the Creative Commons Attribution 4.0 License.
the Creative Commons Attribution 4.0 License.
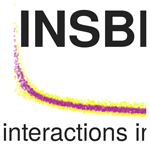
Complex interactions of in-stream dissolved organic matter and nutrient spiralling unravelled by Bayesian regression analysis
Matthias Pucher
Peter Flödl
Daniel Graeber
Klaus Felsenstein
Thomas Hein
Gabriele Weigelhofer
Uptake and release patterns of dissolved organic matter (DOM) compounds and co-transported nutrients are entangled, and the current literature does not provide a consistent picture of the interactions between the retention processes of DOM fractions. We performed plateau addition experiments with five different complex DOM leachates in a small experimental stream impacted by diffuse agricultural pollution. The study used a wide range of DOM qualities by including leachates of cow dung, pig dung, corn leaves, leaves from trees, and whole nettle plants. We measured changes in nutrient and dissolved organic carbon (DOC) concentrations along the stream course and determined DOM fractions by fluorescence measurements and parallel factor (PARAFAC) decomposition. To assess the influences of hydrological transport processes, we used a 1D hydrodynamic model.
We developed a non-linear Bayesian approach based on the nutrient spiralling concept, which we named the “interactions in nutrient spirals using Bayesian regression” (INSBIRE) approach. This approach can disentangle complex interactions of biotic and abiotic drivers of reactive solutes' uptake in multi-component DOM sources. It can show the variability of the uptake velocities and quantify their uncertainty distributions. Furthermore, previous knowledge of nutrient spiralling can be included in the model using prior probability distributions. We used INSBIRE to assess interactions of compound-specific DOM and nutrient spiralling metrics in our experiment.
Bulk DOC uptake varied among sources, showing decreasing uptake velocities in the following order: corn > pig dung > leaves > nettles > cow dung. We found no correlations between bulk DOC uptake and the amounts of protein-like compounds or co-leached soluble reactive phosphorus (SRP). The fastest uptake was observed for SRP and the tryptophan-like component, while the other DOM components' uptake velocities more or less resembled that of the bulk DOC. Almost all DOM components showed a negative relationship between uptake and concentration, known as efficiency loss. Furthermore, we observed a few negative and (weak) positive interactions between the uptake and the concentration of different components, such as a decreased uptake of protein-like compounds at high concentrations of a high-molecular-weight humic-like compound. We also found an influence of the wetted width on the uptake of SRP and a microbially derived humic substance, which indicates the importance of the sediment–water interface for P and humic C cycling in the studied stream.
Overall, we show that bulk DOC is a weak predictor of DOC uptake behaviour for complex DOM leachates. Individual DOM compound uptake, including co-leached nutrients, is controlled by both internal (quality-related) and external (environmental) factors within the same aquatic ecosystem. We conclude that the cycling of different C fractions and their mutual interaction with N and P uptake in streams is a complex, non-linear problem, which can only be assessed with advanced non-linear approaches, such as the presented INSBIRE approach.
- Article
(4658 KB) -
Supplement
(320 KB) - BibTeX
- EndNote
Dissolved organic matter (DOM) from terrestrial sources plays a key role in the metabolism and the ecological state of streams and rivers by controlling the activity and the composition of microbial communities (e.g. Freixa et al., 2016) and influencing a variety of aquatic biogeochemical processes (Tank et al., 2010). The quantity and the quality of DOM affect the aquatic bacterial respiration (e.g. Besemer et al., 2009; Niño-García et al., 2016), change the ratio between autotrophy and heterotrophy (Lutz et al., 2012; Martínez et al., 2017), and influence the microbial uptake of dissolved inorganic nitrogen (DIN; e.g. Bernhardt and Likens, 2002; Taylor and Townsend, 2010; Wymore et al., 2016) and soluble reactive phosphorus (SRP; Gibson and O'Reilly, 2012; Stutter et al., 2020; Weigelhofer et al., 2020), amongst others.
The influence of DOM on nutrients is mutual (e.g. Mineau et al., 2013; Stutter et al., 2020; Weigelhofer et al., 2020) due to the demand of microbes for carbon (C), nitrogen (N), and phosphorus (P) in a molar ratio that approximates their C : N : P ratio (Small et al., 2009; Stutter et al., 2018; Welti et al., 2017; Godwin and Cotner, 2018). The importance of this stoichiometric control of nutrient and organic carbon uptake in streams and rivers has been known for some time (Cross et al., 2005; Dodds et al., 2004) but has increasingly gained attention over the last few years with the discovery of anthropogenic impacts on pristine C : N : P ratios and DOM compositions (Stutter et al., 2018; Xenopoulos et al., 2021). Intensive land use has changed the origin, amount, and transport of terrestrial DOM to streams, thereby probably altering the DOM in-stream processing (Weigelhofer et al., 2020).
According to ecological stoichiometry, in-stream DOM uptake and retention is primarily related to the availability of inorganic nutrients, whether they already exist in the freshwater ecosystem (environmental control of DOM uptake) or are provided by the DOM source itself (intrinsic control through the DOM quality; e.g. Bernhardt and McDowell, 2008; Graeber et al., 2015; Gücker et al., 2016; Wickland et al., 2012). Field and laboratory studies show that DOC uptake can be positively affected by N and P concentrations in the water column (Catalán et al., 2018; Mineau et al., 2013). DOC uptake is also high in N- and P-rich DOM sources, such as leaves from fertilized trees or agricultural areas (Mineau et al., 2013; Mutschlecner et al., 2018; Weigelhofer et al., 2020).
In addition to nutrient interactions, DOM uptake depends on the structure and the bioavailability of the individual DOM compounds (Guillemette and Giorgio, 2012; Mineau et al., 2016). High uptake rates have been observed for protein-rich, low-molecular-weight DOM sources such as leachates of fresh leaf litter, macrophytes, and periphyton (Berggren et al., 2010; Koehler et al., 2012). In contrast, the soil leachates' biodegradability has been described as generally low (e.g. Fellman et al., 2009b; Hansen et al., 2016). Finally, the in-stream uptake of DOM may be influenced by environmental factors other than nutrient concentrations, such as the hydrology and morphology of the respective reach or the biofilms' composition (Casas-Ruiz et al., 2017; Romani et al., 2004; Weigelhofer et al., 2020).
Thus, unravelling the underlying mechanisms of in-stream DOM uptake is complex. While a considerable part of the reactive N and P exists as small and simple molecules, dissolved organic carbon (DOC) is bound in a mixture of differently structured organic molecules, with retention times varying by several orders of magnitude (Cory and Kaplan, 2012; Mineau et al., 2016). The production of new compounds during DOM decomposition may further complicate an accurate assessment of the DOM uptake (Stevenson and He, 1990; Tsutsuki and Kuwatsuka, 1979). Mass balance approaches or calculations of first-order decay curves from addition experiments have been successfully used in numerous studies to estimate in-stream uptake of DIN, SRP, and bulk DOC (e.g. Bernhardt and McDowell, 2008; Catalán et al., 2018; Covino, 2012; Ensign and Doyle, 2005; Mineau et al., 2013; Schiller et al., 2011; Stream Solute Workshop, 1990; Weigelhofer et al., 2018b). However, these methods are often limited in quantifying the uptake of individual DOM components. This limitation restricts and complicates the analyses of interactions among different DOM components and their role in the overall DOC uptake (Mineau et al., 2013; Stream Solute Workshop, 1990; Weigelhofer, 2017; Weigelhofer et al., 2018b).
Our study aimed at investigating the effects of DOM quality on the in-stream DOM uptake. We developed an approach to quantify complex interactions between individual DOM compounds, including co-leached N and P, and to elucidate their combined role in the overall DOM retention. For this purpose, we performed several short-term plateau additions with different DOM sources in an agriculturally influenced headwater stream according to the nutrient spiralling concept (Stream Solute Workshop, 1990). We used leachates from natural and human sources (e.g. leaves, manure) to see how in-stream DOM processing may be altered due to anthropic land use changes. Because of the diverse composition of DOM, we decided to extend the equations from the nutrient spiralling concept (Stream Solute Workshop, 1990) and use a Bayesian approach to analyse interactions between and influences of different DOM components and nutrients, including uncertainty propagation. Bayesian statistics is a suitable tool for ecological and biogeochemical questions, allowing users to assess the natural variability and assign degrees of belief in hypotheses based on measured data (Arhonditsis et al., 2008; Berger and Berry, 1988; Cox, 1946; Ellison, 2004; Jaynes, 2003; McCarthy, 2007). We incorporated non-linear nutrient uptake models observed in previous studies, such as the Michaelis–Menten kinetics or the nutrient efficiency loss model (Dodds et al., 2002; O'Brien et al., 2007), in our approach to describe relationships between concentrations and uptake velocities mathematically. Our approach enabled us to (1) analyse how uptake processes of different components influence each other; (2) test our mathematically pre-formulated assumptions with the measured data, including the remaining errors; (3) consider the natural variability of each parameter; and (4) include knowledge of nutrient and DOC uptake kinetics from previous studies in our models. We called our approach “interactions in nutrient spirals using Bayesian regression” (INSBIRE). With INSBIRE, we addressed the following questions:
-
What are the differences in the bulk DOC uptake velocity of different leachates?
-
How do selected DOM components behave in comparison to the bulk DOC uptake velocity?
-
Which factors and interactions influence the uptake velocity of the bulk DOC, the individual DOM components, and the co-leached nutrients N and P?
We expected nutrient- and protein-rich leachates to show higher uptake velocities than the other leachates. Low-molecular-weight protein-like compounds would show a faster uptake, while high-molecular-weight, aromatic compounds would show a slower uptake than the bulk DOC. We also expected to find positive influences of co-transported nutrients on the bulk DOC uptake and negative influences of low-molecular-weight protein-like compounds on the uptake of high-molecular-weight, aromatic compounds.
2.1 Site description
The experiment was carried out in the Hydrological Open Air Laboratory (HOAL; Fig. 1) in Petzenkirchen, Austria (Blöschl et al., 2016; for further information and a detailed map, see https://hoal.hydrology.at/the-hoal, last access: 3 April 2021). The HOAL is a small catchment that was transformed into a hydrologic observatory to foster scientific research. It features several permanently installed sensors measuring discharge, different water parameters, and the weather. Many studies on surface and subsurface flow paths, evaporation, soil erosion, sediment transport, and nutrient dynamics have already been performed in the HOAL (Blöschl et al., 2016). The first-order stream has several inflows, including two natural springs, six drainage pipes, and one site with groundwater infiltration from a small wetland. The stream flows through a deciduous forest with two short open sections in the middle (points 4–5 and 7 in Fig. 2) dominated by herbaceous vegetation and grass on the banks. All inflows as well as the stream discharge are continuously monitored regarding water quantity and quality. Sediments are dominated by clay, which is washed in from the adjacent fields during storm events. Table 1 shows the extent and basic environmental characteristics of the stream.
