate the high amount of burning along the Balkan Adriatic coast. Observational data also showed some areas of fire occurrence in temperate and boreal Europe (these may correspond to a single fire event) which were not simulated by BASE. The IAV was well captured (Fig. 5), with an NME of 0.58 and the reproduction of both the observed weakly declining trend and the timing of peak fire years (although peak amplitudes were underestimated). The model also reproduced the observed timing of both spring and summer fire peaks but underestimated their magnitude (Fig. 6) and produced an overall MPD of 0.28.
3.4 Cropland model performance
BASE Cropland successfully simulated the large extents of cropland burning in the Balkans, Greece, and Italy (Fig. 4) and gave a good overall spatial NME of 0.61 (Table 3). It did, however, considerably overestimate cropland burning across the Iberian Peninsula, where little burning was present in the ESA FireCC51 data. In terms of interannual variability, the model did less well (Fig. 5), with an NME of 0.99. The model reasonably reproduced the observed seasonal timing of cropland burning, with a MPD of 0.26 but underestimated the length of the summer fire peak considerably and missed the spring peak (Fig. 6).
3.5 Alternative model formations
Tables 2 and 3 show the performance of the fitted sensitivity models. Changes equal to or larger than 0.005 (i.e. 0.5 % change in deviance explained or NME) are in bold when they decreased model fit and italics if they improved it. In general, all changes from the chosen model either worsened model agreement metrics or had negligible impact. In the rare case that a metric improved, it was almost always accompanied by a larger decrease in performance as measured with other metrics.
One noteworthy result is the large degradation of the IAV performance of BASE Cropland associated with the inclusion of GDP, which resulted in a 13 % decrease in IAV NME. However, this was set against improvements in deviance explained and spatial NME (6 % and 10 %, respectively). We also investigated swapping HDI for GDP, which resulted in a further 13 % degradation of IAV NME with only very small improvements in deviance explained and spatial NME. Examination of the temporal trends showed that HDI was responsible for introducing a decreasing trend in the cropland burning model that is not observed in the data (Fig. S11), which explains its deleterious effect on IAV NME. We also note that the inclusion of either HDI or GDP in the cropland burning model improves the broad spatial patterns markedly by increasing cropland burning in the Balkans and decreasing it in western Europe (Fig. S12). We therefore chose to include GDP because of its lesser negative impact on the IAV NME. In contrast, in BASE NCV, we found that the inclusion of HDI improved the description of the trend as the simulations correctly produced the slightly decreasing trend found in the data as opposed to an incorrectly increasing trend without HDI included (Fig. S13). We also found that HDI was a superior predictor to GDP in all respects for NCV burning (Table 2).
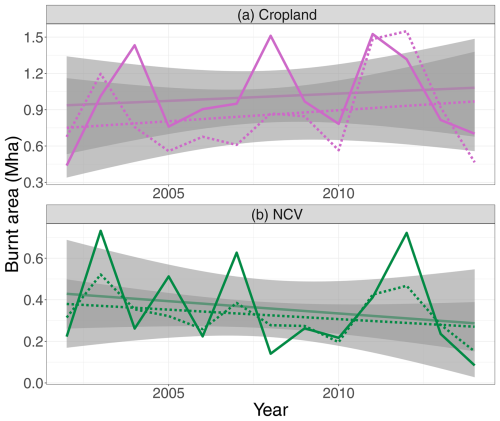
Figure 5Annual time series of cropland (a) and non-cropland vegetation (NCV; b) burning for BASE and ESA FireCCI51. The trend (calculated with linear regression) is plotted as a straight line with the 95 % confidence interval shown as grey shading.
The results from the fitted GLMs broadly conformed to our expectations of the drivers of fire occurrence in both land cover types. The models demonstrated reasonable explanatory power when viewed as statistical models, and when viewed as simulators of fire occurrence, they showed similar model skill to global more complex vegetation-fire models applied on a global scope in terms of NME scores (Hantson et al., 2020). The models were constructed using predictors which either are easily calculable in most DGVMs or can be taken as prescribed input layers (including pre-existing future projections where appropriate). We suggest that these models could be integrated immediately into DGVMs for application on the European scale, particularly as we are not currently aware of any such models developed specifically for Europe.
Splitting the burnt area by LCT proved to be particularly illuminating. Whilst there was considerable overlap of the drivers of cropland and NCV burning, there were also some marked differences in terms of the direction of the responses and functional forms that provided the best fit. Furthermore, cropland burning modelling is comparatively underdeveloped; we are only aware of two global fire-enabled DGVMs that do so (Burton et al., 2019; Li et al., 2013) and one that prescribes it (Rabin et al., 2018), although the reader is advised to see also recent developments from Perkins et al. (2024). Recent studies have indicated that there is a larger amount of cropland burning than previously estimated (Chen et al., 2023; Hall et al., 2024). Indeed, in this study, cropland burning comprised 74 % of the total burnt area, which is much higher than our initial expectation. Although these may not strongly impact the global carbon cycle, the trace gas emissions associated with this burning have significant implications for regional air quality and atmospheric chemistry and also have ecological implications for cropland soils. The results and methods here can be tested and extended to simulate cropland burning on a global scale.
4.1 Contrasting drivers of NCV and cropland fire occurrence
Cropland areas are fairly evenly distributed across Europe (Fig. S1), with the exception of north Scandinavia and the west coast of British Isles, where no fires occur. We have demonstrated that within this broad European climate niche (Mediterranean, temperate, and boreal), croplands burn with very different temporal and spatial patterns compared to NCV. Consistent with this observation and our expectations, we also found the drivers of each to differ in some respects (variables associated with fire danger and spread, population density, and vegetation properties) while retaining some broad similarity in others (MEPI and socioeconomic development).
4.1.1 Fire weather and spread rate drivers
While NCV burning increased with FWI as would be expected, cropland burning showed a unimodal peak at intermediate FWI values. This makes sense as farmers would likely not burn fields during the most intensive period of fire weather due to the risk of losing control of the fire or contravening fire bans.
Similarly, wind was not found to be a useful predictor in BASE NCV (likely because of the monthly resolution and because local wind speeds are highly modified by terrain and vegetation cover) but was a negative predictor for BASE Cropland. Topographic slope was a positive driver in BASE NCV but a negative one for BASE Cropland. Again, this can be understood as farmers not burning fields in circumstances which will encourage fast or unpredictable fire spread – i.e. during windy periods and on steep terrain.
These results imply that current mechanistic modelling approaches are likely not well suited to modelling cropland fires. Mechanistic models are typically based on biophysical relationships concerning flammability and rate of spread and are run with the general assumption that higher flammability or faster rates of spread produce more burnt area. Our findings imply that this approach is not valid for cropland burning as more flammable conditions do not necessarily imply more burning in the croplands. Given this and the complexities of human land management and other socioeconomic factors, the inclusions of statistical or agent-based (Perkins et al., 2024) approaches in future cropland modelling efforts may prove fruitful.
4.1.2 Population density
We also saw opposing effects of population density on burnt area in each LCT. For NCV, our results show that more people implies more fires, which is consistent with the logic that more people and infrastructure cause more fire starts (Haas et al., 2022). But for croplands, we found that more people implies fewer fires, perhaps because burning near population centres is forbidden, because burning is forbidden generally and only enforced near population centres, or because burning is unpopular with local residents.
4.1.3 Vegetation properties
We also found the vegetation-related drivers of fire were different between the different land cover types. FAPAR during the preceding 12 months, a proxy for fuel buildup (particularly fine fuels), has a positive effect in BASE NCV, which is in line with a previous study (Kuhn-Régnier et al., 2021). The better performance of FAPAR12 than GPP12 can be explained by FAPAR's specific relationship to leaf biomass rather than GPP's relationship to general biomass production and the importance of fine fuel (i.e. leaves) for enabling fire ignition and spread. However, in BASE Cropland, the best similar predictor was the GPP of the last 12 months with a quadratic form, which gave a strong response at intermediate values. The low burning at low values of GPP can be easily explained by having insufficient biomass to burn. The low burning at high GPP is harder to explain, but we suggest this may be because higher GPP areas have more intense farming practices which use less fire or do not have an appropriate burn window due to insufficient precipitation seasonality.
