the Creative Commons Attribution 4.0 License.
the Creative Commons Attribution 4.0 License.
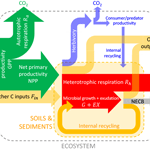
Reviews and syntheses: Carbon use efficiency from organisms to ecosystems – definitions, theories, and empirical evidence
Stefano Manzoni
Petr Čapek
Philipp Porada
Martin Thurner
Mattias Winterdahl
Christian Beer
Volker Brüchert
Jan Frouz
Anke M. Herrmann
Björn D. Lindahl
Steve W. Lyon
Hana Šantrůčková
Giulia Vico
Danielle Way
The cycling of carbon (C) between the Earth surface and the atmosphere is controlled by biological and abiotic processes that regulate C storage in biogeochemical compartments and release to the atmosphere. This partitioning is quantified using various forms of C-use efficiency (CUE) – the ratio of C remaining in a system to C entering that system. Biological CUE is the fraction of C taken up allocated to biosynthesis. In soils and sediments, C storage depends also on abiotic processes, so the term C-storage efficiency (CSE) can be used. Here we first review and reconcile CUE and CSE definitions proposed for autotrophic and heterotrophic organisms and communities, food webs, whole ecosystems and watersheds, and soils and sediments using a common mathematical framework. Second, we identify general CUE patterns; for example, the actual CUE increases with improving growth conditions, and apparent CUE decreases with increasing turnover. We then synthesize > 5000 CUE estimates showing that CUE decreases with increasing biological and ecological organization – from unicellular to multicellular organisms and from individuals to ecosystems. We conclude that CUE is an emergent property of coupled biological–abiotic systems, and it should be regarded as a flexible and scale-dependent index of the capacity of a given system to effectively retain C.
- Article
(1312 KB) - Full-text XML
-
Supplement
(345 KB) - BibTeX
- EndNote
Carbon cycling is driven by biological, physical, and chemical processes – vegetation and phytoplankton take up CO2 from the atmosphere and convert it to biomass, decomposers and animals convert organic C to biomass and release it as CO2, and physico-chemical processes redistribute and store C. Many of these processes involve the conversion of C from various sources into biological products and the efficiency of this biological conversion is generally referred to as C-use efficiency (CUE). Low CUE values imply that little C is converted to biomass and biological products relative to the amount consumed. As a result, less C is retained in the organism and more is released as CO2 or other forms of C, in comparison to circumstances under which CUE is high and the organism retains more C. In other words, from this perspective, low CUE is indicative of a more open biological C cycle. Therefore, understanding the degree of variation in CUE – especially along gradients of environmental conditions – is key for quantifying how much C is retained in biomass and potentially in an ecosystem in the long term (Allison et al., 2010; Manzoni et al., 2012; Hessen et al., 2004; Jiao et al., 2014; Sterner and Elser, 2002). However, the connection between CUE and long-term C storage is complex and mediated by multiple biological, ecological, and physical factors.
For biological systems (organs, individual organisms, or even entire communities), CUE is defined as the ratio between the amount of C allocated to biosynthesis (new biomass and biological products, including exudates) and the amount of C taken up. While the term CUE was proposed in the mid-1990s in the context of plant C balances (Gifford, 1995), other terms – e.g. “growth yield” – referring to the efficiency of substrate conversion into biomass had been in use since the early 1900s (Monod, 1949). Now, efficiency definitions are proliferating across many disciplines in biology, ecology, and Earth sciences. While some of these definitions are comparable (and all are deceptively simple), subtle differences often emerge, partly due to conceptual and methodological advances that allow quantification of previously ignored C exchanges. These differences make interpretation of results difficult and complicate cross-disciplinary comparisons.
The main difficulty is to unambiguously define what represents growth and release of extracellular compounds or C storage and reconcile conceptual definitions with empirical estimates (Geyer et al., 2016; Chapin et al., 2006; Clark et al., 2001). In fact, CUE is a property of the biological system considered under the specific conditions it experiences, and the term synthesizes various biological processes occurring across a range of spatial and temporal scales in a single variable (Geyer et al., 2016). Because the proportion of growth vs. maintenance respiration, the growth rate, the synthesis and release of products, and the availability of C all vary in time in any organism, CUE is also expected to change. Changes in environmental conditions that favour growth over respiration will shift the balance of C allocation towards biosynthesis (or C storage at the ecosystem level), thus increasing CUE (Manzoni et al., 2017; Öquist et al., 2017; Vicca et al., 2012). Instantaneous responses to an environmental change may also differ from long-term responses involving acclimation and adaptation to the new conditions – both of which can potentially affect C allocation to different metabolic processes and hence CUE (Allison, 2014). In addition to responses to environmental change, metabolic processes also differ across levels of biological organization, leading to decreasing values of CUE as organisms become more complex and require more energetically expensive structures (DeLong et al., 2010).
While the aforementioned mechanisms can be identified for individual organisms or uniform populations, natural plant, microbial, and animal communities are composed of a number of different organisms whose metabolism may respond differently to environmental drivers. In addition, various interactions among organisms in an ecosystem lead to emergent patterns that are different from the sum of individual contributions. Therefore, by integrating the contribution of individual organisms with a range of different CUE values, patterns in community-level CUE may be different from those expected based on organism-level CUE (del Giorgio and Cole, 1998; Ettema and Wardle, 2002; Geyer et al., 2016). For example, seedlings of conifer species can have a whole-plant CUE of around 0.7 (Wang et al., 2015), but conifer forests encompassing a range of tree ages and species exhibit a CUE (defined as net primary productivity, NPP, divided by gross primary productivity, GPP) of around 0.45 (DeLucia et al., 2007; Gifford, 2003). Similarly, ecosystem-level CUE (defined as net ecosystem productivity, NEP, divided by GPP) emerges from linkages between plants and decomposers and the way both communities process and exchange C (Bradford and Crowther, 2013; Sinsabaugh et al., 2017). Because at the ecosystem level CO2 is released by both autotrophs and heterotrophs, ecosystem CUE values are lower than those of plant communities.
While variability in biological and ecological processes affects CUE at organism to ecosystem levels, the efficiency of long-term C storage in ecosystems depends on how much C enters physically protected or chemically recalcitrant compartments or is removed from the system by abiotic transport processes. The more C is removed via leaching and lateral transfer, for example (Chapin et al., 2006; Cole et al., 2007), the lower the C-storage efficiency (CSE) of an ecosystem. The term CSE is used here instead of CUE to emphasize that C storage in soils and sediments also depends on abiotic processes that do not “use” C for their fitness in a manner similar to organisms, or on incomplete C turnover due to hampered heterotrophic activity, e.g. in the uppermost organic layers of forest soils or in peatlands. Moreover, as C is recycled in the soil or sediment system and progressively more C is lost, C accumulation becomes more dependent on physico-chemical protection mechanisms that reduce accessibility of C to decomposers and abiotic removal processes (Schmidt et al., 2011; Canfield, 1994; Mendonça et al., 2017; Stewart et al., 2007).
From these examples (and others that will be presented in the following), it is clear that CUE (or CSE) should be regarded as a flexible quantity that emerges from the underlying biological and abiotic processes at various spatial and temporal scales. Understanding to what degree CUE is stable or variable across scales is important for correct partitioning of C in biogeochemical models, in which these efficiencies are sometimes assumed to be constant (e.g. microbial CUE), and in other cases the result of modelled C fluxes. Measured CUE and CSE thus offer an opportunity for testing the capacity of models to describe how C is partitioned among different pathways.
Table 1Definition of symbols and acronyms.
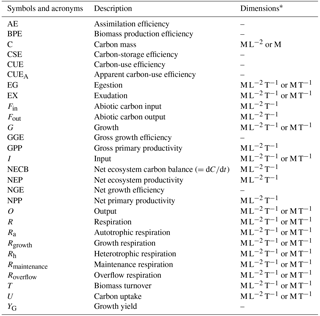
* M: mass; L: length; T: time; –: non-dimensional quantity.
With this aim in mind, we synthesize the numerous definitions of CUE and CSE currently employed across levels of biological and ecological organization and spatial–temporal scales and develop a coherent mathematical framework for these different definitions. Next, we analyse how these efficiencies vary across scales and levels of organization and how physico-chemical processes that lead to stabilization or incomplete turnover of organic matter become relevant to evaluate C retention at the whole-ecosystem level. While previous syntheses have investigated drivers of CUE in specific systems (Canfield, 1994; del Giorgio and Cole, 1998; DeLucia et al., 2007; Manzoni et al., 2017; Sinsabaugh et al., 2015; Sterner and Elser, 2002), we focus on scale dependencies of CUE and CSE across systems and discuss the limitations that arise in the interpretation of efficiency values due to these scaling issues. Finally, we discuss the relevance of observed trends in relation to our understanding of the C cycle for informing ecosystem model development and for overcoming disciplinary boundaries that have led to numerous conceptually similar CUE definitions.
2.1 General carbon balance equations and definitions of C-use and C-storage efficiencies
In this section, general equations are presented to define CUE and CSE (CUE and CSE, respectively). We use the term CUE for efficiencies that are relevant for biological systems (from individuals to communities), in which C is actually used for functions related to the fitness of organisms, either as individuals or in communities. In contrast, systems in which both biological and abiotic storage processes occur do not literally use C, but store it and thus the term CSE will be used instead of CUE. The term “storage” is used instead of “sequestration” because we do not focus on the long-term stabilization of C but only on the efficiency of C retention in relation to C inputs. All symbols are defined in Table 1 and all quantities are expressed in carbon units. Table 2 summarizes the different definitions of CUE proposed in the literature, and Table 3 relates C exchange rates and fluxes used in the theory section to processes specific to the system under consideration.
Table 3Processes associated with the terms of Eqs. (5) and (10) at different levels or organization (indicated as subscripts).
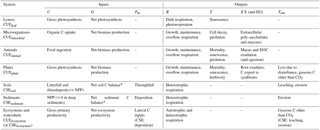
* When Fin and Fout are negligible.
