the Creative Commons Attribution 4.0 License.
the Creative Commons Attribution 4.0 License.
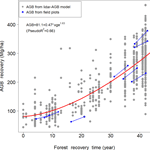
Forest aboveground biomass stock and resilience in a tropical landscape of Thailand
Nitin Kumar Tripathi
Wirong Chanthorn
Warren Brockelman
Anuttara Nathalang
Raphaël Pélissier
Siriruk Pimmasarn
Pierre Ploton
Nophea Sasaki
Salvatore G. P. Virdis
Maxime Réjou-Méchain
Half of Asian tropical forests were disturbed in the last century resulting in the dominance of secondary forests in Southeast Asia. However, the rate at which biomass accumulates during the recovery process in these forests is poorly understood. We studied a forest landscape located in Khao Yai National Park (Thailand) that experienced strong disturbances in the last century due to clearance by swidden farmers. Combining recent field and airborne laser scanning (ALS) data, we first built a high-resolution aboveground biomass (AGB) map of over 60 km2 of forest landscape. We then used the random forest algorithm and Landsat time series (LTS) data to classify landscape patches as non-forested versus forested on an almost annual basis from 1972 to 2017. The resulting chronosequence was then used in combination with the AGB map to estimate forest carbon recovery rates in secondary forest patches during the first 42 years of succession. The ALS-AGB model predicted AGB with an error of 14 % at 0.5 ha resolution (RMSE=45 Mg ha−1) using the mean top-of-canopy height as a single predictor. The mean AGB over the landscape was 291 Mg ha−1, showing a high level of carbon storage despite past disturbance history. We found that AGB recovery varies non-linearly in the first 42 years of the succession, with an increasing rate of accumulation through time. We predicted a mean AGB recovery rate of 6.9 , with a mean AGB gain of 143 and 273 Mg ha−1 after 20 and 40 years, respectively. This rate estimate is about 50 % larger than the rate prescribed for young secondary Asian tropical rainforests in the 2019 refinement of the 2006 IPCC guidelines for national greenhouse gas inventories. Our study hence suggests that the new IPCC rates, which were based on limited data from Asian tropical rainforests, strongly underestimate the carbon potential of forest regrowth in tropical Asia. Our recovery estimates are also within the range of those reported for the well-studied Latin American secondary forests under similar climatic conditions. This study illustrates the potential of ALS data not only for scaling up field AGB measurements but also for predicting AGB recovery dynamics when combined with long-term satellite data. It also illustrates that tropical forest landscapes that were disturbed in the past are of utmost importance for the regional carbon budget and thus for implementing international programs such as REDD+.
- Article
(1606 KB) -
Supplement
(432 KB) - BibTeX
- EndNote
Tropical forest disturbances and subsequent biomass recovery through time significantly affect the global carbon cycle (Harris et al., 2012). Although secondary forests in the tropics could constitute a major global carbon sink, the magnitude of such a sink remains poorly known (Chazdon, 2014; Lugo and Brown, 1992). A previous study estimated that 40 years of carbon storage in regenerating tropical forests from Latin America offset the past 19 years of carbon emissions from fossil fuels and industrial production in this region (Chazdon et al., 2016). Thus, there has been much interest in quantifying the ability of tropical secondary forests to sequester carbon in order to reduce uncertainties in the global carbon balance (e.g., Chai, 1997; Lohbeck et al., 2015; Stas et al., 2017).
Previous studies have used long-term forest plot surveys along chronosequences to quantify forest carbon dynamics in secondary tropical forests (Chazdon et al., 2007; N'Guessan et al., 2019; Norden et al., 2011, 2015; Poorter et al., 2016a; Rozendaal and Chazdon, 2015). Although long-term forest plots are essential for understanding the dynamics of tropical forests (Losos and Leigh, 2004), they are scarce, inherently labor-intensive, expensive and time-consuming to maintain, and not evenly distributed in the tropics. In addition, most studies of carbon dynamics along tropical forest successions are concentrated in Latin America (Chave et al., 2020; Letcher and Chazdon, 2009; Norden et al., 2015; Poorter et al., 2016a; Rozendaal et al., 2017; Rozendaal and Chazdon, 2015, but see N'Guessan et al., 2019, for Africa). They show high among-site variation in forest carbon recovery rates, suggesting a high context dependence (Chazdon et al., 2007; Norden et al., 2011, 2015), partly depending on climate conditions (Poorter et al., 2016a). A few pantropical studies have shown that the carbon potential of Latin American forests is smaller than that of Southeast Asian and African forests (Feldpausch et al., 2012; Sullivan et al., 2017). However, a recent study based on a compilation of published data throughout the pantropics surprisingly found that the forest carbon sequestration potential of Asian tropical secondary rainforest was in fact much lower than in American and African rainforests. This work led to a recent refinement of the 2006 IPCC guidelines for national greenhouse gas inventories (Requena Suarez et al., 2019; IPCC, 2019). Whether these new estimates are representative of Asian tropical rainforests is highly uncertain, due to a critical lack of data for this region. This issue is especially crucial for Asian tropical forests where half of the forests have been disturbed during the last century, resulting in the dominance of secondary forests throughout the region (Achard et al., 2014; Mitchard et al., 2013; Stibig et al., 2014).
Remote sensing technology has emerged as a promising tool for extrapolating local field carbon estimates over landscapes, regions, or at the global scale (Gibbs et al., 2007; Goetz et al., 2009). However, current long-term (>20-year) satellite data such as Landsat are weakly sensitive to forest carbon, especially in high-biomass forests (Ferraz et al., 2018; Lu, 2006; Meyer et al., 2019; Zheng et al., 2004). Yet, these data may be used to produce reliable land-cover classifications (e.g., forest versus non-forest areas; FAO, 2010). They allow for assessing the dynamics of deforestation and reforestation worldwide (Hansen et al., 2013) and can thus monitor disturbance history, particularly the time since abandonment of agriculture (Cohen et al., 1996; Masek and Collatz, 2006). However, the forest carbon dynamics associated with such deforestation and reforestation events remains highly uncertain due to the large uncertainties of global carbon maps (Mitchard et al., 2013, 2014; Réjou-Méchain et al., 2019).
On other hand, airborne laser scanning (ALS) provides accurate landscape-scale estimates of forest structural parameters (Maltamo et al., 2005; Næsset, 2002; Wulder et al., 2012). When calibrated with field-based estimates of aboveground biomass (AGB), ALS metrics can be used to produce high-resolution forest carbon maps, even for highly carbon-dense tropical forests (Asner et al., 2010; Cao et al., 2016; Ferraz et al., 2018; Kronseder et al., 2012; Labriere et al., 2018; Zhao et al., 2009; Zolkos et al., 2013). Multi-temporal ALS acquisitions may thus provide direct estimates of the carbon balance of tropical forest landscapes (Dubayah et al., 2010; Meyer et al., 2013; Réjou-Méchain et al., 2015). However, due to its relatively recent emergence, ALS technology cannot yet be used to investigate long-term dynamics directly (>10 years).
Combining long-term (>40-year) land cover change assessment from satellite data archives (e.g., Landsat) and contemporary lidar AGB maps may be a promising avenue for understanding long-term forest carbon dynamics. Such an approach has been successfully implemented in temperate and boreal forests (Bolton et al., 2015; Pflugmacher et al., 2012, 2014; White et al., 2018; Zald et al., 2014). However, to our knowledge, it has not been yet used to assess the forest carbon resilience of tropical forests (but see Helmer et al., 2009, who used satellite-based lidar).
In this study, we combined extensive field, ALS, and Landsat time series (LTS) data to assess the spatial variation of AGB and forest AGB dynamics of secondary forests in a Thai landscape. More specifically, we first calibrated a robust ALS-AGB model to produce a fine-scale AGB map at the landscape scale. We then used a random forest machine-learning algorithm to classify historical Landsat images from 1972 to 2017 into forest and non-forest classes. Using this information over time, we generated a cumulative forest gain map over a period of 42 years of succession. We finally combined this chronosequence with our ALS-AGB map to estimate the forest carbon resilience of secondary forests during the 42 first years after land abandonment.
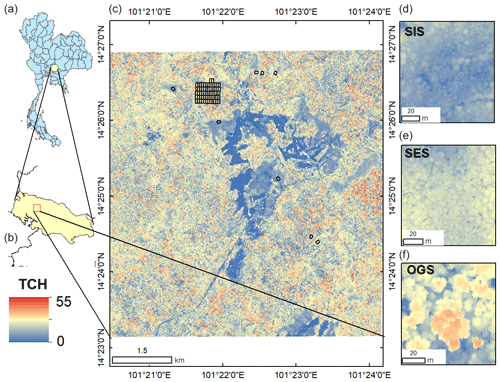
Figure 1Location of the study area in Thailand (a) and in the Khao Yai reserve (b). The central map (c) illustrates the lidar top of canopy height (TCH) in the study area at 1 m resolution and the location of the 70 studied plots (in black). Examples of the different stand development stages are illustrated (d–f; SIS: stand initiation stage; SES: stem exclusion stage; and OGS: old-growth stage).
2.1 Study area
The study area of ca. 6400 ha is part of Khao Yai National Park in central Thailand (14∘25′20.4′′ N, 101∘22′36.9′′ E; Fig. 1). Khao Yai is the first national park established in Thailand, in 1962. It is home to numerous endangered plant and animal species (Kitamura et al., 2004). The area receives approximately 2200 mm of precipitation annually, with a dry season of 5 to 6 months (precipitation below 100 mm month−1) from November to April (Brockelman et al., 2011; Chanthorn et al., 2016). The annual mean temperature is about 22–23 ∘C (Jenks et al., 2011), and the altitude of the study area varies from 650 to 870 m. Before establishment of the park, some areas were used for low-intensity agriculture activities that likely started at the end of the 19th century (Brockelman et al., 2011, 2017) and then naturally reforested at different times depending on when burning ceased (Chanthorn et al., 2016). As a consequence, the landscape constitutes a mosaic of secondary forests of different ages amidst old-growth forests (Chanthorn et al., 2016).