For NCV burning, we found that intermediate levels of tree cover had the strongest positive effects on fire activity, although the exact mechanisms behind this correlation are hard to attribute. We suggest that this might occur because semi-forested ecosystems are, on the one hand, productive enough to produce sufficient quantities of fuel and, on the other hand, open enough that a lot of this fuel will be surface fine fuels – grasses and shrubs – which strongly support fire spread. This openness also implies a drier and windier microclimate, which also encourages fire spread. Further work is required to disentangle these mechanisms, and we further note that some caution is required here as it cannot be excluded that the reduced tree cover in such areas is a consequence of fire occurrence rather than a cause of it.
4.2 Simulation of the seasonal cycle and the monthly GPP-derived indices
From very few monthly predictors BASE produces an acceptable representation of the seasonal fire cycle. In particular, BASE NCV predicts seasonal fire patterns very well using only two monthly predictors (MEPI and FWI) and their interaction (technically, FAPAR12 data are monthly, but their effect is heavily damped because it is a 12-month rolling mean). We suggest this offers a simple approach for separately quantifying two distinct but easily conflated factors when considering fire danger: (1) the meteorologically determined fire weather risk, here captured by FWI, and (2) the moisture and phenological status of the vegetation, captured here using MEPI. The good performance indicates that using FWI offers a simpler alternative to using multiple climate variables as has been used in other studies. These climate variables are often highly correlated, which may risk overfitting and cause difficulties for causal attribution. Similarly, MEPI – based only on GPP – provides a means of using current vegetation functioning to determine the vegetation state and therefore flammability in a single predictor variable.
The seasonal cycle produced by BASE Cropland does not match the observations as well those produced by BASE NCV despite BASE Cropland using a larger number of monthly predictor variables. The factors determining the timing of cropland burning are less clear than for NCV burning as its timing is controlled by human agency rather than biophysical factors. Flammability must still play a role in cropland burning, and our results indicate that preferred residue burning conditions are intermediate fire weather and low wind speeds. Beyond this, it is a matter of agricultural practice and crop rotations, which are not taken into account here but could be explored in future work with an expanded version of BASE.
4.2.1 Applicability of MEPI and PHI
The monthly ecosystem productivity index (MEPI) was constructed for this study as a robust way to quantify months when an ecosystem is in a more flammable state – which we equate to when it is not photosynthesising because it is either phenologically dormant or under drought stress. We also note that over croplands, relatively low GPP may also occur after harvest if photosynthesising biomass has been removed or at the end of the summer, and it is at these times when residue clearing fires may occur. This approach worked well, and MEPI gave the expected response and was the most important and second-most-important variable in BASE Cropland and BASE NCV, respectively. However, MEPI also includes periods when photosynthesis is low due to other factors which does not necessarily imply high flammability, in particular because of low temperatures. This will always be mitigated to some extent because low temperatures imply low fire risk, but it may be possible to improve the index to better handle periods where low temperatures reduce photosynthesis.
We designed the post-harvest index (PHI) to identify preceding 3-month periods with high GPP as an indication that crops could have been grown to the point of harvest and therefore when burning to clear residues may occur. This was to keep maximum model flexibility by avoiding the use of data-specific harvest dates, which might not be available. PHI was a high-importance predictor which improved the overall deviance explained and the spatial and interannual patterns, although it decreased the model skill with respect to seasonal patterns. This may be because the indicator was designed to capture fire immediately after summer harvest but may not predict spring crop burning.
Overall, our results suggest that defining indicators based on monthly GPP is a promising approach. We chose GPP over greenness measures such as the normalised difference vegetation index (NDVI) because it can capture the ecosystem response to hot dry conditions (i.e. reduced photosynthesis due to water stress) before changes in greenness occur. Furthermore, GPP is a standard variable in DGVMs with recent advances in both observing it using solar-induced chlorophyll fluorescence (Mohammed et al., 2019) and simulating it using eco-evolutionary optimality methods (EEO; Stocker et al., 2020; Wang et al., 2017a). Thus, GPP and derived indicators could provide a relatively robust contact point for coupling DGVMs and fire models.
4.3 Strengths and weaknesses of BASE
BASE NCV did a good job of reproducing the timing of spatially aggregated seasonal and interannual fire observations, but it underestimated both the seasonal and the long-term peak burnt area amplitudes. It was less skilful in reproducing the observed spatial patterning of fire activity, which, although broadly correct, was too diffuse. The observed fire hotspots of central Portugal and the Adriatic coasts of Croatia, Montenegro, and Albania were not reproduced, and, in general, the model failed to pick out local regions of the burnt area. To some extent, this may be because the data comprise discrete fire events which include a strong stochastic aspect that is inherently difficult for a statistical model (which predicts mean values) to reproduce. However, even allowing for this, the details of the fire patterns in much of fire-prone southern Europe were not well captured. This might indicate an over-reliance on fire weather as a driver along with a failure to include local factors that may lead to high danger such as particularly flammable vegetation types or high ignition risks due to human activities, land cover interface zones, and infrastructure (Rodrigues et al., 2014).
These findings suggest that BASE NCV successfully captures broad fire drivers in terms of meteorological danger, coarse vegetation properties, and socioeconomic indicators and is suitable for projecting future fire occurrence across Europe. Although the simulated spatial distribution of fire is imperfect, future work may improve this by including more detailed datasets concerning infrastructure, socioeconomic indicators, and vegetation types. However, such datasets might not be available for future scenarios and so including them would inhibit the use of the model for future projections, and so it was not done here.
In contrast to the BASE NCV, BASE Cropland's representation of the spatial distribution of fire occurrence is comparatively better than its temporal distribution. It picks out all the hotspots of cropland burning, although it does overpredict in some other regions. However, its simulation of the summer burning peak is too narrow and does not resolve the October shoulder, while the interannual variability is poorly reproduced. It does capture a weakly increasing interannual trend, but this trend may be spurious as over a longer period cropland burning actually shows a decreasing trend (Fig. 1c). From this we can conclude we have captured the broad dependency of European cropland fires on socioeconomic development and suitable burning weather but have not captured some specific factors affecting the likelihood or timing of burning, such as sowing and harvest dates, crop types and systems (including double-cropping systems), or legislative pressures, which would require significantly more data input than is available at present in a spatially gridded format.
4.3.1 Spain: an outlier demonstrating the importance of regional effects
In contrast to other southern European countries, Spain stands out for its low observed wildfire fire incidence despite its fire-enabling Mediterranean characteristics. This is particularly clear in the observations when comparing as the observed NCV fires in Spain to neighbouring Portugal (Fig. 4). However, BASE NCV fails to simulate this change in fire occurrence on the national border. Furthermore, BASE Cropland also overestimates in Spain, predicting an extensive area of cropland burning when in fact there are only limited areas. This overestimation is larger than the low levels of overprediction seen in, for example, France and Poland, which is a consequence of the GLM tendency to predict a lot of low values (which is here overemphasised by the threshold in the colour scale). These substantial overestimates of both cropland and NCV fire occurrence may indicate phenomena specific to Spain which are not accounted for in BASE. We suggest that the answer may lie in changes made to its approach to wildfire risk at political and management levels during our study period. The period from 2003 to 2014 saw a decreasing trend in forest fires (Jiménez-Ruano et al., 2017; Vilar et al., 2015) due to the development, implementation, and efficacy of wildfire suppression practices after the devolution of responsibility for them to regional authorities (Galiana et al., 2013; Pastor et al., 2020). This was associated with a decrease in the number of fire incidents and burnt area due to improved fire control, potentially pushing fire sizes below the level which is detectable in the FireCCI51 product. The Spanish example highlights the importance of governance factors which cannot easily be quantified by broad indicators such as HDI or GDP as well as the challenges associated with simulating fire regimes across multiple governmental or organisational jurisdictions. The inclusion of regional datasets and random effect terms (based on, for example, administrative areas or legislative changes) may improve model skill, increase understanding, and be useful for short-term forecasting. However, these techniques will be difficult to apply on larger spatial scales and in longer range projections, so accounting for such effects remains an open challenge for fire modelling on continental to global scales.
