the Creative Commons Attribution 4.0 License.
the Creative Commons Attribution 4.0 License.
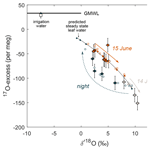
Examination of the parameters controlling the triple oxygen isotope composition of grass leaf water and phytoliths at a Mediterranean site: a model–data approach
Ilja M. Reiter
Jean-Philippe Orts
Christine Vallet-Coulomb
Clément Piel
Jean-Charles Mazur
Julie C. Aleman
Corinne Sonzogni
Helene Miche
Jérôme Ogée
Triple oxygen isotopes (17O-excess) of water are useful to trace evaporation at the soil–plant–atmosphere interface. The 17O-excess of plant silica, i.e., phytoliths, inherited from leaf water, was previously calibrated in growth chambers as a proxy of atmospheric relative humidity (RH). Here, using a model–data approach, we examine the parameters that control the triple oxygen isotope composition of bulk grass leaf water and phytoliths in natura, at the O3HP experimental platform located in the French Mediterranean area. A grass plot was equipped to measure for 1 year, all environmental and plant physiological parameters relevant for modeling the isotope composition of the grass leaf water. In particular, the triple oxygen and hydrogen isotope composition of atmospheric water vapor above the grass was measured continuously using a cavity ring-down spectrometer, and the grass leaf temperature was monitored at plot scale using an infrared (IR) radiometer. Grass leaves were collected in different seasons of the year and over a 24 h period in June. Grass leaf water was extracted by cryogenic vacuum distillation and analyzed by isotope ratio mass spectrometry (IRMS). Phytoliths were analyzed by IR–laser fluorination–IRMS after chemical extraction. We showed that the traditional Craig–Gordon steady-state model modified for grass leaves reliably predicts the triple oxygen isotope composition of leaf water during daytime but is sensitive to uncertainties on the leaf-to-air temperature difference. Deviations from isotope steady state at night are well represented in the triple oxygen isotope system and predictable by a non-steady-state model. The 17O-excess of phytoliths confirms the applicability of the 17O-excessphyto vs. RH equation established in previous growth chamber experiments. Further, it recorded average daytime RH over the growth period rather than daily RH, related to low transpiration and silicification during the night. This model–data approach highlights the utility of the triple oxygen isotope system to improve the understanding of water exchange at the soil–plant–atmosphere interface. The in natura experiment underlines the applicability of 17O-excess of phytoliths as a RH proxy.
- Article
(3755 KB) -
Supplement
(398 KB) - BibTeX
- EndNote
Continental atmospheric relative humidity (RH) is a key factor of soil evaporation, transpiration, dryness stress, and ecosystem productivity (Grossiord et al., 2020; Liu et al., 2021; López et al., 2021). However, RH is estimated with low precision in the Earth system models (IPCC, 2013; Tierney et al., 2020). Long-term data beyond the instrumental period are needed to improve the representation of RH in these models. Leaf organic and mineral compounds formed during plant growth, such as cellulose, n-alkanes of leaf waxes, or phytoliths, are used as past climate indicators when preserved in soils, sediments, or peat (Helliker and Ehleringer, 2002a, b; Kahmen et al., 2011, 2013; Zech et al., 2014; Tuthorn et al., 2015; Alexandre et al., 2018, 2019; Outrequin et al., 2021; Garcin et al., 2012, 2022). To accurately interpret the isotope signal of these compounds in terms of paleoclimate, their relationship to that of leaf water and the factors driving their isotope variability need to be determined. Regarding the phytolith isotope signature, previous calibrations have often been performed in controlled environmental conditions, not representative of the diurnal, daily, and seasonal climate variations encountered in the natural environment (Alexandre et al., 2018, 2019; Outrequin et al., 2021). Therefore, the question of the time span (seasonal vs. annual, diurnal vs. daily) integrated in the phytolith isotope composition remains open.
Leaf waters generally show higher δ2H, δ18O, and lower d-excess [= δ2H–8δ18O] than meteoric waters due to significant evaporative fractionation during transpiration. The magnitude of this isotope fractionation can be predicted by the isotope–evaporation model developed by Craig and Gordon (1965) and later adapted to leaf transpiration (Dongmann et al., 1974; Farquhar and Cernusak, 2005). This model (hereafter referred to as the C–G model) considers three main processes occurring in the boundary layer of the leaf during transpiration: (i) liquid-water–water-vapor equilibrium at the boundary layer interface, (ii) diffusion of water vapor from the evaporative sites in the leaf to the surrounding air, and (iii) back-diffusion of atmospheric water vapor to the leaf (Craig and Gordon, 1965; Farquhar et al., 2007; Cernusak et al., 2016). The C–G model is based on the steady-state assumption; i.e., all water that is lost by evaporation is continuously replenished by xylem water. This assumption neglects small diurnal changes in leaf water content that are expected to result in only 3 % error in the predicted leaf water δ18O enrichment (Farris and Strain, 1978; Farquhar and Cernusak, 2005). The C–G model also assumes isotope steady state, so that the isotope composition of transpired water matches that of source (xylem) water. To take into account the advection of less evaporated stem water to the evaporation site, as well as the diffusion of the evaporating water back to the leaf lamina, a transpiration-dependent correction, called the Péclet effect, can be added to the C–G model (e.g., Buhay et al., 1996; Helliker and Ehleringer, 2000; Roden et al., 2000; Farquhar and Gan, 2003; Farquhar and Cernusak, 2005; Ripullone et al., 2008; Treydte et al., 2014). For grasses, a two-pool model including a pristine water pool that coincides to the xylem tissues and an evaporated water pool that corresponds to leaf lamina water has been found to best represent bulk leaf water (Liu et al., 2017; Hirl et al., 2019; Barbour et al., 2021). This mixing effect is independent from transpiration, so that a two-endmember mixing equation is combined with the C–G model (Leaney et al., 1985).