2.2 Field data
We used three sets of forest inventory plots with a total sample area of 35 ha (Fig. 1). First, a large 30 ha contiguous (500 m×600 m) forest dynamics plot, named Mo Singto, was established in old-growth forest after 1998 and completely censused in 2004–2005, 2010–2011 and 2016–2017. The census method follows the protocol of the Center for Tropical Forest Science (CTFS) network to which the plot belongs since 2009 (Brockelman et al., 2011). The second set of plots included eight separate 0.48 ha plots (60 m×80 m) that were established from March to May 2013 and re-censused from November 2017 to January 2018 (Chanthorn et al., 2017). These plots are set along a successional gradient varying from near stand initiation to old-growth forest. Lastly, a 1 ha plot (100 m×100 m) located near the north border of the 30 ha Mo Singto plot was established in a secondary forest in 2005 and then re-censused in 2010 and 2017. In all plots, trees ≥1 cm in diameter at breast height (dbh) were tagged, identified to species, mapped and measured for their diameter, except in the 0.48 ha plots where the minimum dbh was 4 cm. A total of 184 239 individual trees were measured across all the plots, from which 517 trees were measured for height using a pole for short trees (<5 m), a laser range finder (Nikon Forestry 550) for medium height trees (5–7 m) and a Vertex III hypsometer for tall (>7 m) trees (Chanthorn et al., 2017). In this paper, we used the 2017 census data, concomitant with the ALS campaign, to estimate AGB and multiple censuses to estimate the AGB dynamics of secondary plots. For the sake of homogeneity in tree measurements, we used only trees ≥5 cm in dbh in the whole data set.
In order to homogenize plot size, we subdivided all plots ≥1 ha into 0.5 ha subplots. This resulted in 70 plots of either 50 m×100 m (n=62) or 60 m×80 m (n=8) that we classified in three successional stages from young- to old-growth forests following the classification from Chanthorn et al. (2017): stand initiation (early) stage (SIS; n=3); stem exclusion (intermediate) stage (SES; n=5); and old-growth stage (OGS; n=62). Based on interviews of senior park rangers and using Landsat remote sensing data, Chanthorn et al. (2017) estimated that the ages were approximately 15–20 years for SIS forests, 35–40 years for SES forests and unknown but probably older than 200 years for OGS forests. This classification into successional stages followed the framework of Oliver and Larson (1996) who studied successional gradients in temperate forests. Although the original framework considered four successional stages, we did not find any area corresponding to the understory re-initiation stage in the study landscape, i.e., the stage following SES and preceding OGS. Most second-growth forests have regenerated since the Park was established about 50–60 years ago so that old second-growth forests, where understory re-initiation occurs, are very rare in this area. Note also that our study period (1972–2017; see below) cannot account for forests from the SES stage older than 40 years, e.g., that directly started regenerating at the establishment of the park in 1962, as suggested by some hand-drawn historical maps (Cumberlege and Cumberlege, 1963; Smitinand, 1968).
2.3 ALS data
The airborne laser scanning (ALS) campaign was conducted on 10 April 2017 over ca. 64 km2 (Fig. 1). The Asian Aerospace Services Limited company (Bangkok) acquired the ALS data with a RIEGL LMS Q680i installed into a Diamond Aircraft “Airborne Sensors” DA-42 fixed-wing airplane. The flying altitude was about 500–600 m above ground level with a 60∘ field of view, and a pulse repetition frequency of 400 kHz, for which the aircraft maintained an average ground speed of 185 km h−1 capturing the area of interest in 50 overlapping laser strips. We discretized the full waveform data for subsequent analyses resulting in an average point density of ca. 22 points m−2.
Post-processing of ALS data and point cloud classification into ground, vegetation or noise was done using TerraScan of Terrasolid Version 14. Points classified as ground were used to build a digital terrain model (DTM) at 1 m resolution using a k nearest-neighbor kriging approach implemented in the LidR R package (Roussel and Auty, 2017). A 1 m resolution canopy height model (CHM) was then computed from the height of the normalized vegetation points, discarding outliers classified as air or noise. Finally, we used the CHM and the normalized vegetation point cloud to derive different forest height metrics at the plot level (Table S1 in the Supplement).
2.4 Landsat data
We retrieved Landsat images (MSS, TM, OLI and TIRS products) for the study area from the Landsat archive (http://glovis.usgs.gov, last access: 17 April 2019) in the 1972–2017 period (WRS-1 138/50 and WRS-2 path/row: 129/50). To minimize the impact of clouds and potentially varying phenology within years, we mostly selected images acquired during the dry season, from November to March. We thus collected Landsat 1–3 MSS data (1972–1983), Landsat 4–5 TM (1984–2011) and Landsat 8 OLI & TIRS (2013–2017) data. We did not consider Landsat 7 ETM+ images due to the failure of the Scan Line Corrector, leading to data gaps. All Landsat images were already orthorectified and displayed an accurate co-registration with ALS data. Before 1984, Landsat MSS collected data at 60 m×60 m spatial resolution in most bands. Thus, to have consistent time series data, we aligned all the post-1983 Landsat data using a reference image from 1972 and aggregated each image to 60×60 m. Over the 44 years, we selected a total of 34 high-quality images, each representing 1 year. For the 11 missing years, we could not find cloud-free images and no image was available in 2012 since we discarded Landsat 7 ETM+ data.
2.5 Field aboveground biomass calculation
We estimated tree aboveground biomass (AGB) using a pantropical allometric model (Eq. 4 from Chave et al., 2014). This model uses the diameter (D), total tree height (H) and wood density (WD) as the predictors and was shown to hold across tropical vegetation types and regions. Wood density was estimated using species- (47 % of stems), genus- (50 %) or stand-averaged (3 %) values from the global wood density database (Chave et al., 2009; Zanne et al., 2009). Tree height was estimated through locally adjusted height–diameter (H-D) models of the form given in Eq. (1):
where a and b are model parameters to be adjusted and ε is a normally distributed error with mean 0 and standard error σlogH. Tree height was subsequently estimated using the back-transformation formula including a known bias correction (Baskerville, 1972) using following Eq. (2):
Because the H-D relationship varies along the successional gradient (Chanthorn et al., 2017), we fitted three independent H-D models for the three different successional growth forest stages using 177 measured trees for SIS plots, 159 for SES plots and 181 for OGS plots.
AGB at the plot level was then estimated in Mg ha−1 by summing individual tree AGB for all trees with dbh ≥5 cm belonging to the plot. We did all these analyses using the R BIOMASS package (Réjou-Méchain et al., 2017).
2.6 Lidar AGB model
We relied on a log–log model form given in Eq. (3) to model AGB from ALS data (Asner et al., 2012; Réjou-Méchain et al., 2015):
where L1, L2, … are the lidar metrics to be selected (see Table S1) and ε the error term assumed to be normally distributed with zero mean and residual standard error σlogL. Fitting the model with log-transformed variables allows us to model a multiplicative error and thus to account for higher model prediction error with larger AGB values (Zolkos et al., 2013). Using this model, we selected the most predictive lidar metrics from our full set of lidar metrics using a leave-one-out cross-validation (LOOCV) scheme nested within a forward selection procedure. The LOOCV consists of fitting models with all observations except one, and then using the model to predict the value of the observation held out of model calibration. The process is repeated for all observations so that model prediction accuracy, here the root mean squared error (RMSE), can be independently assessed from all observations. This LOOCV approach was repeated for different models following a forward procedure that begins by selecting the most discriminant variable according to the LOOCV-RMSE criterion. The procedure then continues by selecting the second most discriminant variable and so on. To minimize the problem of model overfitting, we only kept explanatory variables that contribute to a decrease in relative RMSE (RMSE divided by the mean observed AGB) by more than 1 %. The selected lidar-AGB model was then used to predict AGB values over the landscape at 60 m resolution, to match the resolution of Landsat images.
2.7 AGB recovery analysis
2.7.1 Forest and non-forest classification
To classify areas as forest or non-forest, we applied the random forest (RF) algorithm independently on each Landsat image to minimize inter-image classification error that may otherwise arise from instrumental (e.g., differences in sensors spectral characteristics) and phenological effects. We used all Landsat bands and their ratios as predictors in our RF classification models, i.e., the four raw bands for Landsat 1–3 MSS data (1972–1983), the seven raw bands for Landsat 4–5 TM (1984–2011) and the nine raw bands for Landsat 8 OLI & TIRS (2013–2017). The normalized difference vegetation index (NDVI) was additionally used as a predictor for all the sensors while the normalized burn ratio (NBR) was only used for Landsat 4–5 and Landsat 8 due to non-availability of short-wave infrared (SWIR) bands in MSS sensors. Thus, we used 18 predictors for MSS, 51 predictors for TM and 83 for OLI & TIRS as an input for the RF algorithm. RF model for each year of the study period was then trained on the same set of pixels that likely remained either forested or non-forested from 1972 up until 2017. This training data set was built using the 2017 ALS data. We first aggregated the 1 m lidar-derived CHM at the same resolution as the Landsat images (60 m resolution) and defined non-forest pixels as pixels having a mean top of canopy height of <5 m (FAO, 2012; Sasaki and Putz, 2009). Because 60 m-scale deforestation is unlikely to have occurred in the area since the establishment of the national park in 1962, areas that were classified as non-forest with the 2017 lidar data very likely corresponded to non-forested areas during the whole study period. By contrast, we defined as forested areas all 60 m pixels that had a lidar mean top of canopy height of >30 m because these tall forests very likely corresponded to forested areas during the whole study period. We thus used a reference set of 400 60 m pixels classified as non-forest and 110 as forest. This data set was then randomly divided into a training data set (60 %) to calibrate the RF models and a validation data set (40 %) to assess the accuracy of the forest and non-forest classification. We only considered classified pixels that had a post-probability of assignment >90 % in the RF outputs (Pickell et al., 2016; White et al., 2018) and calculated the classification accuracy as the proportion of pixels that were correctly classified as forest or non-forest in the validation data set. This statistical analysis was done using the randomForest R package (Liaw and Wiener, 2002).
