the Creative Commons Attribution 4.0 License.
the Creative Commons Attribution 4.0 License.
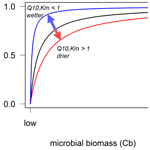
Modelling the effect of climate–substrate interactions on soil organic matter decomposition with the Jena Soil Model
Marleen Pallandt
Marion Schrumpf
Holger Lange
Markus Reichstein
Bernhard Ahrens
Soil organic carbon (SOC) is the largest terrestrial carbon pool, but it is still uncertain how it will respond to climate change. Specifically, the fate of SOC due to concurrent changes in soil temperature and moisture is uncertain. It is generally accepted that microbially driven SOC decomposition will increase with warming, provided that sufficient soil moisture (and hence sufficient C substrate) is available for microbial decomposition. We use a mechanistic, microbially explicit SOC decomposition model, the Jena Soil Model (JSM), and focus on the depolymerisation of litter and microbial residues by microbes at different soil depths as well as the sensitivities of the depolymerisation of litter and microbial residues to soil warming and different drought intensities. In a series of model experiments, we test the effects of soil warming and droughts on SOC stocks, in combination with different temperature sensitivities (Q10 values) for the half-saturation constant Km (Q10,Km) associated with the breakdown of litter or microbial residues. We find that soil warming can lead to SOC losses at a timescale of a century and that these losses are highest in the topsoil (compared with the subsoil). Droughts can alleviate the effects of soil warming and reduce SOC losses, by posing strong microbial limitation on the depolymerisation rates, and even lead to SOC accumulation, provided that litter inputs remain unchanged. While absolute SOC losses were highest in the topsoil, we found that the temperature and moisture sensitivities of Km were important drivers of SOC losses in the subsoil – where microbial biomass is low and mineral-associated OC is high. Furthermore, a combination of drought and different Q10,Km values associated with different enzymes for the breakdown of litter or microbial residues had counteracting effects on the overall SOC balance. In this study, we show that, while absolute SOC changes driven by soil warming and drought are highest in the topsoil, SOC in the subsoil is more sensitive to warming and drought due to the intricate interplay between Km, temperature, soil moisture, and mineral-associated SOC.
- Article
(1764 KB) - Full-text XML
- BibTeX
- EndNote
Soils are an important component of the global carbon (C) cycle, as they store large quantities of C (e.g. Crowther et al., 2019; Fan et al., 2020). Soils can act as C sources or sinks, depending on the balance between C inputs and outputs over time (Davidson, 2020; Kirschbaum, 2006). Apart from plant litter inputs, microbial residues are recognised as an important precursor for the formation of stable, mineral-associated soil organic carbon (Cotrufo et al., 2013; Kallenbach et al., 2016; Liang et al., 2017; Xiao et al., 2023). Therefore, to determine whether soils are a net C source or sink, the speed at which soil organisms decompose litter inputs and existing soil organic carbon (SOC) stocks including microbial residues is of particular importance (Kallenbach et al., 2016).
Soil temperature and soil moisture are two primary controlling factors of microbial decomposition rates and, thereby, the carbon turnover rate of soils (Davidson and Janssens, 2006; Moyano et al., 2013; Yan et al., 2018). Additionally, the interaction between microbial SOC decomposition and the adsorption and desorption of SOC to and from mineral surfaces is an important controlling factor determining the fate of SOC stocks (Ahrens et al., 2020; Dwivedi et al., 2017; Sokol et al., 2022). Given the importance of SOC stocks and their sensitivities to climate change, a better understanding and representation of these complex interactions in coupled C cycle–climate models is extremely important for a better understanding of the carbon–climate feedback (Todd-Brown et al., 2014).
Temperature and warming effects on SOC stocks and soil respiration have been extensively studied (Kirschbaum, 2006; Subke and Bahn, 2010; Tang et al., 2019), both experimentally (e.g. Benbi et al., 2014; Bradford et al., 2019; Carey et al., 2016; Chen et al., 2024; Conant et al., 2011; Gentsch et al., 2018; Gillabel et al., 2010; Hao et al., 2023; Hartley et al., 2021; Hicks Pries et al., 2017; Li et al., 2020; Moinet et al., 2020; Ofiti et al., 2021; Qin et al., 2019; Reichstein et al., 2005; Soong et al., 2021; Walker et al., 2018; Wang et al., 2022a; Yan et al., 2022) and with coupled C cycle–climate models (e.g. Bauer et al., 2008; Georgiou et al., 2024; Koven et al., 2017; Todd-Brown et al., 2018; Varney et al., 2020; Zhang et al., 2022). Most of these coupled C cycle–climate models, however, have simple process representation, with conceptual SOC pools that decay according to first-order kinetics and rate modifier functions to represent the effects of soil temperature and soil moisture changes and/or the effects of soil clay content on SOC decay rates (Le Noë et al., 2023; Sierra et al., 2015). Since the paradigm shift that SOC persistence is mediated by microbial activity and organo-mineral interactions (Schmidt et al., 2011), SOC decomposition and stabilisation can be more mechanistically described in models. This was realised by the development of next-generation soil models (NGSMs) of various complexity (Le Noë et al., 2023; Sulman et al., 2018). These NGSMs have the direct representation of microbial biomass (which drives depolymerisation of SOC pools through enzyme production) and the representation of mineral-associated organic matter, which both reduce microbial growth and, thus, depolymerisation by limiting substrate availability (e.g. Abramoff et al., 2017, 2019; Sulman et al., 2014; Tang and Riley, 2015, 2024; Wang et al., 2015; Wieder et al., 2014; Yu et al., 2020; Zhang et al., 2022). Through these mechanistic process representations in NGSMs, more explicit representations of their individual temperature and moisture dependencies can be explored.
According to current process understanding, it is now, for example, possible to assign microbial depolymerisation rates a higher temperature sensitivity than SOC sorption and desorption processes (Ahrens et al., 2020; Tang and Riley, 2015; Wang et al., 2012, 2013). However, of the enzyme-driven depolymerisation steps, which are often described using (reverse) Michaelis–Menten kinetics (Tang, 2015), primarily the effects of the temperature dependence of the maximum depolymerisation rate (Vmax) have been explored to date (Fanin et al., 2022; Liu et al., 2022; Nottingham et al., 2016; Wang et al., 2012). However, several studies have shown that the half-saturation constant, Km, is also temperature sensitive (Davidson et al., 2006; Davidson and Janssens, 2006; Wang et al., 2012, 2013). Although the number of laboratory studies on the Q10 values for Km of soil enzymes is limited, values have been shown to range between 0.7 and 2.8 for different enzymes (Allison et al., 2018b; Gershenson et al., 2009; Stone et al., 2012; Wu et al., 2022). When the Q10 value for Km>1, this indicates that the relationship between temperature and the substrate binding affinity of the enzyme (Km) is positive, whereas Q10 values <1 indicate a negative temperature sensitivity of Km (Allison et al., 2018b; Tang et al., 2019). In the Michaelis–Menten equation (Michaelis and Menten, 1913), Km is in the denominator, which means that a positive temperature sensitivity of Km can reduce the enzymatic reaction rate, or vice versa, increase the reaction rate when the temperature sensitivity of Km is negative (Fig. 1; Abramoff et al., 2019; Davidson et al., 2006; Davidson and Janssens, 2006).
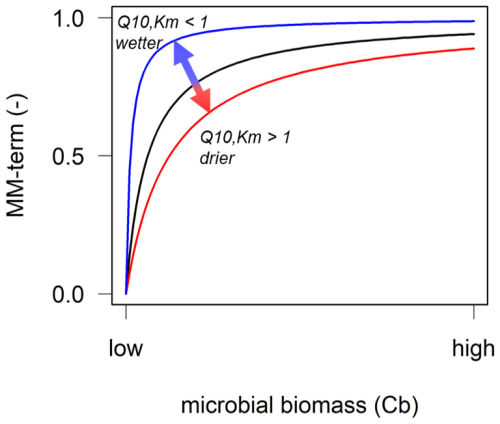
Figure 1Conceptual depiction of the relationship between microbial biomass (CB) and a reverse Michaelis–Menten (MM) term, defined as ), to represent microbial limitation of the depolymerisation rate. In a forward MM term (for e.g. microbial uptake of dissolved organic carbon), CB can be replaced by the substrate concentration. Temperature and soil moisture sensitivities of the half-saturation constant Km can increase or decrease the MM term: the temperature sensitivity of Km can be negative (Q10,Km<1) and, thus, increase the MM term (blue line); be absent (, black line); or be positive (Q10,Km>1) and, thus, decrease the MM term (red line). The MM term decreases when the soil gets drier (red line) or increases when the soil gets wetter (blue line).
At high substrate concentrations, the relative importance of the temperature sensitivity of Km may be smaller than that of Vmax (Wang et al., 2012), although this has not been explored much in modelling studies and only for positive temperature sensitivities of Km (Davidson et al., 2006). In their study, Davidson et al. (2006) used Q10 values of 2 for Vmax and values of 1, 1.5, or 2 for Km, and they showed that the individual temperature sensitivities of Km and Vmax can cancel each other out at low substrate concentrations. However, Davidson et al. (2006) did not study how dynamic changes in the substrate would interact with temperature sensitivity of Km. Furthermore, the soil enzyme kinetic study by Allison et al. (2018b) showed that the Q10 values of Km for enzymes primarily associated with the breakdown of plant-derived litter were generally positive (Q10 values >1), whereas those for enzymes associated with the breakdown of microbial residues were negative (Q10 values <1). Such opposing temperature sensitivities of Km for different substrate types have not been explored in dynamic modelling studies before.
Besides rising soil temperatures, soil moisture is also expected to change in the future with an increased risk of regional droughts (Berg and Sheffield, 2018). Drought can reduce temperature-induced SOC losses by reducing substrate availability for microbial decomposition (e.g. Schimel, 2018). The moisture sensitivity of the Michaelis–Menten term depends on soil moisture, with lower soil moisture values resulting in stronger substrate limitation on microbial uptake (in the case of forward MM kinetics) or stronger microbial limitation on enzymatic depolymerisation (in the case of reverse MM kinetics) (Fig. 1; Zhang et al., 2022). At present, the response to soil moisture changes is less well documented and implemented in microbially explicit SOC decomposition models (Wang et al., 2020), although some modelling work has integrated (semi-)mechanistic moisture sensitivity functions to represent substrate and sometimes also oxygen limitation on decomposition rates using volumetric water content (Davidson et al., 2012; Yan et al., 2018) or soil matric potential (Ghezzehei et al., 2019; Manzoni et al., 2014). In some NGSMs, reduced substrate availability directly affects microbial C uptake/growth and depolymerisation rates (Abramoff et al., 2017; Wang et al., 2015; Yu et al., 2020). Not only soil moisture but also organo-mineral interactions affect substrate availability for microbial depolymerisation, as microbes and soil minerals compete for the available SOC (Ahrens et al., 2015). In return, soil moisture and temperature affect mineral sorption and desorption rates. In NGSMs that are microbially explicit and include organo-mineral interactions, these temperature and moisture sensitivities can now also be specifically assigned to each process (Ahrens et al., 2020) and further explored in the context of climate change.
Albeit at lower concentrations, subsoils store huge amounts of organic carbon (OC) (e.g. Blume et al., 2016). Recently, the response of deep soils to climate change has received increased attention (Hicks Pries et al., 2023), especially with respect to soil warming (Chen et al., 2024; Hao et al., 2023; Hicks Pries et al., 2017; Li et al., 2020; Ofiti et al., 2021; Soong et al., 2021) and the role of mineral-associated organic matter (Benbi et al., 2014; Gentsch et al., 2018; Georgiou et al., 2024; Gillabel et al., 2010; Hartley et al., 2021; Sokol et al., 2022). However, to date, most NGSM studies have assumed that the soil is only composed of one homogenous layer (Wieder et al., 2015), thereby omitting the vertical profile, which displays differences in OC inputs from plant and roots, substrate availability, and microbial biomass as well as associated gradients in organo-mineral associations, temperature, and moisture (Hicks Pries et al., 2023). As the relevance of Km increases with declining microbial biomass (Fig. 1), these gradients within the soil profile will also affect SOC responses to climate change (Pallandt et al., 2022).
Overall, the climate sensitivities of microbially mediated SOC decomposition and their potential impacts on SOC stocks at different soil depths have been partially overlooked in dynamic modelling studies. In this study, we bridge these gaps by applying the C cycle version of the Jena Soil Model (JSM; Yu et al., 2020) to investigate the dynamic interactions between soil moisture, soil temperature, and C substrates at different soil depths. JSM is a vertically resolved NGSM with mechanistic descriptions of microbially driven decomposition and organo-mineral interactions; thus, C substrate depletion by microbes or sorption can be explicitly simulated at different soil depths. We use JSM to test the various soil temperature and soil moisture controls on SOC decomposition either individually or simultaneously. As such, these model experiments can be particularly helpful to elucidate the importance of these new processes. Specifically, we focus on the following research questions:
-
How do temperature and soil moisture changes affect modelled SOC decomposition through Vmax and the Michaelis–Menten term?