4.4 HDI and GDP as predictors of fire occurrence
Whereas GDP represents economic development, HDI reflects broad trends in human wellbeing across health, education, and economic development. As such, they have correlative rather than causative relationships with burnt area as neither explicitly captures the effectiveness of human fire management nor the tendency to utilise fire as a land management tool and may be collinear with urbanisation and other infrastructural developments that may fragment landscapes and lead to declining burnt area (Haas et al., 2022). Nevertheless, increasing societal wellbeing is likely reflective of increased state capacity for fire management and public awareness as well as the enforcement of environmentally focussed policies (Bhuvaneshwari et al., 2019; Zhang et al., 2020), and one could conjecture that HDI, as the broader indicator, will be a better proxy for such developments. Our results support this supposition but only for NCV burning. We found HDI to be an important predictor for NCV burning; it was superior to GDP and its inclusion was important to capture the declining trend in NCV burning.
The picture is less clear for cropland burning. Both HDI and GDP improved the deviance explained and spatial patterns of cropland burning. We chose GDP over HDI for BASE Cropland because HDI introduced a declining trend in the cropland during predictions, which is not seen in the data and correspondingly worsened the temporal NME. GDP did not introduce this trend and had less of a negative impact on the reproduction of IAV. GDP may be a better predictor because it more directly reflects capitalisation and investment, which in turn directly affects agricultural practices and hence cropland burning.
However, we do not consider the result that GDP is a better predictor than HDI for cropland burning to be fully robust for a number of reasons. Firstly, due to the short record length and high IAV, the increasing trend in observed cropland burning (which appears to indicate that GDP is the better predictor) may be spurious. Indeed, a decreasing trend is seen over a longer period (Fig. 1c). Secondly, it is not clear what actually drives the IAV of cropland burning and a priori one would not expect such large variability, particularly as small fires generally have a lower IAV than larger ones (Randerson et al., 2012). Year-to-year variability in appropriate burning conditions will likely play a role, and BASE Cropland clearly indicates that burning conditions are important. There are likely further influences on cropland burning IAV not captured here, possibly related to legislative factors, such as Bulgaria's and Romania's accession to the EU in 2007 (although we note that the decline in cropland burning commenced after 2007). However, one would assume these would affect the trend rather than the IAV in cropland burning. Thirdly, it is possible that changes in land use, specifically the abandonment of croplands, are not immediately captured in the land cover data, leading to the erroneous allocation of fires as cropland when they are in fact NCV. Such abandoned areas are considered to have high fire danger (Moreira et al., 2001; San-Miguel-Ayanz et al., 2012), which exacerbates the misclassification of land cover, and so wildfires in these areas may contaminate the cropland burning signal, with implications for IAV. Finally, inclusion of either GDP or HDI worsens the IAV NME (but GDP less so) whilst improving other metrics. Because these indicators represent broad socioeconomic changes through the years, we expected them to improve the temporal reproduction of observations.
There are other issues that might arise with using GDP/HDI. We note that there is greater variation in HDI and GDP in space than in time, and it is likely that the spatial variation dominates the fitted model response. However, introducing HDI does allow the model to capture the declining trend in NCV burning (Fig. S13), so the temporal response seems to be reasonable. Another potential issue is that annual GDP is sensitive to short-term financial crises or other abrupt changes. Such a drop in GDP would have an immediate effect on the model, and this is likely not entirely realistic (although we are unaware of any studies attempting to quantify this). HDI is likely a better indicator in this regard as economic activity comprises only one-third of its value, and the other two factors (life expectancy and years in education) are not so immediately susceptible to short-term changes in economic circumstances.
4.5 Implications and outlook for simulation of crop residue burning
The drivers of human fire use and their relation to wider fire regimes remain poorly understood on large spatial scales (Ford et al., 2021; Shuman et al., 2022). In our study area, cropland burning accounts for nearly three-quarters of burnt area but has been comparatively understudied. For example, within the Database of Anthropogenic Fire Impacts – a global meta-analysis of academic literature on human–fire interactions – 43 % (n=300) of instances of human fire use in our study area document prescribed fire to tackle extreme wildfires, whilst another 35 % (n=245) of cases focus on diagnosing the human sources of unmanaged wildfires. By contrast, just 5 % (n=38) of instances of human fire use document crop residue burning (Millington et al., 2022).
This study contributes to filling this knowledge gap by demonstrating how socioeconomic and environmental processes have contrasting impacts on cropland and NCV burning. This finding is in broad alignment with local-scale empirical studies (Millington et al., 2022) and the few existing modelling studies of large spatial extents (Perkins et al., 2024). Most importantly, we found that the drivers of crop residue burning differ in key ways from NCV fire occurrence. This is not surprising as they are very different phenomena. In Europe, the majority of NCV fires can be characterised as undesirable blazes either set accidentally or burning out of control, for which high fire danger and fuel loads play a large role. In contrast, residue burning is a deliberate process where socioeconomic factors and the avoidance of burning at times of high fire danger are highly relevant. These facts are codified in our results, providing tangible evidence that cropland and NCV fires must be modelled separately. Many previous attempts to reproduce large-scale burnt area patterns have not explicitly taken this into account, including many global fire models in DGVMs. We suggest that in the future better results can be obtained by explicitly simulating cropland fires and accounting for their different drivers and dynamics or by simply removing burnt areas occurring in croplands from the target dataset if they are deemed not relevant.
This study elucidates some of the controls over cropland burning, but further research is needed, particularly that focussing on its temporal dynamics. For maximum flexibility and parsimony, BASE Cropland does not use information about harvest dates, crop types, or cropping systems, but including these could potentially provide new insights and improve the representation of the seasonal cycle, which is one of the weaker aspects of BASE Cropland. Introducing regionally specific factors to account for legislative changes, such as when countries joined the EU, in which residue burning is, in principle, forbidden, may also prove helpful, in particular for understanding the temporal evolution of cropland burning. It may also help resolve questions about the use of the broader socioeconomic predictors, such as HDI and GDP.
4.6 Limitations and caveats
4.6.1 GLM approach
Overall, our approach of fitting GLMs to monthly data worked well and therefore demonstrates that this method can give serviceable fire occurrence estimates with a seasonal cycle suitable for integration into other models such as DGVMs. However, the approach does come with a few limitations. What it has in common with other GLM studies (e.g. Bistinas et al., 2014; Haas et al., 2022) is that our model tends to “smear out” the burnt area by underestimating extremes and predicting many small values instead of zero. This may in part be due to the fact that, in reality, fire manifests in discrete events, whereas GLMs only predict mean values. This also explains the distinctive “many small underestimates and a few large overestimates” pattern in the partial residuals (Figs. S7 and S8). Another caveat is that due to spatial autocorrelation in the datasets, the standard errors are likely to be considerable underestimations and the uncertainty bands in Figs. 2, S7, and S8 should be viewed as lower bounds (although this does not affect the central parameter estimates). Finally, the necessary use of the quasi-binomial distribution to model burnt fraction precludes the use of standard statistical tools and diagnostics, such as Q–Q plots and information criteria. It may be possible to overcome some of these limitations in the future by moving away from burnt fraction as the target variable and focussing on other aspects of the fire regime, thus putting understanding of fire occurrence on a more rigorous statistical footing.
4.6.2 Uncertainties and errors in remote sensing data
This study relies heavily on remote sensing data, particularly the burnt area and land cover data used to construct the target variable. Remote sensing products based on MODIS (including the ESA FireCCI data used here) are known to struggle with detecting small fires and have high omission errors, particularly in the Mediterranean (Katagis and Gitas, 2021), although FireCCI51 does feature improved sensitivity to small fires (Lizundia-Loiola et al., 2020). This has implications for all fires, but cropland fires in particular are typically small, brief, and low-intensity and more often missed by remote sensing (Hall et al., 2021; Zhu et al., 2017). So while it accounts for the majority of the burning in the study region, the estimate of cropland burning used here is likely an underestimation, and analyses with newer remote sensing datasets may modify and ultimately improve on our results. Misclassifications of land cover will also affect our results – in particular, imperfect separation of croplands from other vegetation types due to rapid land use change (Winkler et al., 2021; Zubkova et al., 2023). Notably, abandoned croplands in their early stage of transition represent a major fire risk (Moreira et al., 2001; San-Miguel-Ayanz et al., 2012) and may cause commission error in the identification of cropland fires. However, the prevalence and predictability of this signal and the support from existing literature (Millington et al., 2022) give us confidence that the bulk of this signal is indeed cropland residue burning. In particular, one recent study based on higher-resolution remote sensing data in Romania (one of the cropland burning hotspots in Europe) confirmed many of the results here (Mattes et al., 2024) – namely, that the majority of burning in Romania is indeed in arable land, that these fires occur less often in areas with steep topography, and that their frequency is reducing due to socioeconomic factors.