2.7.2 Forest AGB recovery analysis
We combined time-series-classified Landsat images with the 60 m resolution lidar AGB map to quantify AGB recovery as a function of time. We used classified time series data to assign to each pixel the last date at which a shift from a non-forest to forest status occurred during the study period. Thus, all pixels that did not experience any shift, i.e., that remained non-forested or forested during the whole study period, were discarded from this analysis. To minimize false detection of land cover change due, for example, to atmospheric pollution, we only considered shifts that entailed land cover change for at least two consecutive images. Thus, we did not consider any shift before 1975 because, to be considered, the non-forest to forest shift of a pixel should occur after being classified as non-forest in the two previous images (in our case in 1972 and 1973). Finally, we also discarded pixels that underwent more than four different shifts during the whole study period because numerous shifts are likely to indicate areas prone to forest degradation, e.g., close to human occupancy areas such as roads, introducing a bias in our inferences on the natural successional dynamics. We thus assigned to each pixel the year of the last non-forest to forest shift, if any, and considered this year as the forest recovery starting time. The AGB predicted by the lidar AGB map in 2017 was then used to estimate how much AGB was stored between the forest recovery starting time and 2017 through a non-linear power model.
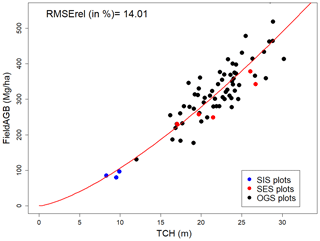
Figure 2Lidar-AGB model showing the relationship between field-derived plot AGB and the lidar top-of-canopy height (TCH) at 0.5 ha resolution. The power model is illustrated by the red line, and the points represent the field plot AGB estimates at different successional stages: stand initiation (early) stage (SIS; n=3); stem exclusion (intermediate) stage (SES; n=5); old-growth stage (OGS; n=62) according to the classification by Chanthorn et al. (2017).
3.1 Forest biomass stocks
Field plots AGB ranged from 80 to 577 Mg ha−1 (mean of 315 Mg ha−1), with a mean AGB of SIS, SES and OGS plots of 87, 291 and 328 Mg ha−1, respectively. Among all the lidar metrics, the mean of top-of-canopy height (TCH, defined as the maximum height of 1 m resolution pixels) was the best predictor of field AGB estimates with a relative RMSE of 14 % (RMSE=45 Mg ha−1; R2=0.85) at 0.5 ha scale (Fig. 2). Adding a second predictor did not reduce the relative RMSE by more than 1 % (Table S2). We thus kept TCH as a single predictor for our analyses resulting in the Eq. (4) for the lidar-AGB model:
Using this lidar-AGB model, we predicted AGB over the whole landscape (Fig. 3a). The distribution of AGB values over the landscape was not normally distributed due to an over-representation of pixels with low AGB values. At the landscape scale, predicted AGB ranged from 0 to 681 Mg ha−1 with a mean of 291 Mg ha−1 (Fig. 3b), close to the mean AGB of the field plots.
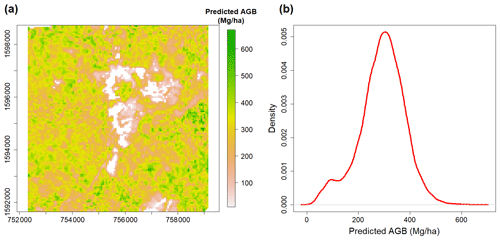
Figure 3Lidar-AGB map and the distribution of AGB values over the landscape at 60 m resolution. (a) Spatial distribution of AGB predicted from the lidar-AGB inversion model over the study area; (b) density distribution of predicted AGB over the landscape.
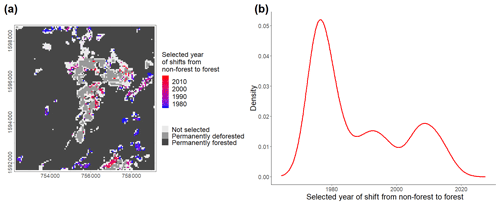
Figure 4Landsat time-series-derived map showing non-forest-to-forest change over the study area. (a) Map showing spatialized-selected pixel shifts from non-forests to forests over the years. The shade gradient represents pixels that did not experience any shift (permanently forested or deforested) and pixels that experienced a shift but that did not pass our quality procedure during the study period (not selected). (b) Density distribution of selected pixel shifts over the landscape during the study period.
3.2 AGB recovery analysis
Our forest and non-forest classification through time was highly accurate, with 90 % to 99 % of well-classified validation pixels in individual classified images (Table S3, Figs. S1–S2 in the Supplement). Figure 4a illustrates the resulting spatialized time series of non-forest-to-forest shifts over the study area and showed that most (83 %) of the landscape did not experience such a shift at 60 m resolution. About 78 % and 5 % of the study area remained permanently forested and non-forested over the 42-year study period, respectively. Most of the stable non-forested areas correspond to National Park building areas, including tourist shops and guesthouses or to continuously cleared areas such as camping locations. Over the 17 % remaining pixels that experienced a shift, we concentrated our analyses on the 4 % pixels (n=550; ca. 198 ha) that passed our selection procedure and that were well distributed over the landscape (Fig. 4b).
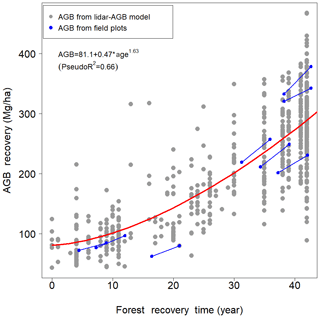
Figure 5Relationship between forest biomass estimated from a lidar-AGB model and forest recovery time estimated from a time series of classified Landsat images (grey dots). The fitted power model is represented by the red line. Blue lines and dots represent the AGB directly estimated from eight field plots (same plots are joined by a line) in 2013 and in 2017/18 and for which the forest recovery time was inferred from Landsat-derived forest age (Fig. S5).
Considering the selected pixels that experienced a shift from non-forest to forest, we found that AGB accumulated non-linearly through time during the 42 first years of the succession (Fig. 5). A simple power model led to a pseudo-R2 of 0.66 and a power exponent of greater than 1, indicating an increase in the rate of AGB accumulation with recovery time. This model predicts an AGB gain of 143 Mg ha−1 after 20 years of recovery and of 273 Mg ha−1 after 40 years (spatialized gain in AGB is shown in Fig. S3). Using field AGB estimates at two different census dates from eight secondary forest plots that started regenerating during the study period (see Fig. S5), we showed that the observed rate of AGB accumulation was similar to the one predicted by our model and also tended to increase with forest age (in blue dots in Fig. 5). Finally, focusing on the 17 % pixels that experienced at least one shift from non-forest to forest since 1972, we estimated that the study area has stored a minimum AGB of 455 Gg, as observed in the 2017 lidar AGB map, equivalent to 214 Gg C during the study period.
In this study, we showed that the integration of field inventory, Landsat archives and lidar data provides a powerful approach for characterizing the spatio-temporal dynamics of aboveground biomass in tropical forests. While the carbon stocks and recovery potential of Southeast Asian tropical forests are globally poorly known, our approach contributes to a better understanding of the role of these forests in global carbon dynamics. We specifically showed that our study site stores a large amount of carbon, despite its disturbance history, and acts as a strong carbon sink, through secondary succession pathways.
4.1 Spatial variation in AGB
Using extensive field data, we have shown that forest AGB can be predicted with an error of 14 % at a 0.5 ha resolution using a single lidar metric, the mean top-of-canopy-height (TCH), a metric previously identified as a robust predictor of AGB (Asner and Mascaro, 2014). This error typically falls within the range of expected errors at this resolution (Zolkos et al., 2013). Using a robust metric selection approach, we showed that adding any other lidar metrics did not bring any additional information and that our single predictor did not show any saturation for large AGB values. Many studies have used a combination of several lidar metrics selected through less robust approaches, i.e., not through independent validation approaches such as our LOOCV procedure, potentially generating overfitting problems (Junttila et al., 2015). We here confirm, similarly to Asner et al. (2012) and Réjou-Méchain et al. (2015), that simple parsimonious models should be preferred, at least within a given tropical forest landscape. Due to a limited number of field plots in low-biomass areas, we were, however, unable to test whether model prediction error varied with forest stand AGB.
Using this lidar model, we predicted a mean AGB over the landscape of 291 Mg ha−1, corresponding to a carbon density of 137 Mg C ha−1 (using a ratio of biomass to carbon conversion of 0.47; Thomas and Martin, 2012). Using a large network of field plots, a recent pantropical study suggested that Southeast Asian and African forests store significantly more carbon than Amazonian forests (Sullivan et al., 2017). However, in this latter study, Southeast Asian forests were only represented by field data from Indonesia and Malaysia where trees are known to be particularly tall (Coomes et al., 2017; Feldpausch et al., 2011; Jucker et al., 2018). Here, we found that our study forests stored significantly less carbon than forests in Indonesia and Malaysia, where the mean carbon density reached ca. 200 Mg C ha−1 (Sullivan et al., 2017), but as much as in Amazonian forests (mean of 140 Mg C ha−1; Sullivan et al., 2017), even when considering only old-growth forest plots. Whether the relatively low carbon density of our study site, compared to other Southeast Asian forests, is specific to our study area or representative of other Southeast Asian forests should be further investigated.