-
Do top- and subsoil layers respond differently to warming and drought?
In a series of model experiments, we test the effects of soil warming and different drought intensities on SOC stocks, in combination with different temperature sensitivities of Km associated with the depolymerisation of the polymeric litter pool or of microbial residues.
2.1 Model description
JSM is a vertically explicit soil organic matter (SOM) decomposition model with microbial interactions and the representation of organic matter (de)sorption to mineral surfaces. For this study, we represent and describe the C cycle, but JSM is also capable of simulating the coupled C, N, and P cycles and isotope (13C, 14C, and 15N) tracking. A full mathematical description of JSM and its coupled nutrient cycles can be found in the supplementary material of Yu et al. (2020). Here, we summarise the most important C cycle processes relevant to this study, with a conceptual overview given in Fig. 2 and parameters and units listed in Table 1.
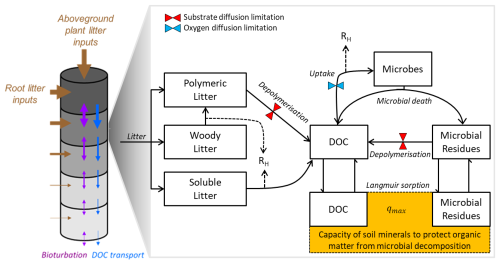
Figure 2Schematic representation of the C cycle in JSM, following Yu et al. (2020) and Ahrens et al. (2020). Each soil layer (grey cylinders) receives vertically resolved C litter inputs (brown arrows) from roots, and aboveground litter enters the first soil layer. Carbon can be transported between soil layers through bioturbation (purple arrows) and downwards with the water flux through advection (blue arrows). Carbon pools (black rectangles) and C fluxes (black arrows) are considered for each individual soil depth: litter inputs are partitioned into a polymeric, woody, and soluble litter pools. Polymeric litter and microbial residues can be depolymerised to DOC (dissolved organic carbon) by microbes. DOC and microbial residues can adsorb to mineral surfaces to form MAOM (mineral-associated organic matter; yellow box) and desorb through Langmuir sorption, where qmax is the maximum sorption capacity for the sorption of DOC and microbial residues to mineral surfaces. The dotted lines are heterotrophic respiration (RH) fluxes. The coloured hourglasses represent different soil moisture controls on SOC decomposition steps: microbial limitation of depolymerisation (red) and oxygen limitation (blue) on microbial C uptake for growth.
Table 1Parameter values and constants related to temperature-sensitive processes in JSM.
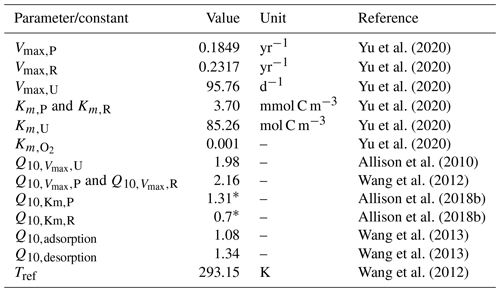
All Q10 values are reported for the model's reference temperature (Tref) of 293.15 K (20 °C), and their respective activation energies were taken from the literature (Allison et al., 2010; Wang et al., 2012, 2013) by Ahrens et al. (2020, their Table 1). Q10 values marked with an asterisk (*) are unique to this study (taken from Allison et al., 2018b) and were measured at a reference temperature of 289.15 K (16 °C). Activation energies for JSM were adjusted accordingly using Eq. (3). All units in JSM are SI units (moles, seconds, and kelvin); however, for table readability and direct cross-referencing, values are reported directly from the literature.
The soil profile is divided into multiple layers that receive vertically distributed root litter inputs or transported C from other soil layers via advection or bioturbation (Sect. 2.2; Ahrens et al., 2020). Additionally, the top soil layer receives aboveground plant litter inputs (Fig. 2). Above- and belowground non-woody litter inputs are partitioned into soluble and polymeric litter following Parton et al. (1993). JSM does not explicitly simulate enzyme production, but this is implicitly described using Michaelis–Menten (MM) kinetics. For the depolymerisation steps, reverse Michaelis–Menten kinetics is used, as Tang and Riley (2019) found these to be more appropriate than traditional, forward MM kinetics. The depolymerisation rate (mmol C m−3 h−1) of litter or microbial residues into the dissolved organic carbon (DOC) pool (following Ahrens et al., 2015, 2020) is described as follows:
Thus, the depolymerisation rate is limited by the size of the microbial biomass pool (CB) at a certain depth. X is either the polymeric litter pool (P) or the microbial residues pool (R) at a certain depth, Vmax,X is the maximum specific depolymerisation rate of X, CX is the respective litter pool X, and CB is the microbial biomass pool. ) is an exponential function expressed with a Q10 base (Wang et al., 2012):
where is the temperature sensitivity of the maximum specific depolymerisation rate of litter pool X, and Tsoil and Tref are the soil temperature and reference temperature, respectively.
The Q10 coefficient is the ratio of reaction rates when temperature increases by 10 K. For use within JSM, all Q10 values were converted to apparent activation energies (Ea) for the model's reference temperature (Tref) of 293.15 K following Eq. (7) from Wang et al. (2012):
where R is the universal gas constant and Texp is the temperature at which the experiment was conducted (Table 2). Inclusion of soil moisture is done through Eq. (4):
This is a function to describe the sensitivity of the half-saturation constant (Km,X) to soil moisture and temperature (Davidson et al., 2012), where is the temperature sensitivity of the half-saturation constant of CX, and θ and θfc are the volumetric water content and water content at field capacity, respectively.
Microbial C uptake for growth is described using traditional forward MM kinetics (Tang and Riley, 2019):
so that the uptake rate (mmol C m−3 h−1) is limited by the size of the available substrate (CDOC) and by the air-filled pore space (a), which is calculated as
and functions as a proxy to describe the amount of oxygen available for the reaction (Davidson et al., 2012). Vmax,U is the maximum uptake rate of DOC by CB, CDOC is the DOC pool, Km,U is the half-saturation constant for the uptake of DOC by CB, and is the half-saturation constant of the reaction with oxygen. DOC and microbial residues can adsorb to mineral surfaces to form MAOC (mineral-associated organic carbon), which is protected from microbial decomposition (yellow box in Fig. 2). In JSM, adsorption and desorption rates are temperature dependent (with literature-based Q10 values, as reported in Table 1), with a full description of the process implementation and successful application in Ahrens et al. (2020). The particulate organic carbon (POC) pool, accessible to microbes for depolymerisation and growth, consists of polymeric litter, DOC, and microbial residues (Fig. 2). Soil moisture, water fluxes between soil layers, and the maximum sorption capacity (qmax) are affected by soil texture (as described in Ahrens et al., 2015, 2020; Thum et al., 2019; and Sect. 2.1 in Yu et al., 2020).
2.2 Vertical process representation in the Jena Soil Model
JSM requires depth-specific soil temperature, soil moisture, and litterfall forcing data at a half-hourly time step as input. Following Thum et al. (2019), these soil forcing data were generated for a temperate forest site in Germany as a realistic test bed for in silico model experiments (Hainich, DE-Hai). First, the QUINCY land surface model was run for 500 years beforehand to bring the soil C pools into equilibrium, using site-specific information on soil physical and chemical parameters (soil texture, bulk density, and pH), plant functional type, rooting depth, and meteorological reanalysis data from 1901 to 1930. Second, directly following the 500-year spin-up period, QUINCY was run as a transient simulation in combination with FLUXNET3 forcing data for Hainich from 1901 to 2012. These soil forcing data were then used for the JSM spin-up for 500 years to bring the soil C pools in equilibrium, where soil forcing data from 2000 to 2012 were used repeatedly. The soil moisture and soil temperature forcing data from 2000 to 2012 are referred to as ambient soil moisture and soil temperature data from this point. After the 500-year spin-up period, JSM was run for 100 simulation years for each model experiment (Sect. 2.4), again using the soil forcing data from 2000 to 2012 repeatedly.
JSM works with 15 soil layers up to 9.5 m depth, where soil layer thickness increases with increasing soil depth (Yu et al., 2020). In this study we focus on the first six soil layers between 0 and 50 cm depth, at the following depth intervals: 0–6, 6–13, 13–20, 20–26, 26–36, and 36–50 cm. In each individual soil layer, all C pools and fluxes and the C fluxes between the different layers are tracked through time (Fig. 2, Eqs. 1–6). Through its vertically explicit structure and input data, the model is capable of considering processes and variables that vary with soil depth, such as soil moisture, soil temperature, root litter inputs, SOC content, microbial biomass, and the sorption and desorption of DOC and microbial residues to mineral surfaces. In the topsoil layers, microbial biomass is highest and closely follows the distribution of the root litter inputs (Ahrens et al., 2020) generated by the QUINCY model. In the deeper soil layers, the fraction of C adsorbed to mineral surfaces (MAOM) increases, and advective and diffusive transport C inputs decrease with depth (Ahrens et al., 2020). In JSM, all pools are transported via bioturbation according to the formulation by Jarvis et al. (2010) as a diffusive process whose diffusion coefficient decreases as a function of bulk density. The DOC pool is additionally transported via an advection velocity which represents the water mass flow between soil layers. A special feature of JSM is the vertically continuous modelling of potential organic layers and mineral soil layers via an additional advective transport term that accounts for the accumulation and decomposition of organic matter on top of and within the soil profile. This ensures that the build-up of organic matter, for example, in the form of an organic layer, leads to a concurrent decrease in the mineral soil volumetric fraction and, thereby, the sorption capacity qmax. For a full description of JSM, a reference is made to Yu et al. (2020).
2.3 Choice of Q10,Km values for polymeric litter and microbial residues
Microbes process SOC by depolymerising a wide array of C substrates derived from plant litter or microbial residues, which greatly differ with respect to chemistry (Buckeridge et al., 2022; Cotrufo and Lavallee, 2022), and therefore produce a variety of different enzymes that target those different substrate types. In JSM, the polymeric litter and microbial residues pools are depolymerised by extracellular enzymes produced by the microbial pool (CB) to enter the DOC pool (Fig. 2, Eq. 1). Enzyme production is not explicitly simulated, but it is assumed to be proportional to the size of the microbial biomass pool. The half-saturation constants for the depolymerisation of polymeric litter and microbial residues (Km,X; Eq. 1) are temperature dependent, but knowledge about their value is restricted to laboratory studies of individual enzymes. In this study, we explore different temperature sensitivities, expressed as Q10 values, for our model's half-saturation constants for the microbial depolymerisation of polymeric litter (Km,P) and microbial residues (Km,R). We base these Q10 values on the work of Allison et al. (2018b), who give an extensive overview of the temperature sensitivities of different enzymes and their substrate targets. We chose values from this study that would likely be, or closely resemble, the main enzymes involved in the breakdown of our model's polymeric litter (CP) and microbial residues (CR) pools. For the depolymerisation of CP, we targeted a value measured for the enzymes β-xylosidase and total oxidase, as these are involved in the degradation of hemicellulose, lignin, and phenolics. For the depolymerisation of CR, we selected a value measured for the enzyme leucine aminopeptidase, which is involved in the degradation of polypeptides, the main component of microbial cell walls. The selected value for the depolymerisation of polymeric litter is 1.3 (Table 2), i.e. a positive temperature sensitivity of Km, and that for the depolymerisation of the microbial residues is 0.7, i.e. a negative temperature sensitivity of Km. In the various model experiments (described in more detail in Sect. 2.4), we explore the effects of these different temperature sensitivities on SOC decomposition. Because so little is known about these effects through the temperature sensitivity of the Michaelis–Menten term, we conduct experiments in which we assign individual Q10,Km values for the depolymerisation of litter and microbial residues, but we also explore the effect of using only a negative temperature sensitivity of Km (representing a hypothetical situation in which all available C substrates have a Q10,Km value <1) or only a positive temperature sensitivity of Km (representing a hypothetical situation in which all available C substrates have a Q10,Km value >1).
