the Creative Commons Attribution 4.0 License.
the Creative Commons Attribution 4.0 License.
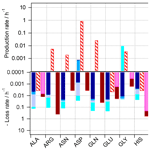
Biotic and abiotic transformation of amino acids in cloud water: experimental studies and atmospheric implications
Saly Jaber
Muriel Joly
Maxence Brissy
Martin Leremboure
Amina Khaled
Barbara Ervens
Anne-Marie Delort
The interest in organic nitrogen and particularly in quantifying and studying the fate of amino acids (AAs) has been growing in the atmospheric-science community. However very little is known about biotic and abiotic transformation mechanisms of amino acids in clouds.
In this work, we measured the biotransformation rates of 18 amino acids with four bacterial strains (Pseudomonas graminis PDD-13b-3, Rhodococcus enclensis PDD-23b-28, Sphingomonas sp. PDD-32b-11, and Pseudomonas syringae PDD-32b-74) isolated from cloud water and representative of this environment. At the same time, we also determined the abiotic (chemical, OH radical) transformation rates within the same solutions mimicking the composition of cloud water. We used a new approach by UPLC–HRMS (ultra-performance liquid chromatography–high-resolution mass spectrometry) to quantify free AAs directly in the artificial-cloud-water medium without concentration and derivatization.
The experimentally derived transformation rates were used to compare their relative importance under atmospheric conditions with loss rates based on kinetic data of amino acid oxidation in the aqueous phase. This analysis shows that previous estimates overestimated the abiotic degradation rates and thus underestimated the lifetime of amino acids in the atmosphere, as they only considered loss processes but did not take into account the potential transformation of amino acids into each other.
- Article
(824 KB) - Full-text XML
-
Supplement
(1363 KB) - BibTeX
- EndNote
The organic matter (OM) content of the cloud water phase is very complex; it has been described using Fourier-transform ion cyclotron resonance mass spectrometry (FT-ICR MS) (Bianco et al., 2018; Zhao et al., 2013). These global analytical methods revealed a very large number of organic carbon, organic sulfur and organic nitrogen compounds. For instance, in cloud water at the Puy de Dôme station, 5258 monoisotopic molecular formulas were assigned to CHO, CHNO, CHSO, and CHNSO (Bianco et al., 2018). Organic nitrogen compounds contribute a significant fraction to the total nitrogen in cloud water (18 %) (Hill et al., 2007) and in aerosol particles (7 %–10 % in urban areas) (Xu et al., 2017) and even exceed other nitrogen contributions in marine aerosol (Miyazaki et al., 2011). Among these organic nitrogen molecules, amino acids (AAs) have been recently analyzed and quantified in cloud droplets collected at the Puy de Dôme station and on the Cabo Verde islands (Triesch et al., 2021). AAs were also quantified in rain collected in marine and suburban sites (Mace et al., 2003a, b; Mopper and Zika, 1987; Sidle, 1967; Xu et al., 2019; Yan et al., 2015) and in fog samples in northern California (Zhang and Anastasio, 2003). In cloud water, free-AA concentrations range from 2.4 ± 2.0 to 74.3 ± 43.8 µg C L−1 at the rural site of the Puy de Dôme station (Bianco et al., 2016a) and from 17 to 757 µg C L−1 at the marine site of Cabo Verde (Triesch et al., 2021). These AAs are of biological origin and are the building blocks of peptides (also called “combined AA”) and proteins. They are initially present in aerosols which are further dissolved in atmospheric waters (Matos et al., 2016). Primary and secondary atmospheric sources of AAs are discussed in previous reviews (Cape et al., 2011; Sutton et al., 2011). Biomass burning (Zhu et al., 2020b), grassland (Scheller, 2001), ocean (Triesch et al., 2021), and agricultural activities (Song et al., 2017) were identified as major emission sources of amino acids.
Although organic carbon has been studied for a long time by atmospheric scientists, the interest in organic nitrogen and particularly in quantifying and studying the fate of AAs has been growing these last decades due to their specific properties. Some AAs can act as ice nuclei; for instance L-leucine nucleates ice at −4.5 ∘C (Szyrmer and Zawadzki, 1997). Their mass can also add to the hygroscopic fraction of cloud condensation nuclei due to their high water solubility (Kristensson et al., 2010). Another point concerns the participation of AAs in the global nitrogen and carbon cycles. For example, it has been estimated that organonitrogen compounds are a significant fraction (28 %) of the total nitrogen deposited (Zhang et al., 2012). Their ubiquity in living organisms makes their presence in atmospheric deposition very important for both terrestrial and aquatic ecosystems, as AAs represent the most bioavailable form of nitrogen (Cornell, 2011).
Finally, as part of the atmospheric OM, AAs are expected to undergo chemical processes in the atmospheric water phase (clouds, fog, and aerosol). Due to their low volatility, it can be assumed that they are not present in the gas phase. However little is known about their transformation processes occurring in the atmospheric compartments and particularly in clouds.
Concerning abiotic transformation (photo-transformation and radical chemistry) in atmospheric waters, some studies determined kinetic rate constants (k) of AAs with radicals (e.g., OH) (Scholes et al., 1965; Motohashi and Saito, 1993; Prütz and Vogel, 1976), singlet oxygen (1O2) (Kraljić and Sharpatyi, 1978; Matheson and Lee, 1979; McGregor and Anastasio, 2001; Michaeli and Feitelson, 1994; Miskoski and García, 1993; McGregor and Anastasio, 2001), or ozone (O3) (Ignatenko and Cherenkevich, 1985; Pryor et al., 1984). Based on such kinetic data, some studies have reported the time of life of amino acids in fog (McGregor and Anastasio, 2001) or in cloud water (Triesch et al., 2021). From these studies it is clear that some amino acids are transformed very rapidly, while others are almost never transformed within the timescale of fog or cloud life. When the additional effect of 1O2 was considered, MET (methionine), TRP (tryptophan), TYR (tyrosine), and HIS (histidine) remained the most degraded AAs (McGregor and Anastasio, 2001). Among other mechanisms, this fast degradation could explain why these AAs are usually among the less concentrated in aerosols (Barbaro et al., 2015, 2011; Matsumoto and Uematsu, 2005; Helin et al., 2017; Mashayekhy Rad et al., 2019; Mace et al., 2003b; Samy et al., 2013; Yang et al., 2004), in rain (Mace et al., 2003b; Xu et al., 2019; Yan et al., 2015), or in clouds (Triesch et al., 2021). The characterization of amino acids in dew showed differences depending on seasons, meteorological parameters, and irradiation conditions (Scheller, 2001).
Even less is known about the abiotic transformation pathways of these amino acids, as only some AAs have been studied in detail. Most mechanistic studies are limited to the transformation of AAs (GLY, glycine; TRP, tryptophan, ASP, aspartate; and SER, serine) into small carboxylic acids such as acetic, oxalic, malonic, or formic acids (Berger et al., 1999; Bianco et al., 2016b; Marion et al., 2018). In some cases, an amino acid can be converted into another one or into very different molecules (Bianco et al., 2016b; Mudd et al., 1969; Prasse et al., 2018; Stadtman, 1993; Stadtman and Levine, 2004). The main concern with these mechanistic studies is that they were performed under conditions rather far from atmospheric conditions. Incubation media did not contain a mixture of AAs or real atmospheric samples. They were sometimes measured with proteins in which the peptidyl bond might change the reactivity compared to free AAs (Pattison et al., 2012).
Another missing aspect concerns the potential biotransformation of these AAs in atmospheric waters. The microbial community which is present in cloud waters is metabolically active (Amato et al., 2017, 2019; Vaïtilingom et al., 2012) and has been shown to biotransform mono- and dicarboxylic acids, methanol, formaldehyde, phenol, and catechol (Ariya et al., 2002; Husárová et al., 2011; Jaber et al., 2020; Vaïtilingom et al., 2010, 2011, 2012). It is well-known that microorganisms have enzymatic networks able to biodegrade or biosynthesize amino acids. These pathways are complex and very interconnected (KEGG pathway database, n.d.). In cloud water, the biodegradation and biosynthesis of AAs is suspected to occur, as (i) it was shown that bacteria can use AAs as substrates in incubations with real cloud water containing endogenous bacteria and AAs because they can produce proteins and other cellular components allowing for their growth in this medium (Amato et al., 2007) and (ii) a recent metatranscriptomic study performed directly in cloud water showed the presence of transcripts of genes coding for AA biodegradation and synthesis (Amato et al., 2019). This is proof of in situ activity of bacteria in clouds. However, no data exist about the biotransformation rates of AAs in cloud water.