We found a very high spatial heterogeneity of AGB at the landscape scale with an apparent over-representation of low AGB values. This is most probably the consequence of past human activities in this area up to the establishment of the park that led to the present mosaic of secondary and mature forests. This result indicates that this area is currently likely to be a net carbon sink.
4.2 AGB recovery through time
Combining classified images obtained from LTS and lidar data, we quantified the recovery rate of forests after land abandonment. As expected, we showed a significant increase of AGB with recovery time. After 20 years of recovery, our model predicts an AGB accumulation of 143 Mg ha−1, an estimate slightly higher than the one predicted by Poorter et al. (2016a) in Neotropical secondary forests (122 Mg ha−1). However, this difference can partly be explained by the inclusion of trees between 5 and 10 cm dbh in our study, contrary to the study of Poorter et al. (2016b). AGB accumulation in our study corresponds to a net carbon uptake of 3.4 for the first 20 years. This rate of carbon accumulation is close to the pantropical estimate from Silver et al. (2000) and is similar to the default continent recovery rates given by the previous 2006 IPCC guidelines for national greenhouse gas inventories (IPCC, 2006). However, the 2019 refinement of these guidelines halved the recovery rate estimate for young Asian secondary rainforest (≤20 years; Requena Suarez et al., 2019; IPCC, 2019), suggesting that young secondary forests in Asia store carbon at a much lower rate than in Latin America or in Africa. This new estimate is derived from a very limited data set (seven chronosequences) that may not be representative of Asian tropical rainforests. Besides, these data included very small field plots (≤0.01 ha in size; Hiratsuka et al., 2006; Ewel et al., 1983), potentially leading to important sampling errors (Réjou-Méchain et al., 2014). Given the serious implications of these updated IPCC default rates for Asian countries, we here call for further testing of these new IPCC rates across tropical Asia.
Our model showed that a non-linear power model with an exponent of >1 best fit our data, suggesting an increase in the rate of carbon accumulation during the first 42 years of succession. Contrary to the results found by Feldpausch et al. (2007), the rates of AGB accumulation inferred with our approach provided estimates similar to those obtained from long-term field plot surveys (Fig. 5), validating the chronosequence approach in our study area. Assuming that the carbon recovery rate rapidly decreases after 50–60 years (Brown and Lugo, 1990; Silver et al., 2000), our result suggests a sigmoid relationship of AGB accumulation with time in our study area. Previous studies have shown different models of AGB accumulation with forest age. Saldarriaga et al. (1988) showed that the AGB of Neotropical forests from the upper Rio Negro increased linearly with stand age during the 40 years, while Jepsen (2006) reported a sigmoidal increase in AGB accumulation in Sarawak, Malaysia, as is likely the case in our study area. Finally, working on 41 Neotropical sites, Poorter et al. (2016a) assumed a logarithmic trend in the AGB accumulation over time, hence a decrease of the rate of carbon accumulation through time, probably because they investigated a longer time period. Selecting the sites of Poorter et al. (2016a, b) that had at least 10 observations over the first 44 years (n=21 out of 28 sites, i.e., excluding 7 sites for which model fitting was not possible), site-specific power models revealed that two-thirds of the sites displayed a power exponent of <1 and one-third showed an exponent of >1 (Fig. S4). Thus, the accumulation of AGB with age follows different trends across sites, as already highlighted in previous studies (Kennard et al., 2002; Poorter et al., 2016a; Ray and Brown, 2006; Ruiz et al., 2005; Silver et al., 2000; Toledo and Salick, 2006). Understanding how these trends vary according to abiotic factors (e.g., soil type, rainfall), species assemblage and diversity, and prioritizing effects such as types of land use and land management existing before forest recolonization, constitute an important avenue of research (Chazdon, 2014; McMahon et al., 2019).
Our analysis was based on a forest/non-forest classification through time and our independent validation suggested high overall accuracy (90 % to 99 %), similar to that reported by other studies using Landsat data classification in boreal systems (Bolton et al., 2015; White et al., 2018). Furthermore, our estimate of forest age using this approach was highly consistent with our expectations. Indeed, using our forest plots, we found that the SES and SIS forest stages lasted on average 40 years (range 38–42) and 13 years (range 8–20), respectively, hence very close to suggestions of Chanthorn et al. (2017; Fig. S5). However, our overall approach cannot be replicated easily in human-occupied areas. Indeed, human disturbances lead to forest degradation that, in contrast to deforestation, is not captured by the Landsat signal, so that, when combined with a reference AGB map, natural carbon recovery potential could be seriously underestimated. Because our study area was protected from human disturbances during the study period, we were in very favorable conditions to estimate forest carbon recovery rates and strongly encourage researchers benefiting from similar conditions to replicate our analyses in other study sites.
Our study demonstrates that combining field, lidar and long-term satellite data provides an efficient way to assess forest carbon recovery rates during secondary successions. We showed that it produces similar estimates as those inferred from long-term field plots, but at a much lower cost and within a much shorter time frame. Replicating this approach in other protected tropical landscapes, notably in the Asian subcontinent, would thus considerably increase the representativeness of forest carbon recovery rates. This would improve our understanding of the environmental and historical drivers of these varying rates between ecological zones and continents. This is especially important in Southeast Asian forests that constitute a hotspot of biodiversity and carbon, and that are under threat due to the fast changing of both the environment and socioeconomics in this region. Quantifying the rates at which different forest types accumulate carbon should thus stay at the forefront of the research agenda and would greatly benefit the Earth system model community and international policy initiatives such as REDD+.
Code and data are available upon request to the corresponding author.
The supplement related to this article is available online at: https://doi.org/10.5194/bg-17-121-2020-supplement.
NJ, NKT and MRM designed the study; NJ and MRM analyzed the data and wrote the first draft of the paper; WC, WB and AN provided field data. All authors provided valuable feedback on analyses and the manuscript.
The authors declare that they have no conflict of interest.
We gratefully thank the National Science and Technology Development Agency (Thailand) for supporting long-term monitoring of all forest plots and the Department of National Parks, Wildlife and Plant Conservation (DNP) that supported our research. Nidhi Jha benefitted from the French Eiffel Excellence Scholarship Program and a mobility grant from the IRD institute in the AMAP laboratory (9 months).
This study was supported by the project AIT/SET – 2016 – R011 sponsored by the French Ministry of Foreign Affairs and International Development initiated during the Regional Forum on Climate Change. Raphaël Pélissier, Pierre Ploton and Maxime Réjou-Méchain were supported by the project 3DForMod funded by ERA-GAS (grant no. ANR-17-EGAS-0002-01).
This paper was edited by Anja Rammig and reviewed by Rico Fischer and two anonymous referees.
Achard, F., Beuchle, R., Mayaux, P., Stibig, H.-J., Bodart, C., Brink, A., Carboni, S., Desclée, B., Donnay, F., Eva, H. D., Lupi, A., Raši, R., Seliger, R., and Simonetti, D.: Determination of tropical deforestation rates and related carbon losses from 1990 to 2010, Glob. Change Biol., 20, 2540–2554, https://doi.org/10.1111/gcb.12605, 2014.
Asner, G. P. and Mascaro, J.: Mapping tropical forest carbon: Calibrating plot estimates to a simple LiDAR metric, Remote Sens. Environ., 140, 614–624, https://doi.org/10.1016/j.rse.2013.09.023, 2014.
Asner, G. P., Powell, G. V. N., Mascaro, J., Knapp, D. E., Clark, J. K., Jacobson, J., Kennedy-Bowdoin, T., Balaji, A., Paez-Acosta, G., Victoria, E., Secada, L., Valqui, M., and Hughes, R. F.: High-resolution forest carbon stocks and emissions in the Amazon, P. Natl. Acad. Sci. USA, 107, 16738–16742, https://doi.org/10.1073/pnas.1004875107, 2010.
Asner, G. P., Mascaro, J., Muller-Landau, H. C., Vieilledent, G., Vaudry, R., Rasamoelina, M., Hall, J. S., and van Breugel, M.: A universal airborne LiDAR approach for tropical forest carbon mapping, Oecologia, 168, 1147–1160, https://doi.org/10.1007/s00442-011-2165-z, 2012.
Baskerville, G. L.: Use of Logarithmic Regression in the Estimation of Plant Biomass, Can. J. For. Res., 2, 49–53, https://doi.org/10.1139/x72-009, 1972.
Bolton, D. K., Coops, N. C., and Wulder, M. A.: Characterizing residual structure and forest recovery following high-severity fire in the western boreal of Canada using Landsat time-series and airborne lidar data, Remote Sens. Environ., 163, 48–60, https://doi.org/10.1016/j.rse.2015.03.004, 2015.
Brockelman, W. Y., Nathalang, A., and Gale, G. A.: The Mo Singto forest dynamics plot, Khao Yai National Park, Thailand, Nat. Hist. Bull. Siam Soc., 57, 35–55, 2011.
Brockelman, W. Y., Nathalang, A., and Maxwell, J. F.: Mo Singto Plot: Flora and Ecology, National Science and Technology Development Agency, and Department of National Parks, Wildlife and Plant Conservation, Bangkok, 2017.
Brown, S. and Lugo, A. E.: Tropical secondary forests, J. Trop. Ecol., 6, 1–32, https://doi.org/10.1017/S0266467400003989, 1990.
Cao, L., Coops, N. C., Innes, J. L., Dai, J., Ruan, H., and She, G.: Tree species classification in subtropical forests using small-footprint full-waveform LiDAR data, Int. J. Appl. Earth Obs. Geoinformation, 49, 39–51, https://doi.org/10.1016/j.jag.2016.01.007, 2016.
Chai, F. Y. C.: Above-Ground biomass estimation of a secondary forest in Sarawak, J. Trop. For. Sci., 9, 359–368, 1997.
Chanthorn, W., Ratanapongsai, Y., Brockelman, W. Y., Allen, M. A., Favier, C., and Dubois, M. A.: Viewing tropical forest succession as a three-dimensional dynamical system, Theor. Ecol., 9, 163–172, https://doi.org/10.1007/s12080-015-0278-4, 2016.