2.4 Ambient model run and model experiments
After the 500-year spin-up period (Sect. 2.2), we conducted an ambient model run in which the 13 years of half-hourly ambient soil moisture and soil temperature forcing data (generated by the QUINCY model, as described in Sect. 2.2) were recycled for a 100-year simulation. This ambient model run represents the published C cycle version of JSM with its default settings (Yu et al., 2020); therefore, the results of the model experiments with varying temperature and moisture interactions can be evaluated against this default. To ensure that all model experiments started from steady-state conditions, we verify that the SOC pools between 0 and 50 cm depth reached steady state after the 500-year spin-up period by applying a simple linear regression on the slope of the change in SOC pools for the ambient model run. Then, to investigate the effects of soil warming on SOC decomposition, we ran several warming experiments in which all ambient soil temperatures were increased by 4.5 K throughout the whole soil column over the 100-year simulation period, keeping the original seasonality in the ambient input data intact and without altering the ambient soil moisture (SM) values. We chose a 4.5 K step increase for soil warming because soils, including the deep soil up to 1 m, are expected to warm by 4.5 K by the end of the century under Representative Concentration Pathway (RCP) 8.5 (Soong et al., 2020). To test the sensitivity of SOC decomposition to warming and to investigate the potential feedbacks through the temperature sensitivity of the half-saturation constants in the Michaelis–Menten term for microbial depolymerisation (Eq. 1), we ran four warming experiments using different values for and (Table 1). For the first warming experiment, both the and values were 1 (i.e. not temperature sensitive, default settings as in Yu et al., 2020). For the second warming experiment, we designated separate Q10 values for the breakdown of the microbial residues pool and the polymeric litter pool, where was set to 0.7 and was set to 1.3 (Sect. 2.3). Then, to explore the effects of only negative or positive Q10,Km values for depolymerisation (i.e. the effect of having different C substrate compositions), we ran warming experiment three, in which both and were 0.7 (representing a hypothetical situation in which all available C substrates would have a Q10,Km value below 1), and warming experiment four, in which both and were 1.3 (representing a hypothetical situation in which all available C substrates would have a Q10,Km value above 1). All model experiment settings are summarised in Table 2.
Then, to investigate the effects of soil warming and drying on SOC decomposition, we ran the first set of drought experiments; in these experiments, we kept all values at 1 and used ambient soil temperature + 4.5 K. Soil drying is expected for most of the globe (Wang et al., 2022b, and references therein), but drought intensity is uncertain and may vary locally (Cook et al., 2020; Hsu and Dirmeyer, 2023). Soil moisture change projections are very uncertain: 60 projected global lateral and vertical distributions of future soil moisture were highly diverse in their predicted lateral and vertical distributions (Berg et al., 2017). The multi-model mean of this study showed reductions in subsurface and deep surface soil moisture of up to 30 % by the year 2100. Given the large divergence between these model projections, we chose to simulate drought by reducing the depth-specific soil moisture values from the forcing dataset in steps of 10 %, to be able to compare the effects of a relatively mild versus increasingly stronger droughts on SOC stocks. Specifically, we compared three drought scenarios in which the model's ambient SM inputs were reduced by 10 %: each ambient SM value was multiplied by 0.9, 0.8, or 0.7, respectively (Table 2). As with the warming experiments, the original seasonality in the ambient SM input values was kept intact.
As a last step, we investigated the combined effects of soil warming and drying on SOC decomposition including the feedback through the half-saturation constants' temperature sensitivities. Similar to the first set of three drought experiments, ambient soil temperature was raised by 4.5 K, and three different drought intensities were simulated (SM × 0.9, SM × 0.8, and SM × 0.7). Reflecting the most likely realistic combination of Q10,Km values for microbial depolymerisation, as soil will contain both microbial residues and polymeric litter for microbes to depolymerise, we used the two individual Q10,Km values from Allison et al. (2018b) for the breakdown of the microbial residues pool and the polymeric litter pool ( and , respectively).
2.5 Model output analyses
Each model experiment was run for 100 simulation years, yielding daily output files for different soil variables. We calculate SOC stocks as the sum of the soluble litter, polymeric litter, DOC, microbial residues, adsorbed DOC, and adsorbed microbial residues pools (Fig. 2). Woody litter is excluded, as it is considered part of the aboveground litter layer. To calculate the annual changes in SOC stocks, expressed as percentage change (%) since the start of the simulation, we used SOC values from the last day of each simulation year. All analyses and plots were done using the “tidyverse”, “ggplot2”, and “viridis” packages with R version 4.3.1 in RStudio (Garnier et al., 2023; Posit Team, 2024; R Core Team, 2023; Wickham, 2016; Wickham et al., 2019). In Figs. 3, 4, 5, A1, and A2, solely as a visual aid, a smoothed line is added to the annual data points using the ggplot2 “geom_smooth()” function.
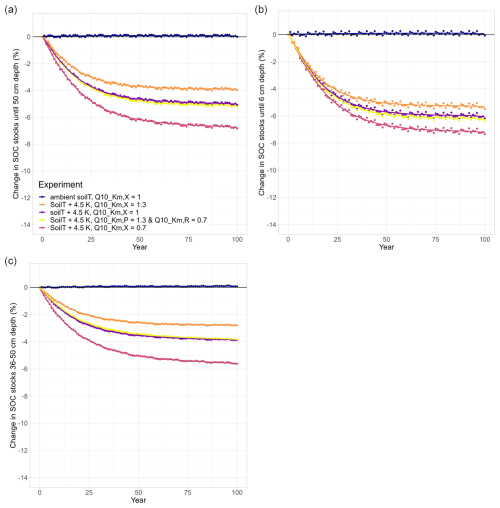
Figure 3Temperature effects on long-term changes in modelled SOC stocks (% SOC lost since simulation year 0) in (a) the whole soil column (0–50 cm), (b) the topsoil layer (0–6 cm), and (c) a subsoil layer (36–50 cm). Each subplot shows an ambient model run (dark blue) and the results of four warming experiments (soilT + 4.5 K) with different temperature sensitivities (Q10 values) of the half-saturation constants (). In all runs, ambient SM (SM × 1.0) was used.
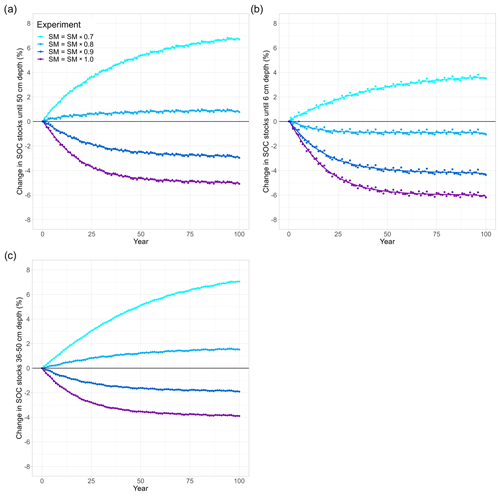
Figure 4Soil moisture and temperature effects on long-term changes in modelled SOC stocks (% SOC lost since simulation year 0) in (a) the whole soil column (0–50 cm), (b) the topsoil layer (0–6 cm), and (c) a subsoil layer (36–50 cm). In all model runs, the soil was warmed by 4.5 K. Soil moisture is reduced in 10 % steps from ambient levels (SM × 1.0, purple) to 70 % (SM × 0.7, light blue). In all runs, (not temperature sensitive). The purple line (SM = SM × 1.0) is included from Fig. 3 for visual comparison.
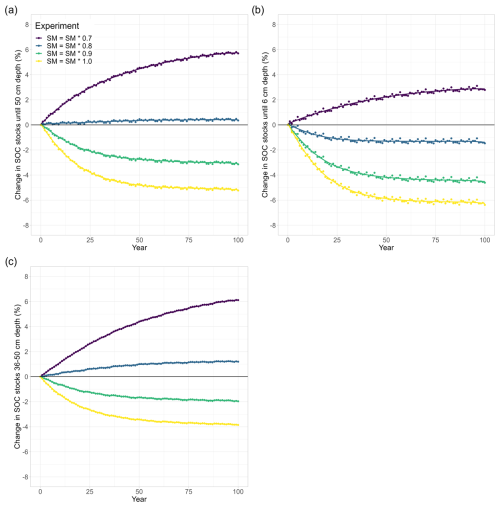
Figure 5Temperature and soil moisture effects on long-term changes in modelled SOC stocks (% SOC lost since simulation year 0) in (a) the whole soil column (0–50 cm), (b) the topsoil layer (0–6 cm), and (c) a subsoil layer (36–50 cm). Similar to Fig. 4, the soil was warmed by 4.5 K in all model runs and soil moisture was reduced in 10 % steps. In contrast to Fig. 4 (no temperature sensitivity of the half-saturation constants), was 1.3 for the depolymerisation of litter and was 0.7 for the depolymerisation of microbial residues in these model runs. For visual comparison, the yellow line (SM = SM × 1.0) is included from Fig. 3.
3.1 Warming effects on SOC decomposition
3.1.1 Modelled SOC stock changes at ambient and elevated soil temperatures
The ambient model run was conducted as a reference with which we could compare our model experiments. To check whether JSM reached steady state after spin-up, a linear regression test was carried out and confirmed that the first six soil layers of the ambient model run (0–50 cm) were in steady state, as there was no SOC loss or accumulation over the complete simulation period (dark blue in Fig. 3). The small interannual variability in modelled SOC stocks reflects the interannual variability in the litter inputs and other forcing. Warming the soil by 4.5 K in a model experiment led to SOC losses for all simulation years (purple in Fig. 3) until 5.1 % of the initial stock was lost by the end of the simulation period (Table 2). The topsoil loses more SOC (−6.2 %) than the subsoil (−3.9 %), which is related to (1) the fact that the proportion of mineral-associated organic C (MAOC) increases with depth, leaving less available substrate for microbes to depolymerise in the subsoil (Fig. A1), and (2) the lower microbial biomass in these layers, which strongly reduces the Michaelis–Menten term for the depolymerisation rates (Fig. 1). Additionally, the processes of adsorption and desorption have lower temperature sensitivities than microbial processes in JSM; therefore, warming affects the topsoil layers more strongly than the deeper layers, where more SOC is associated with mineral surfaces. The soil warming effect is strongest at the beginning of the simulation period, and it decreases towards the end of the 100-year simulation period.
3.1.2 Temperature sensitivity of the half-saturation constants
To study the effects of temperature on SOC decomposition through the half-saturation constant for depolymerisation (), the model was run using three different combinations of values for the depolymerisation of the polymeric litter and microbial residues pools (Table 2): both values were 0.7; both values were 1.3; or was 0.7 and was 1.3. Using a Q10 value for the half-saturation constants Km,P and Km,R of 0.7 (reflecting the temperature sensitivity of the depolymerisation of microbial residues) substantially accelerates SOC losses in response to warming. SOC losses until 50 cm depth reach 6.8 % (pink points in Fig. 3). The topsoil loses more SOC than the subsoil (−7.3 % and −5.6 %, respectively); however, in comparison with the run that uses values of 1, the relative difference in the subsoil is larger than in the topsoil. This indicates that the relative importance of is larger in the subsoil, where microbial biomass is lower, than in the topsoil. In contrast, using a Q10 value for the half-saturation constants Km,P and Km,R of 1.3 (reflecting the temperature sensitivity of the depolymerisation of the polymeric litter pool) counteracts the warming effect and reduces SOC losses from the soil. The result is still a net loss: SOC stocks in the top 50 cm decrease by 4 %, with higher SOC losses from the topsoil compared with the subsoil (−5.5 % and −2.8 %, respectively; orange points in Fig. 3). Similar to the model run in which is 0.7, the temperature sensitivity of Km in the model run in which both and Km,R are 1.3 has a relatively larger impact on the subsoil compared with the topsoil.
Using an individual Q10 value for the half-saturation constant for the depolymerisation of microbial residues (Km,R) of 0.7 and a value of 1.3 for the polymeric litter pool (Km,P) results in SOC losses from the top 50 cm (−5.2 %; yellow in Fig. 3). In comparison to the run in which , this result is very similar (5.1 % loss from warming alone), indicating that the opposing temperature sensitivities of the depolymerisation of the microbial residues and polymeric litter pools cancel each other out. The topsoil loses more SOC (−6.4 %) than the subsoil (−3.9 %); however, in comparison to the run in which , the losses from the topsoil layer are slightly higher (0.2 % additional SOC loss when ) compared with the subsoil (no difference).
3.2 Drought effects on SOC decomposition
Inducing drought strongly dampens the warming effect on SOC (Fig. 4). For the sake of comparison, Fig. 4 also includes the purple line from Fig. 3 (no drought, SM = SM × 1). Depending on the drought intensity, the top 50 cm of soil loses less SOC or even acts as a sink and starts accumulating SOC over the course of the simulation period. A 10 % reduction in SM results in an SOC loss of 3 %, whereas at 80 % and 70 % SM, the soil column accumulates 1.0 % and 6.8 % of SOC, respectively (Table 2). A stronger drought intensity led to a larger difference in modelled SOC stocks at 0–50 cm, from −5.1 % at the original SM to + 6.8 % at 70 % SM, resulting in a difference of 11.9 percentage points. This increased drought response of SOC decomposition is a direct result of the increase in the value of ) with decreasing SM (θ; Eq. 2). Again, the topsoil and subsoil layers show a different response: in the topsoil, there is high microbial biomass; therefore, the effect of the drought on microbial depolymerisation is not as strong as in the subsoil. Additionally, drought decreases the amount of MAOC in the subsoil, while POC accumulates (Fig. A1). As a result, the POC : MAOC ratio increases, especially in the topsoil (Fig. A2). In the 0–6 cm layer, SOC losses are 4.4 % at 90 % SM and 1.1 % at 80 % SM, whereas a 3.8 % SOC accumulation is noted at 70 % SM. In the subsoil layer between 36 and 50 cm, there is a loss of 1.9 % SOC at 90 % SM, whereas there is a 1.6 % and 7.1 % SOC accumulation at 80 % and 70 % SM, respectively. At 80 % SM, the drought has an opposite effect in the topsoil (a net source) compared with the subsoil (a net sink). The whole column response, however, is a net sink, highlighting the strong contribution of the subsoil to the overall response.