Although the modeling approaches described above reproduce the observed trends in the isotope composition of bulk leaf water, discrepancies between modeled and observed δ18O values as high as 6 ‰ have been reported (e.g., Flanagan et al., 1991; Gan et al., 2002; Loucos et al., 2014; Song et al., 2015; Cernusak et al., 2016; Bögelein et al., 2017). These discrepancies can arise from uncertainties in key parameters of the C–G model that are difficult to measure, such as the isotope composition of atmospheric water vapor and the difference between leaf temperature and air temperature (Cernusak et al., 2002; Flanagan and Farquhar, 2014; Li et al., 2017; Alexandre et al., 2018). The isotope composition of atmospheric water vapor varies greatly in space and time, in principle depending on the climate conditions in the air mass source region and processes affecting the air mass during transport, including rainout, moisture recycling, and mixing. In the absence of direct measurements, the isotope composition of atmospheric water vapor is often estimated, assuming isotope equilibrium with local precipitation. This assumption can be valid on monthly timescales, but large deviations can occur on daily or hourly timescales (Jacob and Sonntag, 1991; Lee et al., 2006; Aemisegger et al., 2015; Graf et al., 2019; Penchenat et al., 2020). Variations in leaf temperature slightly influence the equilibrium isotope fractionation at the liquid–vapor interface. More importantly the deviation of the leaf temperature from the air temperature (ΔTleaf-air) determines the water vapor pressure gradient between the leaf and the atmosphere, one of the major controls of the isotope composition of bulk leaf water. However, large spatial and temporal variability of leaf temperatures complicate measurement or accurate estimation of ΔTleaf-air. Ultimately, deviations from isotope steady state resulting from low stomatal conductance (gs) and transpiration rate and thus long leaf water residence time in the mesophyll cells, notably occurring at night or during drought, can also account for model–data discrepancies (Cuntz et al., 2007; Ogée et al., 2007; Cernusak et al., 2016; Wang et al., 2018).
Recent analytical advances enable the analysis of δ17O in addition to δ18O, allowing one to derive the secondary parameter 17O-excess [= O–0.528 O with δ′ = ln(δ+1)], with 0.528 being the slope of the global meteoric water line (GMWL) (Luz and Barkan, 2010). The small variations in 17O-excess are usually reported in “per meg”, i.e., 0.001 ‰. As d-excess, 17O-excess of liquid water decreases with increasing evaporation. However, in contrast to δ18O, δ2H or d-excess, the 17O-excess is weakly affected by temperature changes and Rayleigh distillation. This is due to its low sensitivity to equilibrium isotope fractionation between liquid water and water vapor (Barkan and Luz, 2005). Consequently, 17O-excess varies little in meteoric water, which feeds the soil water taken up by the plants and is also assumed to vary little in atmospheric water vapor (Luz and Barkan, 2010; Aron et al., 2021; Surma et al., 2021). The 17O-excess of bulk leaf water is thus essentially controlled by the molecular diffusion of water vapor between the leaf and the atmosphere during transpiration (Barkan and Luz, 2007). The extent of this process depends mainly on the water pressure gradient between the leaf and the atmosphere. The few existing studies on 17O-excess of bulk leaf water showed that it is inversely related to RH. Discrepancies between modeled and observed 17O-excess values higher than 100 per meg have been reported (Li et al., 2017; Alexandre et al., 2018; Outrequin et al., 2021). These discrepancies have been attributed to deviations from isotope steady state in the early morning hours (Li et al., 2017) and uncertainty in the estimates of leaf temperature and the isotope composition of atmospheric water vapor (Li et al., 2017; Alexandre et al., 2018). Large discrepancies observed by Li et al. (2017) may also result from neglecting potential mixing of evaporated and non-evaporative grass leaf water pools.
Phytoliths are micrometric silica particles that form in temperature-dependent isotope equilibrium with water in living plant tissues within a few hours to days (Perry et al., 1987). In grasses, the majority of phytoliths form in sheaths and leaves, due to concentration of solutes by transpiration (e.g., Webb and Longstaffe, 2000, 2002). Phytolith morphological assemblages recovered from soils and sediments are used to reconstruct vegetation changes and qualitatively inform on climatic conditions at the time of soil formation (Bremond et al., 2005; Aleman et al., 2012; Nogué et al., 2017). Previous studies investigated the potential of δ18O of phytoliths as a proxy for past temperature (Webb and Longstaffe, 2000, 2002, 2006; Alexandre et al., 2012). However, accurate temperature reconstruction using this proxy requires an independent estimate of the δ18O of soil water and an estimate of the effect of RH and transpiration on δ18O of leaf water. These studies have also shown the dependency of δ18O of phytoliths on RH, but its utility to reconstruct past RH has not been further explored given the large number of factors influencing δ18O of precipitation, soil, and leaf water. Recent studies in growth chambers and at natural sites demonstrated that unlike the δ18O, the 17O-excess of phytoliths (17O-excessphyto), inherited from the 17O-excess of leaf water, is primarily controlled by RH around the plant, according to a gradient of 4.3 ± 0.3 per meg %−1 (Outrequin et al., 2021). This relationship is independent of grass leaf length and vegetation type (Alexandre et al., 2018, 2019; Outrequin et al., 2021). Further, the 17O-excessphyto is not affected by changes in air temperature or atmospheric CO2 levels (Outrequin et al., 2021).
In this study, using a model–data approach, we examined the parameters controlling the triple oxygen isotope composition of bulk grass leaf water and phytoliths at a natural site. For that purpose, a grass plot was equipped to measure for the course of 1 year, all environmental and plant physiological parameters relevant for modeling the isotope composition of the grass leaf water. In particular, the triple oxygen and hydrogen isotope composition of atmospheric water vapor above the grass was measured continuously over the year using a cavity ring-down spectrometer (CRDS), and the grass leaf temperature was monitored at plot scale using an infrared (IR) radiometer. Grass leaf blades were collected at midday on eight days in different seasons of the year and over a 24 h period in June for triple oxygen and hydrogen isotope analysis of bulk leaf waters. In addition, grass leaf blades were harvested in spring, summer, and autumn for phytolith extraction and triple oxygen isotope analysis to examine which RH average is recorded in 17O-excessphyto of phytolith assemblages that are formed over growth periods of several months.