Chanthorn, W., Hartig, F., and Brockelman, W. Y.: Structure and community composition in a tropical forest suggest a change of ecological processes during stand development, For. Ecol. Manag., 404, 100–107, https://doi.org/10.1016/j.foreco.2017.08.001, 2017.
Chave, J., Coomes, D., Jansen, S., Lewis, S. L., Swenson, N. G., and Zanne, A. E.: Towards a worldwide wood economics spectrum, Ecol. Lett., 12, 351–366, https://doi.org/10.1111/j.1461-0248.2009.01285.x, 2009.
Chave, J., Réjou-Méchain, M., Búrquez, A., Chidumayo, E., Colgan, M. S., Delitti, W. B. C., Duque, A., Eid, T., Fearnside, P. M., Goodman, R. C., Henry, M., Martínez-Yrízar, A., Mugasha, W. A., Muller-Landau, H. C., Mencuccini, M., Nelson, B. W., Ngomanda, A., Nogueira, E. M., Ortiz-Malavassi, E., Pélissier, R., Ploton, P., Ryan, C. M., Saldarriaga, J. G., and Vieilledent, G.: Improved allometric models to estimate the aboveground biomass of tropical trees, Glob. Change Biol., 20, 3177–3190, https://doi.org/10.1111/gcb.12629, 2014.
Chave, J., Piponiot, C., Maréchaux, I., de Foresta, H., Larpin, D., Fischer, F. J., Derroire, G., Vincent, G., and Hérault, B.: Slow rate of secondary forest carbon accumulation in the Guianas compared with the rest of the Neotropics, Ecol. Appl., 30, e02004, https://doi.org/10.1002/eap.2004, 2020.
Chazdon, R. L.: Second Growth: The Promise of Tropical Forest Regeneration in an Age of Deforestation, University of Chicago Press, Chicago, Illinois, United States, 2014.
Chazdon, R. L., Letcher, S. G., van Breugel, M., Martínez-Ramos, M., Bongers, F., and Finegan, B.: Rates of change in tree communities of secondary Neotropical forests following major disturbances, Philos. Trans. R. Soc. B Biol. Sci., 362, 273–289, https://doi.org/10.1098/rstb.2006.1990, 2007.
Chazdon, R. L., Broadbent, E. N., Rozendaal, D. M. A., Bongers, F., Zambrano, A. M. A., Aide, T. M., Balvanera, P., Becknell, J. M., Boukili, V., Brancalion, P. H. S., Craven, D., Almeida-Cortez, J. S., Cabral, G. A. L., de Jong, B., Denslow, J. S., Dent, D. H., DeWalt, S. J., Dupuy, J. M., Durán, S. M., Espírito-Santo, M. M., Fandino, M. C., César, R. G., Hall, J. S., Hernández-Stefanoni, J. L., Jakovac, C. C., Junqueira, A. B., Kennard, D., Letcher, S. G., Lohbeck, M., Martínez-Ramos, M., Massoca, P., Meave, J. A., Mesquita, R., Mora, F., Muñoz, R., Muscarella, R., Nunes, Y. R. F., Ochoa-Gaona, S., Orihuela-Belmonte, E., Peña-Claros, M., Pérez-García, E. A., Piotto, D., Powers, J. S., Rodríguez-Velazquez, J., Romero-Pérez, I. E., Ruíz, J., Saldarriaga, J. G., Sanchez-Azofeifa, A., Schwartz, N. B., Steininger, M. K., Swenson, N. G., Uriarte, M., van Breugel, M., van der Wal, H., Veloso, M. D. M., Vester, H., Vieira, I. C. G., Bentos, T. V., Williamson, G. B., and Poorter, L.: Carbon sequestration potential of second-growth forest regeneration in the Latin American tropics, Sci. Adv., 2, e1501639, https://doi.org/10.1126/sciadv.1501639, 2016.
Cohen, W. B., Harmon, M. E., Wallin, D. O., and Fiorella, M.: Two Decades of Carbon Flux from Forests of the Pacific Northwest: Estimates from a new modeling strategy, BioScience, 46, 836–844, https://doi.org/10.2307/1312969, 1996.
Coomes, D. A., Dalponte, M., Jucker, T., Asner, G. P., Banin, L. F., Burslem, D. F. R. P., Lewis, S. L., Nilus, R., Phillips, O. L., Phua, M.-H., and Qie, L.: Area-based vs tree-centric approaches to mapping forest carbon in Southeast Asian forests from airborne laser scanning data, Remote Sens. Environ., 194, 77–88, https://doi.org/10.1016/j.rse.2017.03.017, 2017.
Cumberlege, P. F. and Cumberlege, V. M. S.: A preliminary list of the orchids of Khao Yai National Park, Nat. Hist. Bull. Siam Soc, 20, 155–182, 1963.
Dubayah, R. O., Sheldon, S. L., Clark, D. B., Hofton, M. A., Blair, J. B., Hurtt, G. C., and Chazdon, R. L.: Estimation of tropical forest height and biomass dynamics using lidar remote sensing at La Selva, Costa Rica, J. Geophys. Res.-Biogeo., 115, https://doi.org/10.1029/2009JG000933, 2010.
Ewel, J. J., Chai, P., and Tsai, L. M.: Biomass and Floristics of three young second-growth forests in Sarawak, Malaysian Forester, 46, 347–364, 1983.
FAO: Global forest resources assessment 2010: terms and definitions. FAO working paper 144/E, Food and Agriculture Organization (FAO) of the United Nations (UN), Rome, 2010.
FAO: The State of Food and Agriculture, Investing in agriculture for a better future, FAO publications, Rome, Italy, 2012.
Feldpausch, T. R., Prates-Clark, C. da C., Fernandes, E. C. M., and Riha, S. J.: Secondary forest growth deviation from chronosequence predictions in central Amazonia, Glob. Change Biol., 13, 967–979, https://doi.org/10.1111/j.1365-2486.2007.01344.x, 2007.
Feldpausch, T. R., Banin, L., Phillips, O. L., Baker, T. R., Lewis, S. L., Quesada, C. A., Affum-Baffoe, K., Arets, E. J. M. M., Berry, N. J., Bird, M., Brondizio, E. S., de Camargo, P., Chave, J., Djagbletey, G., Domingues, T. F., Drescher, M., Fearnside, P. M., França, M. B., Fyllas, N. M., Lopez-Gonzalez, G., Hladik, A., Higuchi, N., Hunter, M. O., Iida, Y., Salim, K. A., Kassim, A. R., Keller, M., Kemp, J., King, D. A., Lovett, J. C., Marimon, B. S., Marimon-Junior, B. H., Lenza, E., Marshall, A. R., Metcalfe, D. J., Mitchard, E. T. A., Moran, E. F., Nelson, B. W., Nilus, R., Nogueira, E. M., Palace, M., Patiño, S., Peh, K. S.-H., Raventos, M. T., Reitsma, J. M., Saiz, G., Schrodt, F., Sonké, B., Taedoumg, H. E., Tan, S., White, L., Wöll, H., and Lloyd, J.: Height-diameter allometry of tropical forest trees, Biogeosciences, 8, 1081–1106, https://doi.org/10.5194/bg-8-1081-2011, 2011.
Feldpausch, T. R., Lloyd, J., Lewis, S. L., Brienen, R. J. W., Gloor, M., Monteagudo Mendoza, A., Lopez-Gonzalez, G., Banin, L., Abu Salim, K., Affum-Baffoe, K., Alexiades, M., Almeida, S., Amaral, I., Andrade, A., Aragão, L. E. O. C., Araujo Murakami, A., Arets, E. J. M. M., Arroyo, L., Aymard C., G. A., Baker, T. R., Bánki, O. S., Berry, N. J., Cardozo, N., Chave, J., Comiskey, J. A., Alvarez, E., de Oliveira, A., Di Fiore, A., Djagbletey, G., Domingues, T. F., Erwin, T. L., Fearnside, P. M., França, M. B., Freitas, M. A., Higuchi, N., E. Honorio C., Iida, Y., Jiménez, E., Kassim, A. R., Killeen, T. J., Laurance, W. F., Lovett, J. C., Malhi, Y., Marimon, B. S., Marimon-Junior, B. H., Lenza, E., Marshall, A. R., Mendoza, C., Metcalfe, D. J., Mitchard, E. T. A., Neill, D. A., Nelson, B. W., Nilus, R., Nogueira, E. M., Parada, A., Peh, K. S.-H., Pena Cruz, A., Peñuela, M. C., Pitman, N. C. A., Prieto, A., Quesada, C. A., Ramírez, F., Ramírez-Angulo, H., Reitsma, J. M., Rudas, A., Saiz, G., Salomão, R. P., Schwarz, M., Silva, N., Silva-Espejo, J. E., Silveira, M., Sonké, B., Stropp, J., Taedoumg, H. E., Tan, S., ter Steege, H., Terborgh, J., Torello-Raventos, M., van der Heijden, G. M. F., Vásquez, R., Vilanova, E., Vos, V. A., White, L., Willcock, S., Woell, H., and Phillips, O. L.: Tree height integrated into pantropical forest biomass estimates, Biogeosciences, 9, 3381–3403, https://doi.org/10.5194/bg-9-3381-2012, 2012.
Ferraz, A., Saatchi, S., Xu, L., Hagen, S., Chave, J., Yu, Y., Meyer, V., Garcia, M., Silva, C., Roswintiart, O., Samboko, A., Sist, P., Walker, S., Pearson, T. R. H., Wijaya, A., Sullivan, F. B., Rutishauser, E., Hoekman, D., and Ganguly, S.: Carbon storage potential in degraded forests of Kalimantan, Indonesia, Environ. Res. Lett., 13, 095001, https://doi.org/10.1088/1748-9326/aad782, 2018.