3.3 Combined effects of drought and temperature sensitivity of the half-saturation constants on SOC decomposition
To investigate the potentially counteracting responses of the temperature sensitivity of Km and droughts, we also run the model for the three different drought intensities in conjunction with a value of 1.3 for the polymeric litter pool and a value of 0.7 for the microbial residues pool. Under ambient SM conditions, the temperature sensitivity of both Km,X values only marginally amplified the warming effects (the yellow points are identical in Figs. 3 and 5). When SM is reduced, however, this slows down the decomposition rates (Fig. 5, Table 2): at a 10 % reduction in available SM, less SOC is lost from the top 50 cm (−3.1 %) compared with when SM is kept at ambient levels (−5.2 %). At 80 % and 70 % SM, the soil starts accumulating SOC (+0.5 % and 5.8 %, respectively). Generally, modelled SOC stocks for this combination of temperature sensitivities of Km and different drought intensities closely resemble the simulated drought response (Fig. 4), with the temperature sensitivity of Km counteracting the drought effects: when Km is sensitive to the temperature, SOC losses are always higher, and SOC accumulations are always smaller than when Km is not sensitive to temperature (Table 2). Similar to the drought experiments (Sect. 3.2), the differences in modelled SOC stocks at 0–50 cm increase with stronger drought intensity. Interestingly, the temperature sensitivity effect through and also increases with stronger drought intensity; for example, at ambient SM, SOC stocks decreased by 5.1 % when both values were 1, whereas they decreased by 5.2 % when and , which is a difference of only 0.1 percentage points (Table 2). However, at 70 % SM, SOC stocks increased to 6.8 % when both values were 1 and increased to 5.8 % when and , resulting in a difference of 1.0 percentage point. The same trend is also visible for both the topsoil (relative difference from 0.2 percentage points at SM = 1.0 to 0.7 percentage points at SM = 0.7) and the subsoil (no difference at SM = 1.0 to a 1.0-percentage-point difference at SM = 0.7). At the same time, the POC : MAOC ratio did not change much compared to the model run in which Km,X was not temperature sensitive (yellow and pink in Fig. A2). This indicates that, rather than causing a shift in the litter and microbial residues C pools, microbial limitation is strong under dry conditions (low CB; Fig. 1), which in turn increases the importance of and for the overall SOC decomposition rates. In contrast to the results from the isolated warming and drought experiments, the differences in SOC stock changes between the topsoil and subsoil are not very large: from a −6.4 % SOC loss to a +3.1 % SOC accumulation, which is a 9.5 percentage point change, in the topsoil and from a −3.9 SOC loss to a +6.1 SOC accumulation in the subsoil (Table 2). These results indicate that the combined sensitivity of SOC stocks to moisture and temperature in topsoil and subsoil is similar due to the counteracting effects of temperature and soil moisture on Km (Eq. 4): higher temperatures promote SOC decomposition rates due to the stronger influence of the depolymerisation of microbial residues (which have a value of 0.7), whereas drought decreases SOC decomposition rates.
4.1 Warming effects on SOC decomposition
We find that warming the soil by 4.5 K accelerates SOC losses and that these losses are proportionally higher in the topsoil compared with the subsoil. This is expected, as a higher soil temperature increases the maximum depolymerisation rates and microbial growth rates through (Eq. 2). Our findings are also consistent with other modelling studies that investigated isolated soil warming effects (Pallandt et al., 2022; Sulman et al., 2018; Todd-Brown et al., 2014; Wieder et al., 2018) as well as with the results of a recent study inferring space-for-time-substitution SOC estimates from a meta-analysis of profiles (Wang et al., 2022a), which reported higher losses of SOC stock and SOC content from topsoil (0–30 cm) compared with subsoils (0.3–1 m). In our study, during the warming experiments, the topsoil almost always lost more SOC than the subsoil, except when and were both set to 0.7 and SOC losses were accelerated in the subsoil (−5.6 % loss). Two depth-dependent model processes play an important role in these top- and subsoil differences. Firstly, microbial biomass (Cb) decreases with depth, and as microbial biomass declines, the Michaelis–Menten term for depolymerisation decreases (Fig. 1, Eq. 1), thereby limiting the depolymerisation rates at lower depths. Secondly, SOC is protected from microbial decomposition by sorption to mineral surfaces, and the proportion of mineral-associated organic carbon (MAOC, consisting of adsorbed DOC and microbial residues) strongly increases with soil depth (Fig. A1). The increase in the proportion of MAOC is partly driven by the advective transport term that represents the downward displacement of the mineral matrix and SOC when organic matter builds up in organic layers and within the soil profile. The lower SOC concentrations with increasing soil depth lead to a higher proportion of the soil volume being occupied by minerals compared with organic matter. The higher mineral soil volume thereby provides a higher sorption capacity qmax. Adsorption rates in the subsoil are consequently higher than in the topsoil, as qmax is farther from saturation. In JSM, the Q10 values of the mineral-associated C pools are 1.08 for adsorption and 1.34 for desorption, which are much lower than the Q10 values of the particulate organic carbon (POC) pools: the Q10 value for microbial depolymerisation of polymeric litter and microbial residues is 2.16, whereas it is 1.98 for microbial C uptake (Table 1; Allison et al., 2010; Wang et al., 2012, 2013). These literature-based values reflect the current understanding that MAOM is less temperature sensitive than POC (Bradford et al., 2016; Tang and Riley, 2015). Thus, as the ratio of MAOC to POC strongly increases in the subsoil, this leads to an overall lower apparent temperature sensitivity of SOC pools in the subsoil. Furthermore, higher adsorption rates in the subsoil lead to this higher proportion of MAOC to POC, and as the adsorption rate has the lowest Q10 value of all the rates in JSM (Table 1), this further contributes to the emergent lower temperature sensitivity with increasing depth. Total SOC losses consist of DOC, POC, and MAOC from the 36–50 cm subsoil layer, so the overall SOC losses may be relatively small, as the majority of SOC in this layer consists of protected MAOC – which decreases its overall temperature sensitivity.
Whether or not the apparent temperature sensitivity of SOC declines with depth, as we observe in this study, is still a topic of debate. According to kinetic theory (Bosatta and Ågren, 1999), subsoils may have lower apparent Q10 values when they contain less complex, necromass-derived substrates (Davidson and Janssens, 2006; Hicks Pries et al., 2023). Contrastingly, the same argument is used to explain higher temperature sensitivities in subsoils when they may contain molecules with higher activation energies (e.g. Li et al., 2020). These observed higher temperature sensitivities could be the result of deriving the apparent Q10 values from bulk soil samples containing both POC and MAOC, although several other studies have demonstrated that this trend can be counteracted by the strong mineral protection of SOC in subsoils (Gentsch et al., 2018; Gillabel et al., 2010; Qin et al., 2019) and that reported high apparent Q10 values originate from the decomposition of POC (Soong et al., 2021). In a recent review, Hicks Pries et al. (2023) concluded that the temperature response of deep soils is likely to be context dependent and that subsoils with a high POC content or a low reactive mineral content are likely to be more susceptible to warming than soils with limited POC or with highly reactive mineral surfaces that protect SOC from microbial decomposition. In our model experiments, the relative contribution of MAOC strongly increased with soil depth, which resulted in smaller total SOC losses from the subsoil than the topsoil layer in response to warming.
We observe stronger model responses to different values in subsoils than in topsoils, firstly, because microbial limitation is stronger in subsoils than in topsoils. At low microbial biomass (CB), the value of Km,X becomes increasingly important (Fig. 1, Eq. 1). At the same time, depolymerisation rates only affect the POC pools (CP and CR) and not the MAOC pools (adsorbed DOC and adsorbed microbial residues). As the POC : MAOC ratio is low in subsoils (Fig. A2), the total SOC losses from subsoils are lower than from the topsoil, despite the higher sensitivity to different values. Therefore, when <1, SOC losses can be further accelerated, especially in the deep soil. In our study, this lower Q10 was associated with the breakdown of proteins from the microbial residues pool. The contribution of microbial residues to total SOC in the deep soil is highly significant and can be up to 54 % in grasslands (Wang et al., 2021). If free POC in deep soils is indeed more sensitive to warming as a result of low microbial biomass, our model results support the finding that deep soils which are rich in microbial residues are more sensitive to temperature changes than those that contain less microbially derived POC, due to the lower Q10,Km value of the breakdown of polypeptides. However, compared with plant-derived POC, microbial residues have a high mineral sorption potential (Buckeridge et al., 2022; Liu et al., 2021) and could, therefore, be more protected from decomposition.
4.2 Drought effects on SOC decomposition
Our results show that soil drying can alleviate the losses of SOC from soil warming. In our model, this is the result of the soil moisture sensitivity of the half-saturation constants for microbial depolymerisation (Km,R and Km,P; Eq. 4): lower soil moisture reduces the Michaelis–Menten term for depolymerisation (Fig. 1), which lowers the SOC decomposition rates. Microbial C uptake for growth is also sensitive to changes in soil moisture through changes in the air-filled pore space (Eqs. 5 and 6), but this would result in faster SOC decomposition rates, as microbial growth is less affected by oxygen limitation, which was not the case for any of the modelled drought experiments. Generally, SOC decomposition peaks at intermediate soil moisture, but most soils are below these optimal soil moisture levels; as a result, drying leads to reduced decomposition rates due to stronger microbial limitation, whereas wetting the soil leads to an acceleration of the decomposition rates until oxygen limitation limits SOC decomposition rates (Davidson et al., 2012; Moyano et al., 2018; Pallandt et al., 2022; Skopp et al., 1990; Yan et al., 2018). In our model framework, substrate and oxygen limitation is split between two processes: we simulate moisture-driven diffusion limitation on the microbial depolymerisation rates (reverse MM kinetics; Eq. 4), and oxygen and DOC availability affect microbial growth (forward MM kinetics; Eq. 5). We found that soil drying consistently reduced modelled SOC losses compared with SOC losses due to soil warming alone, indicating that microbial limitation of depolymerisation is more important than oxygen limitation on microbial growth in our study. Additional support for strong microbial limitation on SOC decomposition comes from our observation that particulate organic C (POC) accumulates in both the topsoil and subsoil layers in response to the most intense drought scenario (SM = SM × 0.7; Fig. A1). If microbes were not limited by drought, they would degrade POC quickly in response to warming.
Our finding that microbial SOC decomposition consistently declines in response to drought is in agreement with other studies that have explored drought effects on SOC decomposition using microbially explicit models (Liang et al., 2021; Wang et al., 2020; Zhang et al., 2022). In the topsoil, we find that the impact of each 10 % reduction in SM has a relatively small impact on alleviating SOC losses through warming, compared with the subsoil (Fig. 4). These observed differences in the drought response between top- and subsoil can mainly be explained by the vertical differences in the microbial biomass concentration (CB), which is higher in the topsoil than in the subsoil. Therefore, at low CB, the relative impact of drought on the MM term for depolymerisation is larger in the subsoil than in the topsoil, making the modelled subsoil SOC stocks more sensitive to drought. For example, at 80 % SM, modelled SOC stocks in the topsoil decrease in response to soil warming (from −6.2 % to −1.1 % as SM decreases to 80 %, or a net difference of 5.1 percentage points), whereas subsoil SOC stocks decrease at ambient SM but increase at 80 % SM (from −3.9 % to +1.6 %, or a difference of 5.5 percentage points). As discussed in Sect. 4.1, the relatively higher sensitivity of the subsoil to not only warming but also to drought is also related to the strong increase in MAOC with depth and its lower temperature sensitivity compared with that of the POC pools. In order to focus completely on drought effects on microbial SOC decomposition, adsorption and desorption rates were not sensitive to changes in soil moisture during our experiments. Drought favours the stabilisation of SOC on mineral surfaces (Blankinship and Schimel, 2018), thereby protecting it from microbial depolymerisation. Therefore, if we considered the moisture sensitivity of adsorption and desorption rates in our model, we would expect a further decrease in the SOC decomposition rates in response to drought. However, to our knowledge, the formulation of moisture sensitivity of adsorption and desorption is not well established.