The aim of the present study is thus to measure biotic and abiotic rates of transformation of free AAs in microcosms mimicking cloud water with an incubation medium containing 19 AAs, other major carbon (acetate, succinate, formate, and oxalate) and nitrogen sources (NH and NO), and major salts (e.g., Na+, Cl−, and SO) present in cloud water collected at the Puy de Dôme station (Deguillaume et al., 2014). In addition, abiotic transformation rates are calculated based on rate constants of oxidation reactions with OH, 1O2, and O3 as reported in the literature. These experimental and theoretical rates of transformation are compared with each other and to previous literature studies and are discussed in terms of their atmospheric implications.
2.1 Experiments in microcosms
The experiments of biotic and abiotic transformation of amino acids were performed in microcosms mimicking cloud conditions at the Puy de Dôme station (1465 m). Solar light was fitted to that measured directly under cloudy conditions, and the temperature (17 ∘C) was representative of the average temperature in the summer. Incubations were performed in an artificial-cloud water medium containing inorganic ions, carboxylic acids, and amino acids within the same range of concentrations as those measured in clouds that were impacted by marine air masses collected at the Puy de Dôme station (Table S1, pH = 6.0) (Bianco et al., 2016a; Deguillaume et al., 2014). Rhodococcus enclensis PDD-23b-28, Pseudomonas graminis PDD-13b-3, Pseudomonas syringae PDD-32b-74, and Sphingomonas sp. PDD-32b-11 bacterial strains were chosen because they belong to the most abundant and active bacterial genera in cloud water (Amato et al., 2017; Vaïtilingom et al., 2012). In addition, the complete genome sequences of Rhodococcus enclensis PDD-23b-28, Pseudomonas graminis PDD-13b-3, and Pseudomonas syringae PDD-32b-74 have been published recently, giving access to their metabolic pathways in more detail (Besaury et al., 2017a, b; Lallement et al., 2017). In this work the total AA concentration used for the incubations was 19 µM, as we have included 19 AAs at a concentration of 1 µM each in the solution. This concentration is about 5 times higher than the concentrations measured in cloud water collected at the Puy de Dôme station by Bianco et al. (2016a) (the total AA concentration varied from 2.7 to 3.1 µM). To take this factor of 5 into account we used an artificial-cloud water whose composition in inorganic ions, carboxylic acids, and amino acids was multiplied by 5 compared to what is observed in clouds (Vaïtilingom et al., 2011). We also used a 5× concentration for bacteria (∼ 5 × 105 cell mL−1) (Vaïtilingom et al., 2012). So we have respected the concentration ratio of chemical compounds [(main organic and inorganic ions + AA)/number of cells] present in cloud water. In the past we have shown that is the ratio is constant; the rate of biodegradation is constant (Vaïtilingom et al., 2010). All experiments were performed in triplicates.
2.1.1 Cell preparation for further incubations
Rhodococcus enclensis PDD-23b-28, Pseudomonas graminis PDD-13b-3, Pseudomonas syringae PDD-32b-74, and Sphingomonas sp. PDD-32b-11 were grown in 10 mL of Reasoner Agar (R2A) medium for 16 h at 17 ∘C, with 130 rpm agitation (Reasoner and Geldreich, 1985). Then 1 mL of cultures was centrifuged at 12 500 rpm for 3 min. Bacteria pellets were rinsed two times with 1 mL of artificial-marine-cloud water, previously sterilized by filtration under sterile conditions using a 0.22 µm PES (polyethersulfone) filter. The bacterial cell concentration was estimated by optical density at 600 nm using a UV3100 spectrophotometer to obtain a concentration close to 5 × 105 cell mL−1. Finally, the concentration of cells was precisely determined by counting the colonies on R2A Petri dishes or by flow cytometry technique.
2.1.2 Biotransformation of amino acids
Rhodococcus enclensis PDD-23b-28, Pseudomonas graminis PDD-13b-3, Pseudomonas syringae PDD-32b-74, and Sphingomonas sp. PDD-32b-11 cells were each resuspended in a 50 mL flask of 1 µM amino acids (19 amino acids namely alanine (ALA, Sigma), arginine (ARG, Simafex), asparagine (ASN, Sigma), aspartate (ASP, Sigma-Aldrich), glutamine (GLN, Sigma), glutamic acid (GLU), glycine (GLY, Merck), histidine (HIS, Sigma), isoleucine (ILE, Sigma-Aldrich), lysine (LYS, Sigma-Aldrich), methionine (MET, Sigma), phenylalanine (PHE, Acros Organics), proline (PRO, Sigma-Aldrich), serine (SER, Sigma), threonine (THR, Sigma), tryptophan (TRP, Sigma), tyrosine (TYR, Sigma-Aldrich), valine (VAL, Sigma-Aldrich), and cysteine (CYS, Sigma-Aldrich) at 1 µM of each amino acid), prepared in artificial-cloud water (Table S1) and incubated at 17 ∘C, with 130 rpm agitation for 7 h in the dark. A control experiment was performed by incubating amino acids without bacteria; AA concentration remained stable over time (1 µM for each amino acid was obtained at the end of the experiment).
2.1.3 Abiotic transformation of amino acids
The same 19 amino acids, at a concentration of 1 µM each in the artificial-cloud medium (Table S1), were incubated at 17 ∘C, with 130 rpm agitation for 7 h in photo-bioreactors designed by Vaïtilingom et al. (2011). ⋅OH radicals were generated by photolysis adding a 0.5 mM Fe-ethylenediamine-N,N′-disuccinic acid (EDDS) complex solution. The Fe(EDDS) solution (iron complex with 1:1 stoichiometry) was prepared from iron(III) chloride hexahydrate (FeCl3, 6H2O; Sigma-Aldrich) and (S,S)-ethylenediamine-N,N′-disuccinic acid trisodium salt (EDDS, 35 % in water). A complementary experiment was also performed consisting of incubation of this solution in the presence of light without an Fe(EDDS) complex. The experimental conditions of the irradiation experiments (Sylvania Reptistar lamps; 15 W; 6500 K) and the mechanism of the OH radical production under light irradiation are described by Jaber et al. (2020). Assuming steady-state conditions for ⋅OH at the beginning of the experiments (i.e., equal ⋅OH production and loss rates), an ⋅OH concentration of 8.3 × 10−13 M was calculated as described by Jaber et al. (2020). This concentration is at the upper limit of ⋅OH concentrations in cloud water as derived from various model studies (Arakaki et al., 2013; Lallement et al., 2018).
2.2 Analytical methods
2.2.1 Amino acid UPLC–HRMS (ultra-performance liquid chromatography–high-resolution mass spectrometry) analyses
During the experiments in microcosms, 600 µL of the incubation medium was sampled regularly and centrifuged at 10 500 × g for 3 min, and the supernatants were kept frozen until analyses. In order to quantify the amino acid concentrations in the incubations, we developed here a new approach using an LC–HRMS technique based on a direct measurement by injection of the incubation medium without derivatization. The volume of injection was 5 µL.
All AAs could be quantified under these conditions, except cysteine.
LC–HRMS analyses of amino acids were performed using an UltiMate™ 3000 (Thermo Scientific™) UPLC equipped with a Q Exactive™ Hybrid Quadrupole-Orbitrap™ Mass Spectrometer (Thermo Scientific™) ionization chamber. Chromatographic separation of the analytes was performed on an ethylene bridged hybrid (BEH) Amide HILIC (1.7 µM, 100 mm × 2.1 mm; hydrophilic interaction liquid chromatography) column with a column temperature of 30 ∘C. The mobile phases consisted of 0.1 % formic acid and water (A) and 0.1 % formic acid and acetonitrile (B) with a flow rate 0.4 mL min−1. A four-step linear gradient of 10 % (A) and 90 % (B) for 8 min, 42 % (A) and 58 % (B) for 0.1 min, 50 % (A) and 50 % (B) for 0.9 min, and 10 % (A) and 90 % (B) for 3 min was used throughout the analysis.