Gibbs, H. K., Brown, S., Niles, J. O., and Foley, J. A.: Monitoring and estimating tropical forest carbon stocks: making REDD a reality, Environ. Res. Lett., 2, 045023, https://doi.org/10.1088/1748-9326/2/4/045023, 2007.
Goetz, S. J., Baccini, A., Laporte, N. T., Johns, T., Walker, W., Kellndorfer, J., Houghton, R. A., and Sun, M.: Mapping and monitoring carbon stocks with satellite observations: a comparison of methods, Carbon Balance Manag., 4, 2, https://doi.org/10.1186/1750-0680-4-2, 2009.
Hansen, M. C., Potapov, P. V., Moore, R., Hancher, M., Turubanova, S. A., Tyukavina, A., Thau, D., Stehman, S. V., Goetz, S. J., Loveland, T. R., Kommareddy, A., Egorov, A., Chini, L., Justice, C. O., and Townshend, J. R. G.: High-Resolution Global Maps of 21st-Century Forest Cover Change, Science, 342, 850–853, https://doi.org/10.1126/science.1244693, 2013.
Harris, N. L., Brown, S., Hagen, S. C., Saatchi, S. S., Petrova, S., Salas, W., Hansen, M. C., Potapov, P. V., and Lotsch, A.: Baseline Map of Carbon Emissions from Deforestation in Tropical Regions, Science, 336, 1573–1576, https://doi.org/10.1126/science.1217962, 2012.
Helmer, E. H., Lefsky, M. A., and Roberts, D. A.: Biomass accumulation rates of Amazonian secondary forest and biomass of old-growth forests from Landsat time series and the Geoscience Laser Altimeter System, J. Appl. Remote Sens. 3, 1–31, https://doi.org/10.1117/1.3082116, 2009.
Hiratsuka, M., Toma, T., Diana, R., Hadriyanto, D., and Morikawa, Y.: Biomass Recovery of Naturally Regenerated Vegetation after the 1998 Forest Fire in East Kalimantan, Indonesia, Japan Agric. Res. Quarterly, 40, 277–282, https://doi.org/10.6090/jarq.40.277, 2006.
IPCC: Guidelines for national greenhouse gas inventories, Agriculture, Forestry and other land use (AFLOLU), Institute for Global Environmental strategies, Hayama, Japan, 4, 2006.
IPCC: 2019 Refinement to the 2006 IPCC Guidelines for National Greenhouse Gas Inventories, IPCC-49th Session, Kyoto, Japan (Decision IPCC-XLIX-9), 2019.
Jenks, K. E., Chanteap, P., Kanda, D., Peter, C., Cutter, P., Redford, T., Antony, J. L., Howard, J., and Leimgruber, P.: Using Relative Abundance Indices from Camera-Trapping to Test Wildlife Conservation Hypotheses – An Example from Khao Yai National Park, Thailand, Tropical Conservation Science, 4, 113–131, https://doi.org/10.1177/194008291100400203, 2011.
Jepsen, M. R.: Above-ground carbon stocks in tropical fallows, Sarawak, Malaysia, For. Ecol. Manag., 225, 287–295, https://doi.org/10.1016/j.foreco.2006.01.005, 2006.
Jucker, T., Asner, G. P., Dalponte, M., Brodrick, P. G., Philipson, C. D., Vaughn, N. R., Teh, Y. A., Brelsford, C., Burslem, D. F. R. P., Deere, N. J., Ewers, R. M., Kvasnica, J., Lewis, S. L., Malhi, Y., Milne, S., Nilus, R., Pfeifer, M., Phillips, O. L., Qie, L., Renneboog, N., Reynolds, G., Riutta, T., Struebig, M. J., Svátek, M., Turner, E. C., and Coomes, D. A.: Estimating aboveground carbon density and its uncertainty in Borneo's structurally complex tropical forests using airborne laser scanning, Biogeosciences, 15, 3811–3830, https://doi.org/10.5194/bg-15-3811-2018, 2018.
Junttila, V., Kauranne, T., Finley, A. O., and Bradford, J. B.: Linear Models for Airborne-Laser-Scanning-Based Operational Forest Inventory With Small Field Sample Size and Highly Correlated LiDAR Data, IEEE Trans. Geosci. Remote Sens., 53, 5600–5612, https://doi.org/10.1109/TGRS.2015.2425916, 2015.
Kennard, D. K., Gould, K., Putz, F. E., Fredericksen, T. S., and Morales, F.: Effect of disturbance intensity on regeneration mechanisms in a tropical dry forest, For. Ecol. Manag., 162, 197–208, https://doi.org/10.1016/S0378-1127(01)00506-0, 2002.
Kitamura, S., Yumoto, T., Poonswad, P., Chuailua, P., and Plongmai, K.: Characteristics of hornbill-dispersed fruits in a tropical seasonal forest in Thailand, Bird Conserv. Int., 14, S81–S88, https://doi.org/10.1017/S0959270905000250, 2004.
Kronseder, K., Ballhorn, U., Böhm, V., and Siegert, F.: Above ground biomass estimation across forest types at different degradation levels in Central Kalimantan using LiDAR data, Int. J. Appl. Earth Obs. Geoinformation, 18, 37–48, https://doi.org/10.1016/j.jag.2012.01.010, 2012.
Labriere, N., Tao, S., Chave, J., Scipal, K., Toan, T. L., Abernethy, K., Alonso, A., Barbier, N., Bissiengou, P., Casal, T., Davies, S. J., Ferraz, A., Herault, B., Jaouen, G., Jeffery, K. J., Kenfack, D., Korte, L., Lewis, S. L., Malhi, Y., Memiaghe, H. R., Poulsen, J. R., Rejou-Mechain, M., Villard, L., Vincent, G., White, L. J. T., and Saatchi, S.: In Situ Reference Datasets From the TropiSAR and AfriSAR Campaigns in Support of Upcoming Spaceborne Biomass Missions, IEEE J. Sel. Top. Appl. Earth Obs. Remote Sens., 11, 3617–3627, https://doi.org/10.1109/JSTARS.2018.2851606, 2018.
Letcher, S. G. and Chazdon, R. L.: Rapid Recovery of Biomass, Species Richness, and Species Composition in a Forest Chronosequence in Northeastern Costa Rica, Biotropica, 41, 608–617, https://doi.org/10.1111/j.1744-7429.2009.00517.x, 2009.
Liaw, A. and Wiener, M.: Classification and Regression by RandomForest, R news, 2, 18–22, 2002.
Lohbeck, M., Poorter, L., Martínez-Ramos, M., and Bongers, F.: Biomass is the main driver of changes in ecosystem process rates during tropical forest succession, Ecology, 96, 1242–1252, https://doi.org/10.1890/14-0472.1, 2015.
Losos, E. C. and Leigh, E. G.: Tropical forest diversity and dynamism: findings from a large-scale plot network, Chicago University Press, Chicago, Illinois, USA, 2004.
Lu, D.: The potential and challenge of remote sensing-based biomass estimation, Int. J. Remote Sens., 27, 1297–1328, https://doi.org/10.1080/01431160500486732, 2006.
Lugo, A. E. and Brown, S.: Tropical forests as sinks of atmospheric carbon, For. Ecol. Manag., 54, 239–255, https://doi.org/10.1016/0378-1127(92)90016-3, 1992.
Maltamo, M., Packalén, P., Yu, X., Eerikäinen, K., Hyyppä, J., and Pitkänen, J.: Identifying and quantifying structural characteristics of heterogeneous boreal forests using laser scanner data, For. Ecol. Manag., 216, 41–50, https://doi.org/10.1016/j.foreco.2005.05.034, 2005.
Masek, J. G. and Collatz, G. J.: Estimating forest carbon fluxes in a disturbed southeastern landscape: Integration of remote sensing, forest inventory, and biogeochemical modeling, J. Geophys. Res., 111, G01006, https://doi.org/10.1029/2005JG000062, 2006.
McMahon, S. M., Arellano, G., and Davies, S. J.: The importance and challenges of detecting changes in forest mortality rates, Ecosphere, 10, e02615, https://doi.org/10.1002/ecs2.2615, 2019.
Meyer, V., Saatchi, S. S., Chave, J., Dalling, J. W., Bohlman, S., Fricker, G. A., Robinson, C., Neumann, M., and Hubbell, S.: Detecting tropical forest biomass dynamics from repeated airborne lidar measurements, Biogeosciences, 10, 5421–5438, https://doi.org/10.5194/bg-10-5421-2013, 2013.
Meyer, V., Saatchi, S., Ferraz, A., Xu, L., Duque, A., García, M., and Chave, J.: Forest degradation and biomass loss along the Chocó region of Colombia, Carbon Balance Manag., 14, 2, https://doi.org/10.1186/s13021-019-0117-9, 2019.
Mitchard, E. T., Saatchi, S. S., Baccini, A., Asner, G. P., Goetz, S. J., Harris, N. L., and Brown, S.: Uncertainty in the spatial distribution of tropical forest biomass: a comparison of pan-tropical maps, Carbon Balance Manag., 8, 10, https://doi.org/10.1186/1750-0680-8-10, 2013.