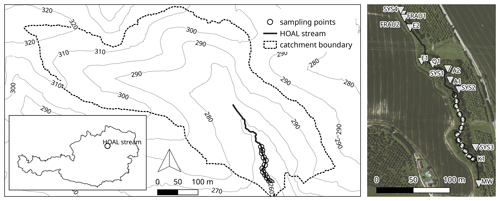
Figure 1Hydrologic Open Air Laboratory (HOAL): catchment, stream, sampling points, and location within Austria. A satellite image of the site also shows the inflows before and after the sampling stretch (catchment outlet: MW; inlet: SYS4; tile drains: FRAU1, FRAU2, SYS1, SYS2, and SYS3; erosion gullies: E1 and E2; springs: Q1 and K1; wetland run-off: A1 and A2). GIS data and aerial provided by the Bundesamt für Wasserwirtschaft in Petzenkirchen, Austria.
For the experiments, we chose a study reach of 215 m situated between two lateral inflows which was divided into 10 subsections of 16 to 26 m lengths, depending on the accessibility (Fig. 2). The first sampling point (1) was located 19.4 m downstream of the injection point (0) to ensure complete mixing of the solutes in the water column. Full mixing was determined by measuring the electrical conductivity across the channel transect during a salt addition before the experiments. The study reach was characterized by a meandering stream course with frequent pools (up to 24 cm in depth) between sampling points 8 and 11 (Fig. 2). Between point 4 and point 5, Equisetum palustre and Juncus sp. were growing in the open channel. At point 7, the patchy canopy cover facilitated the growth of algae on the stream bed. During the experiment, the median temperature was 16.7 ∘C (interquartile range = 2.4), and the median conductivity was 633 µS cm−1 (interquartile range = 23).
2.2 Experimental design
The experiment was performed over six consecutive weeks in July and August 2018. No major rain event occurred in the study area, and the average discharge was between 0.38 and 0.93 L s−1. Ten additions with DOM leachates from five different sources were injected into the study reach using short-term plateau additions according to the Stream Solute Workshop protocol (Stream Solute Workshop, 1990; Weigelhofer et al., 2012). The respective leachate plus a NaCl solution acting as a conservative tracer were pumped into the stream at point 0 over 2 to 2.5 h via a peristaltic pump (Fig. 2). We used a mobile conductivity meter to identify plateau conditions in the stream at each sampling point. Approximately 10 min after reaching stable plateau conditions, water samples were taken at each sampling point to analyse nutrient concentrations, organic carbon concentrations, and DOM composition. Thus, the sampling time exceeded the average water travel time by far, ensuring proper mixing and a stable state during sampling (see Fig. 2). After shutting the addition off, the change in conductivity was recorded until salt concentrations had returned to ambient levels. Additions were limited to a maximum of two times per week with at least 48 h between two consecutive samplings, allowing the system time to recover. Each leachate was added twice to the stream with an interval of 5–7 d to minimize adaptions of the microbial community and interferences among leachates. The additions created concentration peaks equal to or below local rain events. Each Monday, we sampled ambient concentrations to interpolate background conditions for the days with addition experiments. All samples were taken between 10:00 and 14:00 CEST to ensure comparability. As the environment changes naturally over time (e.g. discharge, temperature), different additions cannot be compared if the interval between them is too long. However, extremely short intervals and/or long addition times may lead to adaptions of the microbial community. Thus, the sampling schedule represents a compromise based on our experiences in nutrient addition experiments (Weigelhofer, 2017; Weigelhofer et al., 2012, 2018b) and on the long-term weather and discharge data of the stream. During our experiments, environmental changes were negligible due to highly stable weather conditions and the lack of human activities in the experimental area. We also observed no systematic changes of the DOM, N-NO3, and SRP uptake over time, indicating that any potential adaptions or responses of the microbial community to these short and low pulses did not affect the results of the study significantly.
2.3 Preparation of the leachates
The leachates were prepared from 50 g L−1 dry matter of cow and pig dung, foliage from local trees (Acer platanoides, Acer pseudoplatanus, Lonicera xylosteum, Pteridium aquilinum, Sambucus nigra), nettles (Urtica dioica), and corn plant (Zea mays) leaves. We leached the substrates with nutrient-poor water from a local well under aerated conditions in a barrel over 24 h. The leachates were filtered in steps of 2 and 0.5 mm using stainless-steel sieves and 50 µm using a 25 cm spun filter cartridge (PureOne PS-10). The end volume was between 40 and 60 L. To avoid post-leaching changes in DOM, the leachates were prepared freshly for each addition.
Average DOC concentrations in the stream water were about 1.3 mg L−1. We aimed to achieve an increase by about 3 mg L−1 DOC in the experiments. Some sources proved difficult to leach in sufficient amounts, and parts of the leached DOC were degraded even during short storage. Thus, the DOC increase achieved during the experiments was between 0.2 and 2.3 mg L−1. Even within the same source, leached amounts varied in concentration and composition between different additions. We consider these variations neglectable since we defined the leachates by their measured composition and not solely by their source. However, the fluctuations broadened the distributions of the measured values, providing more stable models and a more general picture of the uptake processes.
2.4 Analyses
Before the lab analyses, samples were filtered through pre-combusted Whatman glass microfiber filters, Grade GF/F (0.7 µm). We measured inorganic nitrogen as N-NO, nitrite (N-NO), and ammonium (N-NH) as well as SRP with a continuous-flow analyser (accuracy ±0.1 µg L−1). DOC was measured with a Sievers*900 portable total organic carbon analyser (accuracy ±2 %). We measured the DOM quality (excitation–emission matrices, or EEMs) via fluorescence spectroscopy with a Hitachi F-7000 fluorescence spectrophotometer and DOM absorbance with a Shimadzu UV-1700 spectrophotometer.
We analysed the data using R software version 3.5 (R Development Core Team, 2019) and tidyverse (Wickham et al., 2019). The DOM EEMs (11 sampling points, 16 sampling dates, 176 samples in total) were pre-processed using eemR (Massicotte, 2019); the fractions by fluorescence measurements and parallel factor (PARAFAC) analysis was done with staRdom (Pucher et al., 2019). The measured fluorescence EEMs were corrected for inner-filter effects, samples of ultra-pure water were subtracted, scatter bands were removed and interpolated, and the samples were normalized to Raman units. Samples were screened visually, and no unusual noise was found. After obtaining the first models, three outliers were identified using the samples' leverages and excluded from the model. The components' spectra were visually checked for plausibility. After that, a suitable model was validated using a split-half analysis. The final model did not express any problems related to those criteria. The outliers were included again to calculate loadings under the already-fixed components. To calculate the PARAFAC models and the split-half validation, we used 256 random initializations, a tolerance of 10−11, and staRdom's standard way of splitting the data (Pucher et al., 2019). We used Open-Fluor (Murphy et al., 2014) to compare and link the found components with other studies (Table 2).
2.5 Hydrodynamic modelling
A hydrodynamic 1D model was used to calculate the necessary hydraulic parameters using the software package HEC-RAS (Hydrologic Engineering Center's River Analysis System). To create the digital terrain model, a cross-sectional approach was applied, where 64 cross sections were recorded at a distance of 0.8 to 6.8 m depending on structural variations and accessibility. A total of 251 points were measured in the stream with a theodolite (Leica TC805) and then merged with a 1 × 1 m floodplain area model (based on the official laser scan data of the province of Lower Austria) using the software package Surface-water Modeling System (Aquaveo, LLC). The model was calibrated with the discharge data recorded at the HOAL site by comparing the measured water surface elevation with the modelled one. The calibrated 1D model was used to calculate the hydraulic parameters flow velocity, water depth, wetted width, and water travel time at each sampling point for each sampling day.
2.6 Calculating interactions in nutrient spirals using Bayesian regression (INSBIRE)
The uptake was calculated using a Bayesian non-linear model and solved with a Markov chain Monte Carlo (MCMC) algorithm as provided in the R package brms (Bürkner, 2017) relying on stan (Carpenter et al., 2017). We used the Bayes factor (BF; Goodman, 1999a, b) for hypothesis testing and model comparisons. The BF is the ratio of the marginal likelihood of two competing hypotheses or models. A BF of 10 favouring a particular hypothesis or model means that this model is 10 times more likely to explain the measured data. The interpretation of the BF was conducted according to Kass and Raftery (1995). There, the evidence is “barely noteworthy” (1 < BF < 3.2), “substantial” (3.2 < BF < 10), “strong” (10 < BF < 100) or “decisive” (BF > 100). A BF < 1 corresponds to the inverse of the BF but in favour of the other hypothesis. Model selection using the BF also allows removing models prone to collinearity problems (Ghosh and Ghattas, 2015). The Bayes R2 (Gelman et al., 2019) for each model was calculated to demonstrate the accuracy of the analysis.
We used the equations of the nutrient spiralling concept provided by the Stream Solute Workshop (1990) to develop our solute spiralling model, INSBIRE. All equations providing the basis of the model from the Stream Solute Workshop (1990) and all equations derived, transformed, and developed from these basic equations for the model development are shown and explained in detail in Sect. S1 in the Supplement. For a straightforward solving scheme like INSBIRE, a single-step analysis is necessary to determine all interdependent parameters' posterior distributions at once. Interactions, model weaknesses, collinearity (Ghosh and Ghattas, 2015), and the variation of parameters can then be assessed and interpreted consistently.
Commonly, uptake length (sw), uptake velocity (vf), and areal uptake rate (U) are used to describe nutrient uptake (Dodds et al., 2002; O'Brien et al., 2007; Trentman et al., 2015; Weigelhofer et al., 2018b). We fitted all three parameters to the equations. The uptake length sw is known to change with different discharges, while vf is independent of discharge (Dodds et al., 2002) and U is related to the solvent concentration. We used vf to address our research questions because the exclusion of hydrology provides the best conditions for analysing biogeochemical relationships. In alignment with Bayesian statistics (e.g. McCarthy, 2007), we defined prior distributions for each parameter based on the knowledge from other studies (e.g. Mineau et al., 2016) to keep the parameters (e.g. vf) within realistic ranges and foster a stable fitting procedure. We provide an exemplary R script that demonstrates INSBIRE (Pucher, 2020). A detailed mathematical description of the INSBIRE approach can be found in Sect. S1.
We used data from all experiments combined to perform the parameter estimation, thus increasing the number of points in our model. By doing that, we got a better insight into processes and interactions underlying uptake that can only be observed with different nutrient and DOM ratios. In contrast to a fitting algorithm, which determines only the most suitable value, the result of a Bayesian fitting is a distribution of probable parameter values showing the variability in the stream and between experiments. Furthermore, we set a threshold for complete retention of the added solutes, at which the difference between plateau and ambient conditions was equal to 2 times the accuracy of the lab analyses. Measured values below this threshold were removed from the analyses. Since the fluorescence of DOM increases linearly with concentration (Kothawala et al., 2013), we used Fmax of the PARAFAC components analogues to concentrations in these models.