This study aimed to disentangle the drivers of fire occurrence across a European study domain and encapsulate this knowledge into a new fire model (the BASE model). Our initial investigations of burnt area in Europe revealed that cropland and non-cropland vegetation (NCV) land cover types burn with very different spatiotemporal patterns. After fitting GLMs to each land cover type separately, we confirmed that there are very different drivers for fire occurrence in the land cover types. This was most clearly manifested in fire weather and other variables connected to rate of spread, where our results indicated that crop residue burning is preferentially conducted in situations of lower fire danger and rate of spread. This outcome has implications for any large-scale studies to simulate burnt area over a mixture of land cover types. Our results also provide some novel insights into the drivers of cropland burning which has, to our knowledge, not previously been systematically studied over Europe. In addition to optimal burning conditions, we found a strong control over spatial patterns by socioeconomic development and over seasonal timing by GPP-derived indices. However, the mechanisms controlling the seasonality and interannual patterns of cropland burning remain poorly understood and require further study. This is of particular importance because fire occurrence in cropland has recently been revealed to be more prevalent than previously estimated.
Overall, the BASE model reasonably captures the spatiotemporal patterns of burnt area in Europe. BASE NCV does particularly well with reproducing observed temporal dynamics and relatively less well with the spatial patterns, while the opposite is true for BASE Cropland. We suggest two potential future applications for BASE. As a simulator, BASE already provides a serviceable means to simulate fire occurrence in Europe that is compatible and easily integrated with other model frameworks, such as DGVMs. In particular, its skilful reproduction of the seasonal and interannual patterns of wildfires indicate that it captures the temporal dynamics and so is suitable for projecting changes in fire hazard over annual to decadal timescales, particularly when considering cropland and non-cropland land cover types. The explicit simulations of cropland fires are also a noteworthy advance. In addition to its use for projections, we suggest that the BASE framework may also be further utilised by considering additional potential predictor datasets to improve understanding of the controls on burnt area in Europe. For BASE Cropland, data about harvest dates and cropping systems and variables to capture changes in legislation may help understand the temporal dynamics. For BASE NCV, additional socioeconomic indicators and maps of vegetation types and infrastructure may explain the spatial patterns. Both of these applications of BASE may help in meeting the challenges of increasing fire risk faced by Europe. In addition, the scientific outcomes and methodology developed here can facilitate the development of similar models for other regions.
Code used in this analysis (including data preparation, model fitting, analysis, and plotting) is available at https://doi.org/10.5281/zenodo.14008936 (Forrest, 2024a).
The data used to fit the models are available on a Zenodo repository at https://doi.org/10.5281/zenodo.12580343 (Forrest, 2024b).
The supplement related to this article is available online at: https://doi.org/10.5194/bg-21-5539-2024-supplement.
MF conceptualised the model and performed the data analysis and model fitting with support from MB, SPKB, JH, TH, EK, LO, and KT. MB, SPKB, JH, EK, and LO processed and provided datasets. OP provided context and interpretation for human dimensions of fire use and socioeconomic indicators. DW assisted with the development of the statistical framework. FAF provided context and interpretation for the southern European results. MF prepared the manuscript with contributions from all co-authors.
At least one of the (co-)authors is a member of the editorial board of Biogeosciences. The peer-review process was guided by an independent editor, and the authors also have no other competing interests to declare.
Publisher's note: Copernicus Publications remains neutral with regard to jurisdictional claims made in the text, published maps, institutional affiliations, or any other geographical representation in this paper. While Copernicus Publications makes every effort to include appropriate place names, the final responsibility lies with the authors.
We thank Marcos Rodrigues for informative discussions.
This paper is part of the FirEUrisk project research funded by the European Union's Horizon 2020 research and innovation program under grant agreement no. 101003890. From FirEUrisk, Jessica Hetzer, Maik Billing, Simon P. K. Bowring, and Eric Kosczor received salary and Jessica Hetzer, Maik Billing, Simon P. K. Bowring, Eric Kosczor, Matthew Forrest, Luke Oberhagemann, Kirsten Thonicke, and Thomas Hickler received travel support. In addition, Fátima Arrogante-Funes was supported by a predoctoral scholarship (FPI) from the Spanish Ministry of Science, Innovation and Universities (grant no. PRE2019-089208). Oliver Perkins is funded by the Leverhulme Trust (grant no. RC-2018-023). Luke Oberhagemann is funded within the research training group Natural Hazards and Risks in a Changing World (NatRiskChange) funded by the Deutsche Forschungsgemeinschaft (DFG; grant no. GRK 2043/2).
This paper was edited by Ivonne Trebs and reviewed by two anonymous referees.
Amatulli, G., Camia, A., and San-Miguel-Ayanz, J.: Estimating future burned areas under changing climate in the EU-Mediterranean countries, Sci. Total Environ., 450–451, 209–222, https://doi.org/10.1016/j.scitotenv.2013.02.014, 2013.
Amatulli, G., McInerney, D., Sethi, T., Strobl, P., and Domisch, S.: Geomorpho90m, empirical evaluation and accuracy assessment of global high-resolution geomorphometric layers, Sci. Data, 7, 162, https://doi.org/10.1038/s41597-020-0479-6, 2020.
Andela, N., Morton, D. C., Giglio, L., Chen, Y., Werf, G. R. van der, Kasibhatla, P. S., DeFries, R. S., Collatz, G. J., Hantson, S., Kloster, S., Bachelet, D., Forrest, M., Lasslop, G., Li, F., Mangeon, S., Melton, J. R., Yue, C., and Randerson, J. T.: A human-driven decline in global burned area, Science, 356, 1356–1362, https://doi.org/10.1126/science.aal4108, 2017.
Archibald, S., Lehmann, C. E. R., Belcher, C. M., Bond, W. J., Bradstock, R. A., Daniau, A.-L., Dexter, K. G., Forrestel, E. J., M Greve, He, T., Higgins, S. I., Hoffmann, W. A., Lamont, B. B., McGlinn, D. J., Moncrieff, G. R., Osborne, C. P., Pausas, J. G., O Price, Ripley, B. S., Rogers, B. M., Schwilk, D. W., Simon, M. F., Turetsky, M. R., Van der Werf, G. R., and Zanne, A. E.: Biological and geophysical feedbacks with fire in the Earth system, Environ. Res. Lett., 13, 033003, https://doi.org/10.1088/1748-9326/aa9ead, 2018.
Arnell, N. W., Freeman, A., and Gazzard, R.: The effect of climate change on indicators of fire danger in the UK, Environ. Res. Lett., 16, 044027, https://doi.org/10.1088/1748-9326/abd9f2, 2021.
Arrogante-Funes, F., Aguado, I., and Chuvieco, E.: Global impacts of fire regimes on wildland bird diversity, Fire Ecol., 20, 1–17, https://doi.org/10.1186/s42408-024-00259-x, 2024.
Bhuvaneshwari, S., Hettiarachchi, H., and Meegoda, J. N.: Crop Residue Burning in India: Policy Challenges and Potential Solutions, Int. J. Env. Res. Pub. He., 16, 832, https://doi.org/10.3390/ijerph16050832, 2019.
Bistinas, I., Harrison, S. P., Prentice, I. C., and Pereira, J. M. C.: Causal relationships versus emergent patterns in the global controls of fire frequency, Biogeosciences, 11, 5087–5101, https://doi.org/10.5194/bg-11-5087-2014, 2014.