Mitchard, E. T. A., Feldpausch, T. R., Brienen, R. J. W., Lopez-Gonzalez, G., Monteagudo, A., Baker, T. R., Lewis, S. L., Lloyd, J., Quesada, C. A., Gloor, M., Steege, H. ter, Meir, P., Alvarez, E., Araujo-Murakami, A., Aragão, L. E. O. C., Arroyo, L., Aymard, G., Banki, O., Bonal, D., Brown, S., Brown, F. I., Cerón, C. E., Moscoso, V. C., Chave, J., Comiskey, J. A., Cornejo, F., Medina, M. C., Costa, L. D., Costa, F. R. C., Fiore, A. D., Domingues, T. F., Erwin, T. L., Frederickson, T., Higuchi, N., Coronado, E. N. H., Killeen, T. J., Laurance, W. F., Levis, C., Magnusson, W. E., Marimon, B. S., Junior, B. H. M., Polo, I. M., Mishra, P., Nascimento, M. T., Neill, D., Vargas, M. P. N., Palacios, W. A., Parada, A., Molina, G. P., Peña-Claros, M., Pitman, N., Peres, C. A., Poorter, L., Prieto, A., Ramirez-Angulo, H., Correa, Z. R., Roopsind, A., Roucoux, K. H., Rudas, A., Salomão, R. P., Schietti, J., Silveira, M., Souza, P. F. de, Steininger, M. K., Stropp, J., Terborgh, J., Thomas, R., Toledo, M., Torres-Lezama, A., van Andel, T. R., Heijden, G. M. F. van der, Vieira, I. C. G., Vieira, S., Vilanova-Torre, E., Vos, V. A., Wang, O., Zartman, C. E., Malhi, Y., and Phillips, O. L.: Markedly divergent estimates of Amazon forest carbon density from ground plots and satellites, Glob. Ecol. Biogeogr., 23, 935–946, https://doi.org/10.1111/geb.12168, 2014.
Næsset, E.: Predicting forest stand characteristics with airborne scanning laser using a practical two-stage procedure and field data, Remote Sens. Environ., 80, 88–99, https://doi.org/10.1016/S0034-4257(01)00290-5, 2002.
N'Guessan, A. E., N'dja, J. K., Yao, O. N., Amani, B. H. K., Gouli, R. G. Z., Piponiot, C., Zo-Bi, I. C., and Hérault, B.: Drivers of biomass recovery in a secondary forested landscape of West Africa, For. Ecol. Manag., 433, 325–331, https://doi.org/10.1016/j.foreco.2018.11.021, 2019.
Norden, N., Mesquita, R. C. G., Bentos, T. V., Chazdon, R. L., and Williamson, G. B.: Contrasting community compensatory trends in alternative successional pathways in central Amazonia, Oikos, 120, 143–151, https://doi.org/10.1111/j.1600-0706.2010.18335.x, 2011.
Norden, N., Angarita, H. A., Bongers, F., Martínez-Ramos, M., Granzow-de la Cerda, I., van Breugel, M., Lebrija-Trejos, E., Meave, J. A., Vandermeer, J., Williamson, G. B., Finegan, B., Mesquita, R., and Chazdon, R. L.: Successional dynamics in Neotropical forests are as uncertain as they are predictable, P. Natl. Acad. Sci. USA, 112, 8013–8018, https://doi.org/10.1073/pnas.1500403112, 2015.
Oliver, C. D. and Larson, B. C.: Forest stand dynamics, Wiley, New York, 520, 1996.
Pflugmacher, D., Cohen, W. B., and Kennedy, E. R.: Using Landsat-derived disturbance history (1972–2010) to predict current forest structure, Remote Sens. Environ., 122, 146–165, https://doi.org/10.1016/j.rse.2011.09.025, 2012.
Pflugmacher, D., Cohen, W. B., Kennedy, R. E., and Yang, Z.: Using Landsat-derived disturbance and recovery history and lidar to map forest biomass dynamics, Remote Sens. Environ., 151, 124–137, https://doi.org/10.1016/j.rse.2013.05.033, 2014.
Pickell, P. D., Hermosilla, T., Frazier, R. J., Coops, N. C., and Wulder, M. A.: Forest recovery trends derived from Landsat time series for North American boreal forests, Int. J. Remote Sens., 37, 138–149, https://doi.org/10.1080/2150704X.2015.1126375, 2016.
Poorter, L., Bongers, F., Aide, T. M., Almeyda Zambrano, A. M., Balvanera, P., Becknell, J. M., Boukili, V., Brancalion, P. H. S., Broadbent, E. N., Chazdon, R. L., Craven, D., de Almeida-Cortez, J. S., Cabral, G. A. L., de Jong, B. H. J., Denslow, J. S., Dent, D. H., DeWalt, S. J., Dupuy, J. M., Durán, S. M., Espírito-Santo, M. M., Fandino, M. C., César, R. G., Hall, J. S., Hernandez-Stefanoni, J. L., Jakovac, C. C., Junqueira, A. B., Kennard, D., Letcher, S. G., Licona, J.-C., Lohbeck, M., Marín-Spiotta, E., Martínez-Ramos, M., Massoca, P., Meave, J. A., Mesquita, R., Mora, F., Muñoz, R., Muscarella, R., Nunes, Y. R. F., Ochoa-Gaona, S., de Oliveira, A. A., Orihuela-Belmonte, E., Peña-Claros, M., Pérez-García, E. A., Piotto, D., Powers, J. S., Rodríguez-Velázquez, J., Romero-Pérez, I. E., Ruíz, J., Saldarriaga, J. G., Sanchez-Azofeifa, A., Schwartz, N. B., Steininger, M. K., Swenson, N. G., Toledo, M., Uriarte, M., van Breugel, M., van der Wal, H., Veloso, M. D. M., Vester, H. F. M., Vicentini, A., Vieira, I. C. G., Bentos, T. V., Williamson, G. B., and Rozendaal, D. M. A.: Biomass resilience of Neotropical secondary forests, Nature, 530, 211–214, https://doi.org/10.1038/nature16512, 2016a.
Poorter, L., Bongers, F., Aide, T. M., Almeyda Zambrano, A. M., Balvanera, P., Becknell, J. M., Boukili, V., Brancalion, P. H. S., Broadbent, E. N., Chazdon, R. L., Craven, D., de Almeida-Cortez, J. S., Cabral, G. A. L., de Jong, B. H. J., Denslow, J. S., Dent, D. H., DeWalt, S. J., Dupuy, J. M., Durán, S. M., Espírito-Santo, M. M., Fandino, M. C., César, R. G., Hall, J. S., Hernandez-Stefanoni, J. L., Jakovac, C. C., Junqueira, A. B., Kennard, D., Letcher, S. G., Licona, J.-C., Lohbeck, M., Marín-Spiotta, E., Martínez-Ramos, M., Massoca, P., Meave, J. A., Mesquita, R., Mora, F., Muñoz, R., Muscarella, R., Nunes, Y. R. F., Ochoa-Gaona, S., de Oliveira, A. A., Orihuela-Belmonte, E., Peña-Claros, M., Pérez-García, E. A., Piotto, D., Powers, J. S., Rodríguez-Velázquez, J., Romero-Pérez, I. E., Ruíz, J., Saldarriaga, J. G., Sanchez-Azofeifa, A., Schwartz, N. B., Steininger, M. K., Swenson, N. G., Toledo, M., Uriarte, M., van Breugel, M., van der Wal, H., Veloso, M. D. M., Vester, H. F. M., Vicentini, A., Vieira, I. C. G., Vizcarra Bentos, T., Williamson, G. B., and Rozendaal, D. M. A.: Data from: Biomass resilience of Neotropical secondary forests, Dryad, Dataset, https://doi.org/10.5061/dryad.82vr4, 2016b.
Ray, G. J. and Brown, B. J.: Seed Ecology of Woody Species in a Caribbean Dry Forest, Restor. Ecol., 2, 156–163, https://doi.org/10.1111/j.1526-100X.1994.tb00063.x, 2006.
Réjou-Méchain, M., Muller-Landau, H. C., Detto, M., Thomas, S. C., Le Toan, T., Saatchi, S. S., Barreto-Silva, J. S., Bourg, N. A., Bunyavejchewin, S., Butt, N., Brockelman, W. Y., Cao, M., Cárdenas, D., Chiang, J.-M., Chuyong, G. B., Clay, K., Condit, R., Dattaraja, H. S., Davies, S. J., Duque, A., Esufali, S., Ewango, C., Fernando, R. H. S., Fletcher, C. D., Gunatilleke, I. A. U. N., Hao, Z., Harms, K. E., Hart, T. B., Hérault, B., Howe, R. W., Hubbell, S. P., Johnson, D. J., Kenfack, D., Larson, A. J., Lin, L., Lin, Y., Lutz, J. A., Makana, J.-R., Malhi, Y., Marthews, T. R., McEwan, R. W., McMahon, S. M., McShea, W. J., Muscarella, R., Nathalang, A., Noor, N. S. M., Nytch, C. J., Oliveira, A. A., Phillips, R. P., Pongpattananurak, N., Punchi-Manage, R., Salim, R., Schurman, J., Sukumar, R., Suresh, H. S., Suwanvecho, U., Thomas, D. W., Thompson, J., Uríarte, M., Valencia, R., Vicentini, A., Wolf, A. T., Yap, S., Yuan, Z., Zartman, C. E., Zimmerman, J. K., and Chave, J.: Local spatial structure of forest biomass and its consequences for remote sensing of carbon stocks, Biogeosciences, 11, 6827–6840, https://doi.org/10.5194/bg-11-6827-2014, 2014.
Réjou-Méchain, M., Tymen, B., Blanc, L., Fauset, S., Feldpausch, T. R., Monteagudo, A., Phillips, O. L., Richard, H., and Chave, J.: Using repeated small-footprint LiDAR acquisitions to infer spatial and temporal variations of a high-biomass Neotropical forest, Remote Sens. Environ., 169, 93–101, https://doi.org/10.1016/j.rse.2015.08.001, 2015.
Réjou-Méchain, M., Tanguy, A., Piponiot, C., Chave, J., and Hérault, B.: Biomass: an R package for estimating above-ground biomass and its uncertainty in tropical forests, Methods Ecol. Evol., 8, 1163–1167, https://doi.org/10.1111/2041-210X.12753, 2017.