For a generic C compartment representing an individual organism or a whole ecosystem with clearly defined boundaries, a general mass balance equation can be written in the form
where C is the amount of C in the compartment, and the balance of inputs I and outputs O determines the rate of change of C. Inputs and outputs typically depend on external environmental factors and internal state variables and are defined differently for organisms and ecosystems, as discussed in the following. In this general equation, changes in stored C can be linked to the rate of C input. This linkage represents a simple definition for an apparent CUE (CUEA) – the ratio of C remaining in the system (i.e. ) to C added to the system (I). The term CUEA is used for convenience, noting that CSEA should be used for systems involving abiotic C exchanges. This is an apparent efficiency because it is calculated solely from C input and change in storage, without any consideration of the underlying processes that determine the C outputs. As will become clear, this pragmatic definition is insufficient for CUE to have a biological meaning. Based on this definition, Eq. (1) can be recast as
where CUEA describes the fraction of the input that ends up in the organic carbon pool C. Expanding the definition of CUEA using the mass balance Eq. (1), CUEA can also be defined in terms of input and output rates,
These two equalities allow the estimation of CUEA from measured changes in C pool size and C exchange rates. Hence, the apparent CUE is a dynamic quantity that depends on the ratio of output to input rates or the ratio of change in storage and input rate. For systems in which inputs are larger than outputs, . In contrast, when outputs are larger than inputs, the system loses mass and CUEA<0. For biological systems in which C represents the biomass of the organism, CUEA represents the fraction of C uptake contributing to a biomass increase. Similar considerations hold for whole ecosystems, and CSEA is accordingly defined as the fraction of C inputs via photosynthesis and physical transport contributing to C storage in vegetation and soils, or sediments (Alin and Johnson, 2007; Canfield, 1994; Stewart et al., 2007).
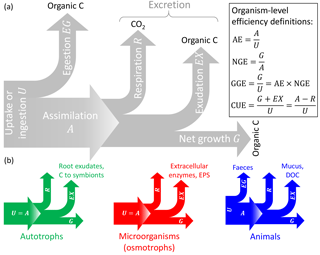
Figure 1General conceptual summary of C exchanges of individual organisms (or populations). (a) General terminology and C-based efficiency definitions (Sterner and Elser, 2002). (b) C exchanges of autotrophs, microorganisms feeding through the cell membranes (osmotrophs), and animals; note that assimilation is equal to uptake (or ingestion) in autotrophs and osmotrophs that lack guts so that egestion cannot occur. The type of excretion product is also indicated (EPS: extracellular polysaccharides). Colour codes for the different organisms are also used in other figures.
2.2 Carbon balance and efficiency equations for biological systems
Equation (3) is not particularly useful to describe how effectively an organism uses C because it does not provide much mechanistic insight into the processes leading to the allocation of C to storage or output rates. However, Eq. (3) is used to estimate apparent CUE in many practical applications in which input, change in storage, and/or output are measured – including the estimation of CUE for biological systems. If the observational setup is such that changes in storage and output rates can be unambiguously attributed to certain processes (e.g. gross growth and respiration), then the apparent CUE estimated from Eq. (3) is also a useful measure of the CUE of that organism. However, in general, a more accurate description of the organism C balance is required to define a biologically meaningful CUE, leading to numerous definitions of CUE (Table 2).
Let us now focus on C compartments representing the biomass of an individual organism or of a community. Here, “organism” indicates any living entity, ranging from unicellular to multicellular, and including both autotrophs and heterotrophs; regardless of their physiology and size, they are all treated as a C compartment with a well-defined boundary that allows the definition of inputs and outputs. In Table 3, specific processes for each type of organism are matched to the general C balance terms used below. In this context, the input I represents C uptake or ingestion (U), and the output O represents the sum of egestion (EG), respiration (R), exudation (EX), and turnover (T) (Sterner and Elser, 2002); i.e. the output rate is expressed as (Fig. 1). Distinguishing among these processes is motivated by the different timescales for respiration (seconds to hours) and turnover (minutes to years) processes. EG includes C that passes through the guts without being assimilated (faeces); for plants and microorganisms, EG=0. The EX term may include excretion of C compounds such as extracellular enzymes and polysaccharides, secondary metabolites in microbial communities (Manzoni et al., 2012; Azam and Malfatti, 2007), dissolved organic carbon (DOC) and mucus in animals and phytoplankton (Darchambeau et al., 2003; Azam and Malfatti, 2007), and rhizodeposits (Hutsch et al., 2002) or C export to symbionts (Hobbie, 2006; Ekblad et al., 2013) in plants. Using these definitions, the C balance Eq. (1) can be rewritten in more biologically meaningful terms for an individual organism or community as (Fig. 1)
where U is the uptake rate, U−EG is the assimilation rate (i.e. A in Fig. 1), and G is the net growth rate. We now define CUE at the organism level as the ratio between the rate of production of biomass and products (G+EX) and the rate of C uptake (U),
As a result, the mass balance Eq. (4) can be rewritten as
With this definition CUE represents the fraction of C taken up that is allocated to biosynthesis (biomass and products that are eventually exuded), but excluding respired and egested C, which do not contribute to biosynthesis. Including exudates such as enzymes and polymeric compounds in the CUE definition may be motivated by the clear fitness advantage these products have for the organism. Moreover, C-storage compounds and osmolytes are also regarded as biomass, as they would be measured as cellular material.
Other measures of C conversion efficiency have been proposed (Fig. 1) (Sterner and Elser, 2002): (i) assimilation efficiency ( assimilation/uptake), (ii) net growth efficiency ( net growth/assimilation), and (iii) gross growth efficiency ( net growth/uptake; see the last equality on the right-hand side of Eq. 6). The GGE can be regarded as a biomass yield or production efficiency, as it considers respired, egested, and exuded C as lost from the organism (Payne, 1970; Manzoni et al., 2012; Campioli et al., 2015), which is different from CUE, which includes exuded C as a product of the C conversion.
The CUE definition in Eq. (5) is consistent with previous work on plant C budgets (Thornley and Cannell, 2000), but it differs from definitions often used for soil microorganisms in which only biomass synthesis is considered and CUE is equal to GGE (Manzoni et al., 2012; Geyer et al., 2016) (Table S3). It is thus important to emphasize that CUE as defined in Eq. (5) is in general higher than GGE because . The difference between GGE and CUE is relevant when EX is large, as in the case of organic C exchanges between roots and plant symbionts (Hobbie, 2006; Ekblad et al., 2013), or in anaerobic metabolism (Šantrůčková et al., 2004). In the oceans, 10 %–30 % of microbial production is released as dissolved organic C, but this figure also includes dissolved C from microbial turnover (Benner and Herndl, 2011; Jiao et al., 2014). For soil microbial communities, the extent of the extracellular enzyme and polysaccharide synthesis is unknown but presumably small compared to the other rates involved, at least in aerobic soils in which CUE ≈ GGE (Frey et al., 2001; Šantrůčková et al., 2004). Therefore, making the distinction between GGE and CUE is less important in these systems (for further discussions, see Geyer et al., 2016).
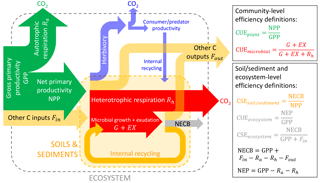
Figure 2Conceptual scheme of C fluxes in a generic ecosystem, following the terminology by Chapin et al. (2006), but adding the herbivory pathway. The ratio between the flux of C retained in a given subsystem (e.g. vegetation in green, microbial biomass in red, soil and sediments in yellow, whole ecosystem in grey) to the C flux taken up represents the C-use or C-storage efficiencies (CUE or CSE) of that subsystem. The net ecosystem C balance is denoted by NECB and the net ecosystem productivity by NEP (not shown). C transport processes and C exchanges in forms other than CO2 are denoted “other C inputs” or “other C outputs”. Colour codes for the different organisms and subsystems are also used in other figures.
Respiration in Eq. (5) can be further broken down into growth (Rgrowth), maintenance (Rmaintenance), and overflow (Roverflow) components, the last including futile cycles and compensation for stoichiometric imbalances that are activated when C cannot be used for growth or maintenance (Cannell and Thornley, 2000; Thornley and Cannell, 2000; van Bodegom, 2007; Russell and Cook, 1995). Hence, CUE can be expressed in terms of physiologically distinct respiration rates as
which demonstrates that any increase in the maintenance and overflow respiration rates relative to growth respiration due to starvation or environmental stresses decreases CUE (Sect. 4.1).
Finally, combining Eqs. (2) and (6) provides the relation among CUEA, CUE, and C losses via EX and turnover,
Based on this equation, higher turnover or EX rate reduces CUEA but not CUE (Eq. 5).
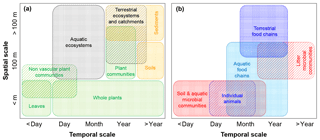
Figure 3Illustration of typical spatial and temporal scales at which C fluxes are calculated to estimate CUE (or CSE) in various subsystems. (a) Scales typical of observations of vegetation, whole ecosystems, and soils and sediments; (b) scales typical of observations of heterotrophic organisms and food chains. Colour codes are as in Fig. 2.
2.3 Carbon balance and efficiency equations for systems including abiotic components
We argued that CUE can be defined for biological entities that use C to improve their fitness but that CSE should be defined for systems including abiotic components (or when organic matter turnover is incomplete) for which fitness cannot be defined. Examples of such coupled biotic–abiotic systems are whole ecosystems (terrestrial and aquatic), soils, and sediments, for which different biological actors (primary producers, decomposers, herbivores, predators) mediate C cycling in association with abiotic processes such as C transport by advection (Chapin et al., 2006; Cole et al., 2007) and C mineral interactions (Schmidt et al., 2011; Kaiser and Kalbitz, 2012). For these integrated systems, Eq. (1) should be expanded to include these additional C exchange processes (Fig. 2),
In Eq. (9), U and R represent the C uptake and respiration rates by the biotic components of the ecosystem (as in Eq. 4), whereas U refers to litterfall and C deposition when considering soils and sediments, respectively (Table 3). Fin and Fout are C inputs and outputs occurring via abiotic exchanges of organic and inorganic C in natural ecosystems but also account for anthropogenic inputs (e.g. manure) and outputs (e.g. harvested products) in managed ecosystems. With reference to ecosystems, the C balance of Eq. (9) can also be expressed in terms of the net ecosystem C balance, NECB (Chapin et al., 2006).
Analogously with Eq. (2) and using the rates defined in Eq. (9), the CSE for the whole system can thus be defined as
In a purely abiotic system (), Eq. (10) can be simplified to . In contrast, when the abiotic exchange rates are negligible (), Eq. (10) is simplified to , i.e. the CUE of the biological components in the system (as in Eq. 5, with EG = 0). Based on Eq. (10), CSE > 0 when an ecosystem stores C (e.g. systems with long-term accumulation of C in un-decomposed necromass, mineral-associated pools, or sediments). As for Eq. (5), the meaning of the C exchange rates in Eq. (10) depends on the system under consideration, e.g. U= GPP for entire ecosystems, but U is equal to the rate of C input to soils when calculating soil CSE (Table 3).
Substituting the definition of CUE for the biological components into Eq. (10), and assuming dominant biological C losses via respiration, an expression linking the system CSE and the biological CUE is found to be
This relation essentially expresses the C losses from the system in two complementary ways – on the left-hand side as the fraction of the total C input that is not stored and on the right-hand side as the fraction of the biotic C input that is not stored plus the abiotic losses.