Boer, M. M., Resco de Dios, V., and Bradstock, R. A.: Unprecedented burn area of Australian mega forest fires, Nat. Clim. Change, 10, 171–172, https://doi.org/10.1038/s41558-020-0716-1, 2020.
Boulanger, Y., Parisien, M.-A., and Wang, X.: Model-specification uncertainty in future area burned by wildfires in Canada, Int. J. Wildland Fire, 27, 164–175, https://doi.org/10.1071/WF17123, 2018.
Bowman, D. M. J. S., Balch, J. K., Artaxo, P., Bond, W. J., Carlson, J. M., Cochrane, M. A., D'Antonio, C. M., DeFries, R. S., Doyle, J. C., Harrison, S. P., Johnston, F. H., Keeley, J. E., Krawchuk, M. A., Kull, C. A., Marston, J. B., Moritz, M. A., Prentice, I. C., Roos, C. I., Scott, A. C., Swetnam, T. W., van der Werf, G. R., and Pyne, S. J.: Fire in the Earth System, Science, 324, 481–484, https://doi.org/10.1126/science.1163886, 2009.
Bowman, D. M. J. S., Kolden, C. A., Abatzoglou, J. T., Johnston, F. H., van der Werf, G. R., and Flannigan, M.: Vegetation fires in the Anthropocene, Nat. Rev. Earth Environ., 1, 500–515, https://doi.org/10.1038/s43017-020-0085-3, 2020.
Breheny, P. and Burchett, W.: Visualization of Regression Models Using visreg, R J., 9, 56–71, 2017.
Buchhorn, M., Smets, B., Bertels, L., De Roo, B., Lesiv, M., Tsendbazar, N. E., Linlin, L., and Tarko, A.: Copernicus Global Land Service: Land Cover 100m: Version 3 Globe 2015–2019: Product User Manual, Geneve, Switzerland, September 2020, Zenodo, https://doi.org/10.5281/zenodo.3938963, 2020.
Burton, C., Betts, R., Cardoso, M., Feldpausch, T. R., Harper, A., Jones, C. D., Kelley, D. I., Robertson, E., and Wiltshire, A.: Representation of fire, land-use change and vegetation dynamics in the Joint UK Land Environment Simulator vn4.9 (JULES), Geosci. Model Dev., 12, 179–193, https://doi.org/10.5194/gmd-12-179-2019, 2019.
Carvalho, A., Flannigan, M. D., Logan, K., Miranda, A. I., and Borrego, C.: Fire activity in Portugal and its relationship to weather and the Canadian Fire Weather Index System, Int. J. Wildland Fire, 17, 328–338, https://doi.org/10.1071/WF07014, 2008.
Chen, Y., Hall, J., van Wees, D., Andela, N., Hantson, S., Giglio, L., van der Werf, G. R., Morton, D. C., and Randerson, J. T.: Multi-decadal trends and variability in burned area from the fifth version of the Global Fire Emissions Database (GFED5), Earth Syst. Sci. Data, 15, 5227–5259, https://doi.org/10.5194/essd-15-5227-2023, 2023.
Chuvieco, E., Pettinari, M. L., Koutsias, N., Forkel, M., Hantson, S., and Turco, M.: Human and climate drivers of global biomass burning variability, Sci. Total Environ., 779, 146361, https://doi.org/10.1016/j.scitotenv.2021.146361, 2021.
Chuvieco, E., Yebra, M., Martino, S., Thonicke, K., Gómez-Giménez, M., San-Miguel, J., Oom, D., Velea, R., Mouillot, F., Molina, J. R., Miranda, A. I., Lopes, D., Salis, M., Bugaric, M., Sofiev, M., Kadantsev, E., Gitas, I. Z., Stavrakoudis, D., Eftychidis, G., Bar-Massada, A., Neidermeier, A., Pampanoni, V., Pettinari, M. L., Arrogante-Funes, F., Ochoa, C., Moreira, B., and Viegas, D.: Towards an Integrated Approach to Wildfire Risk Assessment: When, Where, What and How May the Landscapes Burn, Fire, 6, 215, https://doi.org/10.3390/fire6050215, 2023.
Cunningham, C. X., Williamson, G. J., and Bowman, D. M. J. S.: Increasing frequency and intensity of the most extreme wildfires on Earth, Nat. Ecol. Evol., 8, 1420–1425, https://doi.org/10.1038/s41559-024-02452-2, 2024.
Dijkstra, J., Durrant, T., San-Miguel-Ayanz, J., and Veraverbeke, S.: Anthropogenic and Lightning Fire Incidence and Burned Area in Europe, Land, 11, 651, https://doi.org/10.3390/land11050651, 2022.
Dury, M., Hambuckers, A., Warnant, P., Henrot, A., Favre, E., Ouberdous, M., and François, L.: Responses of European forest ecosystems to 21(st) century climate: assessing changes in interannual variability and fire intensity, IForest Biogeosciences For., 4, 82–99, https://doi.org/10.3832/ifor0572-004, 2011.
El Garroussi, S., Di Giuseppe, F., Barnard, C., and Wetterhall, F.: Europe faces up to tenfold increase in extreme fires in a warming climate, Npj Clim. Atmospheric Sci., 7, 1–11, https://doi.org/10.1038/s41612-024-00575-8, 2024.
ESA: Land Cover CCI Product User Guide Version 2. Tech. Rep., http://maps.elie.ucl.ac.be/CCI/viewer/download/ESACCI-LC-Ph2-PUGv2_2.0.pdf, (last access: July 2023), 2017.
European Commission Directorate-General Joint Research Centre: Fraction of Absorbed Photosynthetically Active Radiation 1999–2020 (raster 1 km), global, 10-daily – version 2, https://globalland.vito.be/geonetwork/srv/api/records/clms_global_fapar_1km_v2_10daily (last access: 6 December 2024), 2020.
Ford, A. E. S., Harrison, S. P., Kountouris, Y., Millington, J. D. A., Mistry, J., Perkins, O., Rabin, S. S., Rein, G., Schreckenberg, K., Smith, C., Smith, T. E. L., and Yadav, K.: Modelling Human-Fire Interactions: Combining Alternative Perspectives and Approaches, Front. Environ. Sci., 9, 418, https://doi.org/10.3389/fenvs.2021.649835, 2021.
Forkel, M., Dorigo, W., Lasslop, G., Teubner, I., Chuvieco, E., and Thonicke, K.: A data-driven approach to identify controls on global fire activity from satellite and climate observations (SOFIA V1), Geosci. Model Dev., 10, 4443–4476, https://doi.org/10.5194/gmd-10-4443-2017, 2017.
Galiana, L., Aguilar, S., and Lázaro, A.: An assessment of the effects of forest-related policies upon wildland fires in the European Union: Applying the subsidiarity principle, Forest Policy Econ., 29, 36–44, https://doi.org/10.1016/j.forpol.2012.10.010, 2013.
Gorelick, N., Hancher, M., Dixon, M., Ilyushchenko, S., Thau, D., and Moore, R.: Google Earth Engine: Planetary-scale geospatial analysis for everyone, Remote Sens. Environ., 202, 18–27, https://doi.org/10.1016/j.rse.2017.06.031, 2017.
Forrest, M.: BASE-v1.0.2 Typeset version, Zenodo [code], https://doi.org/10.5281/zenodo.14008936, 2024a.
Forrest, M.: Data for fitting Burnt Area Simulator for Europe (BASE v1.0) (1.0), Zenodo [data set], https://doi.org/10.5281/zenodo.12580343, 2024b.
Galizia, L. F., Curt, T., Barbero, R., Rodrigues, M., Galizia, L. F., Curt, T., Barbero, R., and Rodrigues, M.: Understanding fire regimes in Europe, Int. J. Wildland Fire, 31, 56–66, https://doi.org/10.1071/WF21081, 2021.
Giannaros, T. M., Papavasileiou, G., Lagouvardos, K., Kotroni, V., Dafis, S., Karagiannidis, A., and Dragozi, E.: Meteorological Analysis of the 2021 Extreme Wildfires in Greece: Lessons Learned and Implications for Early Warning of the Potential for Pyroconvection, Atmosphere, 13, 475, https://doi.org/10.3390/atmos13030475, 2022.