We tested trends in the longitudinal nutrient concentrations by comparing the assumption of constant concentration with exponential decay as proposed in the nutrient spiralling concept (Stream Solute Workshop, 1990). The BF between those two models was calculated to show which one is more likely. For the analysis of the uptake of bulk DOM and individual components (research questions 1 and 2), we used the equations from the nutrient spiralling concept (Stream Solute Workshop, 1990) to calculate vf via a one-step fitting procedure (for details, see Sect. S1 and Eq. S4). By adding the leachate source to the uptake models as a random factor, we could determine quality-related differences between the leachate sources. We also added the sampling date as a random effect to our models to see if there are differences between the two sampling dates of the same leachate source (e.g. Ohno and Bro, 2006). A systematic change with the sampling date for all or at least most nutrients and components can also reveal experimental or analytical problems in the execution process but was not observed in this study.
The analyses of influencing factors in bulk DOM and component-specific uptake (research question 3) required the adaption of the original concept by including relationships among different DOM components and co-leached nutrients. This step was motivated by nutrient addition studies showing different uptake models such as linear functions, power functions (efficiency loss model), and Michaelis–Menten kinetics (Dodds et al., 2002; O'Brien et al., 2007; Trentman et al., 2015). We additionally tested an exponential function and an asymptotic regression function. We decided to present only the results of the power function (Eq. 1) because its inclusion in the models showed the highest BFs (highest probability to explain the observed data) in most cases. A considerable advantage of the power function is that there is only one parameter to fit, making it less prone to over-fitting in complex models (e.g. discussed in McElreath, 2016, chap. 7). Also, in our experiment, the concentrations of the DOM components and the co-leached nutrients did not reach uptake limits. In such cases, uptake rate curves often exhibit a power function, probably representing the lower unsaturated part of a saturation model within a concentration range naturally met in stream systems. O'Brien et al. (2007) limited the exponent of the power function (mi in Eq. 1) to negative values (m<1 for U, but m<0 for vf) to describe the efficiency loss behaviour. However, positive mi can also be used in the models to describe situations where a substance improves the uptake of another (e.g. Stutter et al., 2020). In our study, we looked for both positive and negative interactions among components, and thus we did not constrain the sign of mi. For positive exponents mi in Eq. (1), the function would pass through zero, which means that the absence of a stimulating component automatically leads to a total collapse of DOM or nutrient uptake. However, such a total collapse is not expected for DOM fractions since microbes can use other C sources. Thus, we incorporated an added value l in Eq. (1) as a degree of freedom, whose relevance was tested during the model selection process.
From a modelling point of view, any available variable can be included and tested at that point. We decided to include the wetted width in the formula because we expected an influence of the available benthic surface on the uptake processes. Furthermore, the stream showed a fluctuation of the wetted width by a factor of 2.8 between different dates and cross sections. The considerations made in this chapter resulted in the following equation:
where vf is nutrient uptake velocity; k is the uptake rate factor (model parameter); w is wetted width, constant at 1 to represent no influence (calculated by HEC-RAS and then fixed); l is the additive value (model parameter); i is the index of DOM component or nutrient; is the concentration of compound i at point x and date t (measured variable); and mi is an exponent determining the strength of the relations (model parameter).
The same fitting algorithm as for research questions 1 and 2 was used to derive the parameters k, mi, and l in Eq. (1). Additional information is provided in Sect. S1 and Eq. (S8). Relationships among components (including co-leached nutrients) were tested individually and in different combinations by adding factors of power functions according to the single factors in Eq. (1). The different combinations were compared to the initial model and to the next simpler models and were rated according to their BFs. When models with specific variables did not improve the predictability of the observed data, they were rejected and are not presented in the Results section below. By doing that, we determined models with meaningful component relationships and derived BFs for each variable included, representing the strength of evidence to support this inclusion.
For comparisons of uptake velocities of all co-leached nutrients and DOM fractions, we transformed the equation from the nutrient spiralling concept (Stream Solute Workshop, 1990) to derive vf for each nutrient and DOM component and between all pairs of sequent sampling points directly. Uptake velocities between nutrients and DOM fractions were compared using a Bayesian test for linear correlation (Jeffreys, 1998; Ly et al., 2016) implemented in the R package BayesFactor (Morey et al., 2018) to be aware of and avoid the effects of collinearity on the models calculated. Furthermore, the distributions of differences between vf of different DOM components and nutrients were calculated using a Monte Carlo simulation. In this way, we gained a probability distribution of differences that can be used to measure the evidence favouring a difference (motivated by the posterior distribution of the difference in means; Kruschke, 2013).
3.1 PARAFAC components
We could successfully fit a six-component PARAFAC model (Fig. 3, Table 2). Leachates of pig and cow dung exhibited high levels of tryptophan-like (Trp, C5) and tyrosine-like (Tyr, C6) compounds. Leaf leachate showed high peaks of microbially produced humic-like (Hum-mic, C1) fluorescence, which is assumed to represent low-molecular-weight, aliphatic DOM originating from microbial degradation. Ambient water was characterized by humic-like material from terrestrial sources (Hum-ter, C2) and microbially processed terrestrial DOM associated with agriculture (Hum-micter, C3). Another humic-like fluorophore, which showed a similar fluorescence to pure quinone, was identified in all sources (Qui, C4). The ambient DOM composition resembled the leachate of pig dung.
3.2 Ambient concentrations and introduced material
Peak DOC concentrations were highest for cow dung leachate, followed by corn and leaves, and lowest in nettles and pig dung (Fig. 4). Leachates of cow dung, pig dung, and leaves showed the highest concentrations of SRP. The overall background concentrations of N-NO3 were highly fluctuating, high in concentration, and hardly influenced by leachate additions. Most components declined during downstream travel, while Hum-ter (C2) and Hum-micter (C3) increased during corn and leaf additions. Concentrations and fluorescence tended to return to ambient conditions while travelling downstream. The BFs for an exponential decay during downstream travel compared to a conservative behaviour (no concentration change along the stream course) were 3.3 for DOC and larger than 107 for all DOM components and SRP. Thus, the evidence for an exponential decay curve was strong to decisive for these components. N-NO3 only exhibited a BF of 0.03, which means that there is strong evidence that the concentration was constant along the stream course during each sampling. The correlations of DOC, N-NO3, SRP concentrations, and the fluorescence-based concentrations of the DOM fractions can be found in Table 3.
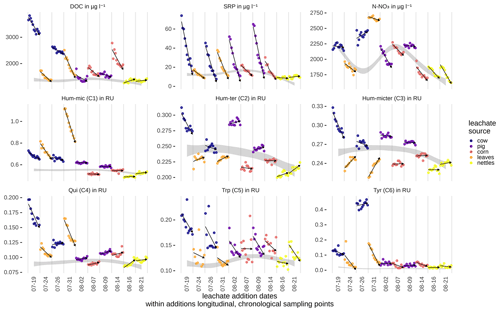
Figure 4Measured concentrations of DOC, SRP, and N-NO3, and Raman units (RUs) of DOM fractions along the stream course for the different samplings. The values are corrected for dilution effects (see also Table S1 in the Supplement). Arrows show the general trend of the concentrations/RUs from up- to downstream. The ambient concentrations were interpolated from measurements taken between leachate additions and are visualized as grey ribbons (see Table S1 for ambient conditions and additional amounts from leachate additions at the upstream station).
3.3 Uptake velocities of bulk DOC and DOM components
Probability densities of DOC uptake velocities from corn leachate, leaf leachate, and cow dung leachate were narrow (Fig. 5), allowing for a clear distinction of vf between these three leachates (Table 4). Here, corn leachate was taken up fastest, followed by leaf and cow dung leachate. During nettle and pig dung leachate additions, the DOC peaks were lower (Fig. 4) and measurement errors had a stronger relative influence, leading to broader posterior probability densities (Fig. 5). This hampered a clear separation of the vf of nettle and pig dung leachates from the other leachates' vf probabilities. Nevertheless, we can assume that the uptake velocities of nettles and pig dung leachates did not exceed 6 mm min−1 and that they were faster than the vf of cow dung leachate.
Table 4Comparison of vf of DOC depending on leachate source by the BF of one vf being lower than the other one. Additionally, the table presents median values of the vf distributions in millimetres per minute.
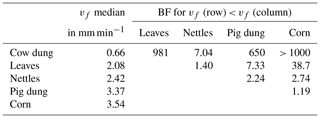
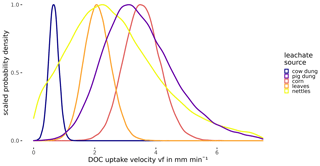
Figure 5Posterior density distribution curves of uptake velocity vf of DOC depending on the leachate source.
Modelling vf of the different components and nutrients without considering interactions or influencing factors showed that bulk DOC reflected the average uptake of the different DOM components. N-NO3 and Hum-mic (C1) were taken up slower, whereas SRP, Hum-micter (C3), and Trp (C5) were taken up faster than the bulk DOC and all other components (Fig. 6, Table 5).
Table 5Comparison of vf of DOM components and nutrients by the BF of one vf being lower than the other one. Additionally, the table presents median values of the vf distributions in millimetres per minute.
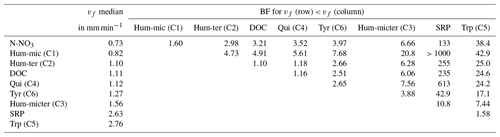
3.4 Influencing factors and component interactions
Differences between samplings using the same source can be caused by day-dependent characteristics, such as discharge and weather, or by differences in the leachate composition due to, e.g., degradation during storage. Hence, we tested whether the date of the different experiments and/or the source significantly affected the uptake of the bulk DOC, the DOM components, and the leached nutrients. Hum-mic (C1) retention was substantially (BF 4.6) and Trp (C5) retention was decisively (BF 134.2) influenced by the addition date. Bulk DOC and Tyr (C6) retentions were influenced by both the DOM source and the date. However, for bulk DOC retention, the source had a stronger effect (BF 1563) than the date (BF 146), while the reverse was true for Tyr (BF 10.7 and 108 for source and date, respectively). Hum-ter (C2), Hum-micter (C3), and Qui (C4) as well as SRP and N-NO3 showed conservative uptake behaviour independent of the source or the addition date (BF < 1; see also Table S3).
To further disentangle the interaction effects between nutrient and DOM component uptake, as proposed in Eq. (1), component concentrations or fluorescence and wetted width were included and evaluated in the models (Eq. 1; for details, see Sect. S1 and Eq. S8 in the Supplement).