2.4 C-use and C-storage efficiencies in relation to empirical data
Equations (5) and (10) provide general definitions of CUE and CSE for biological and coupled biotic-abiotic systems, respectively (Table 3). The interpretation of these equations is straightforward when a control volume is clearly identified that allows a meaningful empirical estimation of exchange rates and storage changes at the timescale of interest. For example, the body of an animal allows the identification of rates of ingestion, EG, respiration, EX, and net growth that, taken together, close the biomass C balance equation. Even in this conceptually simple case, however, cell turnover is not easily quantified. As such, net growth may be measured, but not gross cell growth – and to actually measure these rates can be challenging. In most cases, defining and separating input and output rates is even more complicated – both conceptually and practically when conducting measurements. For example, closing the C balance of leaves, whole plants or plant communities, and aquatic systems is challenging because both input and output fluxes are in the form of CO2. Net exchange fluxes can be readily measured, but not gross fluxes, complicating the separation of U (gross photosynthetic rate in this case) and R (gross autotrophic respiration rate) – not to mention C exports to other parts of the plant and as exudates. Other challenges arise when separating autotrophic and heterotrophic contributions to a single measured respiratory CO2 flux. Common approaches for measuring C exchange rates relevant for CUE and CSE calculations are presented and discussed in the Supplement and the wide range of spatial–temporal scales involved are illustrated in Fig. 3. In our data collection, we compared systems ranging from individual organisms and communities to soils and sediments, food webs, and whole ecosystems and watersheds (Tables 2 and 3).
Estimates of CUE for a range of organisms (microorganisms, animals, and individual plants), communities (microbial and plant) and ecosystems have been collected from the literature or calculated based on reported C exchange rates (Table S1). Existing datasets or data collections shown in previous publications are used for CUE of heterotrophic organisms (McNaughton et al., 1989; Manzoni et al., 2017), leaves (Atkin et al., 2015), plant communities (Campioli et al., 2015), whole terrestrial (Luyssaert et al., 2007) and aquatic ecosystems (Hoellein et al., 2013), and for lacustrine and marine sediments (Alin and Johnson, 2007; Canfield, 1994). New literature data collections are developed for CUE of microbial isolates, individual plants, non-vascular vegetation, food chains, soils, and watersheds. The whole database encompasses 5309 CUE estimates.
To compile the new data collections, we conducted an online search using ISI Web of Science and Google Scholar with keywords including various synonyms of CUE or CSE. We also gathered publications following relevant references in articles and books, aided by the expert knowledge of the authors. Due to the enormous variability in terminology used across disciplines, and the fact that in many cases CUE or CSE was not reported in the papers (but only C exchange rates to calculate them), a systematic search was not feasible. Nevertheless, while not exhaustive, our selection of publications covers a broad range of conditions for each subset of data, enabling detection of general patterns across disciplines and scales.
CUE values are recorded in our database as they were reported in the original publications and thus reflect variation in environmental conditions (e.g. temperature, water availability) and organism status (e.g. actively growing, energy or nutrient limited), as well as methodological confounding factors. To facilitate comparisons across datasets, instantaneous CUE values estimated for leaves and non-vascular plant communities were converted to daily values by assuming an equal duration of daytime and night-time and that respiration rates were the same throughout the whole day. Moreover, plant community and ecosystem C fluxes (Campioli et al., 2015; Luyssaert et al., 2007) were averaged first when estimates from different approaches were reported for a given site and year and second across years to provide long-term mean fluxes. The large majority of datasets encompass independent data points obtained from different sites or treatments. Some time series are included to illustrate how CUE values change during plant ontogeny or as resources are consumed in soil incubations (these datasets are not included in statistical analyses requiring independent samples). One dataset required the conversion of energy-based to C-based fluxes to calculate CUE (McNaughton et al., 1989). Energy flux data (kJ m−2 yr−1) were first converted to dry weights using animal and ecosystem-specific plant-community heat of combustion values (Golley, 1961). Dry weights were finally converted to C-mass units assuming a conversion factor of 0.45 g C g−1 dry weight.
We aim to illustrate the range of variation in CUE across spatial and temporal scales, and levels of biological and ecological organization, but not to explain the observed variability. This latter goal would require ancillary data on environmental conditions and physiological status that are not available in all studies. Further, a comparison of CUE estimates across these diverse data sources is also challenging because of the contrasting spatial and temporal scales at which measurements were conducted (Fig. 3). As such, and given our aim, we have not attempted to bring individual CUE estimates to a comparable scale. For individual plants and microbial communities, CUE estimation approaches vary, and in some cases GGE was reported. Considering the lack of information on the relevance of EX rates, for these organisms we grouped published efficiencies under the label CUE. For plant communities in which biomass increments were measured, we use the term GGE (equivalent to biomass production efficiency, as in Campioli et al., 2015).
While it is not possible to quantitatively and statistically compare CUE estimates across all the scales involved due to their different meaning, variations can still be interpreted as a result of scale differences. In subsets of the database in which CUE had been estimated in the original sources at consistent spatial and temporal scales, quantitative comparisons among the median values of each subset are possible and are conducted using the Kruskal–Wallis test with a significance threshold set at p=0.05 (MathWorks, 2011). These subsets are (i) long-term average CUE of plant communities and ecosystems, and CSE of soils (plot-to-field spatial scale and annual-to-decadal scale), and (ii) CUE of microbial isolates, soil microbial communities, and aquatic bacterial communities (sample size of a litre or less; typical timescales on the order of days). Moreover, with the same approach we test differences among the medians of smaller data groups within each subset.
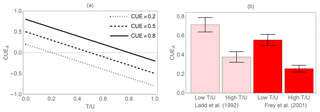
Figure 4Effect of biomass turnover rate on the apparent C-use efficiency (CUEA). (a) Theoretical relation between CUEA and the ratio of turnover rate to C uptake rate (Eq. 8, assuming negligible EX) for three values of the actual CUE. (b) Two examples of how high turnover rates cause a decrease in CUEA in empirical studies on soil microbial communities (Frey et al., 2001; Ladd et al., 1992). Lower turnover rates were caused by lower mortality in the first 3 days of incubation compared to day 112 (Ladd et al., 1992) or by lower grazing in the first 2 days of incubation compared to days 7–8 (Frey et al., 2001). Error bars indicate standard errors of the mean (variability is across three soil types in Ladd et al., 1992, and across replicates and soil types in Frey et al., 2001).
For visual comparison, CUE data are grouped according to the subsystems shown in Fig. 2, and the distribution of the available CUE estimates is shown using box plots. For each subsystem, some examples are extracted to illustrate specific CUE patterns, and the 90th percentile of each group is calculated to provide an indication of the maximum CUE that a subsystem can achieve.
Based on the theory outlined in Sect. 2, we present examples on how measured CUE can be driven by true biological factors that affect C partitioning in organisms and how apparent CUE can be affected by confounding factors such as biomass turnover rates (Sect. 4.1). We then present a synthesis and discussion of CUE trends along biological and ecological levels of organization (Sect. 4.2) and across spatial and temporal scales (Sect. 4.3), and we compare systems with and without abiotic transport processes (Sect. 4.4). Finally, we ask to what degree CUE estimates are useful for characterizing C allocation patterns and eventually informing C-storage calculations and ecosystem models (Sect. 4.5).
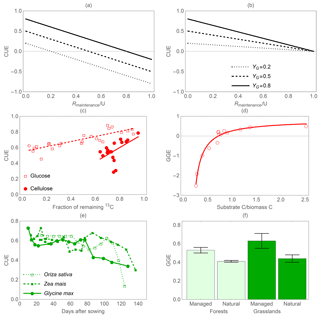
Figure 5Effect of maintenance respiration (Rmaintenance) on C-use efficiency (CUE). Theoretical relations between CUE and the ratio of maintenance respiration to C uptake rate under two different assumptions: (a) priority to growth respiration and (b) priority to maintenance respiration for three values of growth yield (i.e. (C uptake – growth respiration) ∕ C uptake). The central panels show decreasing CUE when (c) the C substrate is consumed (moving right to left along the abscissa) during 12-day (glucose) and 71-day (cellulose) incubations (Öquist et al., 2017) or (d) resource availability (as the ratio of salicylic acid C to biomass C) is low (Collado et al., 2014). (e) Reduction in CUE through time as plants end their growth phase and set seeds (Yamaguchi, 1978). (f) Significantly higher (p<0.05) GGE of managed, and thus more nutrient-rich, forests and grasslands (Campioli et al., 2015). In (c) to (e), CUE or GGE decrease as costs for maintenance respiration increase relative to growth respiration; in (f), GGE decreases when costs for symbiotic associations are higher (natural systems). Curves in (c) and (d) are least-square linear and hyperbolic regressions drawn to guide the eye; error bars indicate standard errors of the mean.
4.1 Biological drivers vs. confounding factors of C-use efficiency at the organism and community levels
Various forms of CUE are used to characterize the fate of C inputs into a system. To this purpose, CUE is often estimated by measuring changes in C content of and C inputs to that system (Eq. 3). If biomass turnover and EX can be neglected, this apparent CUE is a good approximation of the actual CUE (Eq. 8), but in most cases biomass turnover is present and hard to quantify – in such a case, CUEA estimates can be significantly lower than the actual CUE (Hagerty et al., 2014; Grossart and Ploug, 2001). Figure 4a shows how apparent CUE is expected to decrease with increasing turnover rate in relation to C uptake (Eq. 8). The CUEA values can in principle become negative when the turnover rate is higher than the growth rate (similar issues arise at the ecosystem and watershed scales, but due to C transport rather than turnover). Figure 4b illustrates these effects by considering data from two studies on soil systems in which turnover rate was manipulated. In the first study (Ladd et al., 1992), the 14C glucose initially added to the soil is taken up by microorganisms with a certain actual CUE, but as the incubation progresses, the 14C remaining in the microbial biomass decreases partly due to cell turnover. As a result, CUEA at the beginning of the incubation was higher than after about 100 days. It is also possible that during this period substrates became less available, leading to an increase in maintenance respiration compared to growth respiration (as discussed in the following). In the second example, biomass turnover was manipulated by controlling soil fauna feeding on soil microorganisms (Frey et al., 2001). When grazers were active, the CUEA estimated from C accumulation into biomass was lower than in the samples without grazers. However, if CUE was calculated from changes in C substrate (glucose) and respiration, estimates were insensitive to grazing pressure (Frey et al., 2001). Similarly, decreasing CUEA has been found in aquatic bacteria subjected to increasing grazing pressure (Grossart and Ploug, 2001). We therefore expect that for a given experimental setting, higher rates of mortality or predation will lead to underestimation of CUE, when using Eq. (3).