Overall, our model results indicate a potential for net SOC accumulation at 0–50 cm depth when SM is reduced to 80 % or 70 % of its original values and that a large part of the whole soil column response is driven by the subsoil. While data-driven deep-soil drying studies are rare, our simulation results are supported by a recent study (Brunn et al., 2023) in which total annual precipitation throughfall was reduced by 70 % for 5 consecutive years and both SOC stocks and SOC stability increased between 0 and 30 cm. This prior work found that the majority of the SOC stock increase occurred in the top 5 cm as a result of higher root exudates, but we do not consider this in our model experiments. We found that the largest SOC stock increase occurred in the subsoil, because of the higher sensitivity of subsoil to drought at low microbial biomass concentrations and the strong protection of MAOC from microbial depolymerisation. Our finding that SOC stocks can potentially increase with drought, despite the expected losses through warming, is mainly the result of lower microbial depolymerisation (Eq. 4, Fig. 1). Indeed, short-term studies indicate that SOC stocks may increase under drought conditions, as a strong reduction in microbial activity may dominate over the effect of reduced litter and root inputs (Brunn et al., 2023; Deng et al., 2021; Moyano et al., 2013). While results from short-term data-driven studies support our modelling results, long-term drought studies generally show a decline in SOC stocks, which can be mainly attributed to the effects of soil warming and decreased litter inputs (Deng et al., 2021; Meier and Leuschner, 2010). An advantage of our stand-alone soil model environment with prescribed litter inputs is that it allows us to individually test soil warming and drying effects on long-term SOC stocks, while eliminating the potentially confounding effects from changes in plant productivity. Recent research has shown that the chances of drought coinciding with high soil temperatures will further increase in the future (García-García et al., 2023). As a result, the counteracting effects of Km and drought may be at their strongest, and (semiarid) ecosystems dominated by infrequent moisture inputs may show strong sensitivities to soil warming and drought.
4.3 Combined effects of moisture and temperature sensitivity of the half-saturation constants on SOC decomposition
Our results indicate that the temperature sensitivity of the half-saturation constants and had a relatively smaller impact on the overall SOC stock changes (Fig. 5c), compared with the large impact of soil warming on SOC stocks through the temperature sensitivity of the maximum depolymerisation rate, (Fig. 3c). This smaller impact of the half-saturation constants for depolymerisation on SOC stocks was theorised by Wang et al. (2012) if Q10 values for Km are close to 1, as is the case in our study. Saifuddin et al. (2021) also reported a small impact of Q10,Km versus on simulated SOC stocks, but their model study did not consider multiple soil depths, the effects of drought, or organo-mineral interactions. In this study, we show that soil drying in combination with the temperature sensitivity of the half-saturation constants for the depolymerisation of polymeric litter and microbial residues can both increase and decrease SOC stocks and that the direction and magnitude of the effect on SOC stocks depends on drought intensity. The combined effects of soil drying and temperature sensitivity of the half-saturation constants for depolymerisation on SOC stocks closely resembled that of the drought response, which indicates that microbial limitation on depolymerisation poses a strong control on modelled SOC stocks and that drought can indeed alleviate SOC losses in response to soil warming. While the effect of drought on modelled SOC stocks is strong, the temperature sensitivity of Km,X can counteract these effects: compared with the model runs without temperature sensitivity of Km,X, SOC losses are higher and SOC accumulations are smaller. This indicates that the breakdown of microbial residues, which had a value of 0.7, is important for the overall results, as a Q10 value lower than 1 increases the MM term for depolymerisation and accelerates SOC decomposition. Furthermore, this counteracting effect of is stronger with increased drought intensity, while the POC : MAOC ratio does not change much when compared to the model run in which is not temperature sensitive. In line with our results from the isolated drought experiments (Sect. 4.2), this supports the conclusion that microbial limitation increases under drought conditions, so that becomes more important for the overall depolymerisation rates.
Unlike the individual warming and drought experiments, we only find small differences in SOC stock changes between the top- and subsoil for the combination of drought and temperature sensitivity of Km,R and Km,P. This shows that drought and temperature sensitivity can both play a strong role and can counteract each other so that the overall changes in SOC stocks appear similar. This is an important result, as long-term warming can accelerate soil drying, especially at the soil surface (Berg and Sheffield, 2018; Fan et al., 2022; García-García et al., 2023). Our results show divergent responses of top- and subsoil SOC stocks to concurrent soil warming and drying, in particular at a 20 % SM reduction, where modelled SOC stocks increase in the topsoil but decrease in the subsoil. While we only explored the effects of evenly drying out the soil column in this study, the long-term response of SOC stocks to soil moisture changes could be different, as top- and subsoils may not dry out evenly (Berg et al., 2017). Using multi-model predictions, Berg et al. (2017) showed that surface soil moisture decreases by the end of the century, whereas subsoils, especially in the Northern Hemisphere, diverge with either less-severe drying or wetter conditions. On top of soil warming, such dynamic vertical changes in soil moisture have a strong potential to further accelerate or slow down SOC decomposition rates in the deep soil by microbial limitation or oxygen diffusion limitation (Pallandt et al., 2022). We call for modelling studies that address such changes simultaneously by running NGSMs with future climate forcing datasets.
4.4 Microbial response to substrate changes in the POC–MAOC framework
The duration of our experiments is 100 simulation years, but the values of and may not remain constant over time, as the environment changes and microbial communities adapt. However, in light of our long-term warming experiments, we feel confident with the choice of Q10,Km values, as they were measured in microorganisms that showed no sensitivity to a 6 K increase in average temperature but that did show a strong response to changes in substrate type (Allison et al., 2018a). In our model experiments, microbes have access to both litter inputs and microbial residues to depolymerise, which have counteracting Q10,Km values; thus, Q10,Km can simultaneously accelerate and slow down microbial SOC decomposition rates. Therefore, it would be useful to consider soils that are high in POC versus soils that are high in MAOC: soils with high MAOC contents and low litter inputs can have lower apparent Q10 values because Q10,sorption is much lower than the Q10 values of unprotected organic carbon (Table 1; Wang et al., 2012, 2013). Such soils would have necromass rather than fresh litter inputs as the dominant C substrate for microbes. New datasets, such as global maps of necromass C contributions to total SOC stocks (e.g. Liu et al., 2021), can provide modellers with information on substrate type or SOC stabilisation mechanisms and, thereby, help identify the climate sensitivities of SOC stocks in different regions of the world. At the moment, however, there are no clear answers as to which values we should use for , as SOC consists of many different molecules that all have their own specific temperature sensitivities (Allison et al., 2018b). One possibility to investigate the potential climate–substrate feedbacks with a model like JSM would be a further partitioning of the litter pools into functional groups related to their main degrading enzymes. Our current study, which explores different values for , already provides valuable insights into what might be possible. For example, soils with high POC contents, i.e. with a developed organic layer as a result of high litter inputs, low SOC losses, and low bioturbation, are likely to have values >1, which have the potential to counteract soil warming effects through , especially in deeper layers where microbial biomass is low and the temperature sensitivity of the half-saturation constant will have a stronger impact. In combination with soil drought, this would further enhance microbial limitation for depolymerisation and could dampen SOC losses in such organic soil layers over time – if litter inputs remain constant over time. Peat soils could be an exception, as they usually have high volumetric water contents, and reduced soil moisture can lift oxygen limitation, thereby increasing SOC decomposition rates. It can be expected, however, that long-term soil drying would reduce root and leaf litter inputs as plant productivity decreases (Deng et al., 2021). Plants and microbes could also actively compete for nutrients in such a coupled model environment, which, apart from the climatic sensitivities investigated in this study, will introduce additional non-linear feedbacks on future SOC dynamics through changes in factors such as litter inputs and microbial carbon use efficiency (Braghiere et al., 2022; Thurner et al., 2024). Therefore, we recommend that future research focuses on further studying climate–substrate interactions within a fully coupled soil–plant model, such as the coupling between land surface model QUINCY (Thum et al., 2019) and JSM, which is nearing completion.
With our JSM experiments, we show that both soil drying and warming pose strong controls on SOC decomposition. The vertically explicit model structure allows us to demonstrate that subsoil SOC stocks respond differently to warming and drought through a combination of processes. First of all, we show that SOC association with mineral surfaces plays an important role in reducing the overall sensitivity of SOC stocks to microbial decomposition: MAOC strongly increases with soil depth and has a low apparent temperature sensitivity, which results in smaller total SOC losses from the subsoil than the topsoil. At the same time, our model results indicate that unprotected subsoil SOC is more sensitive to soil warming and drought. Secondly, we show that drought can alleviate the effects of soil warming through microbial limitation on depolymerisation rates. As drought gets stronger, microbially mediated depolymerisation rates become severely limited so that less SOC is lost from the soil. In the model experiments with constant litter inputs in this study, this can even lead to SOC accumulation over time, despite soil warming. Thirdly, we show that considering the temperature sensitivities of the half-saturation constants for different C substrates (litter and microbial residues) is important, as they can both slow down and accelerate microbial SOC decomposition rates. Our results highlight the importance of representing SOC decomposition processes in a vertically resolved model, which includes carbon stabilisation on mineral surfaces. We recommend that future model development focuses on further identifying the (un)importance of the temperature sensitivities of Vmax and Km,X for different C substrates and moisture sensitivities of all microbial–mineral interactions in the new class of soil organic carbon models.
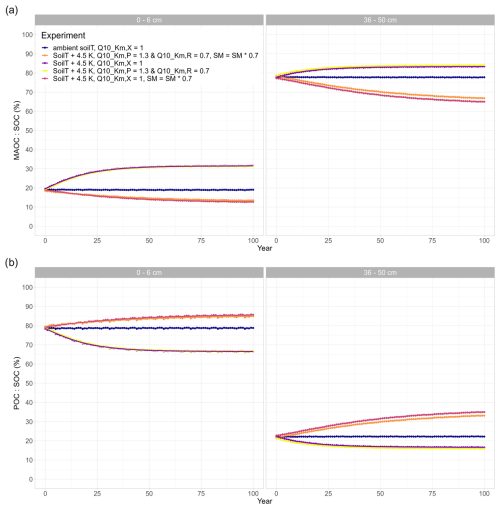
Figure A1(a) Ratio of mineral-associated organic carbon (MAOC) to SOC (%) and (b) particular organic carbon (POC) to SOC (%) for different model runs at two different soil depths: topsoil (0–6 cm) and subsoil (36–50 cm). If not indicated otherwise, SM = SM × 1.0 in the experiment.
The Jena Soil Model (JSM; release01) is fully described in Yu et al. (2020, https://doi.org/10.5194/gmd-13-783-2020). The JSM source code is available online (https://git.bgc-jena.mpg.de/quincy/quincy-model-releases, last access: 21 March 2025, branch “jsm/release01”), but access is restricted to registered users. Readers interested in running the model should request a username and password from Sönke Zaehle or via the Git repository. JSM is developed using the framework of the QUINCY model (Thum et al., 2019, https://doi.org/10.5194/gmd-12-4781-2019). The QUINCY model is free software: it can be distributed and/or modified under the terms of version 3 of the GNU General Public License (https://www.gnu.org/licenses/gpl-3.0.en.html, last access: 27 January 2025). The use of the QUINCY model relies on the application of software developed by the Max Planck Institute for Meteorology, which is subject to the MPI-M ICON software licence (see ICON section: “By using ICON, the user accepts the individual licence, https://code.mpimet.mpg.de/attachments/download/20888/MPI-M-ICONLizenzvertragV2.6.pdf, last access: 27 January 2025). Where software is supplied by third parties, such as the Max Planck Institute for Meteorology, it is indicated in the header of the file. Model users are strongly encouraged to follow the fair-use policy stated at https://www.bgc-jena.mpg.de/en/bsi/projects/quincy/software (last access: 27 January 2025).
MP wrote the original draft of the manuscript with contributions from all co-authors. BA and LY wrote the original JSM model code (version 1.0; see the “Code availability” section), MP and BA made minor code modifications to run the model experiments (as described in Sect. 2), and MP performed the model simulations and created all visualisations. This study was conceptualised by MP, BA, MS, and HL. Formal analysis was carried out by MP and BA. Funding acquisition was carried out by BA, HL, and MR. Supervision of MP was the responsibility of BA, HL, MS, and MR.
The contact author has declared that none of the authors has any competing interests.
Publisher’s note: Copernicus Publications remains neutral with regard to jurisdictional claims made in the text, published maps, institutional affiliations, or any other geographical representation in this paper. While Copernicus Publications makes every effort to include appropriate place names, the final responsibility lies with the authors.
Marleen Pallandt is grateful for discussions with Sönke Zaehle in the early phases of this study as well as for technical support from Julia Nabel and Jan Engel from the QUINCY modelling team at the Max Planck Institute for Biogeochemistry in Jena. Marleen Pallandt received funding support for this work from the Norwegian Research Council (grant no. RCN 255 061; MOisture dynamics and CArbon sequestration in BOReal Soils) and the Max Planck Institute for Biogeochemistry in Jena. During the revisions of the manuscript, Marleen Pallandt was supported by Stockholm University and the European commission through the “Holistic management practices, modelling and monitoring for European forest soils” – HoliSoils project (EU Horizon 2020; grant no. 101000289).