2.1 Experimental setup
The AnaEE in natura experimental platform O3HP is located about 100 km north of Marseille (France) at an altitude of 680 m above sea level (43.935∘ N, 5.711∘ E). On 14 February 2021, seeds of the C3 grass species Festuca arundinacea, also referred to as tall fescue, were sown (8 g m−2) on a 5.5 m2 plot in the understory of an oak-dominated forest. The same grass species was used for the calibration of the relationship between 17O-excessphyto and RH in growth chamber experiments (Alexandre et al., 2018, 2019; Outrequin et al., 2021). Potting soil was added to the shallow calcaric Leptosol (IUSS Working Group WRB, 2015; Belviso et al., 2016) and supplied with ∼ 50 g m−2 organic fertilizer (Engrais Gazon, Neudorff, Emmerthal, Germany) and 2.7 g m−2 SiO2 (General Hydroponics Mineral Magic, Terra Aquatica, Fleurance, France) to ensure a sufficient amount of nutriments and bio-available silica.
The experimental plot was automatically irrigated with tap water (30 mm d−1) from 4 March 2021 until the end of the experiment on 23 November 2021 to avoid water stress in the grasses. The potential evaporation from the grass plot (2–4 mm d−1) estimated using the Penman–Monteith equation (Monteith, 1965) was an order of magnitude lower than the irrigation rate. Therefore, we assume that soil water evaporation was negligible and had no impact on the isotope composition of leaf water. An aliquot of the irrigation water was collected in an evaporation-free water collector (Rain Sampler 1, Palmex d.o.o., Zagreb, Croatia; Gröning et al., 2012), which was sampled weekly. Precipitation was collected on an event-based interval using a second water collector of the same type. Both collectors were emptied and dried after sampling. For isotope analysis of atmospheric water vapor, the air at 0.4 m above the grass plot was pumped continuously (N 86 KN.18, KNF DAC GmbH, Hamburg, Germany) to a Picarro L2140-i CRDS (Picarro Inc., California, USA), installed in an air-conditioned cabin on the experimental site. The air was passed through a 11.5 m long and in. wide PFA tube (PFA-T4-062-100, Swagelok, Ohio, USA), at a flow rate of 5 L min−1. The tubing was insulated and heated to prevent condensation of the water vapor. A funnel covered by a net was placed at the inlet for protection from rain and suction of insects and large aerosol particles.
The following climate parameters were measured on the experimental site: global solar radiation at 6 m above ground (LI-200, LI-COR Biosciences Inc., Nebraska, USA), precipitation amount (15189 H, LAMBRECHT meteo GmbH, Göttingen, Germany), RH and atmospheric temperature (Tair) at 60 cm height next to the grass plot (HMP155, Vaisala Oyj, Vantaa, Finland), atmospheric temperature at 5 cm above the ground (Tground) (DTS12, Vaisala Oyj, Vantaa, Finland), soil water content and soil temperature at ∼ 5 cm depth (CS655, Campbell Scientific Inc, Logan, Utah, USA), plot-scale grass leaf temperature (Tplot) (IR radiometer SI-411-SS, Apogee Instruments Inc., Utah, USA), and sky temperature (Tsky). Tplot is the temperature integrated over the field of view of the IR radiometer that covered ∼ 90 % of the grass plot surface. Each parameter was extracted in hourly resolution from the COOPERATE database (Reiter et al., 2015).
On sampling days (Table 1), stomatal conductance (gs) and transpiration were monitored continuously over the day on a single grass leaf of 4–5 mm width using a Li-6400 XT gas exchange system (LI-COR Biosciences Inc., Nebraska, USA). To assess the spatial variability of gs, this parameter was additionally measured hourly on the adaxial side of 10 leaves of at least 3 mm width, randomly selected on the plot, using an AP4 porometer (Table S1; Delta-T Devices LTD, Cambridge, UK). In addition, leaf temperature (Tleaf) was measured in situ on the adaxial side of 10 grass leaves, randomly selected, in 1 h intervals using an Optris CT IR thermometer (Table S2; Optris GmbH, Berlin, Germany). Tplot and Tleaf measurements were corrected for emissivity of the grass canopy, considering the tree canopy gap fraction:
where ε is the emissivity of the grass canopy (ε = 0.95; Apogee Instruments Inc, 2022) and α is the tree canopy gap fraction, which is estimated to be 0.3 throughout the experimental period. Traw is the temperature recorded by the sensor, Tsky is the sky temperature, and Tcanopy is the canopy temperature, which is assumed to be equal to Tair.
2.2 Sampling
Leaf blades of F. arundinacea were collected at midday on eight days in May, July, August, October, and November 2021 (Table 1), as well as every ∼ 1.5 h over a 24 h period from 14–15 June 2021. About 10 fully developed, not senescent leaf blades from different tillers evenly distributed over the grass plot were immediately transferred to 12 mL Exetainer vials (Labco, High Wycombe, UK), and they were stored in a fridge until water extraction and isotope analysis.
Three grass regrowths were monitored in spring (17 February–20 May 2021), summer (15 June–27 August 2021), and autumn (27 August–23 November 2021) (Table 2). Each regrowth started after the grasses had been cut above the sheath at 2–4 cm height. Grass heights were measured at monthly intervals. At the end of each regrowth, the grass leave blades from the entire plot were harvested and dried at 50 ∘C. Between 120 and 150 g of dry matter was obtained for phytolith extraction and analysis.
Table 1F. arundinacea leaf water isotope composition (δ18O, 17O-excess, and d-excess), stomatal conductance (gs), and transpiration (E) measured on a single leaf blade using the LI-COR gas exchange system, atmospheric temperature (Tair), and relative humidity (RH) at 60 cm height next to the grass plot, plot-scale grass leaf temperature (Tplot), and the ratio of atmospheric vapor pressure at 60 cm height and saturation vapor pressure at Tplot (h), averaged over 30 min before sampling on 8 d at midday between May and November 2021 and 14 samplings during a 24 h period from 14–15 June 2021. The sample ID indicates “sampling location_plant_species_sample type_sampling date_sampling time”. Plant species “FA” denotes the C3 grass Festuca arundinacea; sampling date is in the format yyyymmmdd and sampling time in UTC. ΔTleaf-air = Tplot–Tair.