Figure 5 illustrates how the relative importance of maintenance costs (respiration and EX) compared to growth respiration alters CUE or GGE. Theoretical predictions are shown in Fig. 5a, b, in which two methods often used in models to account for the metabolic costs of maintenance are considered (Thornley and Cannell, 2000). When growth respiration has priority over maintenance respiration, C required to sustain maintenance costs is obtained from the pool of assimilated C. In this case, CUE decreases linearly with increasing maintenance costs and CUE can become negative because maintenance may cause a net biomass loss (Fig. 5a). As an alternative, C required to fulfil maintenance costs can be directly deducted from the C uptake rate, before C is assimilated and available for growth respiration. In this case, CUE can reach zero at the lowest, when all the C taken up is used for maintenance (Fig. 5b). Thus, both modelling approaches yield the same result that CUE decreases with increasing maintenance costs. Empirical evidence lends support to the prediction that maintenance costs decrease the overall CUE, whereas actively growing organisms in which growth respiration is dominant have higher CUE (Sinsabaugh et al., 2015; del Giorgio and Cole, 1998). This simplified view explains some but not all observed patterns in CUE. For example, low-resource environments could select for high-CUE organisms despite low growth rates. At the other end of the resource availability spectrum, to achieve high growth rates, it might be necessary to increase respiratory losses via C overflow, futile cycles, and increasing costs of protein turnover. Thus, at very high G, a trade-off between growth and CUE may emerge (Lipson, 2015). Combining these pieces of evidence, CUE would be expected to first increase with increasing G, then reach a peak and decrease at high G values.
The effect of increasing EX rate on CUE varies depending on how such increases are realized. If the increase in EX is fuelled by a correspondingly higher U, CUE also increases; however, if the increase in EX occurs at the expense of G, such that G+ EX is constant for a given U, CUE will not be affected. In both scenarios, higher EX decreases the net biomass production, and hence lowers GGE. For example, consistent with these expectations, the microbial CUE values of an aerobic soil (in which EX was negligible) and an anaerobic soil (where EX was of the net biomass increment) were comparable (respectively 0.73 vs. 0.70) because the sum of EX and biomass production were similar (Šantrůčková et al., 2004). However, the GGE of the aerobic soil was much higher than in the anaerobic soil (0.72 vs. 0.43).
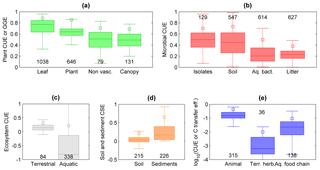
Figure 6Patterns in C-use efficiency (CUE) across scales and levels of organization. (a) CUE of leaves and non-vascular plant communities, and GGE of whole plants and vascular plant communities; (b) CUE of microbial isolates and communities of soil microorganisms, aquatic bacteria, and litter microorganisms; (c) CUE of terrestrial and aquatic ecosystems (note that the y axis extends to −1, indicating C losses larger than primary productivity); (d) C-storage efficiency (CSE) of soils and sediments (note that the y axis extends to −0.5); (e) CUE of individual animals and terrestrial herbivore communities, and C transfer efficiency of aquatic food chains, plotted on a log scale to allow a visual comparison. The box plots display median and quartiles (box) and range excluding outliers (whiskers), and the open squares indicate the 90th percentiles; numbers indicate sample sizes; colour codes are as in Fig. 2. Data sources are described in the Supplement.
To illustrate the effects of higher maintenance costs, we can consider the example of microbial communities. Microbial CUE and GGE tend to be low just after addition of a labile C substrate (a lag phase, which can vary in length depending on the preceding physiological status), then to increase sharply as growth rate increases, and finally to decrease because microbes switch from a relatively efficient growth mode when substrates are available to a less efficient maintenance mode when substrates have been exhausted (Öquist et al., 2017) (Fig. 5c, d). Notably, when reductions in biomass occur under starvation conditions due to catabolic conversion of biomass to cover maintenance respiration, CUE < 0 (Fig. 5d). Similarly, crops maintain a high CUE until they stop growing vegetative tissues, which senesce while resources are translocated to seeds (Fig. 5e). In forests, GGE (defined as biomass accumulation over GPP; see Table 2) declines with decreasing nutrient availability (Fig. 5f). However, different from other examples in Fig. 5, this decline cannot be attributed to higher respiration under nutrient-limited conditions but more likely to higher C investment in plant symbionts (Campioli et al., 2015; Baskaran et al., 2017). Because the effects of higher maintenance respiration or EX rate have the same direction – both decreasing GGE – we can expect that along resource or environmental gradients characterized by increasing maintenance costs (including EX), GGE will decrease. Along the same gradients, CUE would decrease only if maintenance costs increase, while it would be unaffected by changes in EX rates alone.
A similar argument has been proposed to explain increases in GGE with increasing nutrient-to-C ratios of the resources used by heterotrophic organisms (Manzoni et al., 2017, in which the term CUE was used under the implicit assumption CUE ≈ GGE). High nutrient availability with respect to C allows growth of the nutrient-rich cells typical of heterotrophs in C-limited conditions. However, under nutrient shortage and assuming that cell nutrient concentrations are relatively stable (homeostasis), resources contain C in excess, which can be selectively removed via overflow mechanisms (Russell and Cook, 1995; Boberg et al., 2008), increased C excretion (Anderson et al., 2005), and possibly C investment in extracellular compounds that promote resource availability (Middelboe and Sondergaard, 1993). As a result, C losses can become decoupled from growth, leading to reduced GGE under conditions of nutrient shortage (Manzoni et al., 2017).
4.2 C-use efficiency across levels of biological and ecological organization
We start from the C balance of leaves and move towards whole organisms, communities, food webs, and entire ecosystems to illustrate how CUE varies across levels of biological and ecological organization. The majority of C taken up by leaves is converted into products (CUE ≈ 0.8, Fig. 6a), as might be expected for the organ responsible for entry of C into the biosphere. While leaves only have to support their own limited metabolic needs, whole plants require energy to maintain a range of additional functions, including nutrient uptake and use, regulation of ion balances and phloem transport (Cannell and Thornley, 2000; Thornley and Cannell, 2000). Thus, the cost of maintaining a complex organism reduces CUE from leaf-level values of around 0.9 to whole-plant values of 0.6 (maximum CUE ≈ 0.85). Similarly, moving from unicellular to multicellular heterotrophic organisms requires additional C costs to maintain the structures of increasingly complex bodies (DeLong et al., 2010), resulting in a declining average CUE from approximately 0.5 (maximum CUE ≈ 0.7) to 0.15 (maximum CUE ≈ 0.5; Fig. 6b, e).
Comparing terrestrial bacteria and fungi, it has been suggested that they should differ in CUE, mostly due to their contrasting life histories (fast growing, inefficient bacteria vs. slow-growing, efficient fungi). Although this paradigm has been around for some time, the hypothesis was not unequivocally supported (Thiet et al., 2006; Six et al., 2006). Recently, fungi and Gram-negative bacteria have been suggested as important biomarkers when evaluating CUE (Bölscher et al., 2016), but the link between the two is so far not clearly established. The collected CUE data for litter decomposers (arguably mostly fungi, at least in the first phases of litter degradation) suggest a lower CUE than in bacterial communities (Fig. 6b). However, litter decomposers in forest ecosystems face strong stoichiometric imbalances and CUE estimates for these organisms represent long-term averages including periods of slow growth (Manzoni et al., 2017). These factors could explain the lower average CUE of litter decomposers and aquatic microorganisms compared to soil microorganisms and bacterial isolates – these patterns are thus driven by environmental effects, in addition to organism complexity and and methodological differences.
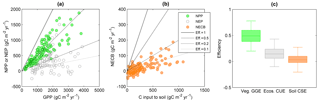
Figure 7Relations between gross primary productivity (GPP) and (a) net primary productivity (NPP) or net ecosystem productivity (NEP), and (b) between NPP and net ecosystem C balance (NECB) in terrestrial systems. In these plots, CUE corresponds to the slopes of lines passing through the origin (four are shown for illustration). (c) Comparison of vegetation GGE, whole ecosystem CUE, and soil CSE (see also Fig. 2). Colour codes are as in Fig. 2. Data sources are described in the Supplement.
Food webs include interacting organisms that exchange C among them and with the environment. Each organism exchanges C according to its own CUE (for a modelling example, see Frouz et al., 2013b), but also provides C to the next organism (consumer or predator) in the food web. The C transfer efficiency, defined as the growth rate of a target organism over the rate of C entering the food web (Sect. 1.5 in the Supplement), is then expected to be lower than the CUE of the constituent organisms, as C is lost at each step in the food web (Fig. 6e). Moreover, antagonistic interactions in a food web may increase metabolic costs, also lowering CUE (Toljander et al., 2006). Similar to the organism-level responses to resource availability, C transfer efficiencies also tend to be higher in resource-rich environments. For example, the fish-to-phytoplankton production ratio is higher under nutrient-rich conditions (Dickman et al., 2008). In soils, a food web developing on low C : N litter can be more efficient at retaining C in the system than one developing on high C : N litter, despite no difference in C input (Frouz et al., 2013a). In the latter example, it is important to emphasize the role of soil fauna in mediating this response to nutrient availability – the presence of macrofauna facilitates the transport of C towards sites where it can be stabilized (via bioturbation).
Like moving from simple towards more complex organisms or from single individuals to interacting organisms in food webs, consideration of whole ecosystems also results in lower C retention capacity compared to individual organisms and communities. Aggregation of processes results in a lower CUE for a given GPP, in particular when adding more heterotrophic components (Fig. 2). In fact, including the contribution of heterotrophic respiration is expected to decrease ecosystem CUE compared to vegetation CUE because more of the C taken up by plants is returned to the atmosphere (Fig. 7; Eq. S4 in the Supplement). The GGE of vascular plant communities is indeed significantly higher (≈ 0.4) than the ecosystem CUE (≈ 0.2), and this difference in efficiency would be even larger if we considered plant CUE (recall that GGE < CUE; Eq. 6). Ecosystem CUE is also expected to be lower than the soil C-storage efficiency because ecosystem CUE is approximately the product of soil CSE (= NECB ∕ NPP) and vegetation CUE (= NPP ∕ GPP; Supplement Sect. 1.6). This is not the case in the data presented in Fig. 7, for which the median soil CSE is significantly lower than the median ecosystem CUE. This unexpected result could be explained by the fact that in agricultural systems such as those we used to estimate soil CSE, soil disturbance strongly reduces C accumulation compared to a natural system.
We can also ask how the CUE of individual ecosystem components affects the overall ecosystem CUE. It could be argued that with more efficient organisms, the ecosystem-level CUE would increase, resulting in larger C accumulation (for soil systems, see Cotrufo et al., 2013). There is indeed evidence that microbial communities with higher CUE enhance soil C storage in terrestrial systems (Kallenbach et al., 2016). However, decomposers alter the kinetics of decomposition via extracellular enzymes that are thought to be produced in proportion to the living biomass (Schimel and Weintraub, 2003). As a result of these feedbacks, it is possible that lower (rather than higher) decomposer CUE increases ecosystem CUE and thus C-storage potential, as indicated by empirical studies in boreal forests (Kyaschenko et al., 2017) and modelling results (Allison et al., 2010; Baskaran et al., 2017).