This research has been supported by the Norges Forskningsråd (grant no. RCN 255 061) and the European Union's Horizon 2020 Research and Innovation programme (grant no. 101000289).
The article processing charges for this open-access publication were covered by the Max Planck Society.
This paper was edited by Bertrand Guenet and reviewed by two anonymous referees.
Abramoff, R., Davidson, E., and Finzi, A. C.: A parsimonious modular approach to building a mechanistic belowground carbon and nitrogen model, J. Geophys. Res.-Biogeo., 122, 2418–2434, https://doi.org/10.1002/2017JG003796, 2017.
Abramoff, R. Z., Torn, M. S., Georgiou, K., Tang, J., and Riley, W. J.: Soil Organic Matter Temperature Sensitivity Cannot be Directly Inferred From Spatial Gradients, Glob. Change Biol., 33, 761–776, https://doi.org/10.1029/2018GB006001, 2019.
Ahrens, B., Braakhekke, M. C., Guggenberger, G., Schrumpf, M., and Reichstein, M.: Contribution of sorption, DOC transport and microbial interactions to the 14C age of a soil organic carbon profile: Insights from a calibrated process model, Soil Biol. Biochem., 88, 390–402, https://doi.org/10.1016/j.soilbio.2015.06.008, 2015.
Ahrens, B., Guggenberger, G., Rethemeyer, J., John, S., Marschner, B., Heinze, S., Angst, G., Mueller, C. W., Kögel-Knabner, I., Leuschner, C., Hertel, D., Bachmann, J., Reichstein, M., and Schrumpf, M.: Combination of energy limitation and sorption capacity explains 14C depth gradients, Soil Biol. Biochem., 148, 107912, https://doi.org/10.1016/j.soilbio.2020.107912, 2020.
Allison, S. D., Wallenstein, M. D., and Bradford, M. A.: Soil-carbon response to warming dependent on microbial physiology, Nat. Geosci., 3, 336, https://doi.org/10.1038/ngeo846, 2010.
Allison, S. D., Romero-Olivares, A. L., Lu, L., Taylor, J. W., and Treseder, K. K.: Temperature acclimation and adaptation of enzyme physiology in Neurospora discreta, Fungal Ecol., 35, 78–86, https://doi.org/10.1016/j.funeco.2018.07.005, 2018a.
Allison, S. D., Romero-Olivares, A. L., Lu, Y., Taylor, J. W., and Treseder, K. K.: Temperature sensitivities of extracellular enzyme Vmax and Km across thermal environments, Glob. Change Biol., 24, 2884–2897, https://doi.org/10.1111/gcb.14045, 2018b.
Bauer, J., Herbst, M., Huisman, J. A., Weihermüller, L., and Vereecken, H.: Sensitivity of simulated soil heterotrophic respiration to temperature and moisture reduction functions, Geoderma, 145, 17–27, https://doi.org/10.1016/j.geoderma.2008.01.026, 2008.
Benbi, D. K., Boparai, A. K., and Brar, K.: Decomposition of particulate organic matter is more sensitive to temperature than the mineral associated organic matter, Soil Biol. Biochem., 70, 183–192, https://doi.org/10.1016/j.soilbio.2013.12.032, 2014.
Berg, A. and Sheffield, J.: Climate Change and Drought: the Soil Moisture Perspective, Curr. Clim. Change Rep., 4, 180–191, https://doi.org/10.1007/s40641-018-0095-0, 2018.
Berg, A., Sheffield, J., and Milly, P. C. D.: Divergent surface and total soil moisture projections under global warming, Geophys. Res. Lett., 44, 236–244, https://doi.org/10.1002/2016GL071921, 2017.
Blankinship, J. C. and Schimel, J. P.: Biotic versus Abiotic Controls on Bioavailable Soil Organic Carbon, Soil Syst., 2, 10, https://doi.org/10.3390/soilsystems2010010, 2018.
Blume, H.-P., Brümmer, G. W., Fleige, H., Horn, R., Kandeler, E., Kögel-Knabner, I., Kretzschmar, R., Stahr, K., and Wilke, B.-M.: Soil Organic Matter, in: Scheffer/SchachtschabelSoil Science, edited by: Blume, H.-P., Brümmer, G. W., Fleige, H., Horn, R., Kandeler, E., Kögel-Knabner, I., Kretzschmar, R., Stahr, K., and Wilke, B.-M., Springer Berlin Heidelberg, Berlin, Heidelberg, 55–86, https://doi.org/10.1007/978-3-642-30942-7_3, 2016.
Bosatta, E. and Ågren, G. I.: Soil organic matter quality interpreted thermodynamically, Soil Biol. Biochem., 31, 1889–1891, https://doi.org/10.1016/S0038-0717(99)00105-4, 1999.
Bradford, M. A., Wieder, W. R., Bonan, G. B., Fierer, N., Raymond, P. A., and Crowther, T. W.: Managing uncertainty in soil carbon feedbacks to climate change, Nat. Clim. Change, 6, 751–758, https://doi.org/10.1038/nclimate3071, 2016.
Bradford, M. A., McCulley, R. L., Crowther, T. W., Oldfield, E. E., Wood, S. A., and Fierer, N.: Cross-biome patterns in soil microbial respiration predictable from evolutionary theory on thermal adaptation, Nat. Ecol. Evol., 3, 223–231, https://doi.org/10.1038/s41559-018-0771-4, 2019.
Braghiere, R. K., Fisher, J. B., Allen, K., Brzostek, E., Shi, M., Yang, X., Ricciuto, D. M., Fisher, R. A., Zhu, Q., and Phillips, R. P.: Modeling Global Carbon Costs of Plant Nitrogen and Phosphorus Acquisition, J. Adv. Model. Earth Syst., 14, e2022MS003204, https://doi.org/10.1029/2022MS003204, 2022.
Brunn, M., Krüger, J., and Lang, F.: Experimental drought increased the belowground sink strength towards higher topsoil organic carbon stocks in a temperate mature forest, Geoderma, 431, 116356, https://doi.org/10.1016/j.geoderma.2023.116356, 2023.
Buckeridge, K. M., Creamer, C., and Whitaker, J.: Deconstructing the microbial necromass continuum to inform soil carbon sequestration, Funct. Ecol., 36, 1396–1410, https://doi.org/10.1111/1365-2435.14014, 2022.
Carey, J. C., Tang, J., Templer, P. H., Kroeger, K. D., Crowther, T. W., Burton, A. J., Dukes, J. S., Emmett, B., Frey, S. D., Heskel, M. A., Jiang, L., Machmuller, M. B., Mohan, J., Panetta, A. M., Reich, P. B., Reinsch, S., Wang, X., Allison, S. D., Bamminger, C., Bridgham, S., Collins, S. L., de Dato, G., Eddy, W. C., Enquist, B. J., Estiarte, M., Harte, J., Henderson, A., Johnson, B. R., Larsen, K. S., Luo, Y., Marhan, S., Melillo, J. M., Penuelas, J., Pfeifer-Meister, L., Poll, C., Rastetter, E., Reinmann, A. B., Reynolds, L. L., Schmidt, I. K., Shaver, G. R., Strong, A. L., Suseela, V., and Tietema, A.: Temperature response of soil respiration largely unaltered with experimental warming, P. Natl. Acad. Sci. USA, 113, 13797–13802, https://doi.org/10.1073/pnas.1605365113, 2016.
Chen, Y., Qin, W., Zhang, Q., Wang, X., Feng, J., Han, M., Hou, Y., Zhao, H., Zhang, Z., He, J.-S., Torn, M. S., and Zhu, B.: Whole-soil warming leads to substantial soil carbon emission in an alpine grassland, Nat. Commun., 15, 4489, https://doi.org/10.1038/s41467-024-48736-w, 2024.
Conant, R. T., Ryan, M. G., Ågren, G. I., Birge, H. E., Davidson, E. A., Eliasson, P. E., Evans, S. E., Frey, S. D., Giardina, C. P., Hopkins, F. M., Hyvönen, R., Kirschbaum, M. U. F., Lavallee, J. M., Leifeld, J., Parton, W. J., Megan Steinweg, J., Wallenstein, M. D., Martin Wetterstedt, J. Å., and Bradford, M. A.: Temperature and soil organic matter decomposition rates – synthesis of current knowledge and a way forward, Glob. Change Biol., 17, 3392–3404, https://doi.org/10.1111/j.1365-2486.2011.02496.x, 2011.
Cook, B. I., Mankin, J. S., Marvel, K., Williams, A. P., Smerdon, J. E., and Anchukaitis, K. J.: Twenty-First Century Drought Projections in the CMIP6 Forcing Scenarios, Earths Future, 8, e2019EF001461, https://doi.org/10.1029/2019EF001461, 2020.
Cotrufo, M. F. and Lavallee, J. M.: Chapter One – Soil organic matter formation, persistence, and functioning: A synthesis of current understanding to inform its conservation and regeneration, in: Advances in Agronomy, vol. 172, edited by: Sparks, D. L., Academic Press, 1–66, https://doi.org/10.1016/bs.agron.2021.11.002, 2022.
Cotrufo, M. F., Wallenstein, M. D., Boot, C. M., Denef, K., and Paul, E.: The Microbial Efficiency-Matrix Stabilization (MEMS) framework integrates plant litter decomposition with soil organic matter stabilization: do labile plant inputs form stable soil organic matter?, Glob. Change Biol., 19, 988–995, https://doi.org/10.1111/gcb.12113, 2013.
Crowther, T. W., van den Hoogen, J., Wan, J., Mayes, M. A., Keiser, A. D., Mo, L., Averill, C., and Maynard, D. S.: The global soil community and its influence on biogeochemistry, Science, 365, eaav0550, https://doi.org/10.1126/science.aav0550, 2019.
Davidson, E. A.: Carbon dioxide loss from tropical soils increases on warming, Nature, 584, 198–199, https://doi.org/10.1038/d41586-020-02266-9, 2020.
Davidson, E. A. and Janssens, I. A.: Temperature sensitivity of soil carbon decomposition and feedbacks to climate change, Nature, 440, 165, https://doi.org/10.1038/nature04514, 2006.
Davidson, E. A., Janssens, I. A., and Luo, Y.: On the variability of respiration in terrestrial ecosystems: moving beyond Q10, Glob. Change Biol., 12, 154–164, https://doi.org/10.1111/j.1365-2486.2005.01065.x, 2006.
Davidson, E. A., Sudeep, S., Samantha, S. C., and Savage, K.: The Dual Arrhenius and Michaelis–Menten kinetics model for decomposition of soil organic matter at hourly to seasonal time scales, Glob. Change Biol., 18, 371–384, https://doi.org/10.1111/j.1365-2486.2011.02546.x, 2012.
Deng, L., Peng, C., Kim, D.-G., Li, J., Liu, Y., Hai, X., Liu, Q., Huang, C., Shangguan, Z., and Kuzyakov, Y.: Drought effects on soil carbon and nitrogen dynamics in global natural ecosystems, Earth-Sci. Rev., 214, 103501, https://doi.org/10.1016/j.earscirev.2020.103501, 2021.
Dwivedi, D., Riley, W. J., Torn, M. S., Spycher, N., Maggi, F., and Tang, J. Y.: Mineral properties, microbes, transport, and plant-input profiles control vertical distribution and age of soil carbon stocks, Soil Biol. Biochem., 107, 244–259, https://doi.org/10.1016/j.soilbio.2016.12.019, 2017.
Fan, K., Slater, L., Zhang, Q., Sheffield, J., Gentine, P., Sun, S., and Wu, W.: Climate warming accelerates surface soil moisture drying in the Yellow River Basin, China, J. Hydrol., 615, 128735, https://doi.org/10.1016/j.jhydrol.2022.128735, 2022.
Fan, N., Koirala, S., Reichstein, M., Thurner, M., Avitabile, V., Santoro, M., Ahrens, B., Weber, U., and Carvalhais, N.: Apparent ecosystem carbon turnover time: uncertainties and robust features, Earth Syst. Sci. Data, 12, 2517–2536, https://doi.org/10.5194/essd-12-2517-2020, 2020.
Fanin, N., Mooshammer, M., Sauvadet, M., Meng, C., Alvarez, G., Bernard, L., Bertrand, I., Blagodatskaya, E., Bon, L., Fontaine, S., Niu, S., Lashermes, G., Maxwell, T. L., Weintraub, M. N., Wingate, L., Moorhead, D., and Nottingham, A. T.: Soil enzymes in response to climate warming: Mechanisms and feedbacks, Funct. Ecol., 36, 1378–1395, https://doi.org/10.1111/1365-2435.14027, 2022.
García-García, A., Cuesta-Valero, F. J., Miralles, D. G., Mahecha, M. D., Quaas, J., Reichstein, M., Zscheischler, J., and Peng, J.: Soil heat extremes can outpace air temperature extremes, Nat. Clim. Change, 13, 1237–1241, https://doi.org/10.1038/s41558-023-01812-3, 2023.