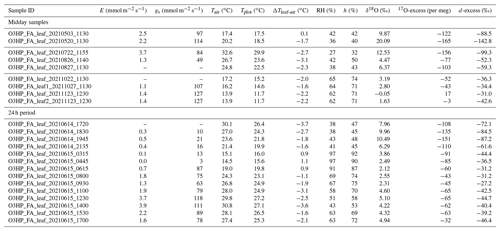
Table 2Grass and phytolith descriptors, phytolith isotope composition, atmospheric temperature (Tair), plot-scale grass leaf temperature (Tplot), relative humidity (RH), and the ratio between actual atmospheric vapor pressure and saturation vapor pressure at Tplot (h) for the three regrowth periods. Grass height indicates grass height at the harvest day. LC – proportion of long-cell phytoliths on the number of short- and long-cell phytoliths in the sample. The silicification rate is inferred from the measured SiO2 concentration in grass leaf blades harvested at the end of the regrowth and the length of the regrowth period, assuming a linear production rate (avg rate). Observed RH and h values are compared to estimated values using 17O-excessphyto and Eqs. (6) and (7), respectively (RHphyto and hphyto, respectively). SD = 1 standard deviation of four replicate measurements on two consecutive days. ΔTleaf-air = Tplot–Tair.
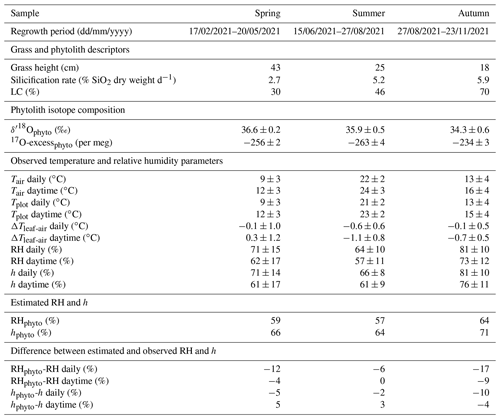
2.3 Extractions and isotope analyses
2.3.1 Irrigation water, precipitation, and atmospheric water vapor
A Picarro L2140-i CRDS (California, USA), operated in 17O dual liquid–vapor mode was installed on-site for the experiment. The isotope composition and mixing ratio of water vapor in the air at 0.4 m above the grass plot was measured for 70 min every 140 min during the spring monitoring and every 280 min during the monitoring in summer and autumn. In between these measurements, the instrument was used for another experiment. The atmospheric water vapor data from the first 10 min of each measurement cycle were removed to account for memory effects and provide sufficient time to establish a stable baseline. The remaining 60 min was averaged. During the 2 h monitoring, air sampling was performed continuously without interruption. Liquid water standard measurement runs were performed on a weekly basis. The mean of four measurement runs of liquid water standards was used to normalize the atmospheric water vapor isotope data to VSMOW-SLAP scale. The calibration protocol is described in detail by Voigt et al. (2022). In brief, three liquid water standards that covered the expected isotope range of atmospheric water vapor at the study site were analyzed at a water mixing ratio of 11 000 ppmv using a Picarro autosampler system (A0325, Picarro Inc., California, USA) coupled to a high-precision vaporizer (A0211, Picarro Inc., California, USA). The liquid standards were injected in a dry air stream, produced by a lubricated mobile air compressor (Montecarlo FC2, ABAC air compressors, Italy), further dried using two drierite columns combined with a dry ice trap (Voigt et al., 2022). Raw isotope compositions of the liquid standards of four consecutive measurement runs were averaged and then corrected to the water mixing ratio of the measured atmospheric water vapor, using the mean of three mixing ratio dependency functions that were determined on site for water mixing ratios between 3000 and 30 000 ppmv in May 2021, October 2021, and January 2022 (Fig. A1). The precision of calibrated and integrated atmospheric water vapor data was determined using a Monte Carlo simulation (Voigt et al., 2022). Precision was better than ± 0.1 ‰, ± 0.2 ‰, ± 1.8 ‰, and ± 14 per meg and ± 0.9 ‰ for δ17O, δ18O, δ2H, 17O-excess, and d-excess, respectively.
A second Picarro L2140-i CRDS operated in 17O–high-precision mode was used at CEREGE to analyze the isotope composition of irrigation water and precipitation. Isotope analyses, correction of memory effects, and VSMOW-SLAP scaling were performed following Vallet-Coulomb et al. (2021). The external reproducibility of a quality control standard (1 standard deviation (SD), n = 12) measured along with the samples in each sequence was ± 0.02 ‰, ± 0.03 ‰, ± 0.3 ‰, and ± 6 per meg and ± 0.1 ‰ for δ17O, δ18O, δ2H, 17O-excess, and d-excess, respectively.
2.3.2 Grass leaf water
Grass leaf water was extracted by cryogenic vacuum distillation (static pressure < 10 Pa) with sample vials placed in the vacuum line and immersed in a heated water bath for 3 h with a final target temperature set to 80 ∘C (attained within 45 min of extraction). A detailed description of the system design is given by Barbeta et al. (2022). Water extraction yield was derived by comparing the volume of water collected (in mL) and the difference of sample weights before and after water extraction (with the exetainer and converted in equivalent mL of water). For our sample set, the average water extraction yield was 103 ± 5 % (102 ± 3 % without one outlier) and average extracted volume was 0.5 ± 0.2 mL, with only one extraction volume below 0.3 mL. Thus, methodological uncertainties linked to cryogenic vacuum distillation should be negligible (Diao et al., 2022). Isotope analysis of grass leaf waters was performed at the University of Cologne. For triple oxygen isotope analysis, pure O2 liberated from grass leaf waters by fluorination was introduced in a Thermo Fisher Scientific MAT 253 dual-inlet mass spectrometer (Massachusetts, USA), following the procedure described by Surma et al. (2015). The reproducibility (1 SD, n = 2) of δ17O, δ18O and 17O-excess measurements was better than ± 0.15 ‰, ± 0.30 ‰, and ± 11 per meg, respectively. Hydrogen isotope ratios were determined by high-temperature carbon reduction in a pyrolysis elemental analyzer (HEKAtech GmbH, Wegberg, Germany), coupled to the mass spectrometer. The reproducibility (1 SD, n = 3) of δ2H measurements was always better than 1.1 ‰. An intercomparison of water analysis at CEREGE and the University of Cologne was performed. The results are presented in Table S3. Differences between the laboratories were lower than 0.2 ‰, 0.3 ‰, 1.1 ‰, 14 per meg, and 1.6 ‰ for δ17O, δ18O, δ2H, 17O-excess, and d-excess, respectively. Similar differences were found in an intercomparison between the two Picarro CRDS instruments (Alexandre et al., 2018).