Comparing aquatic and terrestrial systems, ecosystem CUE and soil or sediment CSE exhibit contrasting patterns. While the CUE of aquatic ecosystems is significantly lower than that of terrestrial ecosystems (Fig. 6c), the CSE of lacustrine and marine sediments is significantly higher than that of soils (Fig. 6d). The first pattern is explained by allochthonous C contributions to respiration (Sect. 4.3). This explanation should also hold considering that aquatic ecosystem CUE values are calculated from daily fluxes, whereas terrestrial ecosystem CUE values are calculated from long-term (inter-annual) mean fluxes. In contrast, the higher CSE of sediments than of soils can be explained by the often high sedimentation rate (Calvert et al., 1992) and the relatively short exposure time to oxygen after burial of organic C (Canfield, 1994; Hedges et al., 1999), whereas most soils remain aerobic and C-storage capacity may saturate (Stewart et al., 2007). Indeed, paddy soils in which respiration is low due to anaerobic conditions store C more efficiently (median CSE = 0.07) than other agricultural soils (median CSE = 0.02; p < 0.05). Moreover, physical losses from soils (leaching, erosion) are probably larger than for sediments, at least in stable depositional environments.
Based on these analyses we can conclude that higher levels of biological or ecological organization generally imply a more open C cycle – this is caused by increasingly costly structures to maintain complex organisms and by increasing heterotrophic contributions when assessing the C-storage potential of ecosystems as opposed to primary producers alone.
4.3 C-use efficiency across spatial and temporal scales
Moving up spatial and temporal scales involves integrating C exchange rates in space and time. In turn, integrating these exchange rates essentially averages out the contributions at the smaller or shorter scales by considering a larger number of organisms (e.g. populations vs. individuals) or species (communities vs. populations), a larger spatial domain, and longer periods of time. This averaging effect generally leads to a lower CUE than at the smaller scales. As shown in Fig. 3, CUE is estimated over a range of spatial and temporal scales depending on the system of interest, which requires us to interpret CUE in the light of averaged C exchange rates at these scales.
Because organism-level CUE estimates are biased towards actively growing individuals often isolated in highly controlled conditions, spatial averaging under field conditions, in which inactive or slowly growing individuals are also included, results in lower population- or community-level CUE. In the case of plants, CUE of individuals is on average around 0.6, whereas in plant communities GGE ≈ 0.4 (Fig. 6a). Part of this difference might be attributed to EX rates that cause CUE > GGE, but other interpretations are also possible. Quoting Gifford (2003), “The difference may be an expression mostly of the impact of recurring stresses and resource limitations and the much greater average age of plants in the forests than in the controlled environments. Presumably the respiratory requirement for acquiring water and nutrients is lower when they are abundantly available” (pp. 179–180). Moreover, antagonistic interactions within communities might increase C costs (Toljander et al., 2006). This contrast between CUE estimates at individual and community scales is not apparent when comparing CUE of microbial isolates and soil microbial communities, which are not statistically different (CUE ≈ 0.45, Fig. 6b). However, CUE of aquatic microbial communities from our dataset is significantly lower than that of microbial isolates (CUE ≈ 0.25), despite the occurrence of high values in some communities (del Giorgio and Cole, 1998). The high CUE of soil microbial communities could be due to generally higher resource availability in soils than in aquatic environments, or to amendment of soils with labile compounds that stimulate microbial activity and mask the contribution of slow-growing organisms (Sinsabaugh et al., 2013).
Integrating C exchange rates through time also tends to lower CUE with respect to short-term measurements often conducted after adding labile substrates to heterotrophic systems (Fig. 5c), or during active growing periods for plants (Fig. 5e). Instead, long-term CUE (assuming biomass turnover is correctly accounted for) includes periods of slow growth due to unsuitable environmental conditions, during which maintenance costs remain high while growth stagnates. As mentioned in Sect. 4.2, this could explain why long-term CUE of litter microorganisms is lower than microbial CUE measured over short periods in other systems (Fig. 6b).
4.4 Interpreting C-use and C-storage efficiencies in systems with abiotic and anthropogenic C fluxes
Transport processes can decouple local GPP from ecosystem respiration by feeding heterotrophs with allochthonous C or removing products of primary productivity before they enter the decomposition and herbivory pathways. Allochthonous C can cause relatively large respiration losses even with low inputs from GPP (Duarte and Prairie, 2005; Hoellein et al., 2013), resulting in low or negative values of CUE when defined as NEP ∕ GPP. A more useful definition of CUE should account for allochthonous C inputs, which are however seldom measured (Eq. 10). This pattern is apparent when comparing the CUE of terrestrial and inland aquatic ecosystems (Fig. 6c) – the former being predominantly positive, the latter being most often strongly negative. Despite inland aquatic systems having negative ecosystem CUE due to large allochthonous inputs, marine systems can act as C sinks due to long-term storage in sediments (with C storage in the range 0.01 %–0.4 % of NPP; Seiter et al., 2005; Falkowski, 2014), as well as accumulation of dissolved inorganic C.
Physical removal of C also alters the estimated CSE. Because physical removal reduces the C that can be stored for a given uptake rate, CSE decreases with increasing abiotic losses of C (Fout). When these losses of C are considered in addition to respiration, CSE decreases with respect to the ecosystem CUE estimated from biological fluxes, as shown at the ecosystem- and watershed-scale respectively by Eqs. (S5) and (S6) in the Supplement. We assessed this effect using the few available watershed-scale studies in which organic C losses via leaching and subsequent advection in surface water bodies were measured. When only the biological components are considered, we found an average ecosystem CUE = 0.137, whereas including abiotic C losses leads to CSE = 0.104 – i.e. a > 30 % reduction in efficiency. Similarly, in marine systems the export of particulate C from the euphotic zone by particle sinking lowers the potential efficiency of C storage in that zone, while allowing long-term storage in the sediments (Dunne et al., 2005).
A large fraction of land and of marine systems are managed to extract food and fibre to support a growing human population (Krausmann et al., 2013). Management of ecosystems has two contrasting effects on CSE, depending on the balance of harvest removal, improved production, and organic amendments. On the one hand, extracting harvested products (Fout>0) lowers CSE because a lower fraction of GPP remains in the system. For example, assuming a crop harvest index ranging from 25 % to 50 % of aboveground biomass (e.g. Unkovich et al., 2010) and a 30 % allocation to roots, the percentage of NPP harvested and the corresponding reductions in CSE would range from 17 % to 33 % (Eq. 10). On the other hand, management may improve CSE by increasing the production efficiency of vegetation (Campioli et al., 2015), or involve addition of organic C to fields (Fin>0; e.g. manure or biochar). These C amendments increase CSE for given respiration and harvest rates, not only thanks to their direct effect through Fin but also thanks to indirect effects when soil amendments promote plant productivity. However, this positive effect lessens as the amended organic C is eventually respired and soil organic C reaches saturation levels (Stewart et al., 2007).
4.5 Do we need C-use efficiency estimates?
The practical difficulties in estimating CUE at various scales and the inherent conceptual challenges with its multiple definitions beg the question as to whether it is useful to even discuss CUE. On the one hand, there are theoretical and conceptual advantages for using CUE as a “macroscopic” parameter characterizing organism or ecosystem metabolism – even without quantifying the underlying drivers (specific metabolic pathways, or detailed input and output rates). In fact, by focusing on the conversion of C into new products rather than on C fluxes per se, CUE and CSE patterns offer alternative insights into the inner workings of the processes regulating the C cycle. On the other hand, full process understanding requires identification of these drivers and in such a case, CUE is merely the result of their combination, and knowledge of CUE values alone would be of little use.
The CUE is less variable than the rates of C exchange that define it and therefore allows the comparison of systems characterized by very different C exchange rates. For example, respiration and growth rates of microbial communities roughly double every 10 ∘C increment in temperature, whereas CUE changes much less – ranging from a 25 % decrease for every 10 ∘C temperature increment (Frey et al., 2013) to no change at all (Dijkstra et al., 2011), depending on the CUE estimation method. Relatively stable efficiencies are particularly useful for modelling purposes, as they allow us to “close” otherwise open (i.e. undetermined) mass balance equations. Similarly, while NPP, GPP, and ecosystem respiration vary by 2 orders of magnitude across biomes (Fernandez-Martinez et al., 2014), CUE values are relatively more constrained (if we exclude ecosystems with negative NEP).
Moreover, non-dimensional numbers – such as CUE and CSE – emerge as key drivers of system dynamics (Vogel, 1998; Buckingham, 1914; Feng et al., 2018). For example, CUE appears in stoichiometric equations describing nutrient fluxes in relation to C fluxes (Manzoni et al., 2010; Sterner and Elser, 2002). In these stoichiometric models, it is often not necessary to distinguish among various respiration components or to define specific kinetic laws for C exchange rates – a single macroscopic, lumped CUE parameter is sufficient to describe the balance of growth and respiration. However, if CUE varies through time or in response to environmental conditions in complex ways, the advantages of having a single lumped parameter may be overcome by a cumbersome parameterization to describe these effects.
A similar issue arises when implementing biological processes that could result in variable CUE in models of soil biogeochemical processes (Allison et al., 2010; Frey et al., 2013), the marine C cycle (Dunne et al., 2005), or vegetation dynamics (Huntingford et al., 2017; Smith et al., 2016). These models differ widely in the way they parameterize the C cycle. For some components of the ecosystem, certain models assume constant CUE values (e.g. CUE of microbial decomposers), whereas for others, more detailed descriptions are employed, resulting in flexible CUE (e.g. separating respiration components in vegetation) (Gifford, 2003). Empirically established patterns of variation in CUE thus help identification of systems and conditions under which CUE is indeed stable or, in contrast, when additional processes driving variable CUE must be accounted for in models. For example, if soil biogeochemical models are parameterized using microbial CUE values obtained from laboratory incubations, erroneous predictions could be made if those incubations are not representative of soils under natural conditions. Apart from possible scale mismatches between empirical estimates of CUE and model interpretation, models that assume a stationary set of metabolic responses could underestimate C retention. This is the case when CUE acclimates and buffers the consequences of climatic changes by reducing C losses from the biosphere. In contrast, if changes in CUE amplify biosphere responses – e.g. due to selection of inefficient early-successional species – these models might underestimate potential feedbacks between the biosphere and global climate.