Efficiency loss effects (i.e. decreasing uptake velocities of one component with increasing concentrations or fluorescence of the same component) were observed for SRP, Hum-mic (C1), Qui (C4), Trp (C5), and Tyr (C6) (Table 6, Fig. 7). The uptake velocities of both SRP and Hum-mic (C1) also increased with wetted width. Including wetted width and concentration improved the Hum-mic (C1) model even more than including the addition date (Table 6). Furthermore, we found several attenuating or stimulating effects among different components. Bulk DOC uptake velocity was lower at higher Tyr (C6) concentrations, although there is strong evidence that the leachate source variable offers a better explanation. Hum-ter (C2) retention was stimulated by higher DOC concentrations, and Qui (C4) retention decreased with increasing Hum-mic (C1). The Trp (C5) retention decreased with Hum-ter (C2), but the inclusion of this interaction could not outperform the model with the sampling date included. Tyr (C6) was also retained more slowly with higher fluorescence of Hum-ter (C2). While the Tyr (C6) model including the interaction with Hum-ter (C2) improved decisively compared to the simple model without interaction terms, the best performance could still be reached with the Tyr (C6) model including the sampling date. For Hum-micter (C3) and N-NO3, no effects of other variables could be identified with our models (Table 6, additional information in Tables S2 and S4).
We also analysed correlations between the uptake velocities of different DOM components to check for concurrent retention, indicating interrelations among or dependencies of different microbial processes, such as the combined need of these substances in the microbial metabolism (Table S2). We found a positive correlation between the vf of Qui (C4) and the vf of Tyr (C6) and DOC.
Table 6Interactions between uptake velocity and concentrations of other nutrients or DOM components using the INSBIRE approach. vf: uptake velocity; k: uptake rate factor; w: wetted width; Ci: fluorescence of PARAFAC components; mi: exponent of relation; l: additive parameter.
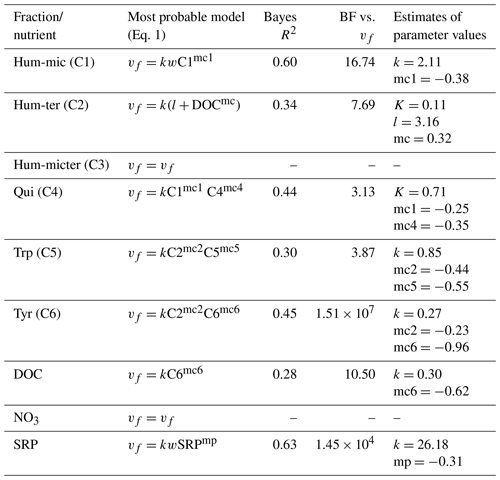
3.5 Brief propagation of uncertainty
The simulated probability density of the residuals (Fig. S1) was compared to the instruments' expected accuracy for DOC and SRP. The models depended on three measured values (Cx,t, Camb,t, ). So we multiplied the instrument errors by 3 to get the effect of their uncertainty on the model results. For DOC measurements with an accuracy of 2 %, three of our concentrations at around 2000 µg L−1 would have an approximate effect of 120 µg L−1 on the model uncertainty. The 95 % probability interval of the model residuals (mixed model including leachate source) was between −172 and 131 µg DOC L−1, which is already close to the error assumed from the measurements. Hence, the information drawn from the DOC data is exhausted, and we do not expect a more sophisticated model to reveal further details. In contrast, the instrument accuracy for SRP was 0.3 µg L−1, while the 95 % probability interval of the residuals was between −4.74 and 4.85 µg L−1 for the model including wetted width and SRP concentration (Table 6). Since the model residuals are higher than the assumed effect of the measurements, the SRP model still has potential for improvement by, e.g., adding other meaningful variables not measured in this study or by increasing the number of observations. Similar uncertainty analysis of the PARAFAC components is unfortunately not feasible because there is no conventional way of calculating the accuracy of the sample loadings of a PARAFAC model.
4.1 Uptake of bulk DOC from different sources
The uptake velocity of bulk DOC varied between leachate sources (Fig. 5), as observed in previous studies (e.g. Bernhardt and McDowell, 2008; Mineau et al., 2016; Mutschlecner et al., 2018). However, in contrast to our original hypothesis, neither a high-SRP content nor an increased amount of low-molecular-weight protein-like compounds could be linked to higher vf of bulk DOC (Table 2). Cow and pig dung leachates, for example, both had high peaks of the tryptophan-like component C5, which showed the fastest uptake of all DOM fractions, indicating a high bioavailability. Nevertheless, cow dung leachate was taken up most slowly, while pig dung leachate was among the leachates with the fastest uptake. Corn leachate showed the highest uptake of all sources, while the uptake velocity of leaf leachate was intermediate. Interestingly, the same sequence of increasing uptake velocities from cow dung leachate to leaf and corn leachate was observed in a laboratory flume experiment using the same organic matter sources as this field study but different sediments (Weigelhofer et al., 2020). However, in that study, DOC uptake was positively influenced by the SRP concentrations in the leachates. Comparisons with the literature are difficult, as other field and laboratory studies have used various organic matter sources. Among those, leaf leachates have been used most frequently so far. Leaf leachates show a wide range of biodegradability, depending on the species, the region, the pre-treatment, and the decomposition or leaching stage (e.g. Wickland et al., 2007). Long-term fertilization of trees, for example, resulted in an increased DOC uptake of leaf leachates due to their increased P content (Mutschlecner et al., 2018). Reported uptake velocities for leaf leachates range from 0.002 to 7.8 mm min−1, showing a high variability among leaf sources and aquatic systems (review by Mineau et al., 2016; Graeber et al., 2019). The median vf of our leaf leachate was 2.08 mm min−1 and thus lies within this range. Regarding the other sources used in our study, we only found one addition study using cow manure (Kuserk et al., 1984). There, the median uptake velocity was slightly lower (0.31 mm min−1; calculated in Mineau et al., 2016) than the one observed in our study (0.66 mm min−1).
4.2 Uptake of DOM fractions and nutrients
The various DOM fluorophores were retained with different uptake velocities, whereby the velocity density curves partly overlapped (Fig. 6, Table 5). Hum-mic (C1), described as a product of microbial degradation of terrestrial organic matter, showed the lowest vf of all components. Hum-ter (C2; high molecular weight, aromatic), Qui (C4; aromatic), and Tyr (C6; tyrosine-like) showed large overlaps and exhibited uptake velocities comparable to the bulk DOC, followed by slightly higher uptake velocities for Hum-micter (C3). As expected, the fastest uptake was observed for the tryptophan-like component Trp (C5), concordant with previous studies of different amino-acid-like fractions (Findlay and Sinsabaugh, 2003). Several studies report a high biodegradability of protein-like components, while humic-like, aromatic components have proved to be much more refractory (e.g. Fellman et al., 2009a, b; Casas-Ruiz et al., 2017). However, in contrast to Trp (C5), the tyrosine-like Tyr (C6) showed only medium uptake velocities. This may have been caused by the release of Tyr (C6) as a degradation product of humic substances during the experiment (Stevenson and He, 1990; Tsutsuki and Kuwatsuka, 1979). Other studies also report the generation of protein-like components during passage through the system due to either the release of algal exudates or the decomposition of humic substances (Casas-Ruiz et al., 2017; Weigelhofer et al., 2020).
The uptake of N-NO3 was the lowest of all components due to its high background concentrations in the water column exceeding even those of the ambient DOC (Fig. 4). In contrast, the co-leached SRP showed the highest uptake velocity (together with Trp, C5). An equally fast uptake was observed in flume experiments, especially in the presence of algae (Weigelhofer et al., 2020). Despite the low-to-moderate background concentrations of P in the stream water (Fig. 4), background molar C : P ratios in the water column of our study stream were usually below 80 : 1, displaying an ideal ratio for a vast number of bacterial strains (Cross et al., 2005; Godwin and Cotner, 2018). The C : P ratios were even decreased by the additions to < 30 : 1 at point 1, followed by an increase to background ratios in the downstream sections. While stoichiometry is a key factor for C, N, and P uptake (e.g. Cross et al., 2005; Gibson and O'Reilly, 2012; Stutter et al., 2020), we thus do not believe that stoichiometric control played a significant role in the P uptake in our study stream. Instead, we assume that the co-leached P was taken up faster than the DOC due to the P demand of both bacteria and algae (in contrast to the exclusive OC demand of heterotrophs; see, e.g., Oviedo-Vargas et al., 2013; Weigelhofer et al., 2020). In general, the bioavailability of a fraction does not only depend on the chemical composition but also on the ecosystem and the involved microbial community (Kamjunke et al., 2015), the overall availability of different fractions and nutrients (Berggren and Giorgio, 2015; Bernhardt and McDowell, 2008; Mutschlecner et al., 2018), and transport characteristics (Ejarque et al., 2017). We performed the experiments in a small homogeneous stretch of a stream and already found considerable variability in DOM fluorophore-specific uptake between sampling dates. Thus, we propose that the bioavailability of DOM fractions and sources should be increasingly determined in situ under different environmental conditions to determine the effective biodegradability range of the respective component.
4.3 Relationships between uptake and concentrations of other compounds
In contrast to our assumptions, we found no influence of the co-leached SRP on the bulk DOC uptake, although there is evidence in other studies that DOC uptake can be stimulated by P, especially in P-limited systems (Mutschlecner et al., 2018; Stutter et al., 2020). However, the molar C : P ratios were low in our stream, showing no P limitation. Furthermore, most P peaks during the additions were relatively small, containing only the leached P from the DOM sources. Thus, potentially stimulating effects of SRP on the DOC retention may have remained undetected. Although the source-independent model showed a relationship between the bulk DOC uptake velocity and the Tyr (C6) fluorescence, the mixed-effects model, including the leachate source, showed a higher probability of explaining the measured values. The better performance of the mixed-effects model indicates that other, probably non-fluorescent components, which we could not detect with our methods, influenced the bulk DOC uptake apart from Tyr. Almost all DOM fractions showed a negative relationship between uptake and concentration of the same component. Decreasing uptake velocities with increasing concentrations have been previously described for nitrogen as nutrient efficiency loss (Dodds et al., 2002; O'Brien et al., 2007). This efficiency loss may occur especially in streams where the microbial community is adapted to chronic loading, showing high flexibility towards increasing nutrient (or DOC) concentrations and thus a delayed saturation (e.g. O'Brien et al., 2007; Mulholland et al., 2009; Merseburger et al., 2011). Furthermore, transport limitations between the source in the water column and the reactive sites in the sediments (e.g. due to eutrophication and sediment clogging) may lead to delays and reductions in the microbial response to short nutrient peaks (Weigelhofer et al., 2018a, b; Teissier et al., 2007; Ribot et al., 2013). Hum-mic (C2), Hum-micter (C3), and DOC retention showed no evidence of efficiency loss (BF was around 1) at the measured concentrations, indicating that the microbial community would have been able to retain more of these substances without a decline in uptake velocity. So far, we have not found any other studies presenting efficiency loss or any other uptake–concentration relationship for DOM fractions.