2.3.3 Phytoliths
The silica contents of harvested grass leaf blades were determined by inductively coupled plasma–atomic emission spectroscopy (Ultima C, Horiba Jobin Yvon, Longjumeau, France). Phytoliths were extracted following the “wet digestion” protocol detailed in Table 2 of Corbineau et al. (2013). The protocol involves treatment of the sample with different chemical agents (HCl, H2SO4, H2O2, HNO3) to remove organic and carbonate compounds. The pure phytolith concentrates were mounted on microscope slides in Canada balsam, and the morphological types were counted using light microscopy at a ×600 magnification. The epidermal silicified intercoastal long cells were quantified relative to the silicified short cells to obtain information on the silicification process (Alexandre et al., 2019).
The phytolith samples (1.6 mg) were dehydrated at 1100 ∘C under a flow of N2 (Chapligin et al., 2010) to prevent the formation of siloxane from silanol groups during dehydroxylation. Molecular O2 was extracted using the IR laser-heating fluorination technique (Alexandre et al., 2006; Crespin et al., 2008; Outrequin et al., 2021). At the end of the procedure, the gas was passed through a −114 ∘C slush to refreeze any molecule interfering with the mass 33 (e.g., NF potentially remaining in the line). The gas was directly sent to a ThermoQuest Finnigan Delta V Plus dual-inlet mass spectrometer (Massachusetts, USA) for triple oxygen isotope analysis. Each gas sample was run twice with each run consisting of eight dual-inlet cycles. A third run was performed when the standard deviation of the first two averages was higher than 12 per meg for 17O-excess. The reproducibility for δ18O and 17O-excess measurements of the quartz laboratory standard was 0.16 ‰ and 8 per meg, respectively (1 SD, n = 5). For the phytolith samples, the precision for δ18O and 17O-excess was always better than 0.5 ‰ and 12 per meg (1 SD), respectively. The sample measurements were corrected using a quartz laboratory standard analyzed at the beginning of the day until a 17O-excess plateau was reached and again at the end of the day. The isotope composition of the reference gas was determined against NBS28. For robust comparisons between silica and water isotope compositions, the phytolith data are normalized to VSMOW-SLAP scale (Outrequin et al., 2021).
2.4 Modeling
According to the C–G isotope steady-state model (Craig and Gordon, 1965; Dongmann et al., 1974; Farquhar et al., 2007; Cernusak et al., 2016), the isotope ratio of the evaporated water pool in the leaf (Re) is
where RV and RS denote the isotope ratios (2H 1H, 17O 16O and 18O 16O) of atmospheric water vapor and source water, respectively. h is the ratio of the actual vapor pressure in the atmosphere to the saturation vapor pressure inside the leaf (i.e., at leaf temperature, Tleaf). When the leaf-to-air temperature gradient is small, h is equal to RH. The isotope fractionation during water vapor diffusion in air through the leaf stomata and boundary layer (αdiff) was estimated as
where gs and gb (mol m−2 s−1) denote the stomatal and leaf boundary layer conductances, and αkin denotes the kinetic isotope fractionation during molecular diffusion of water vapor in air. We took 18αkin = 1.028 and 2αkin = 1.025 from Merlivat et al. (1978) for 18O 16O and 2H 1H, respectively. Stomatal and boundary layer conductances measured continuously on a single leaf using the LI-COR gas exchange system (see Sect. 2.1) are used for modeling. For equilibrium isotope fractionation between water and water vapor, temperature-dependent fractionation factors (αeq) for 18O 16O and 2H 1H reported by Majoube et al. (1971) are used herein. The fractionation factors for 17O 16O are derived from those of 18O 16O according to 17α = 18αθ using θeq = 0.529 for liquid–vapor equilibrium (Barkan and Luz, 2005) and θkin = 0.5185 for the kinetic fractionation during molecular diffusion (Barkan and Luz, 2007).
The bulk grass leaf water at isotope steady state (Rleaf,ss) represents a mixture of an evaporated water pool in the lamina mesophyll whose isotope composition is predicted by the C–G model (Re, Eq. 2), and an unevaporated pool in the leaf veins and associated ground tissues, whose isotope composition matches Rs (Leaney et al., 1985; Yakir et al., 1994; Hirl et al., 2019):
where f represents the water volume fraction of the unevaporated pool and was set to 0.2 in our study. Similar values were used in previous studies on grass leaf water (Wang et al., 2018; Alexandre et al., 2019; Hirl et al., 2019). Instead of a mixing equation, the Péclet effect can be considered to estimate the bulk leaf water isotope composition (Farquhar and Lloyd, 1993; Farquhar et al., 2007; Holloway-Phillips et al., 2016):
with p [= EL CD] the Péclet number, where L is the effective path length, E is the grass leaf transpiration rate, C is the molar density of liquid water (55 500 mol m−3), and D is the diffusivity of water (2.3 × 10−9 m2 s−1 at 25 ∘C). One single value of L was applied for the data set and adjusted to fit the observed grass leaf water isotope composition.
When the steady state cannot be reached, non-steady-state enrichment of bulk leaf water (Rleaf,nss) can be modeled using the following equation (Dongmann et al., 1974; Farquhar and Cernusak, 2005; Hirl et al., 2019):
with
where g = gs gb (gs+ gb), wi is the mole fraction of water vapor in air in the intercellular spaces, W is the leaf water content, and Rleaf,ss denotes the isotope composition of bulk leaf water at steady state, as predicted by Eq. (4). Similar to Farquhar and Cernusak (2005) or Hirl et al. (2019), we neglected diurnal changes in W, which should result in only ∼ 3 % error in predicted leaf water isotope enrichment (Farquhar and Cernusak, 2005). We adjusted W to fit the observed grass leaf water isotope composition. The best fit was found for W of 6 mol m−2.
Both steady-state and non-steady-state model calculations were performed for isotope ratios (2H 1H, 17O 16O and 18O 16O) independently, and the secondary isotope parameters (d-excess and 17O-excess) were derived from predicted primary isotope values (δ17O, δ18O, δ2H) using the equations given in Sect. 1.