Garnier, S., Ross, N., boB Rudis, Filipovic-Pierucci, A., Galili, T., Timelyportfolio, O'Callaghan, A., Greenwell, B., Sievert, C., Harris, D. J., Sciaini, M., and Chen, J. J.: sjmgarnier/viridis: CRAN release v0.6.3, Zenodo [software], https://doi.org/10.5281/ZENODO.4679423, 2023.
Gentsch, N., Wild, B., Mikutta, R., Čapek, P., Diáková, K., Schrumpf, M., Turner, S., Minnich, C., Schaarschmidt, F., Shibistova, O., Schnecker, J., Urich, T., Gittel, A., Šantrůčková, H., Bárta, J., Lashchinskiy, N., Fuß, R., Richter, A., and Guggenberger, G.: Temperature response of permafrost soil carbon is attenuated by mineral protection, Glob. Change Biol., 24, 3401–3415, https://doi.org/10.1111/gcb.14316, 2018.
Georgiou, K., Koven, C. D., Wieder, W. R., Hartman, M. D., Riley, W. J., Pett-Ridge, J., Bouskill, N. J., Abramoff, R. Z., Slessarev, E. W., Ahlström, A., Parton, W. J., Pellegrini, A. F. A., Pierson, D., Sulman, B. N., Zhu, Q., and Jackson, R. B.: Emergent temperature sensitivity of soil organic carbon driven by mineral associations, Nat. Geosci., 17, 205–212, https://doi.org/10.1038/s41561-024-01384-7, 2024.
Gershenson, A., Bader, N. E., and Cheng, W.: Effects of substrate availability on the temperature sensitivity of soil organic matter decomposition, Glob. Change Biol., 15, 176–183, https://doi.org/10.1111/j.1365-2486.2008.01827.x, 2009.
Ghezzehei, T. A., Sulman, B., Arnold, C. L., Bogie, N. A., and Berhe, A. A.: On the role of soil water retention characteristic on aerobic microbial respiration, Biogeosciences, 16, 1187–1209, https://doi.org/10.5194/bg-16-1187-2019, 2019.
Gillabel, J., Cebrian-Lopez, B., Six, J., and Merckx, R.: Experimental evidence for the attenuating effect of SOM protection on temperature sensitivity of SOM decomposition, Glob. Change Biol., 16, 2789–2798, https://doi.org/10.1111/j.1365-2486.2009.02132.x, 2010.
Hao, W., Xia, B., Li, J., and Xu, M.: Deep soil CO2 flux with strong temperature dependence contributes considerably to soil-atmosphere carbon flux, Ecol. Inform., 74, 101957, https://doi.org/10.1016/j.ecoinf.2022.101957, 2023.
Hartley, I. P., Hill, T. C., Chadburn, S. E., and Hugelius, G.: Temperature effects on carbon storage are controlled by soil stabilisation capacities, Nat. Commun., 12, 6713, https://doi.org/10.1038/s41467-021-27101-1, 2021.
Hicks Pries, C., Ryals, R., Zhu, B., Min, K., Cooper, A., Goldsmith, S., Pett-Ridge, J., Torn, M., and Asefaw Berhe, A.: The Deep Soil Organic Carbon Response to Global Change, Annu. Rev. Ecol. Evol. Syst., 54, 375–401, https://doi.org/10.1146/annurev-ecolsys-102320-085332, 2023.
Hicks Pries, C. E., Castanha, C., Porras, R., and Torn, M. S.: The whole-soil carbon flux in response to warming, Science, 355, 1420–1423, https://doi.org/10.1126/science.aal1319, 2017.
Hsu, H. and Dirmeyer, P. A.: Uncertainty in Projected Critical Soil Moisture Values in CMIP6 Affects the Interpretation of a More Moisture-Limited World, Earths Future, 11, e2023EF003511, https://doi.org/10.1029/2023EF003511, 2023.
Jarvis, N. J., Taylor, A., Larsbo, M., Etana, A., and Rosén, K.: Modelling the effects of bioturbation on the re-distribution of 137Cs in an undisturbed grassland soil, Eur. J. Soil Sci., 61, 24–34, https://doi.org/10.1111/j.1365-2389.2009.01209.x, 2010.
Kallenbach, C. M., Frey, S. D., and Grandy, A. S.: Direct evidence for microbial-derived soil organic matter formation and its ecophysiological controls, Nat. Commun., 7, 13630, https://doi.org/10.1038/ncomms13630, 2016.
Kirschbaum, M. U. F.: The temperature dependence of organic-matter decomposition–still a topic of debate, Soil Biol. Biochem., 38, 2510–2518, https://doi.org/10.1016/j.soilbio.2006.01.030, 2006.
Koven, C. D., Hugelius, G., Lawrence, D. M., and Wieder, W. R.: Higher climatological temperature sensitivity of soil carbon in cold than warm climates, Nat. Clim. Change, 7, 817–822, https://doi.org/10.1038/nclimate3421, 2017.
Le Noë, J., Manzoni, S., Abramoff, R., Bölscher, T., Bruni, E., Cardinael, R., Ciais, P., Chenu, C., Clivot, H., Derrien, D., Ferchaud, F., Garnier, P., Goll, D., Lashermes, G., Martin, M., Rasse, D., Rees, F., Sainte-Marie, J., Salmon, E., Schiedung, M., Schimel, J., Wieder, W., Abiven, S., Barré, P., Cécillon, L., and Guenet, B.: Soil organic carbon models need independent time-series validation for reliable prediction, Commun. Earth Environ., 4, 1–8, https://doi.org/10.1038/s43247-023-00830-5, 2023.
Li, J., Pei, J., Pendall, E., Reich, P. B., Noh, N. J., Li, B., Fang, C., and Nie, M.: Rising Temperature May Trigger Deep Soil Carbon Loss Across Forest Ecosystems, Adv. Sci., 7, 2001242, https://doi.org/10.1002/advs.202001242, 2020.
Liang, C., Schimel, J. P., and Jastrow, J. D.: The importance of anabolism in microbial control over soil carbon storage, Nat. Microbiol., 2, 1–6, https://doi.org/10.1038/nmicrobiol.2017.105, 2017.
Liang, J., Wang, G., Singh, S., Jagadamma, S., Gu, L., Schadt, C. W., Wood, J. D., Hanson, P. J., and Mayes, M. A.: Intensified Soil Moisture Extremes Decrease Soil Organic Carbon Decomposition: A Mechanistic Modeling Analysis, J. Geophys. Res.-Biogeo., 126, e2021JG006392, https://doi.org/10.1029/2021JG006392, 2021.
Liu, C., Tian, H., Gu, X., Li, N., Zhao, X., Lei, M., Alharbi, H., Megharaj, M., He, W., and Kuzyakov, Y.: Catalytic efficiency of soil enzymes explains temperature sensitivity: Insights from physiological theory, Sci. Total Environ., 822, 153365, https://doi.org/10.1016/j.scitotenv.2022.153365, 2022.
Liu, Y., Tian, J., He, N., and Tiemann, L.: Global microbial necromass contribution to soil organic matter, https://doi.org/10.21203/rs.3.rs-473688/v1, preprint, 2021.
Manzoni, S., Schaeffer, S. M., Katul, G., Porporato, A., and Schimel, J. P.: A theoretical analysis of microbial eco-physiological and diffusion limitations to carbon cycling in drying soils, Soil Biol. Biochem., 73, 69–83, https://doi.org/10.1016/j.soilbio.2014.02.008, 2014.
Meier, I. C. and Leuschner, C.: Variation of soil and biomass carbon pools in beech forests across a precipitation gradient, Glob. Change Biol., 16, 1035–1045, https://doi.org/10.1111/j.1365-2486.2009.02074.x, 2010.
Michaelis, L. and Menten, M. L.: Michaelis: Die kinetik der invertinwirkung, Biochem. Z., 49, 33–369, 1913.
Moinet, G. Y. K., Moinet, M., Hunt, J. E., Rumpel, C., Chabbi, A., and Millard, P.: Temperature sensitivity of decomposition decreases with increasing soil organic matter stability, Sci. Total Env., 704, 135460, https://doi.org/10.1016/j.scitotenv.2019.135460, 2020.
Moyano, F. E., Manzoni, S., and Chenu, C.: Responses of soil heterotrophic respiration to moisture availability: An exploration of processes and models, Soil Biol. Biochem., 59, 72–85, https://doi.org/10.1016/j.soilbio.2013.01.002, 2013.
Moyano, F. E., Vasilyeva, N., and Menichetti, L.: Diffusion limitations and Michaelis–Menten kinetics as drivers of combined temperature and moisture effects on carbon fluxes of mineral soils, Biogeosciences, 15, 5031–5045, https://doi.org/10.5194/bg-15-5031-2018, 2018.
Nottingham, A. T., Turner, B. L., Whitaker, J., Ostle, N., Bardgett, R. D., McNamara, N. P., Salinas, N., and Meir, P.: Temperature sensitivity of soil enzymes along an elevation gradient in the Peruvian Andes, Biogeochemistry, 127, 217–230, https://doi.org/10.1007/s10533-015-0176-2, 2016.
Ofiti, N. O. E., Zosso, C. U., Soong, J. L., Solly, E. F., Torn, M. S., Wiesenberg, G. L. B., and Schmidt, M. W. I.: Warming promotes loss of subsoil carbon through accelerated degradation of plant-derived organic matter, Soil Biol. Biochem., 156, 108185, https://doi.org/10.1016/j.soilbio.2021.108185, 2021.
Pallandt, M., Ahrens, B., Koirala, S., Lange, H., Reichstein, M., Schrumpf, M., and Zaehle, S.: Vertically Divergent Responses of SOC Decomposition to Soil Moisture in a Changing Climate, J. Geophys. Res.-Biogeo., 127, e2021JG006684, https://doi.org/10.1029/2021JG006684, 2022.
Parton, W. J., Scurlock, J. M. O., Ojima, D. S., Gilmanov, T. G., Scholes, R. J., Schimel, D. S., Kirchner, T., Menaut, J.-C., Seastedt, T., Garcia Moya, E., Kamnalrut, A., and Kinyamario, J. I.: Observations and modeling of biomass and soil organic matter dynamics for the grassland biome worldwide, Glob. Change Biol., 7, 785–809, https://doi.org/10.1029/93GB02042, 1993.
Posit team. RStudio: Integrated Development Environment for R, version 2024.04.01. Posit Software, PBC, Boston, MA [software], https://docs.posit.co/previous-versions/rstudio.html#section-6 (last access: 25 March 2025), 2024.
Qin, S., Chen, L., Fang, K., Zhang, Q., Wang, J., Liu, F., Yu, J., and Yang, Y.: Temperature sensitivity of SOM decomposition governed by aggregate protection and microbial communities, Sci. Adv., 5, eaau1218, https://doi.org/10.1126/sciadv.aau1218, 2019.
R Core Team: R: A language and Environment for Statistical Computing, R Foundation for Statistical Computing, Vienna, Austria, version 4.3.1, [software], https://cran.r-project.org/bin/windows/base/old/4.3.1/ (last access: 21 March 2025), 2023.
Reichstein, M., Subke, J.-A., Angeli, A. C., and Tenhunen, J. D.: Does the temperature sensitivity of decomposition of soil organic matter depend upon water content, soil horizon, or incubation time?, Glob. Change Biol., 11, 1754–1767, https://doi.org/10.1111/j.1365-2486.2005.001010.x, 2005.
Saifuddin, M., Abramoff, R. Z., Davidson, E. A., Dietze, M. C., and Finzi, A. C.: Identifying Data Needed to Reduce Parameter Uncertainty in a Coupled Microbial Soil C and N Decomposition Model, J. Geophys. Res.-Biogeo., 126, e2021JG006593, https://doi.org/10.1029/2021JG006593, 2021.
Schimel, J. P.: Life in Dry Soils: Effects of Drought on Soil Microbial Communities and Processes, Annu. Rev. Ecol. Evol. Syst., 49, 409–432, https://doi.org/10.1146/annurev-ecolsys-110617-062614, 2018.
Schmidt, M. W. I., Torn, M. S., Abiven, S., Dittmar, T., Guggenberger, G., Janssens, I. A., Kleber, M., Kögel-Knabner, I., Lehmann, J., Manning, D. A. C., Nannipieri, P., Rasse, D. P., Weiner, S., and Trumbore, S. E.: Persistence of soil organic matter as an ecosystem property, Nature, 478, 49, https://doi.org/10.1038/nature10386, 2011.
Sierra, C. A., Trumbore, S. E., Davidson, E. A., Vicca, S., and Janssens, I.: Sensitivity of decomposition rates of soil organic matter with respect to simultaneous changes in temperature and moisture, J. Adv. Model. Earth Syst., 7, 335–356, https://doi.org/10.1002/2014MS000358, 2015.