In addition to the correct attribution of changes in CUE to processes or environmental conditions, it remains critical to match the definition of CUE used by empiricists with that implemented in models. Specifically, are the same biosynthesis components (e.g. biomass increment vs. exudate export) accounted for in both empirical efficiency estimates and in the model equations? Are abiotic C exchanges at the ecosystem scale both included in empirical estimates of CSE and described by models? As CUE and CSE represent emerging properties of organisms and ecosystems, they are appealing for model testing, but without a consistent definition, comparisons of model outputs and empirical estimates are not meaningful.
We have synthesized definitions of and explored variations in the efficiency of C use by organisms, communities and ecosystems, and in the efficiency of C storage in soils and sediments. This synthesis highlighted conceptual similarities in the way these efficiencies are defined across disciplines and some common terminological and interpretation issues. In particular, the same term CUE (but also other synonyms) is often used at organism-to-community scales to indicate actual C-use efficiency (Eq. 5), apparent C-use efficiency (related but not equal to CUE, Eq. 8), and gross growth efficiency. This mixed use may cause misinterpretations, as it is not clear whether turnover and biological products are included in the CUE calculations. Similarly, at the ecosystem scale the term CUE is used without specifying whether abiotic and anthropogenic fluxes are accounted for. For improved clarity, we suggest always defining how CUE is estimated with particular attention to C exchanges other than biomass increments and respiration.
Our synthesis shows that turnover deflates apparent CUE estimates, but not actual CUE calculated as the biosynthesis-to-C uptake ratio. Improving growing conditions generally increases CUE and CSE because it promotes growth processes over C loss processes. Finally, CUE tends to decrease with the level of ecological organization – e.g. from rapidly growing individual organisms to natural communities and ecosystems – as less efficient individuals are considered in communities and more heterotrophic components are sequentially added to the system. Because CUE and CSE are outcomes of a wide spectrum of processes, they are expected to be flexible and to respond to both biological (e.g. trends in growth vs. respiration) and physical controls (e.g. C transport and environmental conditions). As such – and provided that empirical and model definitions of these efficiencies are consistent – they are useful indices of changes in the C cycle through time and space and could be employed to benchmark short-term (in the case of CUE) and long-term predictions (CSE) of soil and ecosystem models.
The datasets supporting the results that are not already published are archived in the open-access database of the Bolin Centre for Climate Research (https://bolin.su.se/data/Manzoni-2018, Manzoni et al., 2018).
The supplement related to this article is available online at: https://doi.org/10.5194/bg-15-5929-2018-supplement.
This work was designed by all the authors during two workshops. SM led the writing of the paper and prepared the figures; SM, PČ, PP, MT, MW, and GV collated and analysed data from the literature; all authors contributed to locating literature sources, discussing the content, and writing the paper.
The authors declare that they have no conflict of interest.
Funding was provided by the Bolin Centre for Climate Research (Research Area 4), through the project “Scaling carbon-use efficiency from the organism-
to the global-scale”, and by the Swedish Research Councils, Formas (grant
2015-468 to Björn D. Lindahl and Stefano Manzoni) and Vetenskapsrådet (grants 2016-04146 to Björn D. Lindahl and
Stefano Manzoni, 2016-06313 to Stefano Manzoni, 621-2014-4266 to Christian Beer, and 2016-04910 to Giulia Vico). Hana Šantrůčková
and Jan Frouz acknowledge MEYS CZ grants LM2015075 and EF16_013/0001782 –
SoWa Ecosystems Research, and DAW acknowledges support from an NSERC
Discovery Grant; Giulia Vico acknowledges the project “TC4F – Trees and Crops for
the Future”. We also thank all site investigators, their funding agencies,
the various regional flux networks, and the FLUXNET project, for support to
the development of the database “Global Forest Ecosystem Structure and
Function Data For Carbon Balance Research” (Luyssaert et al., 2009).
The article processing charges for this open-access
publication were covered by Stockholm University.
Edited by: Sebastiaan Luyssaert
Reviewed by: three anonymous referees
Alin, S. R. and Johnson, T. C.: Carbon cycling in large lakes of the world: A synthesis of production, burial, and lake-atmosphere exchange estimates, Global Biogeochem. Cy., 21, GB3002, https://doi.org/10.1029/2006gb002881, 2007.
Allison, S. D.: Modeling adaptation of carbon use efficiency in microbial communities, Front. Microbiol., 5, 1–9, https://doi.org/10.3389/fmicb.2014.00571, 2014.
Allison, S. D., Wallenstein, M. D., and Bradford, M. A.: Soil-carbon response to warming dependent on microbial physiology, Nat. Geosci., 3, 336–340, 2010.
Anderson, T. R., Hessen, D. O., Elser, J. J., and Urabe, J.: Metabolic stoichiometry and the fate of excess carbon and nutrients in consumers, Am. Nat., 165, 1–15, 2005.
Atkin, O. K., Bloomfield, K. J., Reich, P. B., Tjoelker, M. G., Asner, G. P., Bonal, D., Bonisch, G., Bradford, M. G., Cernusak, L. A., Cosio, E. G., Creek, D., Crous, K. Y., Domingues, T. F., Dukes, J. S., Egerton, J. J. G., Evans, J. R., Farquhar, G. D., Fyllas, N. M., Gauthier, P. P. G., Gloor, E., Gimeno, T. E., Griffin, K. L., Guerrieri, R., Heskel, M. A., Huntingford, C., Ishida, F. Y., Kattge, J., Lambers, H., Liddell, M. J., Lloyd, J., Lusk, C. H., Martin, R. E., Maksimov, A. P., Maximov, T. C., Malhi, Y., Medlyn, B. E., Meir, P., Mercado, L. M., Mirotchnick, N., Ng, D., Niinemets, U., O'Sullivan, O. S., Phillips, O. L., Poorter, L., Poot, P., Prentice, I. C., Salinas, N., Rowland, L. M., Ryan, M. G., Sitch, S., Slot, M., Smith, N. G., Turnbull, M. H., VanderWel, M. C., Valladares, F., Veneklaas, E. J., Weerasinghe, L. K., Wirth, C., Wright, I. J., Wythers, K. R., Xiang, J., Xiang, S., and Zaragoza-Castells, J.: Global variability in leaf respiration in relation to climate, plant functional types and leaf traits, New Phytol., 206, 614–636, https://doi.org/10.1111/nph.13253, 2015.
Azam, F. and Malfatti, F.: Microbial structuring of marine ecosystems, Nat. Rev. Microbiol., 5, 782–791, https://doi.org/10.1038/nrmicro1747, 2007.
Baskaran, P., Hyvonen, R., Berglund, S. L., Clemmensen, K. E., Agren, G. I., Lindahl, B. D., and Manzoni, S.: Modelling the influence of ectomycorrhizal decomposition on plant nutrition and soil carbon sequestration in boreal forest ecosystems, New Phytol., 213, 1452–1465, https://doi.org/10.1111/nph.14213, 2017.
Benner, R. and Herndl, G. J.: Bacterially derived dissolved organic matter in the microbial carbon pump, in: Microbial carbon pump in the ocean, edited by: Jiao, N., Azam, F., and Sanders, S., Science/AAAS, Washington, DC, 46–48, 2011.
Boberg, J., Finlay, R. D., Stenlid, J., Nasholm, T., and Lindahl, B. D.: Glucose and ammonium additions affect needle decomposition and carbon allocation by the litter degrading fungus Mycena epipterygia, Soil Biol. Biochem., 40, 995–999, https://doi.org/10.1016/j.soilbio.2007.11.005, 2008.
Bölscher, T., Wadsö, L., Börjesson, G., and Herrmann, A. M.: Differences in substrate use efficiency: impacts of microbial community composition, land use management, and substrate complexity, Biol. Fert. Soils, 52, 1–13, https://doi.org/10.1007/s00374-016-1097-5, 2016.
Bradford, M. A. and Crowther, T. W.: Carbon use efficiency and storage in terrestrial ecosystems, New Phytol., 199, 7–9, https://doi.org/10.1111/nph.12334, 2013.
Buckingham, E.: On physically similar systems, illustrations of the use of dimensional equations, Phys. Rev., 4, 345–376, 1914.
Calvert, S. E., Bustin, R. M., and Pedersen, T. F.: Lack of evidence for enhanced preservation of sedimentary organic-matter in the oxygen minimum of the gulf of California, Geology, 20, 757–760, https://doi.org/10.1130/0091-7613(1992)020<0757:loefep>2.3.co;2, 1992.
Campioli, M., Vicca, S., Luyssaert, S., Bilcke, J., Ceschia, E., Chapin Iii, F. S., Ciais, P., Fernandez-Martinez, M., Malhi, Y., Obersteiner, M., Olefeldt, D., Papale, D., Piao, S. L., Penuelas, J., Sullivan, P. F., Wang, X., Zenone, T., and Janssens, I. A.: Biomass production efficiency controlled by management in temperate and boreal ecosystems, Nat. Geosci., 8, 843–846, https://doi.org/10.1038/ngeo2553, 2015.
Canfield, D. E.: Factors influencing organic-carbon preservation in marine-sediments, Chem. Geol., 114, 315–329, https://doi.org/10.1016/0009-2541(94)90061-2, 1994.
Cannell, M. G. R. and Thornley, J. H. M.: Modelling the components of plant respiration: Some guiding principles, Ann. Bot.-London, 85, 45–54, 2000.
Chapin, F. S., Woodwell, G. M., Randerson, J. T., Rastetter, E. B., Lovett, G. M., Baldocchi, D. D., Clark, D. A., Harmon, M. E., Schimel, D. S., Valentini, R., Wirth, C., Aber, J. D., Cole, J. J., Goulden, M. L., Harden, J. W., Heimann, M., Howarth, R. W., Matson, P. A., McGuire, A. D., Melillo, J. M., Mooney, H. A., Neff, J. C., Houghton, R. A., Pace, M. L., Ryan, M. G., Running, S. W., Sala, O. E., Schlesinger, W. H., and Schulze, E. D.: Reconciling carbon-cycle concepts, terminology, and methods, Ecosystems, 9, 1041–1050, https://doi.org/10.1007/s10021-005-0105-7, 2006.
Clark, D. A., Brown, S., Kicklighter, D. W., Chambers, J. Q., Thomlinson, J. R., and Ni, J.: Measuring net primary production in forests: Concepts and field methods, Ecol. Appl., 11, 356–370, 2001.
Cole, J. J., Prairie, Y. T., Caraco, N. F., McDowell, W. H., Tranvik, L. J., Striegl, R. G., Duarte, C. M., Kortelainen, P., Downing, J. A., Middelburg, J. J., and Melack, J.: Plumbing the global carbon cycle: Integrating inland waters into the terrestrial carbon budget, Ecosystems, 10, 171–184, https://doi.org/10.1007/s10021-006-9013-8, 2007.