Greenwell, B. M. and Boehmke, B. C.: Variable Importance Plots–An Introduction to the vip Package, R J., 12, 343–366, 2020.
Haas, O., Prentice, I. C., and Harrison, S. P.: Global environmental controls on wildfire burnt area, size, and intensity, Environ. Res. Lett., 17, 065004, https://doi.org/10.1088/1748-9326/ac6a69, 2022.
Hall, J. V., Zibtsev, S. V., Giglio, L., Skakun, S., Myroniuk, V., Zhuravel, O., Goldammer, J. G., and Kussul, N.: Environmental and political implications of underestimated cropland burning in Ukraine, Environ. Res. Lett., 16, 064019, https://doi.org/10.1088/1748-9326/abfc04, 2021.
Hall, J. V., Argueta, F., Zubkova, M., Chen, Y., Randerson, J. T., and Giglio, L.: GloCAB: global cropland burned area from mid-2002 to 2020, Earth Syst. Sci. Data, 16, 867–885, https://doi.org/10.5194/essd-16-867-2024, 2024.
Hantson, S., Arneth, A., Harrison, S. P., Kelley, D. I., Prentice, I. C., Rabin, S. S., Archibald, S., Mouillot, F., Arnold, S. R., Artaxo, P., Bachelet, D., Ciais, P., Forrest, M., Friedlingstein, P., Hickler, T., Kaplan, J. O., Kloster, S., Knorr, W., Lasslop, G., Li, F., Mangeon, S., Melton, J. R., Meyn, A., Sitch, S., Spessa, A., van der Werf, G. R., Voulgarakis, A., and Yue, C.: The status and challenge of global fire modelling, Biogeosciences, 13, 3359–3375, https://doi.org/10.5194/bg-13-3359-2016, 2016.
Hantson, S., Kelley, D. I., Arneth, A., Harrison, S. P., Archibald, S., Bachelet, D., Forrest, M., Hickler, T., Lasslop, G., Li, F., Mangeon, S., Melton, J. R., Nieradzik, L., Rabin, S. S., Prentice, I. C., Sheehan, T., Sitch, S., Teckentrup, L., Voulgarakis, A., and Yue, C.: Quantitative assessment of fire and vegetation properties in simulations with fire-enabled vegetation models from the Fire Model Intercomparison Project, Geosci. Model Dev., 13, 3299–3318, https://doi.org/10.5194/gmd-13-3299-2020, 2020.
Hetzer, J., Forrest, M., Ribalaygua, J., Prado-López, C., and Hickler, T.: The fire weather in Europe: large-scale trends towards higher danger, Environ. Res. Lett., 19, 084017, https://doi.org/10.1088/1748-9326/ad5b09, 2024.
Hijmans, R.: terra: Spatial Data Analysis, https://doi.org/10.32614/CRAN.package.terra, 2023.
Hu, Y., Yue, X., and Tian, C.: Climatic drivers of the Canadian wildfire episode in 2023, Atmospheric Ocean. Sci. Lett., 17, 100483, https://doi.org/10.1016/j.aosl.2024.100483, 2024.
Jiménez-Ruano, A., Mimbrero, M. R., and de la Riva Fernández, J.: Understanding wildfires in mainland Spain. A comprehensive analysis of fire regime features in a climate-human context, Appl. Geogr., 89, 100–111, https://doi.org/10.1016/j.apgeog.2017.10.007, 2017.
Johnston, F. H., Henderson, S. B., Chen, Y., Randerson, J. T., Marlier, M., DeFries, R. S., Kinney, P., Bowman, D. M. J. S., and Brauer, M.: Estimated Global Mortality Attributable to Smoke from Landscape Fires, Environ. Health Persp., 120, 695–701, https://doi.org/10.1289/ehp.1104422, 2012.
Jones, M. W., Abatzoglou, J. T., Veraverbeke, S., Andela, N., Lasslop, G., Forkel, M., Smith, A. J. P., Burton, C., Betts, R. A., van der Werf, G. R., Sitch, S., Canadell, J. G., Santín, C., Kolden, C., Doerr, S. H., and Le Quéré, C.: Global and Regional Trends and Drivers of Fire Under Climate Change, Rev. Geophys., 60, e2020RG000726, https://doi.org/10.1029/2020RG000726, 2022.
Katagis, T. and Gitas, I. Z.: Accuracy estimation of two global burned area products at national scale, IOP Conf. Ser. Earth Environ. Sci., 932, 012001, https://doi.org/10.1088/1755-1315/932/1/012001, 2021.
Keeping, T., Harrison, S. P., and Prentice, I. C.: Modelling the daily probability of wildfire occurrence in the contiguous United States, Environ. Res. Lett., 19, 024036, https://doi.org/10.1088/1748-9326/ad21b0, 2024.
Kelley, D. I., Prentice, I. C., Harrison, S. P., Wang, H., Simard, M., Fisher, J. B., and Willis, K. O.: A comprehensive benchmarking system for evaluating global vegetation models, Biogeosciences, 10, 3313–3340, https://doi.org/10.5194/bg-10-3313-2013, 2013.
Khabarov, N., Krasovskii, A., Obersteiner, M., Swart, R., Dosio, A., San-Miguel-Ayanz, J., Durrant, T., Camia, A., and Migliavacca, M.: Forest fires and adaptation options in Europe, Reg. Environ. Change, 16, 21–30, https://doi.org/10.1007/s10113-014-0621-0, 2016.
Klein Goldewijk, K., Beusen, A., Doelman, J., and Stehfest, E.: Anthropogenic land use estimates for the Holocene – HYDE 3.2, Earth Syst. Sci. Data, 9, 927–953, https://doi.org/10.5194/essd-9-927-2017, 2017.
Knorr, W., Jiang, L., and Arneth, A.: Climate, CO2 and human population impacts on global wildfire emissions, Biogeosciences, 13, 267–282, https://doi.org/10.5194/bg-13-267-2016, 2016.
Krüger, R., Blanch Gorriz, X., Grothum, O., and Eltner, A.: Using multi-scale and multi-model datasets for post-event assessment of wildfires , EGU General Assembly 2023, Vienna, Austria, 24–28 Apr 2023, EGU23-13008, https://doi.org/10.5194/egusphere-egu23-13008, 2023.
Kuhn-Régnier, A., Voulgarakis, A., Nowack, P., Forkel, M., Prentice, I. C., and Harrison, S. P.: The importance of antecedent vegetation and drought conditions as global drivers of burnt area, Biogeosciences, 18, 3861–3879, https://doi.org/10.5194/bg-18-3861-2021, 2021.
Kummu, M., Taka, M., and Guillaume, J. H. A.: Gridded global datasets for Gross Domestic Product and Human Development Index over 1990–2015, Sci. Data, 5, 180004, https://doi.org/10.1038/sdata.2018.4, 2018.
Li, F., Levis, S., and Ward, D. S.: Quantifying the role of fire in the Earth system – Part 1: Improved global fire modeling in the Community Earth System Model (CESM1), Biogeosciences, 10, 2293–2314, https://doi.org/10.5194/bg-10-2293-2013, 2013.
Li, X. and Xiao, J.: A Global, 0.05-Degree Product of Solar-Induced Chlorophyll Fluorescence Derived from OCO-2, MODIS, and Reanalysis Data, Remote Sens., 11, 517, https://doi.org/10.3390/rs11050517, 2019.
Lizundia-Loiola, J., Otón, G., Ramo, R., and Chuvieco, E.: A spatio-temporal active-fire clustering approach for global burned area mapping at 250 m from MODIS data, Remote Sens. Environ., 236, 111493, https://doi.org/10.1016/j.rse.2019.111493, 2020.
Mattes, T., Marzolff, I., and Feurdean, A.: Excessive fire occurrence in Romania from 2001 to 2022: Trends and drivers across ecoregions and land cover classes, EGU General Assembly 2024, Vienna, Austria, 14–19 Apr 2024, EGU24-6624, https://doi.org/10.5194/egusphere-egu24-6624, 2024.