The Q Exactive ion source was composed of an electrospray ionization (ESI+) and the Quadrupole-Orbitrap™. Flow injection analyses were performed for individual amino acid solutions in order to obtain the mass spectra, from which ions were selected using the SIM (selected ion monitoring) mode. The instrument was set for maximum ion throughput; the automatic gain control target or the number of ions to fill the C-trap was set to 105 for a maximum injection time of 100 ms. Gas (N2) flow rate and sheath gas (N2) flow rate were set at 13 and 50 p.d.u. (procedure-defined unit), respectively. Other parameters were as follows: 2 p.d.u. for the sweep gas flow rate, 3.2 kV for the spray voltage in positive mode, and 320 and 425 ∘C for the capillary temperature and the heater temperature, respectively. Under these conditions the mass resolution was 35 000 fwhm (full width, half mass). Analysis and visualization of the mass data were performed using Xcalibur™ 2.2 software (Thermo Scientific™).
Table S2 presents the retention times and values of (mass-to-charge ratio) for the ions [M + H] measured under these conditions for each amino acid.
2.2.2 Calibration curves and LOD and LOQ determination
In order to quantify the amino acid concentrations, calibration curves were established for each experimental series of LC–HRMS using the same artificial-cloud medium in the incubations.
In standard solutions, six concentrations of amino acids (0.01, 0.05, 0.1, 0.5, 1.0, and 5.0 µM) were used for these external standard multipoint calibrations. This range of concentration is appropriate considering that the initial amino acid concentration in the biotic and abiotic transformation experiments is 1 µM. Figure S1 presents an example of calibration curves for the 18 amino acids. The limits of detection (LOD) and quantification (LOQ) were calculated based on the standard deviation of the response (Sa) and on the slope of the calibration curves (b) (technical triplicate).
The obtained values of LOD and LOQ were considered to be fit for purpose (Table S2) and are consistent with data from the literature (Helin et al., 2017).
We also have calculated the relative standard deviation (RSD is the standard deviation divided by the mean) for each AA based on calibration curves (three technical replicates). As you can see in Table S3 these RSD values are rather low, ranging from around 0.5 % to 10 %, except for valine and glycine, where they can reach 20 %. It can be noticed that these RSD values due to the LC–MS method are much lower than those due to the transformation experiments, especially for biotransformation where there are biological variations (see error bars in Figs. 1 and 2).
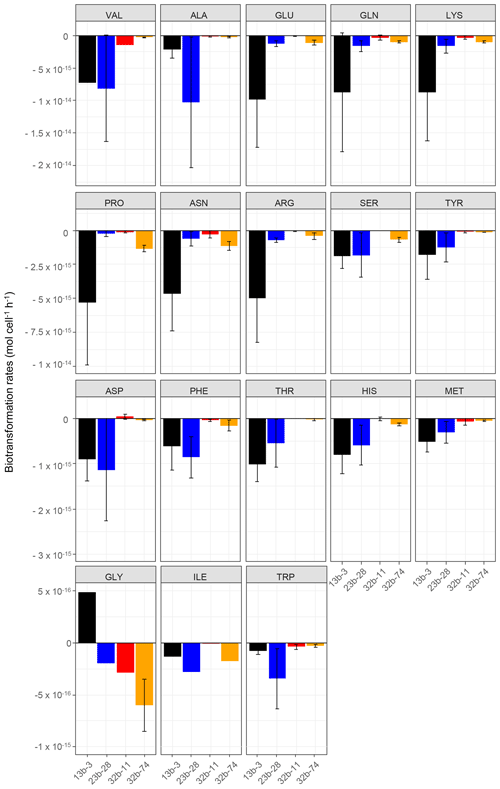
Figure 1Biotransformation rates obtained for each amino acid and each bacterial strain: Pseudomonas graminis PDD-13b-3 (black), Rhodococcus enclensis PDD-23b-28 (blue), Sphingomonas sp. PDD-32b-11 (red), and Pseudomonas syringae PDD-32b-74 (orange). The experiments were performed in microcosms containing the mixture of the 19 AAs in an artificial-cloud medium. The standard error bars reflect the significant biological variability measured from three triplicates (independent incubations).
2.2.3 Calculation of amino acid degradation rates in microcosms
The degradation rates of amino acids were calculated after normalization based on the ratio of the concentration at time t (Ct) and the concentration at time t=0 (C0). The pseudo-first-order rate constants (kisoleucine, kvaline, kproline, etc.) were determined using Eq. (1).
The slopes at the origin were used to calculate the corresponding degradation rates. For biotransformation, the rates were corrected by the precise number of bacterial cells present in the incubations and are expressed in the form of mol cell−1 h−1. An example is given in Figs. S2a and b for the case of the biodegradation of GLN.
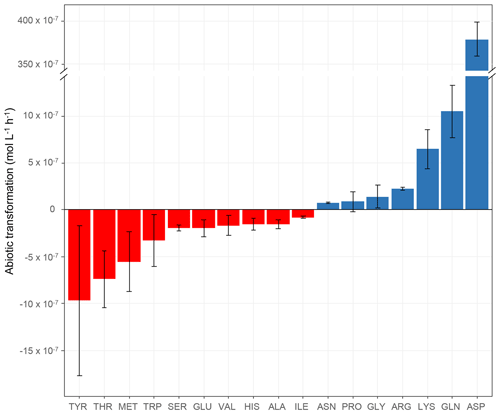
Figure 2Abiotic transformation rates (mol L−1 h−1) obtained for each amino acid in microcosms containing the mixture of the 19 AAs in an artificial-cloud medium under irradiation in the presence of Fe(EDDSS) as source of OH radicals. The standard error bars reflect the variability measured from three triplicates (independent experiments). Negative values represent abiotic degradation, while positive values represent abiotic production.
Table 1Average values of the biotransformation rates (mol cell−1 h−1; bacteria) of 18 amino acids by the four bacterial strains (Pseudomonas graminis PDD-13b-3, Rhodococcus enclensis PDD-23b-28, Pseudomonas syringae PDD-32b-74, and Sphingomonas sp. PDD-32b-11) and by the combination of these strains as representative of the biodiversity in a real cloud (named “Cloud”) as described in Sect. 3.3.2. Positive values correspond to a net biosynthesis, while negative ones correspond to a net biodegradation.
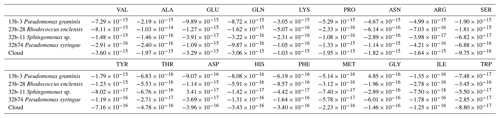
3.1 Biotransformation of amino acids in microcosms
3.1.1 Biotransformation rates of the 18 amino acids by the different bacterial strains
The biotransformation of the amino acids by four different bacterial strains isolated from cloud water at the Puy de Dôme station in an artificial-marine-cloud medium was monitored in four independent microcosms containing only one of the strains. Figure 1 shows the results obtained for each amino acid and each bacterial strain (Rhodococcus enclensis PDD-23b-28, Pseudomonas graminis PDD-13b-3, Pseudomonas syringae PDD-32b-74, and Sphingomonas sp. PDD-32b-11). The standard error bars reflect significant biological variability measured from three triplicates (independent incubations). Note that the biotransformation rates of valine, isoleucine, and glycine could be obtained only for one replicate due to technical problems. Table 1 summarizes the average values of the biodegradation rates of the 18 amino acids for the four bacterial strains. These average values for biodegradation (negative values) range from −1.03 × 10−14 to −8.0210−17 mol cell−1 h−1, i.e., spanning a range of almost 2 orders of magnitude depending on the amino acid and the bacterial strain. Note that in the case of glycine and the strain Pseudomonas graminis PDD-13b-3 and of aspartate and the strain Sphingomonas sp. PDD-32b-11, the values are positive, indicating a net production and not a net loss. The incubations were performed in a complex medium containing all AAs, and as a consequence the rate values are actually net values, as all the AAs are connected through metabolic pathways corresponding to both biodegradation and biosynthetic pathways (Fig. S3).