Skopp, J., Jawson, M. D., and Doran, J. W.: Steady-state aerobic microbial activity as a function of soil water content, Soil Sci. Soc. Am. J., 54, 1619–1625, https://doi.org/10.2136/sssaj1990.03615995005400060018x, 1990.
Sokol, N. W., Whalen, E. D., Jilling, A., Kallenbach, C., Pett-Ridge, J., and Georgiou, K.: Global distribution, formation and fate of mineral-associated soil organic matter under a changing climate: A trait-based perspective, Funct. Ecol., 36, 1411–1429, https://doi.org/10.1111/1365-2435.14040, 2022.
Soong, J. L., Phillips, C. L., Ledna, C., Koven, C. D., and Torn, M. S.: CMIP5 Models Predict Rapid and Deep Soil Warming Over the 21st Century, J. Geophys. Res.-Biogeo., 125, e2019JG005266, https://doi.org/10.1029/2019JG005266, 2020.
Soong, J. L., Castanha, C., Hicks Pries, C. E., Ofiti, N., Porras, R. C., Riley, W. J., Schmidt, M. W. I., and Torn, M. S.: Five years of whole-soil warming led to loss of subsoil carbon stocks and increased CO2 efflux, Sci. Adv., 7, eabd1343, https://doi.org/10.1126/sciadv.abd1343, 2021.
Stone, M. M., Weiss, M. S., Goodale, C. L., Adams, M. B., Fernandez, I. J., German, D. P., and Allison, S. D.: Temperature sensitivity of soil enzyme kinetics under -fertilization in two temperate forests, Glob. Change Biol., 18, 1173–1184, https://doi.org/10.1111/j.1365-2486.2011.02545.x, 2012.
Subke, J.-A. and Bahn, M.: On the “temperature sensitivity” of soil respiration: Can we use the immeasurable to predict the unknown?, Soil Biol. Biochem., 42, 1653–1656, https://doi.org/10.1016/j.soilbio.2010.05.026, 2010.
Sulman, B. N., Phillips, R. P., Oishi, A. C., Shevliakova, E., and Pacala, S. W.: Microbe-driven turnover offsets mineral-mediated storage of soil carbon under elevated CO2, Nat. Clim. Change, 4, 1099, https://doi.org/10.1038/nclimate2436, 2014.
Sulman, B. N., Moore, J. A. M., Abramoff, R., Averill, C., Kivlin, S., Georgiou, K., Sridhar, B., Hartman, M. D., Wang, G., Wieder, W. R., Bradford, M. A., Luo, Y., Mayes, M. A., Morrison, E., Riley, W. J., Salazar, A., Schimel, J. P., Tang, J., and Classen, A. T.: Multiple models and experiments underscore large uncertainty in soil carbon dynamics, Biogeochemistry, 141, 109–123, https://doi.org/10.1007/s10533-018-0509-z, 2018.
Tang, J. and Riley, W. J.: Weaker soil carbon–climate feedbacks resulting from microbial and abiotic interactions, Nat. Clim. Change, 5, 56–60, https://doi.org/10.1038/nclimate2438, 2015.
Tang, J. and Riley, W. J.: Competitor and substrate sizes and diffusion together define enzymatic depolymerization and microbial substrate uptake rates, Soil Biol. Biochem., 139, 107624, https://doi.org/10.1016/j.soilbio.2019.107624, 2019.
Tang, J. and Riley, W. J.: A chemical kinetics theory for interpreting the non-monotonic temperature dependence of enzymatic reactions, Biogeosciences, 21, 1061–1070, https://doi.org/10.5194/bg-21-1061-2024, 2024.
Tang, J., Bradford, M. A., Carey, J., Crowther, T. W., Machmuller, M. B., Mohan, J. E., and Todd-Brown, K.: Chapter 8 – Temperature sensitivity of soil carbon, in: Ecosystem Consequences of Soil Warming, edited by: Mohan, J. E., Academic Press, 175–208, https://doi.org/10.1016/B978-0-12-813493-1.00009-0, 2019.
Tang, J. Y.: On the relationships between the Michaelis–Menten kinetics, reverse Michaelis–Menten kinetics, equilibrium chemistry approximation kinetics, and quadratic kinetics, Geosci. Model Dev., 8, 3823–3835, https://doi.org/10.5194/gmd-8-3823-2015, 2015.
Thum, T., Caldararu, S., Engel, J., Kern, M., Pallandt, M., Schnur, R., Yu, L., and Zaehle, S.: A new model of the coupled carbon, nitrogen, and phosphorus cycles in the terrestrial biosphere (QUINCY v1.0; revision 1996), Geosci. Model Dev., 12, 4781–4802, https://doi.org/10.5194/gmd-12-4781-2019, 2019 (code available at: https://www.gnu.org/licenses/gpl-3.0.en.html, last access: 27 January 2025).
Thurner, M. A., Caldararu, S., Engel, J., Rammig, A., and Zaehle, S.: Modelled forest ecosystem carbon–nitrogen dynamics with integrated mycorrhizal processes under elevated CO2, Biogeosciences, 21, 1391–1410, https://doi.org/10.5194/bg-21-1391-2024, 2024.
Todd-Brown, K., Zheng, B., and Crowther, T. W.: Field-warmed soil carbon changes imply high 21st-century modeling uncertainty, Biogeosciences, 15, 3659–3671, https://doi.org/10.5194/bg-15-3659-2018, 2018.
Todd-Brown, K. E. O., Randerson, J. T., Hopkins, F., Arora, V., Hajima, T., Jones, C., Shevliakova, E., Tjiputra, J., Volodin, E., Wu, T., Zhang, Q., and Allison, S. D.: Changes in soil organic carbon storage predicted by Earth system models during the 21st century, Biogeosciences, 11, 2341–2356, https://doi.org/10.5194/bg-11-2341-2014, 2014.
Varney, R. M., Chadburn, S. E., Friedlingstein, P., Burke, E. J., Koven, C. D., Hugelius, G., and Cox, P. M.: A spatial emergent constraint on the sensitivity of soil carbon turnover to global warming, Nat. Commun., 11, 5544, https://doi.org/10.1038/s41467-020-19208-8, 2020.
Walker, T. W. N., Kaiser, C., Strasser, F., Herbold, C. W., Leblans, N. I. W., Woebken, D., Janssens, I. A., Sigurdsson, B. D., and Richter, A.: Microbial temperature sensitivity and biomass change explain soil carbon loss with warming, Nat. Clim. Change, 8, 885–889, https://doi.org/10.1038/s41558-018-0259-x, 2018.
Wang, B., An, S., Liang, C., Liu, Y., and Kuzyakov, Y.: Microbial necromass as the source of soil organic carbon in global ecosystems, Soil Biol. Biochem., 162, 108422, https://doi.org/10.1016/j.soilbio.2021.108422, 2021.
Wang, G., Post, W. M., Mayes, M. A., Frerichs, J. T., and Sindhu, J.: Parameter estimation for models of ligninolytic and cellulolytic enzyme kinetics, Soil Biol. Biochem., 48, 28–38, https://doi.org/10.1016/j.soilbio.2012.01.011, 2012.
Wang, G., Post, W. M., and Mayes, M. A.: Development of microbial-enzyme-mediated decomposition model parameters through steady-state and dynamic analyses, Ecol. Appl., 23, 255–272, https://doi.org/10.1890/12-0681.1, 2013.
Wang, G., Jagadamma, S., Mayes, M. A., Schadt, C. W., Megan Steinweg, J., Gu, L., and Post, W. M.: Microbial dormancy improves development and experimental validation of ecosystem model, ISME J., 9, 226–237, https://doi.org/10.1038/ismej.2014.120, 2015.
Wang, G., Huang, W., Zhou, G., Mayes, M. A., and Zhou, J.: Modeling the processes of soil moisture in regulating microbial and carbon-nitrogen cycling, J. Hydrol., 585, 124777, https://doi.org/10.1016/j.jhydrol.2020.124777, 2020.
Wang, M., Guo, X., Zhang, S., Xiao, L., Mishra, U., Yang, Y., Zhu, B., Wang, G., Mao, X., Qian, T., Jiang, T., Shi, Z., and Luo, Z.: Global soil profiles indicate depth-dependent soil carbon losses under a warmer climate, Nat. Commun., 13, 5514, https://doi.org/10.1038/s41467-022-33278-w, 2022a.
Wang, Y., Mao, J., Hoffman, F. M., Bonfils, C. J. W., Douville, H., Jin, M., Thornton, P. E., Ricciuto, D. M., Shi, X., Chen, H., Wullschleger, S. D., Piao, S., and Dai, Y.: Quantification of human contribution to soil moisture-based terrestrial aridity, Nat. Commun., 13, 6848, https://doi.org/10.1038/s41467-022-34071-5, 2022b.
Wickham, H.: ggplot2, Springer International Publishing [software], Cham, https://doi.org/10.1007/978-3-319-24277-4, 2016.
Wickham, H., Averick, M., Bryan, J., Chang, W., McGowan, L., François, R., Grolemund, G., Hayes, A., Henry, L., Hester, J., Kuhn, M., Pedersen, T., Miller, E., Bache, S., Müller, K., Ooms, J., Robinson, D., Seidel, D., Spinu, V., Takahashi, K., Vaughan, D., Wilke, C., Woo, K., and Yutani, H.: Welcome to the Tidyverse, J. Open Source Softw. [software], 4, 1686, https://doi.org/10.21105/joss.01686, 2019.
Wieder, W. R., Grandy, A. S., Kallenbach, C. M., and Bonan, G. B.: Integrating microbial physiology and physio-chemical principles in soils with the MIcrobial-MIneral Carbon Stabilization (MIMICS) model, Biogeosciences, 11, 3899–3917, https://doi.org/10.5194/bg-11-3899-2014, 2014.
Wieder, W. R., Allison, S. D., Davidson, E. A., Georgiou, K., Hararuk, O., He, Y., Hopkins, F., Luo, Y., Smith, M. J., Sulman, B., Todd-Brown, K., Wang, Y.-P., Xia, J., and Xu, X.: Explicitly representing soil microbial processes in Earth system models, Glob. Change Biol., 29, 1782–1800, https://doi.org/10.1002/2015GB005188, 2015.
Wieder, W. R., Hartman, M. D., Sulman, B. N., Wang, Y.-P., Koven, C. D., and Bonan, G. B.: Carbon cycle confidence and uncertainty: Exploring variation among soil biogeochemical models, Glob. Change Biol., 24, 1563–1579, https://doi.org/10.1111/gcb.13979, 2018.
Wu, Y., Zhou, H., Sun, W., Zhao, Q., Liang, M., Chen, W., Guo, Z., Jiang, Y., Jiang, Y., Liu, G., and Xue, S.: Temperature sensitivity of soil enzyme kinetics under N and P fertilization in an alpine grassland, China, Sci. Total Environ., 838, 156042, https://doi.org/10.1016/j.scitotenv.2022.156042, 2022.
Xiao, K.-Q., Zhao, Y., Liang, C., Zhao, M., Moore, O. W., Otero-Fariña, A., Zhu, Y.-G., Johnson, K., and Peacock, C. L.: Introducing the soil mineral carbon pump, Nat. Rev. Earth Environ., 4, 135–136, https://doi.org/10.1038/s43017-023-00396-y, 2023.
Yan, Y., Wang, J., Tian, D., Luo, Y., Xue, X., Peng, F., He, J.-S., Liu, L., Jiang, L., Wang, X., Wang, Y., Song, L., and Niu, S.: Sustained increases in soil respiration accompany increased carbon input under long-term warming across global grasslands, Geoderma, 428, 116157, https://doi.org/10.1016/j.geoderma.2022.116157, 2022.
Yan, Z., Bond-Lamberty, B., Todd-Brown, K. E., Bailey, V. L., Li, S., Liu, C., and Liu, C.: A moisture function of soil heterotrophic respiration that incorporates microscale processes, Nat. Commun., 9, 2562, https://doi.org/10.1038/s41467-018-04971-6, 2018.
Yu, L., Ahrens, B., Wutzler, T., Schrumpf, M., and Zaehle, S.: Jena Soil Model (JSM v1.0; revision 1934): a microbial soil organic carbon model integrated with nitrogen and phosphorus processes, Geosci. Model Dev., 13, 783–803, https://doi.org/10.5194/gmd-13-783-2020, 2020 (code available at: https://git.bgc-jena.mpg.de/quincy/quincy-model-releases, last access: 21 March 2025).
Zhang, X., Xie, Z., Ma, Z., Barron-Gafford, G. A., Scott, R. L., and Niu, G.-Y.: A Microbial-Explicit Soil Organic Carbon Decomposition Model (MESDM): Development and Testing at a Semiarid Grassland Site, J. Adv. Model. Earth Syst., 14, e2021MS002485, https://doi.org/10.1029/2021MS002485, 2022.