Additionally, we observed both positive and negative interactions among different DOM fractions, with higher/lower uptake velocities of one component at increased concentrations of another component. These interactions can have various reasons and are, therefore, difficult to interpret. Positive effects on vf can arise, e.g., from the stimulation of the uptake of one substance by the presence of another through priming (but see critical discussion in Bengtsson et al., 2018). Direct negative effects can be caused by the preferential uptake of one fraction over another (Brailsford et al., 2019) or inhibitory effects between different substances (Freeman et al., 1990). Furthermore, the degradation of DOM can cause one molecule to break down into others, causing an increase of the degradation product, while the degraded component decreases (Kamjunke et al., 2017). In our study, the retention of Qui (C4) was lower at higher Hum-mic (C1) fluorescence. The molecular structures found in the literature (Stevenson and He, 1990; Tsutsuki and Kuwatsuka, 1979) suggest that Qui (C4) is a product of Hum-mic (C1) degradation, resulting in reduced net retention due to simultaneous production and degradation processes. Similarly, Trp (C5) and Tyr (C6) might have been degradation products of Hum-ter (C2). In contrast, Hum-ter (C2) degradation was stimulated by high DOC concentrations, probably due to the supply of energy in the form of carbohydrates or other essential components needed for degradation (Bengtsson et al., 2018; Bianchi et al., 2015). We also saw a weak probability that the uptake velocity of Hum-ter (C2) was stimulated by Qui (C4; BF = 1.9) and Tyr (C6; BF = 1.8). Based on the correlation of the uptake velocities, we found substantial evidence that Qui (C4) was degraded simultaneously with Tyr (C6; BF = 6.10) and bulk DOC (BF = 4.63). Good degradation conditions – such as low transport limitations (Weigelhofer et al., 2018b), ideal stoichiometric C : N : P ratios of the organic source for the microbial metabolism (Cross et al., 2005; Godwin and Cotner, 2018; Stutter et al., 2018), or stretch-wise more productive microbial communities – can foster simultaneous turnover (Guillemette and Giorgio, 2012). We consider concurrent degradation and negative and positive interactions to be essential characteristics of the complex DOM degradation processes. Using the INSBIRE approach in future experiments may help to elucidate which of the proposed mechanisms is responsible under certain environmental conditions.
Our models also revealed some hydromorphological effects on DOM fluorophore and nutrient uptake. The wetted width could partly explain the uptake of Hum-mic (C1) and SRP, probably due to adsorption to sediments or extracellular polymeric substances playing a role in retaining these compounds. The adsorption of humic substances to clay is generally strong when the ionic strength is high (Theng, 2012). The conductivity around 630 µS cm−1, which was measured during the experiment, and the clay-dominated sediments offered good conditions for adsorption (Theng, 2012). The role of the sediment surface in the uptake of solutes is not surprising and has been observed elsewhere (Romani et al., 2004; Sabater et al., 2002; Battin et al., 2016). However, the component-specific influence of wetted width suggests that different DOM components are preferably taken up in different stream compartments. Unlike the common assumption that uptake processes are dominated by the benthic community (Battin et al., 2016; Wiegner et al., 2005), Graeber et al. (2018) and Kamjunke et al. (2015) proposed a potentially important impact of planktonic bacteria on in-stream DOM uptake processes. In our study stream, such planktonic uptake might have controlled the uptake of most DOM fractions except Hum-ter (C1), where the substantial influence of wetted width indicates the importance of the benthic community.
4.4 Potential and limitations of the INSBIRE approach
The INSBIRE approach was only developed after the data from the experiment were acquired. Thus, our study represents a case study for the application of INSBIRE in the analysis of DOM uptake but does not claim to be a systematic check of the developed approach. Nevertheless, we can make some statements about the potential and the limitations of INSBIRE. INSBIRE helped to reveal positive and negative interactions among different DOM fractions previously not possible in such detail. We based our model on concepts, such as nutrient spiralling (Stream Solute Workshop, 1990) and Bayesian statistics, which have been investigated and developed for at least some decades. With this available knowledge, it was possible to develop the approach on a solid theoretical basis and with existing algorithms. However, INSBIRE can be adapted to various research questions by changing the underlying equations, using different solving schemes and different types of data. While we used fluorescence measurements to determine the DOM quality, INSBIRE can incorporate any other data of solvents (e.g. toxins or pesticides) and methods (e.g. mass spectroscopy, liquid chromatography). The power function has proven useful in our study, but the approach also facilitates the use of other equations. Due to the formal description of the uptake processes, extrapolations to ambient or event-related concentrations can be performed (Payn et al., 2005).
The presented plots of the vf posterior density curves are intuitive to interpret and can support our understanding of the retention processes. The presentation in the form of probability distributions instead of single values reflects the heterogeneity of ecosystems (McCarthy, 2007). For further studies, these posterior density curves can be directly used as prior information for similar models. The Bayesian nature of the analysis allows even weak relations to be evaluated, which can be tested in further experiments. We could also show the limitation of the bulk DOC retention model due to measurement accuracy and the heterogeneity of the different molecules. When only a small number of observations are available, data from previous studies and expert knowledge can be included using non-conservative prior densities of the parameters. In this way, results can be more precise, and decisions can be based on both measured data and available knowledge (Kuhnert et al., 2010; Lemoine, 2019). Even a low number of observations may show specific trends in DOM uptake (Fig. 5), which can be especially useful for monitoring or management decisions. We want to encourage systematic tests under controlled laboratory conditions to assess the full applicability of the INSBIRE approach for modelling the uptake of complex solutes.
Human impacts, such as agricultural land use or wastewater discharges, have changed the quantity and composition of terrestrially derived DOM in stream ecosystems. Our study demonstrates that in-stream DOM uptake is source-depended and thus influenced by DOM quality. However, bulk DOC uptake did not significantly correlate with any of the co-leached nutrients or individual fluorophores. One reason for this lack of correlation could be the complexity of DOM retention. DOM uptake comprises a variety of simultaneously and sequentially occurring microbial degradation and production processes. Hence, the INSBIRE approach provided evidence for positive and negative effects among the DOM components' uptake, indicating transformations of one substance into another during processing. Furthermore, the identification of different DOM components via spectroscopic characterization may be too imprecise to reveal influences of DOM components on DOM uptake. Non-fluorescent components, not detected by spectroscopy, may also play a role in bulk DOM uptake. Thus, further DOM uptake studies are required which identify important molecular groups – such as amino acids, sugars, and humic acids – more accurately.
Our study also shows that the uptake of bulk DOC and individual DOM components may be subject to efficiency loss, so far only known from nutrient uptake. This means that the uptake efficiency declines with increasing concentration of the respective component. However, individual DOM components were not equally affected by efficiency loss and interactions. Thus, we assume that the component-specific uptake capacity of benthic biofilms depends on the respective microbial processes involved. Further studies need to look more closely into the underlying mechanisms of efficiency loss and components interactions during DOM processing in aquatic ecosystems. Our study also demonstrates that the cycling of different C fractions and their mutual interaction with N and P uptake in streams is a complex, non-linear problem, requiring advanced non-linear approaches. Here, the developed INSBIRE approach may help in finding concurrent retention and interactions of DOM components, thus providing an efficient tool for the analysis and the management of organic carbon cycling in aquatic systems.
The code necessary for applying the INSBIRE approach can be downloaded from https://doi.org/10.5281/zenodo.4071851 (Pucher, 2020).
The supplement related to this article is available online at: https://doi.org/10.5194/bg-18-3103-2021-supplement.
The study concept was prepared by MP and GW. MP conducted the experiment. GW was responsible for the funding acquisition and the project administration. The methodology was developed by MP, GW, KF, and PF. The calculations and the underlying code were made by MP. TH and KF supervised the study from a biogeochemical and a statistical perspective respectively. The original draft was prepared by MP and GW with large contributions from DG. The review and editing was done by all co-authors.
The authors declare that they have no conflict of interest.
We would like to thank Verena Winiwarter and Astrid Harjung for their input on the text and the HR21 faculty and students for their thoughts in several discussions. The field and lab work would not have been possible without the help of Lenardo Zoltan, Yu-Ting Shi, Nikloaus Schobesberger, Isabella Fischer, Elmira Akbari, Ewelina Sonnenberg, André Fonseca, Annette Puritscher, Gertraud Stenicka, and Beate Pitzl. Additionally, we would like to thank the staff from the Bundesamt für Wasserwirtschaft in Petzenkirchen, Austria, especially Gerhard Rab, Alexander Eder, and Günther Schmid for their help at the HOAL stream.
This research has been supported by the NÖ Forschungs- und Bildungsgesellschaft mbH. (grant nos. SC15-002 and SC18-001) and the Universität für Bodenkultur Wien (doctoral school Human River Systems in the 21st century (grant no. HR21)).
This paper was edited by Gwenaël Abril and reviewed by two anonymous referees.
Arhonditsis, G. B., Papantou, D., Zhang, W., Perhar, G., Massos, E., and Shi, M.: Bayesian calibration of mechanistic aquatic biogeochemical models and benefits for environmental management, J. Marine Syst., 73, 8–30, https://doi.org/10.1016/j.jmarsys.2007.07.004, 2008.
Battin, T. J., Besemer, K., Bengtsson, M. M., Romani, A. M., and Packmann, A. I.: The ecology and biogeochemistry of stream biofilms, Nat. Rev. Microbiol., 14, 251–263, https://doi.org/10.1038/nrmicro.2016.15, 2016.
Bengtsson, M. M., Attermeyer, K., and Catalán, N.: Interactive effects on organic matter processing from soils to the ocean: are priming effects relevant in aquatic ecosystems?, Hydrobiologia, 822, 1–17, https://doi.org/10.1007/s10750-018-3672-2, 2018.
Berger, J. O. and Berry, D. A.: Statistical Analysis and the Illusion of Objectivity, Am. Sci., 76, 159–165, 1988.
Berggren, M. and del Giorgio, P. A.: Distinct patterns of microbial metabolism associated to riverine dissolved organic carbon of different source and quality, J. Geophys. Res.-Biogeo., 120, 989–999, https://doi.org/10.1002/2015JG002963, 2015.
Berggren, M., Laudon, H., Haei, M., Ström, L., and Jansson, M.: Efficient aquatic bacterial metabolism of dissolved low-molecular-weight compounds from terrestrial sources, ISME J., 4, 408–416, 2010.
Bernhardt, E. S. and Likens, G. E.: Dissolved Organic Carbon Enrichment Alters Nitrogen Dynamics in a Forest Stream, Ecology, 83, 1689–1700, https://doi.org/10.1890/0012-9658(2002)083[1689:DOCEAN]2.0.CO;2, 2002.
Bernhardt, E. S. and McDowell, W. H.: Twenty years apart: Comparisons of DOM uptake during leaf leachate releases to Hubbard Brook Valley streams in 1979 versus 2000, J. Geophys. Res.-Biogeo., 113, G03032, https://doi.org/10.1029/2007JG000618, 2008.