Overall Pseudomonas graminis PDD-13b-3 appears to be the most active strain followed by Rhodococcus enclensis PDD-23b-28 (Fig. 1 and Table 1). However, for some amino acids, this order is reversed: Rhodococcus enclensis degrades alanine, asparagine, phenylalanine, and tryptophan more efficiently than P. graminis does. For all amino acids, Pseudomonas syringae PDD-32b-74 is less active than R. enclensis and P. graminis followed by Sphingomonas sp. PDD-32b-11.
Considering the best degrading strains (Fig. 1 and Table 1), the most efficiently biodegraded amino acids are in the following order: valine > alanine > arginine > glutamate > glutamine > lysine > proline > asparagine > arginine > serine > tyrosine > aspartate, with biodegradation rates within the range of 10−14 to 10−15 mol cell−1 h−1. A second group of AAs has lower biodegradation rates in the range of 10−16 to 10−17 mol cell−1 h−1 in the following order: phenylalanine > threonine > histidine > methionine > glycine > isoleucine > tryptophan.
3.1.2 Link of the biodegradation rates with metabolic pathways
In bacteria many amino acids are connected within the same metabolic pathways via the enzymatic activities of their biosynthesis or biodegradation. Figure S3 presents a simplified network of the AA metabolic pathways as described in the KEGG (Kyoto Encyclopedia of Genes and Genomes) pathway database where the AA belonging to the same pathway are shown in the same color. We investigated the hypothesis of a potential link between the rates of biodegradation for each amino acid by the four strains with their connection in specific metabolic pathways (Figs. S3 and S4). Glutamate, glutamine, proline, and arginine metabolic pathways are closely linked (blue boxes in Fig. S3), and in parallel their biodegradation rates are on the same order of magnitude (Fig. S4). This is also true for the group of serine, threonine, glycine, and methionine (yellow boxes in Fig. S3) and for the group tyrosine, phenylalanine, and tryptophan (green boxes in Fig. S3), respectively. Alanine, asparagine, and aspartate (purple boxes in Fig. S3) are also related in the network, although the rate of biodegradation of aspartate is lower compared to the other two. Valine and isoleucine biodegradation rates are quite different; this can be explained by two divergent routes: valine is produced from pyruvate, while isoleucine is formed from 2-oxobutanoate. Histidine has a unique metabolic pathway, while lysine is also a special case as two metabolic routes exist: one is linked to 2-oxoadipate, while the other is connected to alanine, aspartate, and asparagine. To conclude, the rates of biodegradation can be grouped according to their presence in common metabolic pathways. This could explain, as suggested by Scheller (2001), why in dew the concentrations ARG, PRO, and GLU, three AAs belonging to the same pathway and connected to the urea cycle (Fig. S3), were increasing simultaneously.
3.1.3 Dependence of the selectivity of AA biodegradation on the bacterial phylogeny
The rates of biodegradation of the different amino acids expressed as a percentage of the highest rate for each strain are presented in the form of a radar plot in Fig. S5. A clear difference is observed between Rhodococcus enclensis PDD-23b-28 belonging to Actinobacteria (Fig. S5a) and the other strains belonging to Proteobacteria (Fig. S5b and c). Within Proteobacteria, it is possible to distinguish Sphingomonas sp. PDD-32b-11 (Fig. S5b) belonging to Alphaproteobacteria from Pseudomonas graminis PDD-13b-3 (grey, Fig. S5c) and Pseudomonas syringae PDD-32b-74 (yellow, Fig. S5c) belonging to Gammaproteobacteria. In addition, the two Pseudomonas strains share very similar trends. So, although the biodegradation rates of P. syringae are much lower than those of P. graminis, they seem to transform preferentially the same type of amino acids. This should be confirmed with a larger set of isolates. It suggests that the selectivity of AA biodegradation could be related to the phylogeny of the bacterial strains.
Table 2Abiotic transformation rates of the 17 AAs (mol L−1 h−1) measured in microcosms containing the mixture of the all AAs in an artificial-cloud medium under irradiation in the presence of Fe(EDDSS) as source of OH radicals. Positive values represent degradation, while negative values represent production. Mean values were calculated from three triplicates (independent experiments), except for ASN, GLN, GLY, and PRO. No value could be obtained for PHE (technical problem).

3.2 Abiotic transformation of amino acids in microcosms
The abiotic transformation rates of the amino acids measured in our microcosms are shown in Table 2 and Fig. 2. The first important result is that some amino acids are degraded (TYR, THR, MET, TRP, SER, GLU, VAL, HIS, ALA, and ILE), while others are produced (ASN, PRO, GLY, ARG, LYS, GLN, and ASP). Abiotic degradation rates (negative values of the transformation rates) were within the range of −7.98 × 10−8 to −9.70 × 10−7 mol L−1 h−1. Net abiotic production rates (positive values) were within the range of 7.69 × 10−8 to 1.05 × 10−6 mol L−1 h−1, except for ASP, whose rate was very high ( mol L−1 h−1). As mentioned in the context of biotic transformations (Sect. 3.1.1), the incubations are performed in artificial-cloud media containing the mixture of the 19 AAs, and, thus, the measured rates of abiotic transformations are net values, integrating various mechanisms.
3.3 Comparison of amino acid biotic and abiotic transformation rates
3.3.1 Kinetic rate constants for chemical oxidation reactions
In order to assess the atmospheric importance for the transformation of individual amino acids, we make the following assumptions. Loss by OH reactions occurs with the rate constants listed in Table S3 and an OH(aq) (aqueous) concentration of 1 × 10−14 M (Arakaki et al., 2013). For the oxidation by ozone, ozone has a concentration in cloud water of 0.5 nM, which corresponds to a gas phase mixing ratio of 50 ppb, using KH(O3) ∼ 10−3 M atm−1 (Sander, 1999). It has been shown previously that the rate constants of amino acids with ozone are strongly pH dependent, with smaller values for the protonated amino form (McGregor and Anastasio, 2001). Since the first acid dissociation constants (pKa1) for all amino acids are in the range of 2–2.5 and the second acid dissociation constants (pKa2) ((de-)protonation of the amino group) are in the range of 9–9.5 (Lide, 2009), it can be assumed that at cloud-relevant pH values (3 < pH < 6) the amino acids are present as carboxylates with protonated amine groups. In addition, we also consider the oxidation by singlet oxygen 1O2. Kinetic rate constants for only about half of the amino acids are available (Table S3). The estimates for 1O2 concentrations in the atmospheric aqueous phase are much sparser and less constrained than for the other oxidants. However, several studies agree that its concentration may be 2 to 3 orders of magnitude higher than the OH radical in clouds, fogs, and aerosol particles, respectively (Faust and Allen, 1992; Manfrin et al., 2019). Therefore, we assume an aqueous concentration of [1O2(aq)] = 10−12 M here. Other oxidants (e.g., HO2 / O and NO3) are not included in our analysis, as based on the few available kinetic data, it can be estimated that reaction rates may be too slow to represent an efficient sink (McGregor and Anastasio, 2001).
3.3.2 Comparison of biotic and abiotic transformation rates
In order to compare the relative importance of biotic (microbial) and abiotic (chemical) transformations under atmospheric conditions, we weighed the experimentally derived biotransformation rates by the relative abundance of the various bacteria strains as found in cloud water. An average concentration of 6.8 × 107 cell L−1 of cloud water was identified in cloud water samples at the Puy de Dôme station (France) (Vaïtilingom et al., 2012). Further characterization of these samples showed that Actinobacteria (Rhodococcus enclensis PDD-23b-28), Alphaproteobacteria (Sphingomonas sp. PDD-32b-1), and Gammaproteobacteria (Pseudomonas graminis PDD-13b-3 and Pseudomonas syringae PDD-32b-74) contributed to 6.3 %, 16.2 %, and 29.8 %, respectively, to the total cell concentration (Amato et al., 2017); the remaining 47.7 % belonged to other phyla or classes (Bacteroidetes, Betaproteobacteria, and Firmicutes).