Collado, S., Rosas, I., Gonzalez, E., Gutierrez-Lavin, A., and Diaz, M.: Pseudomonas putida response in membrane bioreactors under salicylic acid-induced stress conditions, J. Hazard. Mater., 267, 9–16, https://doi.org/10.1016/j.jhazmat.2013.12.034, 2014.
Cotrufo, M. F., Wallenstein, M. D., Boot, C. M., Denef, K., and Paul, E.: The Microbial Efficiency-Matrix Stabilization (MEMS) framework integrates plant litter decomposition with soil organic matter stabilization: do labile plant inputs form stable soil organic matter?, Glob. Change Biol., 19, 988–995, https://doi.org/10.1111/gcb.12113, 2013.
Darchambeau, F., Faerovig, P. J., and Hessen, D. O.: How Daphnia copes with excess carbon in its food, Oecologia, 136, 336–346, https://doi.org/10.1007/s00442-003-1283-7, 2003.
del Giorgio, P. A. and Cole, J. J.: Bacterial growth efficiency in natural aquatic systems, Annu. Rev. Ecol. Syst., 29, 503–541, 1998.
DeLong, J. P., Okie, J. G., Moses, M. E., Sibly, R. M., and Brown, J. H.: Shifts in metabolic scaling, production, and efficiency across major evolutionary transitions of life, P. Natl. Acad. Sci. USA, 107, 12941–12945, https://doi.org/10.1073/pnas.1007783107, 2010.
DeLucia, E. H., Drake, J. E., Thomas, R. B., and Gonzalez-Meler, M.: Forest carbon use efficiency: is respiration a constant fraction of gross primary production?, Glob. Change Biol., 13, 1157–1167, https://doi.org/10.1111/j.1365-2486.2007.01365.x, 2007.
Dickman, E. M., Newell, J. M., Gonzalez, M. J., and Vanni, M. J.: Light, nutrients, and food-chain length constrain planktonic energy transfer efficiency across multiple trophic levels, P. Natl. Acad. Sci. USA, 105, 18408–18412, https://doi.org/10.1073/pnas.0805566105, 2008.
Dijkstra, P., Thomas, S. C., Heinrich, P. L., Koch, G. W., Schwartz, E., and Hungate, B. A.: Effect of temperature on metabolic activity of intact microbial communities: Evidence for altered metabolic pathway activity but not for increased maintenance respiration and reduced carbon use efficiency, Soil Biol. Biochem., 43, 2023–2031, 2011.
Duarte, C. M. and Prairie, Y. T.: Prevalence of heterotrophy and atmospheric CO2 emissions from aquatic ecosystems, Ecosystems, 8, 862–870, https://doi.org/10.1007/s10021-005-0177-4, 2005.
Ducklow, H. W., Steinberg, D. K., and Buesseler, K. O.: Upper ocean carbon export and the biological pump, Oceanography, 14, 50–58, 2001.
Dunne, J. P., Armstrong, R. A., Gnanadesikan, A., and Sarmiento, J. L.: Empirical and mechanistic models for the particle export ratio, Global Biogeochem. Cy., 19, GB4026, https://doi.org/10.1029/2004gb002390, 2005.
Ekblad, A., Wallander, H., Godbold, D. L., Cruz, C., Johnson, D., Baldrian, P., Bjork, R. G., Epron, D., Kieliszewska-Rokicka, B., Kjoller, R., Kraigher, H., Matzner, E., Neumann, J., and Plassard, C.: The production and turnover of extramatrical mycelium of ectomycorrhizal fungi in forest soils: role in carbon cycling, Plant Soil, 366, 1–27, https://doi.org/10.1007/s11104-013-1630-3, 2013.
Ettema, C. H. and Wardle, D. A.: Spatial soil ecology, Trend. Ecol. Evol., 17, 177–183, 2002.
Falkowski, P. G.: 10.5 – Biogeochemistry of Primary Production in the Sea, in: Treatise on Geochemistry edited by: Holland, H. and Turekian, K. K., Elsevier, Oxford, UK, 163–187, 2014.
Feng, X., Ackerly, D. D., Dawson, T. E., Manzoni, S., Skelton, R. P., Vico, G., and Thompson, S. E.: The ecohydrological context of drought and classification of plant responses, Ecol. Lett., in press, https://doi.org/10.1111/ele.13139, 2018.
Fernandez-Martinez, M., Vicca, S., Janssens, I. A., Sardans, J., Luyssaert, S., Campioli, M., Chapin Iii, F. S., Ciais, P., Malhi, Y., Obersteiner, M., Papale, D., Piao, S. L., Reichstein, M., Roda, F., and Penuelas, J.: Nutrient availability as the key regulator of global forest carbon balance, Nat. Clim. Change, 4, 471–476, https://doi.org/10.1038/nclimate2177, 2014.
Frey, S. D., Gupta, V., Elliott, E. T., and Paustian, K.: Protozoan grazing affects estimates of carbon utilization efficiency of the soil microbial community, Soil Biol. Biochem., 33, 1759–1768, 2001.
Frey, S. D., Lee, J., Melillo, J. M., and Six, J.: The temperature response of soil microbial efficiency and its feedback to climate, Nat. Clim. Change, 3, 395–398, https://doi.org/10.1038/nclimate1796, 2013.
Frouz, J., Liveckova, M., Albrechtova, J., Chronakova, A., Cajthaml, T., Pizl, V., Hanel, L., Stary, J., Baldrian, P., Lhotakova, Z., Simackova, H., and Cepakova, S.: Is the effect of trees on soil properties mediated by soil fauna? A case study from post-mining sites, Forest Ecol. Manag., 309, 87–95, https://doi.org/10.1016/j.foreco.2013.02.013, 2013a.
Frouz, J., Thebault, E., Pizl, V., Adl, S., Cajthaml, T., Baldrian, P., Hanel, L., Stary, J., Tajovsky, K., Materna, J., Novakova, A., and de Ruiter, P. C.: Soil Food Web Changes during Spontaneous Succession at Post Mining Sites: A Possible Ecosystem Engineering Effect on Food Web Organization?, Plos One, 8, e79694, https://doi.org/10.1371/journal.pone.0079694, 2013b.
Geyer, K. M., Kyker-Snowman, E., Grandy, A. S., and Frey, S. D.: Microbial carbon use efficiency: accounting for population, community, and ecosystem-scale controls over the fate of metabolized organic matter, Biogeochemistry, 127, 173–188, https://doi.org/10.1007/s10533-016-0191-y, 2016.
Gifford, R. M.: Whole plant respiration and photosynthesis of wheat under increased CO2 concentration and temperature: Long-term vs short-term distinctions for modelling, Glob. Change Biol., 1, 385–396, https://doi.org/10.1111/j.1365-2486.1995.tb00037.x, 1995.
Gifford, R. M.: Plant respiration in productivity models: conceptualisation, representation and issues for global terrestrial carbon-cycle research, Funct. Plant Biol., 30, 171–186, https://doi.org/10.1071/fp02083, 2003.
Golley, F. B.: Energy values of ecological materials, Ecology, 42, 581–584, https://doi.org/10.2307/1932247, 1961.
Grossart, H. P. and Ploug, H.: Microbial degradation of organic carbon and nitrogen on diatom aggregates, Limnol. Oceanogr., 46, 267–277, https://doi.org/10.4319/lo.2001.46.2.0267, 2001.
Hagerty, S. B., van Groenigen, K. J., Allison, S. D., Hungate, B. A., Schwartz, E., Koch, G. W., Kolka, R. K., and Dijkstra, P.: Accelerated microbial turnover but constant growth efficiency with warming in soil, Nat. Clim. Change, 4, 903–906, https://doi.org/10.1038/nclimate2361, 2014.
Hedges, J. I., Hu, F. S., Devol, A. H., Hartnett, H. E., Tsamakis, E., and Keil, R. G.: Sedimentary organic matter preservation: A test for selective degradation under oxic conditions, Am. J. Sci., 299, 529–555, https://doi.org/10.2475/ajs.299.7-9.529, 1999.
Hessen, D. O., Agren, G. I., Anderson, T. R., Elser, J. J., and De Ruiter, P. C.: Carbon, sequestration in ecosystems: The role of stoichiometry, Ecology, 85, 1179–1192, 2004.
Hobbie, E. A.: Carbon allocation to ectomycorrhizal fungi correlates with belowground allocation in culture studies, Ecology, 87, 563–569, https://doi.org/10.1890/05-0755, 2006.
Hoellein, T. J., Bruesewitz, D. A., and Richardson, D. C.: Revisiting Odum (1956): A synthesis of aquatic ecosystem metabolism, Limnol. Oceanogr., 58, 2089–2100, https://doi.org/10.4319/lo.2013.58.6.2089, 2013.
Huntingford, C., Atkin, O. K., Martinez-de la Torre, A., Mercado, L. M., Heskel, M. A., Harper, A. B., Bloomfield, K. J., O'Sullivan, O. S., Reich, P. B., Wythers, K. R., Butler, E. E., Chen, M., Griffin, K. L., Meir, P., Tjoelker, M. G., Turnbull, M. H., Sitch, S., Wiltshire, A., and Malhi, Y.: Implications of improved representations of plant respiration in a changing climate, Nat. Commun., 8, 1602, https://doi.org/10.1038/s41467-017-01774-z, 2017.
Hutsch, B. W., Augustin, J., and Merbach, W.: Plant rhizodeposition – an important source for carbon turnover in soils, J. Plant Nutr. Soil Sci., 165, 397–407, 2002.
Jiao, N., Robinson, C., Azam, F., Thomas, H., Baltar, F., Dang, H., Hardman-Mountford, N. J., Johnson, M., Kirchman, D. L., Koch, B. P., Legendre, L., Li, C., Liu, J., Luo, T., Luo, Y. W., Mitra, A., Romanou, A., Tang, K., Wang, X., Zhang, C., and Zhang, R.: Mechanisms of microbial carbon sequestration in the ocean – future research directions, Biogeosciences, 11, 5285–5306, https://doi.org/10.5194/bg-11-5285-2014, 2014.
Kaiser, K. and Kalbitz, K.: Cycling downwards – dissolved organic matter in soils, Soil Biol. Biochem., 52, 29–32, https://doi.org/10.1016/j.soilbio.2012.04.002, 2012.
Kallenbach, C. M., Frey, S. D., and Grandy, A. S.: Direct evidence for microbial-derived soil organic matter formation and its ecophysiological controls, Nat. Commun., 7, 13630, https://doi.org/10.1038/ncomms13630, 2016.
Krausmann, F., Erb, K. H., Gingrich, S., Haberl, H., Bondeau, A., Gaube, V., Lauk, C., Plutzar, C., and Searchinger, T. D.: Global human appropriation of net primary production doubled in the 20th century, P. Nat. Acad. Sci. USA, 110, 10324–10329, https://doi.org/10.1073/pnas.1211349110, 2013.