Besemer, K., Luef, B., Preiner, S., Eichberger, B., Agis, M., and Peduzzi, P.: Sources and composition of organic matter for bacterial growth in a large European river floodplain system (Danube, Austria), Org. Geochem., 40, 321–331, 2009.
Bianchi, T. S., Thornton, D. C. O., Yvon-Lewis, S. A., King, G. M., Eglinton, T. I., Shields, M. R., Ward, N. D., and Curtis, J.: Positive priming of terrestrially derived dissolved organic matter in a freshwater microcosm system, Geophys. Res. Lett., 42, 5460–5467, https://doi.org/10.1002/2015GL064765, 2015.
Blöschl, G., Blaschke, A. P., Broer, M., Bucher, C., Carr, G., Chen, X., Eder, A., Exner-Kittridge, M., Farnleitner, A., Flores-Orozco, A., Haas, P., Hogan, P., Kazemi Amiri, A., Oismüller, M., Parajka, J., Silasari, R., Stadler, P., Strauss, P., Vreugdenhil, M., Wagner, W., and Zessner, M.: The Hydrological Open Air Laboratory (HOAL) in Petzenkirchen: a hypothesis-driven observatory, Hydrol. Earth Syst. Sci., 20, 227–255, https://doi.org/10.5194/hess-20-227-2016, 2016.
Brailsford, F. L., Glanville, H. C., Golyshin, P. N., Johnes, P. J., Yates, C. A., and Jones, D. L.: Microbial uptake kinetics of dissolved organic carbon (DOC) compound groups from river water and sediments, Sci. Rep.-UK, 9, 11229, https://doi.org/10.1038/s41598-019-47749-6, 2019.
Bürkner, P.-C.: brms: An R Package for Bayesian Multilevel Models Using Stan, J. Stat. Softw., 80, 1–28, https://doi.org/10.18637/jss.v080.i01, 2017.
Carpenter, B., Gelman, A., Hoffman, M. D., Lee, D., Goodrich, B., Betancourt, M., Brubaker, M., Guo, J., Li, P., and Riddell, A.: Stan: A Probabilistic Programming Language, J. Stat. Softw., 76, 1–32, https://doi.org/10.18637/jss.v076.i01, 2017.
Casas-Ruiz, J. P., Catalán, N., Gómez-Gener, L., von Schiller, D., Obrador, B., Kothawala, D. N., López, P., Sabater, S., and Marcé, R.: A tale of pipes and reactors: Controls on the in-stream dynamics of dissolved organic matter in rivers, Limnol. Oceanogr., 62, 85–94, https://doi.org/10.1002/lno.10471, 2017.
Catalán, N., Casas-Ruiz, J. P., Arce, M. I., Abril, M., Bravo, A. G., del Campo, R., Estévez, E., Freixa, A., Giménez-Grau, P., González-Ferreras, A. M., Gómez-Gener, L., Lupon, A., Martínez, A., Palacin-Lizarbe, C., Poblador, S., Rasines-Ladero, R., Reyes, M., Rodríguez-Castillo, T., Rodríguez-Lozano, P., Sanpera-Calbet, I., Tornero, I., and Pastor, A.: Behind the Scenes: Mechanisms Regulating Climatic Patterns of Dissolved Organic Carbon Uptake in Headwater Streams, Global Biogeochem. Cy., 32, 1528–1541, https://doi.org/10.1029/2018GB005919, 2018.
Cory, R. M. and Kaplan, L. A.: Biological lability of streamwater fluorescent dissolved organic matter, Limnol. Oceanogr., 57, 1347–1360, https://doi.org/10.4319/lo.2012.57.5.1347, 2012.
Covino, T. P.: The role of stream network nutrient uptake kinetics and groundwater exchange in modifying the timing, magnitude, and form of watershed export, PhD thesis, Montana State University, Bozeman, Montana, 266 pp., 2012.
Cox, R. T.: Probability, Frequency and Reasonable Expectation, Am. J. Phys., 14, 1, https://doi.org/10.1119/1.1990764, 1946.
Cross, W. F., Benstead, J. P., Frost, P. C., and Thomas, S. A.: Ecological stoichiometry in freshwater benthic systems: recent progress and perspectives, Freshwater Biol., 50, 1895–1912, https://doi.org/10.1111/j.1365-2427.2005.01458.x, 2005.
Dodds, W. K., López, A. J., Bowden, W. B., Gregory, S., Grimm, N. B., Hamilton, S. K., Hershey, A. E., Martí, E., McDowell, W. H., Meyer, J. L., Morrall, D., Mulholland, P. J., Peterson, B. J., Tank, J. L., Valett, H. M., Webster, J. R., and Wollheim, W.: N uptake as a function of concentration in streams, J. N. Am. Benthol. Soc., 21, 206–220, https://doi.org/10.2307/1468410, 2002.
Dodds, W. K., Martí, E., Tank, J. L., Pontius, J., Hamilton, S. K., Grimm, N. B., Bowden, W. B., McDowell, W. H., Peterson, B. J., and Valett, H. M.: Carbon and nitrogen stoichiometry and nitrogen cycling rates in streams, Oecologia, 140, 458–467, 2004.
Ejarque, E., Freixa, A., Vazquez, E., Guarch, A., Amalfitano, S., Fazi, S., Romaní, A. M., and Butturini, A.: Quality and reactivity of dissolved organic matter in a Mediterranean river across hydrological and spatial gradients, Sci. Total Environ., 599–600, 1802–1812, https://doi.org/10.1016/j.scitotenv.2017.05.113, 2017.
Ellison, A. M.: Bayesian inference in ecology, Ecol. Lett., 7, 509–520, https://doi.org/10.1111/j.1461-0248.2004.00603.x, 2004.
Ensign, S. H. and Doyle, M. W.: In-channel transient storage and associated nutrient retention: Evidence from experimental manipulations, Limnol. Oceanogr., 50, 1740–1751, https://doi.org/10.4319/lo.2005.50.6.1740, 2005.
Fellman, J. B., Miller, M. P., Cory, R. M., D'Amore, D. V., and White, D.: Characterizing Dissolved Organic Matter Using PARAFAC Modeling of Fluorescence Spectroscopy: A Comparison of Two Models, Environ. Sci. Technol., 43, 6228–6234, https://doi.org/10.1021/es900143g, 2009a.
Fellman, J. B., Hood, E., D'amore, D. V., Edwards, R. T., and White, D.: Seasonal changes in the chemical quality and biodegradability of dissolved organic matter exported from soils to streams in coastal temperate rainforest watersheds, Biogeochemistry, 95, 277–293, 2009b.
Findlay, S. and Sinsabaugh, R. L.: Aquatic ecosystems: interactivity of dissolved organic matter, Academic Press, Amsterdam, The Netherlands and Boston, Massachusetts, USA, 512 pp., 2003.
Freeman, C., Lock, M. A., Marxsen, J., and Jones, S. E.: Inhibitory effects of high molecular weight dissolved organic matter upon metabolic processes in biofilms from contrasting rivers and streams, Freshwater Biol., 24, 159–166, https://doi.org/10.1111/j.1365-2427.1990.tb00315.x, 1990.
Freixa, A., Ejarque, E., Crognale, S., Amalfitano, S., Fazi, S., Butturini, A., and Romaní, A. M.: Sediment microbial communities rely on different dissolved organic matter sources along a Mediterranean river continuum, Limnol. Oceanogr., 61, 1389–1405, https://doi.org/10.1002/lno.10308, 2016.
Garcia, R. D., Reissig, M., Queimaliños, C. P., Garcia, P. E., and Dieguez, M. C.: Climate-driven terrestrial inputs in ultraoligotrophic mountain streams of Andean Patagonia revealed through chromophoric and fluorescent dissolved organic matter, Sci. Total Environ., 521–522, 280–292, https://doi.org/10.1016/j.scitotenv.2015.03.102, 2015.
Gelman, A., Goodrich, B., Gabry, J., and Vehtari, A.: R-squared for Bayesian Regression Models, Am. Stat., 73, 307–309, https://doi.org/10.1080/00031305.2018.1549100, 2019.
Ghosh, J. and Ghattas, A. E.: Bayesian Variable Selection Under Collinearity, Am. Stat., 69, 165–173, https://doi.org/10.1080/00031305.2015.1031827, 2015.
Gibson, C. A. and O'Reilly, C. M.: Organic matter stoichiometry influences nitrogen and phosphorus uptake in a headwater stream, Freshw. Sci., 31, 395–407, https://doi.org/10.1899/11-033.1, 2012.
Godwin, C. M. and Cotner, J. B.: What intrinsic and extrinsic factors explain the stoichiometric diversity of aquatic heterotrophic bacteria?, ISME J., 12, 598–609, https://doi.org/10.1038/ismej.2017.195, 2018.
Goodman, S. N.: Toward Evidence-Based Medical Statistics, 1: The P Value Fallacy, Ann. Intern. Med., 130, 995–1004, https://doi.org/10.7326/0003-4819-130-12-199906150-00008, 1999a.
Goodman, S. N.: Toward Evidence-Based Medical Statistics, 2: The Bayes Factor, Ann. Intern. Med., 130, 1005–1013, https://doi.org/10.7326/0003-4819-130-12-199906150-00019, 1999b.
Graeber, D., Boëchat, I. G., Encina-Montoya, F., Esse, C., Gelbrecht, J., Goyenola, G., Gücker, B., Heinz, M., Kronvang, B., Meerhoff, M., Nimptsch, J., Pusch, M. T., Silva, R. C. S., von Schiller, D., and Zwirnmann, E.: Global effects of agriculture on fluvial dissolved organic matter, Sci. Rep.-UK, 5, 16328, https://doi.org/10.1038/srep16328, 2015.
Graeber, D., Poulsen, J. R., Heinz, M., Rasmussen, J. J., Zak, D., Gücker, B., Kronvang, B., and Kamjunke, N.: Going with the flow: Planktonic processing of dissolved organic carbon in streams, Sci. Total Environ., 625, 519–530, https://doi.org/10.1016/j.scitotenv.2017.12.285, 2018.
Graeber, D., Gücker, B., Wild, R., Wells, N. S., Anlanger, C., Kamjunke, N., Norf, H., Schmidt, C., and Brauns, M.: Biofilm-specific uptake does not explain differences in whole-stream DOC tracer uptake between a forest and an agricultural stream, Biogeochemistry, 144, 85–101, https://doi.org/10.1007/s10533-019-00573-6, 2019.
Gücker, B., Silva, R. C. S., Graeber, D., Monteiro, J. A. F., and Boëchat, I. G.: Urbanization and agriculture increase exports and differentially alter elemental stoichiometry of dissolved organic matter (DOM) from tropical catchments, Sci. Total Environ., 550, 785–792, https://doi.org/10.1016/j.scitotenv.2016.01.158, 2016.
Guillemette, F. and del Giorgio, P. A.: Simultaneous consumption and production of fluorescent dissolved organic matter by lake bacterioplankton, Environ. Microbiol., 14, 1432–1443, https://doi.org/10.1111/j.1462-2920.2012.02728.x, 2012.