Using these relative contributions, the loss rates as observed in our experiments (Sects. 3.1 and 3.2) were used to compare the loss rates under atmospheric conditions. For this comparison, we calculated the biotransformation rates in cloud water as
We scaled each contribution by a factor 1.91 (), implying that the four bacteria types are representative for the remainder (47.7 %) of the bacteria population.
We compare these rates to the photochemical rates derived in the experiments (Sect. 3.2). However, since the experiments where conducted with OH concentrations likely higher than ambient ones in cloud water, we correct these rates to OH(aq) concentrations in clouds by [OH(aq)]photo,exp = 8.3 × 10−13 M and [OH(aq)]cloud = 1 × 10−14 M.
Finally, these abiotic transformation rates based on the experiments are compared to those calculated based on kinetic data only.
In previous studies, the reactivity towards the OH radical and/or other oxidants was compared in terms of half-lives τ. However, we chose not to present half-lives here because net production terms as observed in the experiments cannot be represented and would result in unphysical, negative values for τ.
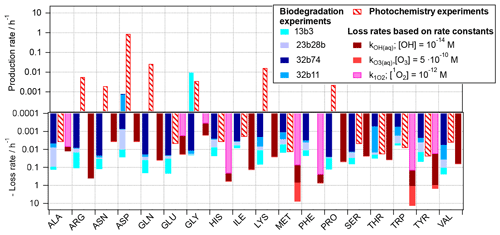
Figure 3Reaction rates for 18 amino acids as observed based on experiments in the present study, scaled to atmospheric conditions (Eqs. 2 and 3) and rates for loss reactions by OH(aq), O3(aq), and 1O2(aq) (Eq. 4).
The three rates, i.e., the biodegradation (Eq. 2) and photochemical (Eq. 3) rates as derived from the experiments, and the kinetic loss rates based on chemical kinetics (Eq. 4), respectively, are compared in Fig. 3 for each of the 18 amino acids. For some of the acids (ALA, GLU, and THR) the predicted losses by OH from both approaches (photochemical experiments (red dashed bars) and based on OH kinetic data (solid dark red bars)) are similar. Thus, we can conclude that these acids are oxidized to products other than amino acids and that the approximation of their loss rates by Eq. (4) is justified, as it has been done in previous studies, e.g., McGregor and Anastasio (2001) and Triesch et al. (2021). For several other amino acids (e.g., ARG, GLN, LYS, SER, and THR) there is a large discrepancy in the observed trends of the predicted chemical loss rates and the ones observed in the photochemical experiments. The latter ones have positive values; i.e., they indicate a net production rather than a net loss. While we cannot conclude on the exact conversion and formation mechanisms of these acids based on our experiments, it is evident that the assumption of a net loss underestimates the lifetime of these acids, as they do not only have chemical sinks but also sources in the atmospheric aqueous phase. As also reflected in Fig. 1, such net production is only seen for ASP and GLY for biotic processes.
The comparison of the predicted role of the three oxidants in cloud water (OH, O3, and 1O2) reveals that for some AAs the oxidation by ozone might contribute significantly more to their loss than the other two oxidants (light-red bars; note the logarithmic scale; i.e., the contributions of the ozone reactions to the total predicted loss greatly exceed those by other oxidants).
For several of the acids (e.g., ALA, ASN, GLU, PRO, and VAL), biotransformation is predicted to exceed the loss by chemical reactions, for the bacteria cell and oxidant concentrations considered here. Given that the ratios of bacteria cells and radicals in our estimate here are similar to those as encountered in cloud water, it may be concluded that both types of pathways might compete in the atmosphere. Similar conclusions were qualitatively drawn based on ambient measurement in a recent study (Zhu et al., 2020a). However, the exact contributions of biotic and abiotic pathways to the loss and conversion of amino acids will depend on the cell concentrations of the different bacteria strains, their distribution among cloud droplets, and oxidant levels.
3.3.3 Amino acid conversions
The oxidation of amino acids by a variety of oxidants has been performed in lab experiments. Results of such experiments are summarized in Table S4. Generally most oxidation reactions lead to smaller fragmentation products and not to amino acids, independent of the oxidant. A detailed discussion of the previously suggested reaction mechanisms of OH- and/or HO2 / O-initiated amino acid oxidation has been given by Stadtman and Levine (2004). The studies summarized in Table S4 were not motivated by the investigation of amino acid oxidation pathways in the atmospheric aqueous phase. However, our experimental results suggest that some of the amino acids may be the product of oxidation reactions from precursor amino acids, in qualitative agreement with some of the experiments listed in Table S4. The products and their distributions, however, are different than in the metabolic pathways shown in the KEGG mechanism (Fig. S3). There are some similarities between the biotransformation and oxidation products, such as the formation of aspartic acid and asparagine from histidine, tyrosine formation from phenylalanine, and glutamic acid formation from proline. However, as the yields in the oxidation reactions were not reported, the efficiency of the various pathways for the formation of these acids cannot be estimated.
Our experiments suggest that amino acids can not only be chemically degraded in cloud water but also produced. While such transformation cycles are known from biological systems (KEGG mechanism, Fig. S3), the production of amino acids by oxidation reactions in cloud water has not been discussed in the literature. Previous model studies of amino acids in the atmospheric aqueous phase only compared the half-lives of the acids to each other or for different oxidants, solely based on kinetic data (McGregor and Anastasio, 2001; Triesch et al., 2021). Our study suggests that such estimates underestimate the concentrations of amino acids in the atmosphere, since they ignore any production. These findings are qualitative, as the product yields and distributions are not known. Many of the experiments listed in Table S4 were performed under conditions that are not necessarily atmospherically relevant.
We measured the biotic (microbial) transformation rates of 18 amino acids with four bacteria strains (Pseudomonas graminis PDD-13b-3, Rhodococcus enclensis PDD-23b-28, Sphingomonas sp. PDD-32b-11, and Pseudomonas syringae PDD-32b-74) that have been previously identified as being representative of the microbial communities in cloud water. At the same time, we also determined the abiotic (chemical, OH radical) transformation rates within the same solutions that resembled the composition of cloud water. We used a new approach by UPLC–HRMS to quantify free AA directly in the artificial-cloud water medium without concentration and derivatization, improving the technique used in cloud water by Triesch et al. (2021). This direct MS method avoids time-consuming work and potential biases.
We used our experimentally derived transformation rates to compare their relative importance under atmospheric conditions, i.e., for atmospherically relevant bacteria cell and OH concentrations in cloud water. These rates were compared to the chemical loss rates based on kinetic data of oxidation reactions of amino acids in the aqueous phase, as they were used previously to derive lifetimes of amino acids in the atmosphere. Our experiments show that previous estimates overestimated the degradation rates and thus underestimated the lifetime of amino acids in the atmosphere, as they only considered kinetic data describing loss processes but did not take into account the transformation of amino acids into each other. While such transformation cycles are well-known for metabolic pathways (KEGG pathways), the mechanisms for chemical transformations are poorly constrained.
Our study qualitatively suggests that the sources and distribution of amino acids in the atmospheric particle and aqueous phases can be modified by metabolic- and chemical-transformation pathways. The distribution and abundance of specific amino acids in particles has been used in previous studies to conclude on aerosol sources (Barbaro et al., 2014, 2015). However, efficient abiotic and or biotic amino acid transformations during aerosol transport might alter the distribution and concentrations of amino acids so that source contributions might be more complex.