3.1 Changes in the isotope composition of atmospheric water vapor, precipitation, and irrigation water
Over the experimental period, the isotope composition of irrigation water that mainly fed the soil water was stable, averaging −7.4 ± 0.2 ‰ for δ18O, −48.5 ± 0.7 ‰ for δ2H, 10.7 ± 0.6 ‰ for d-excess, and 31 ± 6 per meg for 17O-excess (Fig. A2). These values are close to the amount-weighted annual averages of precipitation in 2021: −8.1 ± 2.9 ‰ for δ18O, −52 ± 24 ‰ for δ2H, 12.0 ± 3.5 ‰ for d-excess, and 29 ± 11 per meg for 17O-excess (Table S4). The precipitation (730 mm a−1) was mainly distributed between two periods in spring (April to May) and autumn (October to December) (Fig. 1, Table S4).
The annual average isotope composition of atmospheric water vapor was −17.4 ± 3.1 ‰ for δ18O, −126 ± 24 ‰ for δ2H, 13.0 ± 1.7 ‰ for d-excess, and 28 ± 5 per meg for 17O-excess. These values coincide with δ18O, δ2H, d-excess, and 17O-excess values estimated for a water vapor in isotope equilibrium with the amount-weighted precipitation (Table S4). As for precipitation, the atmospheric water vapor monthly averages in δ18O and δ2H increase from winter to summer, whereas averages in d-excess and 17O-excess decrease (Fig. 1; Table S4). During the 24 h monitoring, δ18O of atmospheric water vapor increased overnight from about −16 ‰ to −12 ‰ and then stabilized. The d-excess and 17O-excess of atmospheric water vapor showed diurnal variations, reaching respective minimum values of −3.2 ‰ and −10 per meg in the early morning and respective maximum values of 18.4 ‰ and 36 per meg at noon (Table S5).
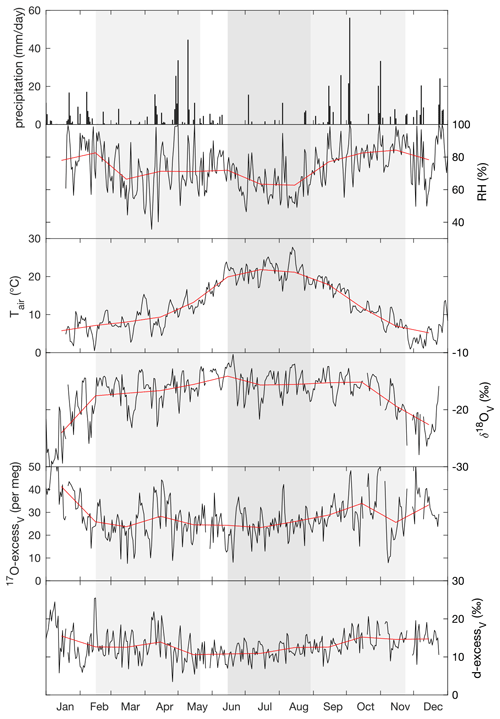
Figure 1Daily precipitation amount, daily (black) and monthly (red) means of relative humidity (RH) and atmospheric temperature (Tair) measured at 60 cm above the ground next to the grass plot, and the isotope composition of atmospheric water vapor (δ18OV, 17O-excessV, d-excessV) measured at 40 cm height above the grass plot monitored at the O3HP platform from February to November 2021. The three regrowth periods lasting from 17 February–20 May 2021 (spring), from 15 June–27 August 2021 (summer), and from 27 August–23 November 2021 (autumn) are indicated by shaded areas.
3.2 Changes in RH, temperature, stomatal conductance, transpiration, and the isotope composition of grass leaf water
Table 1 and Fig. 2 show changes in RH, h, Tair, Tplot, F. arundinacea leaf transpiration, and stomatal conductance averaged over 30 min before the eight grass leaf samplings at midday. RH is always equal to or lower than h (by less than 9 %) but co-varies with h from low values in spring and summer (30 %–40 %) to high values in autumn (ca. 64 %). Tplot is 1–3 ∘C lower than Tair but changes along with Tair from one measurement day to another, with high values in summer (ca. 25 ∘C) and lower values in spring (ca. 18 ∘C) and autumn (ca. 14 ∘C). Figure A3 shows five daily variations of Tair, Tplot, and Tleaf. Although Tleaf varies spatially within the plot, its spatial average around midday is close to Tplot (Fig. A3), supporting that Tplot can be considered an approximation of Tleaf. Transpiration and stomatal conductance are relatively stable from one measurement day to another, varying from 1.1–3.7 and from 50–130 mmol m−2 s−1, respectively (Fig. 2).
The isotope composition of F. arundinacea leaf water sampled at midday is also shown in Table 1 and Fig. 2. The grass leaf water has δ18O (−0.05 ‰ to 20.1 ‰) and δ2H (−31 ‰ to 18 ‰) that are higher than irrigation water and d-excess (−31.0 ‰ to −142.8 ‰) and 17O-excess (17 per meg to −165 per meg) that are lower than irrigation water, as can be expected for an evaporation signal. The changes in δ18O, δ2H, d-excess and 17O-excess observed from one sampling day to another follow the changes in RH and h (Fig. 2). Evaporative isotope enrichment is highest in May and July when RH is low and lowest in November when RH is high. Samples from October and November have similar d-excess as expected from little variation in RH (64 ± 2 %). However, their 17O-excess values differ by 66 per meg. The reason for this difference in 17O-excess remains unclear.
Table 1 and Fig. 3 show the 24 h evolution of the isotope composition of grass leaf water from 14–15 June 2021 in relation to RH, h, Tair, Tplot, F. arundinacea transpiration, and stomatal conductance. Tair and RH range from 14 ∘C to 31 ∘C and 38 % to 97 %, respectively. Tplot is ca. 1 ∘C higher than Tair at night and up to 4 ∘C lower than Tair during daytime. During daytime, stomatal conductance measured continuously on a single leaf ranges from 60 to 120 mmol m−2 s−1 and co-varies with transpiration (1.3–3.9 mmol m−2 s−1). However, stomatal conductance varies greatly (by 200–500 mmol m−2 s−1) between different leaves in the grass plot (Table S1, Fig. A4). At night, stomatal conductance is never higher than 20 mmol m−2 s−1, while transpiration remains lower than 0.5 mmol m−2 s−1. The isotope variability of grass leaf water on this diurnal scale is of the same order of magnitude as the changes observed among samples collected at midday in different months. The evolution of the isotope composition of grass leaf water follows RH and h, except for samples collected at night and in the early morning when transpiration is low. During this time, stomatal closure impeded exchange between the leaf and the atmosphere, decoupling the isotope composition of grass leaf water from RH.