Kyaschenko, J., Clemmensen, K. E., Karltun, E., and Lindahl, B. D.: Below-ground organic matter accumulation along a boreal forest fertility gradient relates to guild interaction within fungal communities, Ecol. Lett., 20, 1546–1555, https://doi.org/10.1111/ele.12862, 2017.
Ladd, J. N., Jocteurmonrozier, L., and Amato, M.: Carbon turnover and nitrogen transformations in an alfisol and vertisol amended with 14C[U]glucose and 15N ammonium sulfate, Soil Biol. Biochem., 24, 359–371, https://doi.org/10.1016/0038-0717(92)90196-5, 1992.
Lipson, D. A.: The complex relationship between microbial growth rate and yield and its implications for ecosystem processes, Front. Microbiol., 6, 615, https://doi.org/10.3389/fmicb.2015.00615, 2015.
Luyssaert, S., Inglima, I., Jung, M., Richardson, A. D., Reichstein, M., Papale, D., Piao, S. L., Schulzes, E. D., Wingate, L., Matteucci, G., Aragao, L., Aubinet, M., Beers, C., Bernhofer, C., Black, K. G., Bonal, D., Bonnefond, J. M., Chambers, J., Ciais, P., Cook, B., Davis, K. J., Dolman, A. J., Gielen, B., Goulden, M., Grace, J., Granier, A., Grelle, A., Griffis, T., Grunwald, T., Guidolotti, G., Hanson, P. J., Harding, R., Hollinger, D. Y., Hutyra, L. R., Kolar, P., Kruijt, B., Kutsch, W., Lagergren, F., Laurila, T., Law, B. E., Le Maire, G., Lindroth, A., Loustau, D., Malhi, Y., Mateus, J., Migliavacca, M., Misson, L., Montagnani, L., Moncrieff, J., Moors, E., Munger, J. W., Nikinmaa, E., Ollinger, S. V., Pita, G., Rebmann, C., Roupsard, O., Saigusa, N., Sanz, M. J., Seufert, G., Sierra, C., Smith, M. L., Tang, J., Valentini, R., Vesala, T., and Janssens, I. A.: CO2 balance of boreal, temperate, and tropical forests derived from a global database, Glob. Change Biol., 13, 2509–2537, https://doi.org/10.1111/j.1365-2486.2007.01439.x, 2007.
Luyssaert, S., Inglima, I., and Jung, M.: Global Forest Ecosystem Structure and Function Data For Carbon Balance Research, ORNL DAAC, Oak Ridge, Tennessee, USA, 2009.
Manzoni, S., Trofymow, J. A., Jackson, R. B., and Porporato, A.: Stoichiometric controls dynamics on carbon, nitrogen, and phosphorus in decomposing litter, Ecol. Monogr., 80, 89–106, 2010.
Manzoni, S., Taylor, P. G., Richter, A., Porporato, A., and Ågren, G. I.: Environmental and stoichiometric controls on microbial carbon-use efficiency in soils, New Phytol., 196, 79–91, https://doi.org/10.1111/j.1469-8137.2012.04225.x, 2012.
Manzoni, S., Čapek, P., Mooshammer, M., Lindahl, B. D., Richter, A., and Šantrůčková, H.: Optimal metabolic regulation along resource stoichiometry gradients, Ecol. Lett., 20, 1182–1191, https://doi.org/10.1111/ele.12815, 2017.
Manzoni, S., Čapek, P., Porada, P., Thurner, M., Winterdahl, M., Beer, C., Brüchert, V., Frouz, J., Herrmann, A. M., Lindahl, B. D., Lyon, S. W., Šantručková, H., Vico, G., and Way, D.: Carbon use efficiency from organisms to ecosystems, Bolin Centre Database, https://bolin.su.se/data/Manzoni-2018, last access: 3 October 3 2018.
MathWorks, T.: Natick, MA, 2011.
McNaughton, S. J., Oesterheld, M., Frank, D. A., and Williams, K. J.: Ecosystem-Level Patterns of Primary Productivity and Herbivory in Terrestrial Habitats, Nature, 341, 142–144, 1989.
Mendonça, R., Müller, R. A., Clow, D., Verpoorter, C., Raymond, P., Tranvik, L. J., and Sobek, S.: Organic carbon burial in global lakes and reservoirs, Nat. Commun., 8, 1694, https://doi.org/10.1038/s41467-017-01789-6, 2017.
Middelboe, M. and Sondergaard, M.: Bacterioplankton Growth Yield: Seasonal Variations and Coupling to Substrate Lability and beta-Glucosidase Activity, Appl. Environ. Microbiol., 59, 3916–3921, 1993.
Monod, J.: The growth of bacterial cultures, Ann. Rev. Microbiol., 3, 371–394, https://doi.org/10.1146/annurev.mi.03.100149.002103, 1949.
Öquist, M. G., Erhagen, B., Haei, M., Sparrman, T., Ilstedt, U., Schleucher, J., and Nilsson, M. B.: The effect of temperature and substrate quality on the carbon use efficiency of saprotrophic decomposition, Plant Soil, 414, 113–125, https://doi.org/10.1007/s11104-016-3104-x, 2017.
Payne, W. J.: Energy Yields and Growth of Heterotrophs, Ann. Rev. Microbiol., 24, 17–52, 1970.
Russell, J. B. and Cook, G. M.: Energetics of bacterial-growth - Balance of anabolic and catabolic reactions, Microbiol. Rev., 59, 48–62, 1995.
Šantrůčková, H., Picek, T., Tykva, R., Šimek, M., and Pavlů, B.: Short-term partitioning of C-14-U-glucose in the soil microbial pool under varied aeration status, Biol. Fert. Soils, 40, 386–392, https://doi.org/10.1007/s00374-004-0790-y, 2004.
Schimel, J. P. and Weintraub, M. N.: The implications of exoenzyme activity on microbial carbon and nitrogen limitation in soil: a theoretical model, Soil Biol. Biochem., 35, 549–563, 2003.
Schmidt, M. W. I., Torn, M. S., Abiven, S., Dittmar, T., Guggenberger, G., Janssens, I. A., Kleber, M., Kogel-Knabner, I., Lehmann, J., Manning, D. A. C., Nannipieri, P., Rasse, D. P., Weiner, S., and Trumbore, S. E.: Persistence of soil organic matter as an ecosystem property, Nature, 478, 49–56, 2011.
Seiter, K., Hensen, C., and Zabel, M.: Benthic carbon mineralization on a global scale, Global Biogeochem. Cy., 19, GB1010, https://doi.org/10.1029/2004gb002225, 2005.
Sinsabaugh, R. L., Manzoni, S., Moorhead, D. L., and Richter, A.: Carbon use efficiency of microbial communities: stoichiometry, methodology and modelling, Ecol. Lett., 16, 930–939, https://doi.org/10.1111/ele.12113, 2013.
Sinsabaugh, R. L., Shah, J. J. F., Findlay, S. G., Kuehn, K. A., and Moorhead, D. L.: Scaling microbial biomass, metabolism and resource supply, Biogeochemistry, 122, 175–190, https://doi.org/10.1007/s10533-014-0058-z, 2015.
Sinsabaugh, R. L., Moorhead, D. L., Xu, X., and Litvak, M. E.: Plant, microbial and ecosystem carbon use efficiencies interact to stabilize microbial growth as a fraction of gross primary production, New Phytol., 47, 777–780, https://doi.org/10.1111/nph.14485, 2017.
Six, J., Frey, S. D., Thiet, R. K., and Batten, K. M.: Bacterial and fungal contributions to carbon sequestration in agroecosystems, Soil Sci. Soc. Am. J., 70, 555–569, https://doi.org/10.2136/sssaj2004.0347, 2006.
Smith, N. G., Malyshev, S. L., Shevliakova, E., Kattge, J., and Dukes, J. S.: Foliar temperature acclimation reduces simulated carbon sensitivity to climate, Nat. Clim. Change, 6, 407–411, https://doi.org/10.1038/nclimate2878, 2016.
Sterner, R. W. and Elser, J. J.: Ecological stoichiometry. The biology of elements from molecules to the biosphere, Princeton University Press, Princeton and Oxford, 439 pp., 2002.
Stewart, C. E., Paustian, K., Conant, R. T., Plante, A. F., and Six, J.: Soil carbon saturation: concept, evidence and evaluation, Biogeochemistry, 86, 19–31, 2007.
Thiet, R. K., Frey, S. D., and Six, J.: Do growth yield efficiencies differ between soil microbial communities differing in fungal: bacterial ratios? Reality check and methodological issues, Soil Biol. Biochem., 38, 837–844, 2006.
Thornley, J. H. M. and Cannell, M. G. R.: Modelling the components of plant respiration: Representation and realism, Ann. Bot., 85, 55–67, 2000.
Toljander, Y. K., Lindahl, B. D., Holmer, L., and Hogberg, N. O. S.: Environmental fluctuations facilitate species co-existence and increase decomposition in communities of wood decay fungi, Oecologia, 148, 625–631, https://doi.org/10.1007/s00442-006-0406-3, 2006.
Unkovich, M., Baldock, J., and Forbes, M.: Variability in harvest index of grain crops and potential significance for carbon accounting: examples from Australian agriculture, edited by: Sparks, D. L., Adv. Agron., 105, 173–219, 2010.
van Bodegom, P.: Microbial maintenance: A critical review on its quantification, Microb. Ecol., 53, 513–523, https://doi.org/10.1007/s00248-006-9049-5, 2007.
Vicca, S., Luyssaert, S., Penuelas, J., Campioli, M., Chapin, F. S., III, Ciais, P., Heinemeyer, A., Hogberg, P., Kutsch, W. L., Law, B. E., Malhi, Y., Papale, D., Piao, S. L., Reichstein, M., Schulze, E. D., and Janssens, I. A.: Fertile forests produce biomass more efficiently, Ecol. Lett., 15, 520–526, https://doi.org/10.1111/j.1461-0248.2012.01775.x, 2012.
Vogel, S.: Exposing Life's Limits with Dimensionless Numbers, Physics Today, 51, 22–27, 1998.
Wang, Z. Q., Ji, M. F., Deng, J. M., Milne, R. I., Ran, J. Z., Zhang, Q., Fan, Z. X., Zhang, X. W., Li, J. T., Huang, H., Cheng, D. L., and Niklas, K. J.: A theoretical framework for whole-plant carbon assimilation efficiency based on metabolic scaling theory: a test case using Picea seedlings, Tree Physiol., 35, 599–607, https://doi.org/10.1093/treephys/tpv030, 2015.
Yamaguchi, J.: Respiration and the growth efficiency in relation to crop productivity, Journal of the Faculty of Agriculture, Hokkaido University, 59, 59–129, 1978.