Free amino acids can represent up to 5 % of WSOC (water-soluble organic carbon) in submicron-sized particles but only 0.04 % of WSOC in supermicron-sized particles (Triesch et al., 2021) or 9.1 % of DOC (dissolved organic carbon) in cloud water (Bianco et al., 2016a). Free AA can also represent 0.4 % and 0.05 % of WSON (water-soluble organic nitrogen) in submicron- and supermicron-sized particles (Triesch et al., 2021). Total hydrolyzed AA (THAA) can account for 0.7 % to 1.8 % of DOC and 3.8 % to 6.0 % of DON (dissolved organic nitrogen) in rain samples (Yan et al., 2015) and 6.2 % to 23 % of DOC in a fog sample (Zhang and Anastasio, 2003). Considering that WSON contributes to 25 % of TDN (total dissolved nitrogen) of ambient aerosols (Lesworth et al., 2010) and WSOC contributes to 20 % of TOC (Saxena and Hildemann, 1996), the understanding of the lifetime and transformation rates of amino acids are essential in order to characterize their atmospheric abundance and residence time. Our study highlights the need for further mechanistic investigations of the biotic (metabolic) and abiotic (chemical) transformations of amino acids under conditions relevant for the atmospheric aqueous phases (clouds, fogs, and aerosols). Such data should be used in atmospheric multiphase models to explore the role and competition of biotic and abiotic processes for the transformation and loss of amino acids and related compounds.
All data can be obtained from the authors upon request.
This work does not involve human or animal subject. There is no ethical problem.
The supplement related to this article is available online at: https://doi.org/10.5194/bg-18-1067-2021-supplement.
AMD designed the experiments in microcosms. SJ, ML, MB, and MJ performed the experiments. BE, MJ, and AK made the calculations for the abiotic and biotic transformations. AMD, MJ, and BE wrote the paper. All the authors read and corrected the paper.
The authors declare that they have no conflict of interest.
The authors thank the I-Site CAP 20-25.
This work was funded by the French National Research Agency (ANR) in the framework of the “Investment for the Future” program, ANR-17-MPGA-0013. Saly Jaber is recipient of a school grant from the Walid Joumblatt Foundation for University Studies (WJF), Beirut, Lebanon and Maxence Brissy is a recipient of a school grant from Clermont Auvergne Metropole.
This paper was edited by Anja Engel and reviewed by Zhanfei Liu and one anonymous referee.
Amato, P., Parazols, M., Sancelme, M., Laj, P., Mailhot, G., and Delort, A.-M.: Microorganisms isolated from the water phase of tropospheric clouds at the puy de Dôme: major groups and growth abilities at low temperatures, FEMS Microbiol. Ecol., 59, 242–254, https://doi.org/10.1111/j.1574-6941.2006.00199.x, 2007.
Amato, P., Joly, M., Besaury, L., Oudart, A., Taib, N., Moné, A. I., Deguillaume, L., Delort, A.-M., and Debroas, D.: Active microorganisms thrive among extremely diverse communities in cloud water, PLOS ONE, 12, e0182869, https://doi.org/10.1371/journal.pone.0182869, 2017.
Amato, P., Besaury, L., Joly, M., Penaud, B., Deguillaume, L., and Delort, A.-M.: Metatranscriptomic exploration of microbial functioning in clouds, Sci. Rep., 9, 4383, https://doi.org/10.1038/s41598-019-41032-4, 2019.
Arakaki, T., Anastasio, C., Kuroki, Y., Nakajima, H., Okada, K., Kotani, Y., Handa, D., Azechi, S., Kimura, T., Tsuhako, A., and Miyagi, Y.: A General Scavenging Rate Constant for Reaction of Hydroxyl Radical with Organic Carbon in Atmospheric Waters, Environ. Sci. Technol., 47, 8196–8203, https://doi.org/10.1021/es401927b, 2013.
Ariya, P. A., Nepotchatykh, O., Ignatova, O., and Amyot, M.: Microbiological degradation of atmospheric organic compounds, Geophys. Res. Lett., 29, 2077, https://doi.org/10.1029/2002GL015637, 2002.
Barbaro, E., Zangrando, R., Moret, I., Barbante, C., Cescon, P., and Gambaro, A.: Free amino acids in atmospheric particulate matter of Venice, Italy, Atmos. Environ., 45, 5050–5057, https://doi.org/10.1016/j.atmosenv.2011.01.068, 2011.
Barbaro, E., Zangrando, R., Vecchiato, M., Piazza, R., Capodaglio, G., Barbante, C., and Gambaro, A.: Amino acids in Antarctica: evolution and fate of marine aerosols, Atmos. Chem. Phys. Discuss., 14, 17067–17099, https://doi.org/10.5194/acpd-14-17067-2014, 2014.
Barbaro, E., Zangrando, R., Vecchiato, M., Piazza, R., Cairns, W. R. L., Capodaglio, G., Barbante, C., and Gambaro, A.: Free amino acids in Antarctic aerosol: potential markers for the evolution and fate of marine aerosol, Atmos. Chem. Phys., 15, 5457–5469, https://doi.org/10.5194/acp-15-5457-2015, 2015.
Berger, P., Leitner, N., Karpel, V., Doré, M., and Legube, B.: Ozone and hydroxyl radicals induced oxidation of glycine, Water Res., 33, 433–441, https://doi.org/10.1016/S0043-1354(98)00230-9, 1999.
Besaury, L., Amato, P., Wirgot, N., Sancelme, M., and Delort, A. M.: Draft Genome Sequence of Pseudomonas graminis PDD-13b-3, a Model Strain Isolated from Cloud Water, Genome Announc., 5, e00464-17, https://doi.org/10.1128/genomeA.00464-17, 2017a.
Besaury, L., Amato, P., Sancelme, M., and Delort, A. M.: Draft Genome Sequence of Pseudomonas syringae PDD-32b-74, a Model Strain for Ice-Nucleation Studies in the Atmosphere, Genome Announc., 5, e00742-17, https://doi.org/10.1128/genomeA.00742-17, 2017b.
Bianco, A., Voyard, G., Deguillaume, L., Mailhot, G., and Brigante, M.: Improving the characterization of dissolved organic carbon in cloud water: amino acids and their impact on the oxidant capacity, Sci. Rep., 6, 37420, https://doi.org/10.1038/srep37420, 2016a.
Bianco, A., Passananti, M., Deguillaume, L., Mailhot, G., and Brigante, M.: Tryptophan and tryptophan-like substances in cloud water: Occurrence and photochemical fate, Atmos. Environ., 137, 53–61, https://doi.org/10.1016/j.atmosenv.2016.04.034, 2016b.
Bianco, A., Deguillaume, L., Vaïtilingom, M., Nicol, E., Baray, J.-L., Chaumerliac, N., and Bridoux, M.: Molecular Characterization of Cloud Water Samples Collected at the Puy de Dôme (France) by Fourier Transform Ion Cyclotron Resonance Mass Spectrometry, Environ. Sci. Technol., 52, 10275–10285, https://doi.org/10.1021/acs.est.8b01964, 2018.
Cape, J. N., Cornell, S. E., Jickells, T. D., and Nemitz, E.: Organic nitrogen in the atmosphere – Where does it come from? A review of sources and methods, Atmos. Res., 102, 30–48, https://doi.org/10.1016/j.atmosres.2011.07.009, 2011.
Cornell, S. E.: Atmospheric nitrogen deposition: Revisiting the question of the importance of the organic component, Environ. Pollut., 159, 2214–2222, https://doi.org/10.1016/j.envpol.2010.11.014, 2011.
Deguillaume, L., Charbouillot, T., Joly, M., Vaïtilingom, M., Parazols, M., Marinoni, A., Amato, P., Delort, A.-M., Vinatier, V., Flossmann, A., Chaumerliac, N., Pichon, J. M., Houdier, S., Laj, P., Sellegri, K., Colomb, A., Brigante, M., and Mailhot, G.: Classification of clouds sampled at the puy de Dôme (France) based on 10 yr of monitoring of their physicochemical properties, Atmos. Chem. Phys., 14, 1485–1506, https://doi.org/10.5194/acp-14-1485-2014, 2014.
Faust, B. C. and Allen, J. M.: Aqueous-phase photochemical sources of peroxyl radicals and singlet molecular oxygen in clouds and fog, J. Geophys. Res.-Atmos., 97, 12913–12926, https://doi.org/10.1029/92JD00843, 1992.