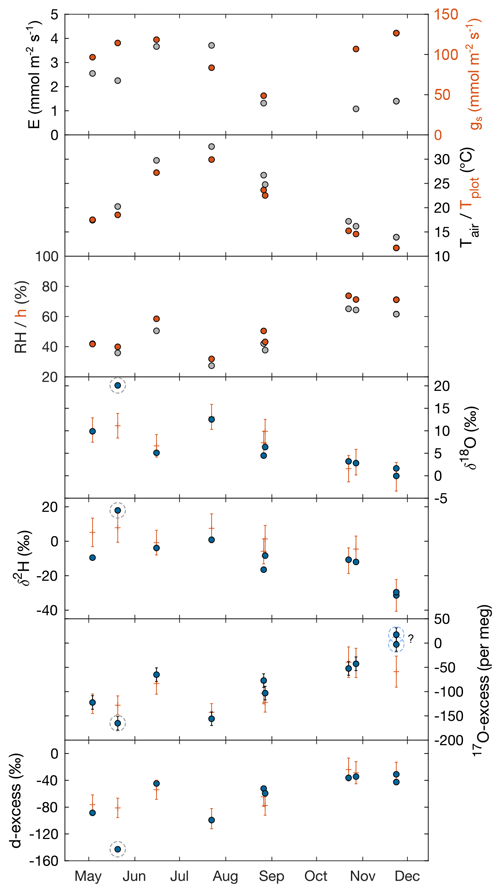
Figure 2F. arundinacea transpiration (E) and stomatal conductance (gs) measured on a single leaf blade using the LI-COR gas exchange system, atmospheric temperature (Tair), plot-scale grass leaf temperature (Tplot), relative humidity (RH), water vapor pressure ratio between leaf and the atmosphere (h), and measured (circles) and predicted (+) isotope composition of F. arundinacea leaf water (δ18O, δ2H, 17O-excess, d-excess) for midday samples over the year 2021 (see Table 1 for sampling dates). Error bars of isotope data represent analytical precision (see method section). The modeled isotope composition of bulk grass leaf water is predicted by the C–G steady-state model combined with the mixing equation (Eq. 4) using average environmental conditions over 30 min before sampling (Tables 1, S5). The model uncertainty (1 SD) was estimated using a Monte Carlo simulation accounting for uncertainty of input variables (RH ± 1 %, Tplot ± 2 ∘C, δ18OS ± 0.2 ‰, δ2HS ± 0.7 ‰, d-excessS ± 0.6 ‰, 17O-excessS ± 6 per meg, δ18OV ± 0.2 ‰, δ2HV ± 1.8 ‰, d-excessV ± 0.9 ‰, 17O-excessV ± 14 per meg, gs ± 100 mmol m−2 s−1, and the fraction of unevaporated water pools (f) ± 0.1). Dashed gray circles indicate the sample that has been likely affected by evaporation during sampling. Dashed light-blue circles indicate samples with anomalously high 17O-excess relative to d-excess.
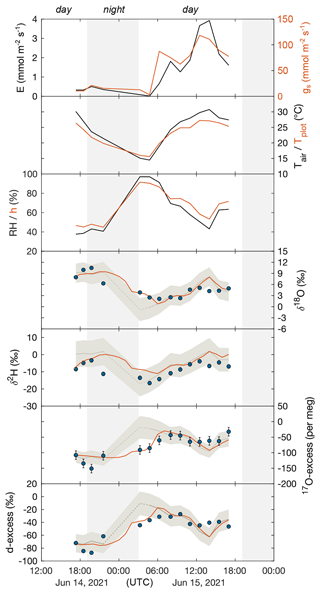
Figure 3The 24 h monitoring of F. arundinacea transpiration (E) and stomatal conductance (gs) measured on a single leaf blade using the LI-COR gas exchange system, atmospheric temperature (Tair), plot-scale grass leaf temperature (Tplot), relative humidity (RH), water vapor pressure ratio between leaf and the atmosphere (h), and the observed (circles) and predicted steady-state (pale gray curve, Eq. 4) and non-steady-state (orange curve, Eq. 6) isotope composition of F. arundinacea leaf water (δ18O, δ2H, 17O-excess, d-excess) from 14–15 June 2021. Error bars of isotope data represent analytical precision (see method section). Shaded areas mark nighttime intervals. The isotope composition of grass leaf water is predicted using average environmental conditions over 30 min before sampling (Tables 1, S5, S6). The pale gray shaded area represents model uncertainty (1 SD) of the predicted steady-state leaf water isotope composition estimated using a Monte Carlo simulation (see caption Fig. 2). The dashed part of the steady-state prediction represents the time when grass leaf water isotope composition deviates from steady state due to low transpiration and long leaf water residence times (see discussion for details).
3.2.1 Model–data comparison
For six of eight midday samplings, the isotope composition of bulk grass leaf water predicted by the C–G steady-state model combined with the mixing equation (Eq. 4) using boundary conditions averaged over 30 min before sampling (Table S5) agrees with the measured isotope values within model uncertainty, which is on average ± 2.8 ‰, ± 7.9 ‰, ± 15 ‰, and ± 24 per meg for δ18O, δ2H, d-excess, and 17O-excess, respectively (Fig. 2). Samples collected on 20 May 2021 and 23 November 2021 show larger discrepancies between observed and predicted values. The May sample has significantly higher δ18O (> 8 ‰) and δ2H (> 10 ‰) and lower d-excess (59 ‰) and 17O-excess (33 per meg) than respective steady-state values predicted by the two-pool mixing model (Eq. 4) (Fig. 2). These large deviations are indicative of stronger evaporation than expected. In view of the large magnitude of the deviation, we suppose that this sample was affected by evaporation during sampling. We therefore exclude this sample from further discussion. For the November sample, δ18O, δ2H, and d-excess agree within 1.1 ‰, 1 ‰, and 8 ‰ with the predicted steady-state values, respectively. However, the 17O-excess is 66 per meg higher than the predicted steady-state value (Fig. 2). The reason for this discrepancy remains unclear.