Hansen, A. M., Kraus, T. E. C., Pellerin, B. A., Fleck, J. A., Downing, B. D., and Bergamaschi, B. A.: Optical properties of dissolved organic matter (DOM): Effects of biological and photolytic degradation, Limnol. Oceanogr., 61, 1015–1032, https://doi.org/10.1002/lno.10270, 2016.
Heibati, M., Stedmon, C. A., Stenroth, K., Rauch, S., Toljander, J., Säve-Söderbergh, M., and Murphy, K. R.: Assessment of drinking water quality at the tap using fluorescence spectroscopy, Water Res., 125, 1–10, https://doi.org/10.1016/j.watres.2017.08.020, 2017.
Jaynes, E. T.: Probability Theory: The Logic of Science, Cambridge University Press, Cambridge, UK, 762 pp., 2003.
Jeffreys, S. H.: The Theory of Probability, 3rd edn., Oxford University Press, Oxford, New York, USA, 470 pp., 1998.
Kamjunke, N., Herzsprung, P., and Neu, T. R.: Quality of dissolved organic matter affects planktonic but not biofilm bacterial production in streams, Sci. Total Environ., 506–507, 353–360, https://doi.org/10.1016/j.scitotenv.2014.11.043, 2015.
Kamjunke, N., von Tümpling, W., Hertkorn, N., Harir, M., Schmitt-Kopplin, P., Norf, H., Weitere, M., and Herzsprung, P.: A new approach for evaluating transformations of dissolved organic matter (DOM) via high-resolution mass spectrometry and relating it to bacterial activity, Water Res., 123, 513–523, https://doi.org/10.1016/j.watres.2017.07.008, 2017.
Kass, R. E. and Raftery, A. E.: Bayes Factors, J. Am. Stat. Assoc., 90, 773–795, https://doi.org/10.1080/01621459.1995.10476572, 1995.
Koehler, B., von Wachenfeldt, E., Kothawala, D., and Tranvik, L. J.: Reactivity continuum of dissolved organic carbon decomposition in lake water, J. Geophys. Res.-Biogeo., 117, G01024, https://doi.org/10.1029/2011JG001793, 2012.
Kothawala, D. N., Murphy, K. R., Stedmon, C. A., Weyhenmeyer, G. A., and Tranvik, L. J.: Inner filter correction of dissolved organic matter fluorescence, Limnol. Oceanogr.-Meth., 11, 616–630, https://doi.org/10.4319/lom.2013.11.616, 2013.
Kruschke, J. K.: Bayesian estimation supersedes the t test, J. Exp. Psychol. Gen., 142, 573–603, https://doi.org/10.1037/a0029146, 2013.
Kuhnert, P. M., Martin, T. G., and Griffiths, S. P.: A guide to eliciting and using expert knowledge in Bayesian ecological models, Ecol. Lett., 13, 900–914, https://doi.org/10.1111/j.1461-0248.2010.01477.x, 2010.
Kuserk, F. T., Kaplan, L. A., and Bott, T. L.: In Situ Measures of Dissolved Organic Carbon Flux in a Rural Stream, Can. J. Fish. Aquat. Sci., 41, 964–973, https://doi.org/10.1139/f84-110, 1984.
Lambert, T., Teodoru, C. R., Nyoni, F. C., Bouillon, S., Darchambeau, F., Massicotte, P., and Borges, A. V.: Along-stream transport and transformation of dissolved organic matter in a large tropical river, Biogeosciences, 13, 2727–2741, https://doi.org/10.5194/bg-13-2727-2016, 2016a.
Lambert, T., Bouillon, S., Darchambeau, F., Massicotte, P., and Borges, A. V.: Shift in the chemical composition of dissolved organic matter in the Congo River network, Biogeosciences, 13, 5405–5420, https://doi.org/10.5194/bg-13-5405-2016, 2016b.
Lambert, T., Bouillon, S., Darchambeau, F., Morana, C., Roland, F. A. E., Descy, J.-P., and Borges, A. V.: Effects of human land use on the terrestrial and aquatic sources of fluvial organic matter in a temperate river basin (The Meuse River, Belgium), Biogeochemistry, 136, 191–211, https://doi.org/10.1007/s10533-017-0387-9, 2017.
Lemoine, N. P.: Moving beyond noninformative priors: why and how to choose weakly informative priors in Bayesian analyses, Oikos, 128, 912–928, https://doi.org/10.1111/oik.05985, 2019.
Lutz, B. D., Bernhardt, E. S., Roberts, B. J., Cory, R. M., and Mulholland, P. J.: Distinguishing dynamics of dissolved organic matter components in a forested stream using kinetic enrichments, Limnol. Oceanogr., 57, 76–89, https://doi.org/10.4319/lo.2012.57.1.0076, 2012.
Ly, A., Verhagen, J., and Wagenmakers, E.-J.: Harold Jeffreys's default Bayes factor hypothesis tests: Explanation, extension, and application in psychology, J. Math. Psychol., 72, 19–32, https://doi.org/10.1016/j.jmp.2015.06.004, 2016.
Martínez, A., Kominoski, J. S., and Larrañaga, A.: Leaf-litter leachate concentration promotes heterotrophy in freshwater biofilms: Understanding consequences of water scarcity, Sci. Total Environ., 599–600, 1677–1684, https://doi.org/10.1016/j.scitotenv.2017.05.043, 2017.
Massicotte, P.: eemR: Tools for Pre-Processing Emission-Excitation-Matrix (EEM) Fluorescence Data, CRAN, https://CRAN.R-project.org/package=eemR (last access: 19 May 2021), 2019.
McCarthy, M. A.: Bayesian Methods for Ecology, Cambridge University Press, Cambridge, UK, 310 pp., 2007.
McElreath, R.: Statistical Rethinking: A Bayesian Course with Examples in R and Stan, Apple Academic Press Inc., Boca Raton, Florida, USA, 469 pp., 2016.
Merseburger, G., Martí, E., Sabater, F., and Ortiz, J. D.: Point-source effects on N and P uptake in a forested and an agricultural Mediterranean streams, Sci. Total Environ., 409, 957–967, https://doi.org/10.1016/j.scitotenv.2010.11.014, 2011.
Mineau, M. M., Rigsby, C. M., Ely, D. T., Fernandez, I. J., Norton, S. A., Ohno, T., Valett, H. M., and Simon, K. S.: Chronic catchment nitrogen enrichment and stoichiometric constraints on the bioavailability of dissolved organic matter from leaf leachate, Freshwater Biol., 58, 248–260, https://doi.org/10.1111/fwb.12054, 2013.
Mineau, M. M., Wollheim, W. M., Buffam, I., Findlay, S. E. G., Hall, R. O., Hotchkiss, E. R., Koenig, L. E., McDowell, W. H., and Parr, T. B.: Dissolved organic carbon uptake in streams: A review and assessment of reach-scale measurements, J. Geophys. Res.-Biogeo., 121, 2019–2029, https://doi.org/10.1002/2015JG003204, 2016.
Morey, R. D., Rouder, J. N., Jamil, T., Urbanek, S., Forner, K., and Ly, A.: BayesFactor: Computation of Bayes Factors for Common Designs, CRAN, available at: https://CRAN.R-project.org/package=BayesFactor (last access: 19 May 2021), 2018.
Mulholland, P. J., Hall, R. O., Sobota, D. J., Dodds, W. K., Findlay, S. E. G., Grimm, N. B., Hamilton, S. K., McDowell, W. H., O'Brien, J. M., Tank, J. L., Ashkenas, L. R., Cooper, L. W., Dahm, C. N., Gregory, S. V., Johnson, S. L., Meyer, J. L., Peterson, B. J., Poole, G. C., Valett, H. M., Webster, J. R., Arango, C. P., Beaulieu, J. J., Bernot, M. J., Burgin, A. J., Crenshaw, C. L., Helton, A. M., Johnson, L. T., Niederlehner, B. R., Potter, J. D., Sheibley, R. W., and Thomasn, S. M.: Nitrate removal in stream ecosystems measured by 15N addition experiments: Denitrification, Limnol. Oceanogr., 54, 666–680, https://doi.org/10.4319/lo.2009.54.3.0666, 2009.
Murphy, K. R., Hambly, A., Singh, S., Henderson, R. K., Baker, A., Stuetz, R., and Khan, S. J.: Organic Matter Fluorescence in Municipal Water Recycling Schemes: Toward a Unified PARAFAC Model, Environ. Sci. Technol., 45, 2909–2916, https://doi.org/10.1021/es103015e, 2011.
Murphy, K. R., Stedmon, C. A., Wenig, P., and Bro, R.: OpenFluor – an online spectral library of auto-fluorescence by organic compounds in the environment, Anal. Methods-UK, 6, 658–661, https://doi.org/10.1039/C3AY41935E, 2014.
Mutschlecner, A. E., Guerard, J. J., Jones, J. B., and Harms, T. K.: Phosphorus Enhances Uptake of Dissolved Organic Matter in Boreal Streams, Ecosystems, 21, 675–688, https://doi.org/10.1007/s10021-017-0177-1, 2018.
Niño-García, J. P., Ruiz-González, C., and del Giorgio, P. A.: Interactions between hydrology and water chemistry shape bacterioplankton biogeography across boreal freshwater networks, ISME J., 10, 1755–1766, https://doi.org/10.1038/ismej.2015.226, 2016.
O'Brien, J. M., Dodds, W. K., Wilson, K. C., Murdock, J. N., and Eichmiller, J.: The saturation of N cycling in Central Plains streams: 15N experiments across a broad gradient of nitrate concentrations, Biogeochemistry, 84, 31–49, https://doi.org/10.1007/s10533-007-9073-7, 2007.
Ohno, T. and Bro, R.: Dissolved Organic Matter Characterization Using Multiway Spectral Decomposition of Fluorescence Landscapes, Soil Sci. Soc. Am. J., 70, 2028–2037, https://doi.org/10.2136/sssaj2006.0005, 2006.
Oviedo-Vargas, D., Royer, T. V., and Johnson, L. T.: Dissolved organic carbon manipulation reveals coupled cycling of carbon, nitrogen, and phosphorus in a nitrogen-rich stream, Limnol. Oceanogr., 58, 1196–1206, https://doi.org/10.4319/lo.2013.58.4.1196, 2013.
Payn, R. A., Webster, J. R., Mulholland, P. J., Valett, H. M., and Dodds, W. K.: Estimation of stream nutrient uptake from nutrient addition experiments, Limnol. Oceanogr.-Meth., 3, 174–182, https://doi.org/10.4319/lom.2005.3.174, 2005.
Pucher, M.: MatthiasPucher/INSBIRE: INSBIRE: Interactions in Nutrient Spirals using BayesIan nonlinear REgression, Zenodo, https://doi.org/10.5281/zenodo.4071851, 2020.