Helin, A., Sietiö, O.-M., Heinonsalo, J., Bäck, J., Riekkola, M.-L., and Parshintsev, J.: Characterization of free amino acids, bacteria and fungi in size-segregated atmospheric aerosols in boreal forest: seasonal patterns, abundances and size distributions, Atmos. Chem. Phys., 17, 13089–13101, https://doi.org/10.5194/acp-17-13089-2017, 2017.
Hill, K. A., Shepson, P. B., Galbavy, E. S., Anastasio, C., Kourtev, P. S., Konopka, A. and Stirm, B. H.: Processing of atmospheric nitrogen by clouds above a forest environment, J. Geophys. Res.-Atmos., 112, https://doi.org/10.1029/2006JD008002, 2007.
Husárová, S., Vaïtilingom, M., Deguillaume, L., Traikia, M., Vinatier, V., Sancelme, M., Amato, P., Matulová, M., and Delort, A.-M.: Biotransformation of methanol and formaldehyde by bacteria isolated from clouds. Comparison with radical chemistry, Atmos. Environ., 45, 6093–6102, https://doi.org/10.1016/j.atmosenv.2011.06.035, 2011.
Ignatenko, A. and Cherenkevich, S.: Reactivity of amino acids and proteins in reactions with ozone, Kinet. Katal., 26, 1332–1335, 1985.
Jaber, S., Lallement, A., Sancelme, M., Leremboure, M., Mailhot, G., Ervens, B., and Delort, A.-M.: Biodegradation of phenol and catechol in cloud water: comparison to chemical oxidation in the atmospheric multiphase system, Atmos. Chem. Phys., 20, 4987–4997, https://doi.org/10.5194/acp-20-4987-2020, 2020.
KEGG pathway database: available at: https://www.genome.jp/kegg/pathway.html, last access: 18 January 2021.
Kraljić, I. and Sharpatyi, V. A.: Determination of singlet oxygen rate constants in aqueous solution, Photochem. Photobiol., 28, 583–586, https://doi.org/10.1111/j.1751-1097.1978.tb06973.x, 1978.
Kristensson, A., Rosenørn, T., and Bilde, M.: Cloud Droplet Activation of Amino Acid Aerosol Particles, J. Phys. Chem. A, 114, 379–386, https://doi.org/10.1021/jp9055329, 2010.
Lallement, A., Besaury, L., Eyheraguibel, B., Amato, P., Sancelme, M., Mailhot, G., and Delort, A. M.: Draft Genome Sequence of Rhodococcus enclensis 23b-28, a Model Strain Isolated from Cloud Water, Genome Announc., 5, e01199-17, https://doi.org/10.1128/genomeA.01199-17, 2017.
Lallement, A., Vinatier, V., Brigante, M., Deguillaume, L., Delort, A. M., and Mailhot, G.: First evaluation of the effect of microorganisms on steady state hydroxyl radical concentrations in atmospheric waters, Chemosphere, 212, 715–722, https://doi.org/10.1016/j.chemosphere.2018.08.128, 2018.
Lesworth, T., Baker, A. R., and Jickells, T.: Aerosol organic nitrogen over the remote Atlantic Ocean, Atmos. Environ., 44, 1887–1893, https://doi.org/10.1016/j.atmosenv.2010.02.021, 2010.
Lide, D. R.: CRC Handbook of Chemistry and Physics, 89th ed., CRC Press/Taylor and Francis, Boca Raton, FL, 2736 pp., 2009.
Mace, K. A., Duce, R. A., and Tindale, N. W.: Organic nitrogen in rain and aerosol at Cape Grim, Tasmania, Australia, J. Geophys. Res., 108, 4338, https://doi.org/10.1029/2002JD003051, 2003a.
Mace, K. A., Kubilay, N., and Duce, R. A.: Organic nitrogen in rain and aerosol in the eastern Mediterranean atmosphere: An association with atmospheric dust, J. Geophys. Res.-Atmos., 108, https://doi.org/10.1029/2002JD002997, 2003b.
Manfrin, A., Nizkorodov, S. A., Malecha, K. T., Getzinger, G. J., McNeill, K., and Borduas-Dedekind, N.: Reactive Oxygen Species Production from Secondary Organic Aerosols: The Importance of Singlet Oxygen, Environ. Sci. Technol., 53, 8553–8562, https://doi.org/10.1021/acs.est.9b01609, 2019.
Marion, A., Brigante, M., and Mailhot, G.: A new source of ammonia and carboxylic acids in cloud water: The first evidence of photochemical process involving an iron-amino acid complex, Atmos. Environ., 195, 179–186, https://doi.org/10.1016/j.atmosenv.2018.09.060, 2018.
Mashayekhy Rad, F., Zurita, J., Gilles, P., Rutgeerts, L. A. J., Nilsson, U., Ilag, L. L., and Leck, C.: Measurements of Atmospheric Proteinaceous Aerosol in the Arctic Using a Selective UHPLC/ESI-MS/MS Strategy, J. Am. Soc. Mass Spectr., 30, 161–173, https://doi.org/10.1007/s13361-018-2009-8, 2019.
Matheson, I. B. C. and Lee, J.: Chemical reaction rates of amino acids with singlet oxygen, Photochem. Photobiol., 29, 879–881, https://doi.org/10.1111/j.1751-1097.1979.tb07786.x, 1979.
Matos, J. T. V., Duarte, R. M. B. O., and Duarte, A. C.: Challenges in the identification and characterization of free amino acids and proteinaceous compounds in atmospheric aerosols: A critical review, TrAC, 75, 97–107, https://doi.org/10.1016/j.trac.2015.08.004, 2016.
Matsumoto, K. and Uematsu, M.: Free amino acids in marine aerosols over the western North Pacific Ocean, Atmos. Environ., 39, 2163–2170, https://doi.org/10.1016/j.atmosenv.2004.12.022, 2005.
McGregor, K. G. and Anastasio, C.: Chemistry of fog waters in California's Central Valley: 2. Photochemical transformations of amino acids and alkyl amines, Atmos. Environ., 35, 1091–1104, https://doi.org/10.1016/s1352-2310(00)00282-x, 2001.
Michaeli, A. and Feitelson, J.: Reactivity of singlet oxygen toward amino acids and peptides, Photochem. Photobiol., 59, 284–289, https://doi.org/10.1111/j.1751-1097.1994.tb05035.x, 1994.
Miskoski, S. and García, N.: Influence of the peptide bond on the singlet molecular oxygen-mediated (O2[1 δ g]) photooxidation of histidine and methionine dipeptides. A kinetic study, Photochem. Photobiol., 57, 447–452, https://doi.org/10.1111/j.1751-1097.1993.tb02317.x, 1993.
Miyazaki, Y., Kawamura, K., Jung, J., Furutani, H., and Uematsu, M.: Latitudinal distributions of organic nitrogen and organic carbon in marine aerosols over the western North Pacific, Atmos. Chem. Phys., 11, 3037–3049, https://doi.org/10.5194/acp-11-3037-2011, 2011.
Mopper, K. and Zika, R. G.: Free amino acids in marine rains: evidence for oxidation and potential role in nitrogen cycling, Nature, 325, 246–249, https://doi.org/10.1038/325246a0, 1987.
Motohashi, N. and Saito, Y.: Competitive Measurement of Rate Constants for Hydroxyl Radical Reactions Using Radiolytic Hydroxylation of Benzoate, Chem. Pharma. Bull., 41, 1842–1845, https://doi.org/10.1248/cpb.41.1842, 1993.
Mudd, J. B., Leavitt, R., Ongun, A., and McManus, T. T.: Reaction of ozone with amino acids and proteins, Atmos. Environ., 3, 669–681, https://doi.org/10.1016/0004-6981(69)90024-9, 1969.
Pattison, D. I., Rahmanto, A. S., and Davies, M. J.: Photo-oxidation of proteins, Photochem. Photobiol. Sci., 11, 38–53, https://doi.org/10.1039/c1pp05164d, 2012.
Prasse, C., Ford, B., Nomura, D. K., and Sedlak, D. L.: Unexpected transformation of dissolved phenols to toxic dicarbonyls by hydroxyl radicals and UV light, P. Natl. Acad. Sci. USA, 115, 2311, https://doi.org/10.1073/pnas.1715821115, 2018.