Réjou-Méchain, M., Barbier, N., Couteron, P., Ploton, P., Vincent, G., Herold, M., Mermoz, S., Saatchi, S., Chave, J., de Boissieu, F., Féret, J.-B., Takoudjou, S. M., and Pélissier, R.: Upscaling Forest Biomass from Field to Satellite Measurements: Sources of Errors and Ways to Reduce Them, Surv. Geophys., 40, 881–911, https://doi.org/10.1007/s10712-019-09532-0, 2019.
Requena Suarez, D., Rozendaal, D. M. A., De Sy, V., Phillips, O. L., Alvarez-Dávila, E., Anderson-Teixeira, K., Araujo-Murakami, A., Arroyo, L., Baker, T. R., Bongers, F., Brienen, R. J. W., Carter, S., Cook-Patton, S. C., Feldpausch, T. R., Griscom, B. W., Harris, N., Hérault, B., Honorio Coronado, E. N., Leavitt, S. M., Lewis, S. L., Marimon, B. S., Monteagudo Mendoza, A., Kassi N'dja, J., N'Guessan, A. E., Poorter, L., Qie, L., Rutishauser, E., Sist, P., Sonké, B., Sullivan, M. J. P., Vilanova, E., Wang, M. M. H., Martius, C., and Herold, M.: Estimating aboveground net biomass change for tropical and subtropical forests: Refinement of IPCC default rates using forest plot data, Glob. Change Biol,, 25, 3609–3624, https://doi.org/10.1111/gcb.14767, 2019.
Roussel, J.-R. and Auty, D.: lidR: Airborne LiDAR Data Manipulation and Visualization for Forestry Applications, R package version 1.5.0, 2017.
Rozendaal, D. M. A. and Chazdon, R. L.: Demographic drivers of tree biomass change during secondary succession in northeastern Costa Rica, Ecol. Appl., 25, 506–516, https://doi.org/10.1890/14-0054.1, 2015.
Rozendaal, D. M. A., Chazdon, R. L., Arreola-Villa, F., Balvanera, P., Bentos, T. V., Dupuy, J. M., Hernández-Stefanoni, J. L., Jakovac, C. C., Lebrija-Trejos, E. E., Lohbeck, M., Martínez-Ramos, M., Massoca, P. E. S., Meave, J. A., Mesquita, R. C. G., Mora, F., Pérez-García, E. A., Romero-Pérez, I. E., Saenz-Pedroza, I., van Breugel, M., Williamson, G. B., and Bongers, F.: Demographic Drivers of Aboveground Biomass Dynamics During Secondary Succession in Neotropical Dry and Wet Forests, Ecosystems, 20, 340–353, https://doi.org/10.1007/s10021-016-0029-4, 2017.
Ruiz, J., Fandino, M. C., and Chazdon, R. L.: Vegetation Structure, Composition, and Species Richness Across a 56-year Chronosequence of Dry Tropical Forest on Providencia Island, Colombia1, Biotropica, 37, 520–530, https://doi.org/10.1111/j.1744-7429.2005.00070.x, 2005.
Saldarriaga, J. G., West, D. C., Tharp, M. L., and Uhl, C.: Long-Term Chronosequence of Forest Succession in the Upper Rio Negro of Colombia and Venezuela, J. Ecol., 76, 938–958, https://doi.org/10.2307/2260625, 1988.
Sasaki, N. and Putz, F. E.: Critical need for new definitions of “forest” and “forest degradation” in global climate change agreements, Conserv. Lett., 2, 226–232, https://doi.org/10.1111/j.1755-263X.2009.00067.x, 2009.
Silver, W. L., Ostertag, R., and Lugo, A. E.: The Potential for Carbon Sequestration Through Reforestation of Abandoned Tropical Agricultural and Pasture Lands, Restor. Ecol., 8, 394–407, https://doi.org/10.1046/j.1526-100x.2000.80054.x, 2000.
Smitinand, T.: Vegetation of Khao Yai National Park, Siam Society, Thailand, 1968.
Stas, S. M., Rutishauser, E., Chave, J., Anten, N. P. R., and Laumonier, Y.: Estimating the aboveground biomass in an old secondary forest on limestone in the Moluccas, Indonesia: Comparing locally developed versus existing allometric models, For. Ecol. Manag., 389, 27–34, https://doi.org/10.1016/j.foreco.2016.12.010, 2017.
Stibig, H.-J., Achard, F., Carboni, S., Raši, R., and Miettinen, J.: Change in tropical forest cover of Southeast Asia from 1990 to 2010, Biogeosciences, 11, 247–258, https://doi.org/10.5194/bg-11-247-2014, 2014.
Sullivan, M. J. P., Talbot, J., Lewis, S. L., Phillips, O. L., Qie, L., Begne, S. K., Chave, J., Cuni-Sanchez, A., Hubau, W., Lopez-Gonzalez, G., Miles, L., Monteagudo-Mendoza, A., Sonké, B., Sunderland, T., ter Steege, H., White, L. J. T., Affum-Baffoe, K., Aiba, S., de Almeida, E. C., de Oliveira, E. A., Alvarez-Loayza, P., Dávila, E. Á., Andrade, A., Aragão, L. E. O. C., Ashton, P., C, G. A. A., Baker, T. R., Balinga, M., Banin, L. F., Baraloto, C., Bastin, J.-F., Berry, N., Bogaert, J., Bonal, D., Bongers, F., Brienen, R., Camargo, J. L. C., Cerón, C., Moscoso, V. C., Chezeaux, E., Clark, C. J., Pacheco, Á. C., Comiskey, J. A., Valverde, F. C., Coronado, E. N. H., Dargie, G., Davies, S. J., De Canniere, C., K, M. N. D., Doucet, J.-L., Erwin, T. L., Espejo, J. S., Ewango, C. E. N., Fauset, S., Feldpausch, T. R., Herrera, R., Gilpin, M., Gloor, E., Hall, J. S., Harris, D. J., Hart, T. B., Kartawinata, K., Kho, L. K., Kitayama, K., Laurance, S. G. W., Laurance, W. F., Leal, M. E., Lovejoy, T., Lovett, J. C., Lukasu, F. M., Makana, J.-R., Malhi, Y., Maracahipes, L., Marimon, B. S., Junior, B. H. M., Marshall, A. R., Morandi, P. S., Mukendi, J. T., Mukinzi, J., Nilus, R., Vargas, P. N., Camacho, N. C. P., Pardo, G., Peña-Claros, M., Pétronelli, P., Pickavance, G. C., Poulsen, A. D., Poulsen, J. R., Primack, R. B., Priyadi, H., Quesada, C. A., Reitsma, J., Réjou-Méchain, M., Restrepo, Z., Rutishauser, E., Salim, K. A., Salomão, R. P., Samsoedin, I., Sheil, D., Sierra, R., Silveira, M., Slik, J. W. F., Steel, L., Taedoumg, H., Tan, S., Terborgh, J. W., Thomas, S. C., Toledo, M., Umunay, P., Valenzuela Gamarra, L., Vieira, I. C. G., Vos, V. A., Wang, O., Willcock, S., and Zemagho, L.: Diversity and carbon storage across the tropical forest biome, Sci. Rep.-UK, 7, 39102, https://doi.org/10.1038/srep39102, 2017.
Thomas, S. C. and Martin, A. R.: Carbon Content of Tree Tissues: A Synthesis, Forests, 3, 332–352, https://doi.org/10.3390/f3020332, 2012.
Toledo, M. and Salick, J.: Secondary Succession and Indigenous Management in Semideciduous Forest Fallows of the Amazon Basin: Secondary Succession and Indigenous Management, Biotropica, 38, 161–170, https://doi.org/10.1111/j.1744-7429.2006.00120.x, 2006.
White, J. C., Saarinen, N., Kankare, V., Wulder, M. A., Hermosilla, T., Coops, N. C., Pickell, P. D., Holopainen, M., Hyyppä, J., and Vastaranta, M.: Confirmation of post-harvest spectral recovery from Landsat time series using measures of forest cover and height derived from airborne laser scanning data, Remote Sens. Environ., 216, 262–275, https://doi.org/10.1016/j.rse.2018.07.004, 2018.
Wulder, M. A., White, J. C., Bater, C. W., Coops, N. C., Hopkinson, C., and Chen, G.: Lidar plots – a new large-area data collection option: context, concepts, and case study, Can. J. Remote Sens., 38, 600–618, https://doi.org/10.5589/m12-049, 2012.
Zald, H. S. J., Ohmann, J. L., Roberts, H. M., Gregory, M. J., Henderson, E. B., McGaughey, R. J., and Braaten, J.: Influence of lidar, Landsat imagery, disturbance history, plot location accuracy, and plot size on accuracy of imputation maps of forest composition and structure, Remote Sens. Environ., 143, 26–38, https://doi.org/10.1016/j.rse.2013.12.013, 2014.
Zanne, A. E., Lopez-Gonzalez, G., Coomes, D. A., Ilic, J., Jansen, S., Lewis, S. L., Miller, R. B., Swenson, N. G., Wiemann, M. C., and Chave, J.: Data from: Towards a worldwide wood economics spectrum, Dryad, Dataset, https://doi.org/10.5061/dryad.234, 2009.
Zhao, K., Popescu, S., and Nelson, R.: Lidar remote sensing of forest biomass: A scale-invariant estimation approach using airborne lasers, Remote Sens. Environ., 113, 182–196, https://doi.org/10.1016/j.rse.2008.09.009, 2009.
Zheng, D., Rademacher, J., Chen, J., Crow, T., Bresee, M., Le Moine, J., and Ryu, S.-R.: Estimating aboveground biomass using Landsat 7 ETM+ data across a managed landscape in northern Wisconsin, USA, Remote Sens. Environ., 93, 402–411, https://doi.org/10.1016/j.rse.2004.08.008, 2004.
Zolkos, S. G., Goetz, S. J., and Dubayah, R.: A meta-analysis of terrestrial aboveground biomass estimation using lidar remote sensing, Remote Sens. Environ., 128, 289–298, https://doi.org/10.1016/j.rse.2012.10.017, 2013.