McLauchlan, K. K., Higuera, P. E., Miesel, J., Rogers, B. M., Schweitzer, J., Shuman, J. K., Tepley, A. J., Varner, J. M., Veblen, T. T., Adalsteinsson, S. A., Balch, J. K., Baker, P., Batllori, E., Bigio, E., Brando, P., Cattau, M., Chipman, M. L., Coen, J., Crandall, R., Daniels, L., Enright, N., Gross, W. S., Harvey, B. J., Hatten, J. A., Hermann, S., Hewitt, R. E., Kobziar, L. N., Landesmann, J. B., Loranty, M. M., Maezumi, S. Y., Mearns, L., Moritz, M., Myers, J. A., Pausas, J. G., Pellegrini, A. F. A., Platt, W. J., Roozeboom, J., Safford, H., Santos, F., Scheller, R. M., Sherriff, R. L., Smith, K. G., Smith, M. D., and Watts, A. C.: Fire as a fundamental ecological process: Research advances and frontiers, J. Ecol., 108, 2047–2069, https://doi.org/10.1111/1365-2745.13403, 2020.
Migliavacca, M., Dosio, A., Camia, A., Hobourg, R., Houston-Durrant, T., Kaiser, J. W., Khabarov, N., Krasovskii, A. A., Marcolla, B., San Miguel-Ayanz, J., Ward, D. S., and Cescatti, A.: Modeling biomass burning and related carbon emissions during the 21st century in Europe, J. Geophys. Res.-Biogeo., 118, 1732–1747, https://doi.org/10.1002/2013JG002444, 2013.
Millington, J. D. A., Perkins, O., and Smith, C.: Human Fire Use and Management: A Global Database of Anthropogenic Fire Impacts for Modelling, Fire, 5, 87, https://doi.org/10.3390/fire5040087, 2022.
Mohammed, G. H., Colombo, R., Middleton, E. M., Rascher, U., van der Tol, C., Nedbal, L., Goulas, Y., Pérez-Priego, O., Damm, A., Meroni, M., Joiner, J., Cogliati, S., Verhoef, W., Malenovský, Z., Gastellu-Etchegorry, J.-P., Miller, J. R., Guanter, L., Moreno, J., Moya, I., Berry, J. A., Frankenberg, C., and Zarco-Tejada, P. J.: Remote sensing of solar-induced chlorophyll fluorescence (SIF) in vegetation: 50 years of progress, Remote Sens. Environ., 231, 111177, https://doi.org/10.1016/j.rse.2019.04.030, 2019.
Moreira, F., Rego, F. C., and Ferreira, P. G.: Temporal (1958–1995) pattern of change in a cultural landscape of northwestern Portugal: implications for fire occurrence, Landsc. Ecol., 16, 557–567, https://doi.org/10.1023/A:1013130528470, 2001.
Mukunga, T., Forkel, M., Forrest, M., Zotta, R.-M., Pande, N., Schlaffer, S., and Dorigo, W.: Effect of Socioeconomic Variables in Predicting Global Fire Ignition Occurrence, Fire, 6, 197, https://doi.org/10.3390/fire6050197, 2023.
Muñoz-Sabater, J., Dutra, E., Agustí-Panareda, A., Albergel, C., Arduini, G., Balsamo, G., Boussetta, S., Choulga, M., Harrigan, S., Hersbach, H., Martens, B., Miralles, D. G., Piles, M., Rodríguez-Fernández, N. J., Zsoter, E., Buontempo, C., and Thépaut, J.-N.: ERA5-Land: a state-of-the-art global reanalysis dataset for land applications, Earth Syst. Sci. Data, 13, 4349–4383, https://doi.org/10.5194/essd-13-4349-2021, 2021.
Ochoa, C., Bar-Massada, A., and Chuvieco, E.: A European-scale analysis reveals the complex roles of anthropogenic and climatic factors in driving the initiation of large wildfires, Sci. Total Environ., 917, 170443, https://doi.org/10.1016/j.scitotenv.2024.170443, 2024.
Pastor, E., Muñoz, J. A., Caballero, D., Àgueda, A., Dalmau, F., and Planas, E.: Wildland–Urban Interface Fires in Spain: Summary of the Policy Framework and Recommendations for Improvement, Fire Technol., 56, 1831–1851, https://doi.org/10.1007/s10694-019-00883-z, 2020.
Perkins, O., Kasoar, M., Voulgarakis, A., Smith, C., Mistry, J., and Millington, J. D. A.: A global behavioural model of human fire use and management: WHAM! v1.0, Geosci. Model Dev., 17, 3993–4016, https://doi.org/10.5194/gmd-17-3993-2024, 2024.
R Core Team: R: A Language and Environment for Statistical Computing, 2024.
Rabin, S. S., Melton, J. R., Lasslop, G., Bachelet, D., Forrest, M., Hantson, S., Kaplan, J. O., Li, F., Mangeon, S., Ward, D. S., Yue, C., Arora, V. K., Hickler, T., Kloster, S., Knorr, W., Nieradzik, L., Spessa, A., Folberth, G. A., Sheehan, T., Voulgarakis, A., Kelley, D. I., Prentice, I. C., Sitch, S., Harrison, S., and Arneth, A.: The Fire Modeling Intercomparison Project (FireMIP), phase 1: experimental and analytical protocols with detailed model descriptions, Geosci. Model Dev., 10, 1175–1197, https://doi.org/10.5194/gmd-10-1175-2017, 2017.
Rabin, S. S., Ward, D. S., Malyshev, S. L., Magi, B. I., Shevliakova, E., and Pacala, S. W.: A fire model with distinct crop, pasture, and non-agricultural burning: use of new data and a model-fitting algorithm for FINAL.1, Geosci. Model Dev., 11, 815–842, https://doi.org/10.5194/gmd-11-815-2018, 2018.
Randerson, J. T., Chen, Y., van der Werf, G. R., Rogers, B. M., and Morton, D. C.: Global burned area and biomass burning emissions from small fires, J. Geophys. Res.-Biogeo., 117, G04012, https://doi.org/10.1029/2012JG002128, 2012.
Rodrigues, M., de la Riva, J., and Fotheringham, S.: Modeling the spatial variation of the explanatory factors of human-caused wildfires in Spain using geographically weighted logistic regression, Appl. Geogr., 48, 52–63, https://doi.org/10.1016/j.apgeog.2014.01.011, 2014.
Rodrigues, M., Cunill Camprubí, À., Balaguer-Romano, R., Coco Megía, C. J., Castañares, F., Ruffault, J., Fernandes, P. M., and Resco de Dios, V.: Drivers and implications of the extreme 2022 wildfire season in Southwest Europe, Sci. Total Environ., 859, 160320, https://doi.org/10.1016/j.scitotenv.2022.160320, 2023.
Roteta, E., Bastarrika, A., Padilla, M., Storm, T., and Chuvieco, E.: Development of a Sentinel-2 burned area algorithm: Generation of a small fire database for sub-Saharan Africa, Remote Sens. Environ., 222, 1–17, https://doi.org/10.1016/j.rse.2018.12.011, 2019.
Rothermel, R. C.: A Mathematical Model for Predicting Fire Spread in Wildland Fuels, Intermountain Forest and Range Experiment Station, Forest Service, US Dept. of Agriculture, Ogden, Utah USDA Forest Service General Technical Report INT-115, 1972.
Rouault, E., Warmerdam, F., Schwehr, K., Kiselev, A., Butler, H., Łoskot, M., Szekeres, T., Tourigny, E., Landa, M., Miara, I., Elliston, B., Chaitanya, K., Plesea, L., Morissette, D., Jolma, A., Dawson, N., Baston, D., de Stigter, C., and Miura, H.: GDAL (v3.10.0), Zenodo, https://doi.org/10.5281/zenodo.14046734, 2024.
San-Miguel-Ayanz, J., Rodrigues, M., de Oliveira, S. S., Pacheco, C. K., Moreira, F., Duguy, B., and Camia, A.: Land Cover Change and Fire Regime in the European Mediterranean Region, in: Post-Fire Management and Restoration of Southern European Forests, edited by: Moreira, F., Arianoutsou, M., Corona, P., and De las Heras, J., Springer Netherlands, Dordrecht, 21–43, https://doi.org/10.1007/978-94-007-2208-8_2, 2012.