Prütz, W. A. and Vogel, S.: Specific Rate Constants of Hydroxyl Radical and Hydrated Electron Reactions Determined by the RCL Method, Z. Naturforsch., 31, 1501–1510, https://doi.org/10.1515/znb-1976-1115, 1976.
Pryor, W. A., Giamalva, D. H., and Church, D. F.: Kinetics of Ozonation, 2. Amino Acids and Model Compounds in Water and Comparisons to Rates in Nonpolar Solvents, J. Am. Chem. Soc., 106, 7094–7100, https://doi.org/10.1021/ja00335a038, 1984.
Reasoner, D. J. and Geldreich, E. E.: A new medium for the enumeration and subculture of bacteria from potable water, Appl. Environ. Microbiol., 49, 1–7, 1985.
Samy, S., Robinson, J., Rumsey, I. C., Walker, J. T., and Hays, M. D.: Speciation and trends of organic nitrogen in southeastern U.S. fine particulate matter (PM2.5), J. Geophys. Res.-Atmos., 118, 1996–2006, https://doi.org/10.1029/2012JD017868, 2013.
Sander, R.: Modeling Atmospheric Chemistry: Interactions between Gas-Phase Species and Liquid Cloud/Aerosol Particle, Surv. Geophys., 20, 1–31, 1999.
Saxena, P. and Hildemann, L. M.: Water-Soluble Organics in Atmospheric Particles: A Critical Review of the Literature and Application of Thermodynamics to Identify Candidate Compounds, J. Atmos. Chem., 24, 57–109, 1996.
Scheller, E.: Amino acids in dew – origin and seasonal variation, Atmos. Environ., 35, 2179–2192, https://doi.org/10.1016/S1352-2310(00)00477-5, 2001.
Scholes, G., Shaw, P., Wilson, R. L., and Ebert, M.: Pulse radiolysis studies of aqueous solutions of nucleic acid and related substances, in: Pulse Radiolysis, Academic Press, 151–164, 1965.
Sidle, A. B.: Amino acid content of atmospheric precipitation, Tellus, 19, 129–135, https://doi.org/10.3402/tellusa.v19i1.9757, 1967.
Song, T., Wang, S., Zhang, Y., Song, J., Liu, F., Fu, P., Shiraiwa, M., Xie, Z., Yue, D., Zhong, L., Zheng, J., and Lai, S.: Proteins and Amino Acids in Fine Particulate Matter in Rural Guangzhou, Southern China: Seasonal Cycles, Sources, and Atmospheric Processes, Environ. Sci. Technol., 51, 6773–6781, https://doi.org/10.1021/acs.est.7b00987, 2017.
Stadtman, E. R.: Oxidation of free amino acids and amino acid residues in proteins by radiolysis and by metal-catalyzed reactions, Annu. Rev. Biochem., 62, 797–821, 1993.
Stadtman, E. R. and Levine, R.: Free radical-mediated oxidation of free amino acids and amino acid residues in proteins, Amino Acids, 25, 207–18, https://doi.org/10.1007/s00726-003-0011-2, 2004.
Sutton, M. A., Howard, C. M., Erisman, J. W., Billen, G., Bleeker, A., Grennfelt, P., van Grinsven, H., and Grizzetti, B., Eds.: The European Nitrogen Assessment: Sources, Effects and Policy Perspectives, Cambridge University Press, Cambridge, 2011.
Szyrmer, W. and Zawadzki, I.: Biogenic and atnthropogenic sources of ice-forming nuclei: A review, B. Am. Meteorol. Soc., 78, 209–228, 1997.
Triesch, N., van Pinxteren, M., Engel, A., and Herrmann, H.: Concerted measurements of free amino acids at the Cabo Verde islands: high enrichments in submicron sea spray aerosol particles and cloud droplets, Atmos. Chem. Phys., 21, 163–181, https://doi.org/10.5194/acp-21-163-2021, 2021.
Vaïtilingom, M., Amato, P., Sancelme, M., Laj, P., Leriche, M. and Delort, A.-M.: Contribution of Microbial Activity to Carbon Chemistry in Clouds, Appl. Environ. Microbiol., 76, 23–29, https://doi.org/10.1128/AEM.01127-09, 2010.
Vaïtilingom, M., Charbouillot, T., Deguillaume, L., Maisonobe, R., Parazols, M., Amato, P., Sancelme, M., and Delort, A.-M.: Atmospheric chemistry of carboxylic acids: microbial implication versus photochemistry, Atmos. Chem. Phys., 11, 8721–8733, https://doi.org/10.5194/acp-11-8721-2011, 2011.
Vaïtilingom, M., Attard, E., Gaiani, N., Sancelme, M., Deguillaume, L., Flossmann, A. I., Amato, P., and Delort, A.-M.: Long-term features of cloud microbiology at the puy de Dôme (France), Atmos. Environ., 56, 88–100, doi:https://doi.org/10.1016/j.atmosenv.2012.03.072, 2012.
Xu, W., Sun, Y., Wang, Q., Du, W., Zhao, J., Ge, X., Han, T., Zhang, Y., Zhou, W., Li, J., Fu, P., Wang, Z., and Worsnop, D. R.: Seasonal Characterization of Organic Nitrogen in Atmospheric Aerosols Using High Resolution Aerosol Mass Spectrometry in Beijing, China, ACS Earth Space Chem., 1, 673–682, https://doi.org/10.1021/acsearthspacechem.7b00106, 2017.
Xu, Y., Wu, D., Xiao, H., and Zhou, J.: Dissolved hydrolyzed amino acids in precipitation in suburban Guiyang, southwestern China: Seasonal variations and potential atmospheric processes, Atmos. Environ., 211, 247–255, https://doi.org/10.1016/j.atmosenv.2019.05.011, 2019.
Yan, G., Kim, G., Kim, J., Jeong, Y.-S., and Kim, Y. I.: Dissolved total hydrolyzable enantiomeric amino acids in precipitation: Implications on bacterial contributions to atmospheric organic matter, Geochim. Cosmochim. Ac., 153, 1–14, https://doi.org/10.1016/j.gca.2015.01.005, 2015.
Yang, H., Xu, J., Wu, W.-S., Wan, C. H., and Yu, J. Z.: Chemical Characterization of Water-Soluble Organic Aerosols at Jeju Island Collected During ACE-Asia, Environ. Chem., 1, 13–17, 2004.
Zhang, Q. and Anastasio, C.: Free and combined amino compounds in atmospheric fine particles (PM2.5) and fog waters from Northern California, Atmos. Environ., 37, 2247–2258, 2003.
Zhang, Y., Song, L., Liu, X. J., Li, W. Q., Lü, S. H., Zheng, L. X., Bai, Z. C., Cai, G. Y., and Zhang, F. S.: Atmospheric organic nitrogen deposition in China, Atmos. Environ., 46, 195–204, https://doi.org/10.1016/j.atmosenv.2011.09.080, 2012.
Zhao, Y., Hallar, A. G., and Mazzoleni, L. R.: Atmospheric organic matter in clouds: exact masses and molecular formula identification using ultrahigh-resolution FT-ICR mass spectrometry, Atmos. Chem. Phys., 13, 12343–12362, https://doi.org/10.5194/acp-13-12343-2013, 2013.
Zhu, R., Xiao, H.-Y., Luo, L., Xiao, H., Wen, Z., Zhu, Y., Fang, X., Pan, Y., and Chen, Z.: Measurement report: Amino acids in fine and coarse atmospheric aerosol: concentrations, compositions, sources and possible bacterial degradation state, Atmos. Chem. Phys. Discuss. [preprint], https://doi.org/10.5194/acp-2020-534, in review, 2020a.
Zhu, R., Xiao, H.-Y., Zhu, Y., Wen, Z., Fang, X., and Pan, Y.: Sources and Transformation Processes of Proteinaceous Matter and Free Amino Acids in PM2.5, J. Geophys. Res.-Atmos., 125, e2020JD032375, https://doi.org/10.1029/2020JD032375, 2020b.