Pucher, M., Wünsch, U., Weigelhofer, G., Murphy, K., Hein, T., and Graeber, D.: staRdom: Versatile Software for Analyzing Spectroscopic Data of Dissolved Organic Matter in R, Water, 11, 2366, https://doi.org/10.3390/w11112366, 2019.
R Development Core Team: A language and environment for statistical computing, R Foundation for Statistical Computing, Vienna, Austria, 2019.
Ribot, M., von Schiller, D., Peipoch, M., Sabater, F., Grimm, N. B., and Martí, E.: Influence of nitrate and ammonium availability on uptake kinetics of stream biofilms, Freshw. Sci., 32, 1155–1167, https://doi.org/10.1899/12-209.1, 2013.
Romani, A. M., Guasch, H., Munoz, I., Ruana, J., Vilalta, E., Schwartz, T., Emtiazi, F., and Sabater, S.: Biofilm structure and function and possible implications for riverine DOC dynamics, Microb. Ecol., 47, 316–328, 2004.
Sabater, S., Guasch, H., Romaní, A., and Muñoz, I.: The effect of biological factors on the efficiency of river biofilms in improving water quality, Hydrobiologia, 469, 149–156, https://doi.org/10.1023/A:1015549404082, 2002.
Shutova, Y., Baker, A., Bridgeman, J., and Henderson, R. K.: Spectroscopic characterisation of dissolved organic matter changes in drinking water treatment: From PARAFAC analysis to online monitoring wavelengths, Water Res., 54, 159–169, https://doi.org/10.1016/j.watres.2014.01.053, 2014.
Small, G. E., Helton, A. M., and Kazanci, C.: Can consumer stoichiometric regulation control nutrient spiraling in streams?, J. N. Am. Benthol. Soc., 28, 747–765, https://doi.org/10.1899/08-099.1, 2009.
Stedmon, C. A. and Markager, S.: Resolving the variability in dissolved organic matter fluorescence in a temperate estuary and its catchment using PARAFAC analysis, Limnol. Oceanogr., 50, 686–697, https://doi.org/10.4319/lo.2005.50.2.0686, 2005.
Stevenson, F. J. and He, X.-T.: Nitrogen in Humic Substances as Related to Soil Fertility, in: Humic Substances in Soil and Crop Sciences: Selected Readings, John Wiley & Sons, Ltd., Madison, Wisconsin, 91–109, https://doi.org/10.2136/1990.humicsubstances.c5, 1990.
Stream Solute Workshop: Concepts and Methods for Assessing Solute Dynamics in Stream Ecosystems, J. N. Am. Benthol. Soc., 9, 95–119, https://doi.org/10.2307/1467445, 1990.
Stutter, M., Graeber, D., and Weigelhofer, G.: Available Dissolved Organic Carbon Alters Uptake and Recycling of Phosphorus and Nitrogen from River Sediments, Water, 12, 3321, https://doi.org/10.3390/w12123321, 2020.
Stutter, M. I., Graeber, D., Evans, C. D., Wade, A. J., and Withers, P. J. A.: Balancing macronutrient stoichiometry to alleviate eutrophication, Sci. Total Environ., 634, 439–447, https://doi.org/10.1016/j.scitotenv.2018.03.298, 2018.
Tank, J. L., Rosi-Marshall, E. J., Griffiths, N. A., Entrekin, S. A., and Stephen, M. L.: A review of allochthonous organic matter dynamics and metabolism in streams, J. N. Am. Benthol. Soc., 29, 118–146, https://doi.org/10.1899/08-170.1, 2010.
Taylor, P. G. and Townsend, A. R.: Stoichiometric control of organic carbon-nitrate relationships from soils to the sea, Nature, 464, 1178–1181, https://doi.org/10.1038/nature08985, 2010.
Teissier, S., Torre, M., Delmas, F., and Garabétian, F.: Detailing biogeochemical N budgets in riverine epilithic biofilms, J. N. Am. Benthol. Soc., 26, 178–190, https://doi.org/10.1899/0887-3593(2007)26[178:DBNBIR]2.0.CO;2, 2007.
Theng, B. K. G. (Ed.): Humic Substances, in: Developments in Clay Science, Elsevier, Amsterdam, the Netherlands, Oxford, United Kingdom and Cambridge, Massachusetts, 391–456, https://doi.org/10.1016/B978-0-444-53354-8.00012-8, 2012.
Trentman, M. T., Dodds, W. K., Fencl, J. S., Gerber, K., Guarneri, J., Hitchman, S. M., Peterson, Z., and Rüegg, J.: Quantifying ambient nitrogen uptake and functional relationships of uptake versus concentration in streams: a comparison of stable isotope, pulse, and plateau approaches, Biogeochemistry, 125, 65–79, https://doi.org/10.1007/s10533-015-0112-5, 2015.
Tsutsuki, K. and Kuwatsuka, S.: Chemical studies on soil humic acids, Soil Sci. Plant Nutr., 25, 183–195, https://doi.org/10.1080/00380768.1979.10433159, 1979.
von Schiller, D., Bernal, S., and Martí, E.: Technical Note: A comparison of two empirical approaches to estimate in-stream net nutrient uptake, Biogeosciences, 8, 875–882, https://doi.org/10.5194/bg-8-875-2011, 2011.
Weigelhofer, G.: The potential of agricultural headwater streams to retain soluble reactive phosphorus, Hydrobiologia, 793, 149–160, https://doi.org/10.1007/s10750-016-2789-4, 2017.
Weigelhofer, G., Fuchsberger, J., Teufl, B., Welti, N., and Hein, T.: Effects of Riparian Forest Buffers on In-Stream Nutrient Retention in Agricultural Catchments, J. Environ. Qual., 41, 373–379, https://doi.org/10.2134/jeq2010.0436, 2012.
Weigelhofer, G., Ramião, J. P., Pitzl, B., Bondar-Kunze, E., and O'Keeffe, J.: Decoupled water-sediment interactions restrict the phosphorus buffer mechanism in agricultural streams, Sci. Total Environ., 628–629, 44–52, https://doi.org/10.1016/j.scitotenv.2018.02.030, 2018a.
Weigelhofer, G., Ramião, J. P., Puritscher, A., and Hein, T.: How do chronic nutrient loading and the duration of nutrient pulses affect nutrient uptake in headwater streams?, Biogeochemistry, 141, 249–263, https://doi.org/10.1007/s10533-018-0518-y, 2018b.
Weigelhofer, G., Jirón, T. S., Yeh, T.-C., Steniczka, G., and Pucher, M.: Dissolved Organic Matter Quality and Biofilm Composition Affect Microbial Organic Matter Uptake in Stream Flumes, Water, 12, 3246, https://doi.org/10.3390/w12113246, 2020.
Welti, N., Striebel, M., Ulseth, A. J., Cross, W. F., DeVilbiss, S., Glibert, P. M., Guo, L., Hirst, A. G., Hood, J., and Kominoski, J. S.: Bridging food webs, ecosystem metabolism, and biogeochemistry using ecological stoichiometry theory, Front. Microbiol., 8, 1298, https://doi.org/10.3389/fmicb.2017.01298, 2017.
Wickham, H., Averick, M., Bryan, J., Chang, W., McGowan, L. D., François, R., Grolemund, G., Hayes, A., Henry, L., Hester, J., Kuhn, M., Pedersen, T. L., Miller, E., Bache, S. M., Müller, K., Ooms, J., Robinson, D., Seidel, D. P., Spinu, V., Takahashi, K., Vaughan, D., Wilke, C., Woo, K., and Yutani, H.: Welcome to the tidyverse, J. Open Source Softw., 4, 1686, https://doi.org/10.21105/joss.01686, 2019.
Wickland, K. P., Neff, J. C., and Aiken, G. R.: Dissolved Organic Carbon in Alaskan Boreal Forest: Sources, Chemical Characteristics, and Biodegradability, Ecosystems, 10, 1323–1340, https://doi.org/10.1007/s10021-007-9101-4, 2007.
Wickland, K. P., Aiken, G. R., Butler, K., Dornblaser, M. M., Spencer, R. G. M., and Striegl, R. G.: Biodegradability of dissolved organic carbon in the Yukon River and its tributaries: Seasonality and importance of inorganic nitrogen, Global Biogeochem. Cy., 26, GB0E03, https://doi.org/10.1029/2012GB004342, 2012.
Wiegner, T. N., Kaplan, L. A., Newbold, J. D., and Ostrom, P. H.: Contribution of dissolved organic C to stream metabolism: a mesocosm study using 13C-enriched tree-tissue leachate, J. N. Am. Benthol. Soc., 24, 48–67, https://doi.org/10.1899/0887-3593(2005)024<0048:CODOCT>2.0.CO;2, 2005.
Williams, C. J., Yamashita, Y., Wilson, H. F., Jaffé, R., and Xenopoulos, M. A.: Unraveling the role of land use and microbial activity in shaping dissolved organic matter characteristics in stream ecosystems, Limnol. Oceanogr., 55, 1159–1171, https://doi.org/10.4319/lo.2010.55.3.1159, 2010.
Williams, C. J., Frost, P. C., and Xenopoulos, M. A.: Beyond best management practices: pelagic biogeochemical dynamics in urban stormwater ponds, Ecol. Appl., 23, 1384–1395, https://doi.org/10.1890/12-0825.1, 2013.
Wünsch, U. J., Murphy, K. R., and Stedmon, C. A.: Fluorescence Quantum Yields of Natural Organic Matter and Organic Compounds: Implications for the Fluorescence-based Interpretation of Organic Matter Composition, Front. Mar. Sci., 2, 98, https://doi.org/10.3389/fmars.2015.00098, 2015.
Wymore, A. S., Coble, A. A., Rodríguez-Cardona, B., and McDowell, W. H.: Nitrate uptake across biomes and the influence of elemental stoichiometry: A new look at LINX II, Global Biogeochem. Cy., 30, 1183–1191, https://doi.org/10.1002/2016GB005468, 2016.
Xenopoulos, M. A., Barnes, R. T., Boodoo, K. S., Butman, D., Catalán, N., D'Amario, S. C., Fasching, C., Kothawala, D. N., Pisani, O., and Solomon, C. T.: How humans alter dissolved organic matter composition in freshwater: relevance for the Earth's biogeochemistry, Biogeochemistry, 1–26, https://doi.org/10.1007/s10533-021-00753-3, 2021.
Yamashita, Y., Kloeppel, B. D., Knoepp, J., Zausen, G. L., and Jaffé, R.: Effects of Watershed History on Dissolved Organic Matter Characteristics in Headwater Streams, Ecosystems, 14, 1110–1122, https://doi.org/10.1007/s10021-011-9469-z, 2011.
Yamashita, Y., Boyer, J. N., and Jaffé, R.: Evaluating the distribution of terrestrial dissolved organic matter in a complex coastal ecosystem using fluorescence spectroscopy, Cont. Shelf Res., 66, 136–144, https://doi.org/10.1016/j.csr.2013.06.010, 2013.