San-Miguel-Ayanz, J., Durrant, T., Boca, R., Maianti, P., Liberta, G., Jacome Felix Oom, D., Branco, A., De Rigo, D., Suarez-Moreno, M., Ferrari, D., Roglia, E., Scionti, N., Broglia, M., Onida, M., Tistan, A., and Loffler, P.: Forest Fires in Europe, Middle East and North Africa 2022, Publications Office of the European Union, Luxembourg, JRC135226, https://doi.org/10.2760/348120, 2023.
Schulzweida, U.: CDO User Guide (2.3.0), Zenodo, https://doi.org/10.5281/zenodo.10020800, 2023.
Sexton, J. O., Song, X.-P., Feng, M., Noojipady, P., Anand, A., Huang, C., Kim, D.-H., Collins, K. M., Channan, S., DiMiceli, C., and Townshend, J. R.: Global, 30-m resolution continuous fields of tree cover: Landsat-based rescaling of MODIS vegetation continuous fields with lidar-based estimates of error, Int. J. Digit. Earth, 6, 427–448, https://doi.org/10.1080/17538947.2013.786146, 2013.
Sharples, J. J.: An overview of mountain meteorological effects relevant to fire behaviour and bushfire risk, Int. J. Wildland Fire, 18, 737–754, https://doi.org/10.1071/WF08041, 2009.
Shuman, J. K., Balch, J. K., Barnes, R. T., Higuera, P. E., Roos, C. I., Schwilk, D. W., Stavros, E. N., Banerjee, T., Bela, M. M., Bendix, J., Bertolino, S., Bililign, S., Bladon, K. D., Brando, P., Breidenthal, R. E., Buma, B., Calhoun, D., Carvalho, L. M. V., Cattau, M. E., Cawley, K. M., Chandra, S., Chipman, M. L., Cobian-Iñiguez, J., Conlisk, E., Coop, J. D., Cullen, A., Davis, K. T., Dayalu, A., De Sales, F., Dolman, M., Ellsworth, L. M., Franklin, S., Guiterman, C. H., Hamilton, M., Hanan, E. J., Hansen, W. D., Hantson, S., Harvey, B. J., Holz, A., Huang, T., Hurteau, M. D., Ilangakoon, N. T., Jennings, M., Jones, C., Klimaszewski-Patterson, A., Kobziar, L. N., Kominoski, J., Kosovic, B., Krawchuk, M. A., Laris, P., Leonard, J., Loria-Salazar, S. M., Lucash, M., Mahmoud, H., Margolis, E., Maxwell, T., McCarty, J. L., McWethy, D. B., Meyer, R. S., Miesel, J. R., Moser, W. K., Nagy, R. C., Niyogi, D., Palmer, H. M., Pellegrini, A., Poulter, B., Robertson, K., Rocha, A. V., Sadegh, M., Santos, F., Scordo, F., Sexton, J. O., Sharma, A. S., Smith, A. M. S., Soja, A. J., Still, C., Swetnam, T., Syphard, A. D., Tingley, M. W., Tohidi, A., Trugman, A. T., Turetsky, M., Varner, J. M., Wang, Y., Whitman, T., Yelenik, S., and Zhang, X.: Reimagine fire science for the anthropocene, PNAS Nexus, 1, pgac115, https://doi.org/10.1093/pnasnexus/pgac115, 2022.
Stocker, B. D., Wang, H., Smith, N. G., Harrison, S. P., Keenan, T. F., Sandoval, D., Davis, T., and Prentice, I. C.: P-model v1.0: an optimality-based light use efficiency model for simulating ecosystem gross primary production, Geosci. Model Dev., 13, 1545–1581, https://doi.org/10.5194/gmd-13-1545-2020, 2020.
Sutanto, S. J., Vitolo, C., Di Napoli, C., D'Andrea, M., and Van Lanen, H. A. J.: Heatwaves, droughts, and fires: Exploring compound and cascading dry hazards at the pan-European scale, Environ. Int., 134, 105276, https://doi.org/10.1016/j.envint.2019.105276, 2020.
Turco, M., Rosa-Cánovas, J. J., Bedia, J., Jerez, S., Montávez, J. P., Llasat, M. C., and Provenzale, A.: Exacerbated fires in Mediterranean Europe due to anthropogenic warming projected with non-stationary climate-fire models, Nat. Commun., 9, 3821, https://doi.org/10.1038/s41467-018-06358-z, 2018.
Turco, M., Jerez, S., Augusto, S., Tarín-Carrasco, P., Ratola, N., Jiménez-Guerrero, P., and Trigo, R. M.: Climate drivers of the 2017 devastating fires in Portugal, Sci. Rep., 9, 13886, https://doi.org/10.1038/s41598-019-50281-2, 2019.
Turner, D., Lewis, M., and Ostendorf, B.: Spatial indicators of fire risk in the arid and semi-arid zone of Australia, Ecol. Indic., 11, 149–167, https://doi.org/10.1016/j.ecolind.2009.09.001, 2011.
Tyukavina, A., Potapov, P., Hansen, M. C., Pickens, A. H., Stehman, S. V., Turubanova, S., Parker, D., Zalles, V., Lima, A., Kommareddy, I., Song, X.-P., Wang, L., and Harris, N.: Global Trends of Forest Loss Due to Fire From 2001 to 2019, Front. Remote Sens., 3, 825190, https://doi.org/10.3389/frsen.2022.825190, 2022.
United Nations Environment Programme: Spreading like Wildfire – The Rising Threat of Extraordinary Landscape Fires, A UNEP Rapid Response Assessment, Nairobi, 2022.
Van Wagner, C. E.: Development and structure of the Canadian Forest Fire Weather Index System, https://cfs.nrcan.gc.ca/pubwarehouse/pdfs/19927.pdf (last access: 4 December 2024), 1987.
Vilar, L., Camia, A., and San-Miguel-Ayanz, J.: A comparison of remote sensing products and forest fire statistics for improving fire information in Mediterranean Europe, Eur. J. Remote Sens., 48, 345–364, https://doi.org/10.5721/EuJRS20154820, 2015.
Wang, H., Prentice, I. C., Keenan, T. F., Davis, T. W., Wright, I. J., Cornwell, W. K., Evans, B. J., and Peng, C.: Towards a universal model for carbon dioxide uptake by plants, Nat. Plants, 3, 734–741, https://doi.org/10.1038/s41477-017-0006-8, 2017a.
Wang, X., Wotton, B. M., Cantin, A. S., Parisien, M.-A., Anderson, K., Moore, B., and Flannigan, M. D.: cffdrs: an R package for the Canadian Forest Fire Danger Rating System, Ecol. Process., 6, 5, https://doi.org/10.1186/s13717-017-0070-z, 2017b.
Winkler, K., Fuchs, R., Rounsevell, M., and Herold, M.: Global land use changes are four times greater than previously estimated, Nat. Commun., 12, 2501, https://doi.org/10.1038/s41467-021-22702-2, 2021.
Wu, M., Knorr, W., Thonicke, K., Schurgers, G., Camia, A., and Arneth, A.: Sensitivity of burned area in Europe to climate change, atmospheric CO2 levels, and demography: A comparison of two fire-vegetation models, J. Geophys. Res.-Biogeo., 120, 2256–2272, https://doi.org/10.1002/2015JG003036, 2015.
Zhang, T., de Jong, M. C., Wooster, M. J., Xu, W., and Wang, L.: Trends in eastern China agricultural fire emissions derived from a combination of geostationary (Himawari) and polar (VIIRS) orbiter fire radiative power products, Atmos. Chem. Phys., 20, 10687–10705, https://doi.org/10.5194/acp-20-10687-2020, 2020.
Zhu, C., Kobayashi, H., Kanaya, Y., and Saito, M.: Size-dependent validation of MODIS MCD64A1 burned area over six vegetation types in boreal Eurasia: Large underestimation in croplands, Sci. Rep., 7, 4181, https://doi.org/10.1038/s41598-017-03739-0, 2017.
Zubkova, M., Humber, M. L., and Giglio, L.: Is global burned area declining due to cropland expansion? How much do we know based on remotely sensed data?, Int. J. Remote Sens., 44, 1132–1150, https://doi.org/10.1080/01431161.2023.2174389, 2023.