For the 24 h monitoring, the C–G steady-state model combined with the two-pool mixing equation (Eq. 4) reproduces the evolution of the isotope composition of grass leaf water during the day, but not at night and in the early morning, when stomatal conductance and transpiration are low (Fig. 3). During daytime, best agreement between predicted and observed grass leaf water is found for samples collected on the morning of 15 June 2021 until midday, with deviations lower than ± 0.6 ‰ for δ18O, ± 5 ‰ for δ2H, ± 6 ‰ for d-excess, and ± 8 per meg for 17O-excess. However, on the afternoon of 15 June 2021, when transpiration is highest, observed δ18O and δ2H are 1.5 ‰–4 ‰ and 3 ‰–9 ‰ lower, and d-excess and 17O-excess are 9 ‰ and 34 per meg lower than predicted values, respectively. In contrast, on the evening of 14 June 2021, observed δ18O values are 1 ‰–2 ‰ higher, whereas δ2H, d-excess, and 17O-excess are respectively 4 ‰–6 ‰, 18 ‰, and 38 per meg lower than respective steady-state values predicted by the two-pool mixing model (Eq. 4). The non-steady-state equation (Eq. 6) was applied for night predictions to match the data (Fig. 3). Differences between predicted non-steady-state and observed values at night range from 0.2 ‰–3.6 ‰ for δ18O, 5 ‰–12 ‰ for δ2H, 3 ‰–19 ‰ for d-excess, and 1–31 per meg for 17O-excess (Table S6). Note that a grass leaf water content of 6 mol m−2 is required for the model to fit the data (Table S6). This value is higher than leaf water contents reported for grasses in previous studies (2–4 mol m−2; Hirl et al., 2019; Barbour et al., 2021).
3.2.2 Sensitivity tests
Figure 4 shows for the 24 h monitoring the uncertainty of the bulk grass leaf water isotope composition predicted for steady-state conditions (Eq. 4) introduced by the precisions associated with the measurement of the main model parameters. A ± 5 % uncertainty on RH introduces an uncertainty of ± 1.5 ‰ on δ18O, ± 4.0 ‰ on δ2H, ± 10 ‰ on d-excess, and ± 13 per meg on 17O-excess of grass leaf water (Fig. 4a–d). For an RH range of 40 %–80 %, an uncertainty of ± 0.1 on the fraction of the unevaporated water pool (f) leads to an uncertainty of 2.2 ‰–0.8 ‰ on δ18O, 6 ‰–2 ‰ on δ2H, 12 ‰–4 ‰ on d-excess, and 16–6 per meg on 17O-excess (Fig. 4e–h). For the same RH range, misestimation of ΔTleaf-air by 2 ∘C leads to an uncertainty of 1.3 ‰–2.7 ‰ on δ18O, 1.5 ‰–5.1 ‰ on δ2H, 9 ‰–17 ‰ on d-excess, and 11–29 per meg on 17O-excess (Fig. 4i–l). Assuming Tleaf equals Tair, instead of measuring Tplot increases the difference between the predicted and observed daytime δ18O, δ2H, d-excess, and 17O-excess values by 1.1 ± 1.2 ‰, 2.4 ± 0.5 ‰, 5 ± 11 ‰, and 10 ± 14 per meg, respectively (Fig. 4i–l, orange curve). By contrast, assuming Tleaf is 2 ∘C lower than Tair only slightly increases the difference between predicted and observed daytime δ18O, δ2H, d-excess, and 17O-excess values by 0.2 ± 0.6 ‰, 3.0 ± 5.5 ‰, 2 ± 4 ‰, and 3 ± 5 per meg, respectively (Fig. 4i–l, light blue curve). In contrast to RH, f, and ΔTleaf-air, measurement uncertainties on the isotope composition of the source water (irrigation water) and atmospheric water vapor introduce uncertainties on the isotope composition of grass leaf water that are close to or lower than analytical precision (Fig. 4m–t). Using the isotope composition of atmospheric water vapor estimated from isotope equilibrium with the mean annual amount-weighted O3HP precipitation (Table S4) instead of measured values increases the difference between predicted and observed daytime δ18O, δ2H, d-excess, and 17O-excess values by 1.2 ± 2.0 ‰, 12.5 ± 12.3 ‰, 0 ± 2 ‰, and 3 ± 8 per meg, respectively (Fig. 4q–t, light blue curve). Observed spatial variability of stomatal conductance of up to 500 mmol m−2 s−1 introduces a bias on the δ18O, δ2H, d-excess, and 17O-excess of less than 0.5 ‰, 0.5 ‰, 3.5 ‰, and 10 per meg, respectively (Fig. 4u–x).
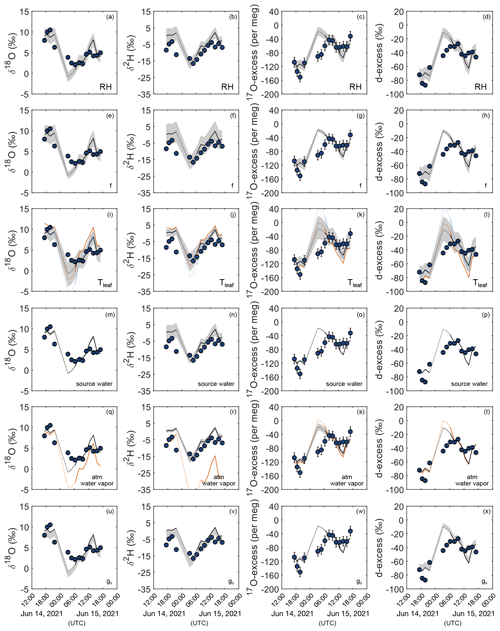
Figure 4Sensitivity of δ18O, δ2H, 17O-excess, and d-excess of leaf water to changes in environmental and plant physiological parameters. Blue circles represent measured F. arundinacea leaf water isotope composition over a 24 h period from 14–15 June 2021. The black line shows the steady-state leaf water isotope composition predicted by the C–G steady-state model combined with the mixing equation (Eq. 4) using mean boundary conditions over 30 min before sampling (Table 1). Shaded areas indicate the sensitivity of the predicted leaf water isotope composition for relative humidity (RH) (± 5 %) (a–d), the fraction of unevaporated water pools (f) (± 0.1) (e–h), leaf temperature (Tleaf) (± 2 ∘C) (i–l), the isotope composition of source water (± 0.2 ‰ for δ18OS, ± 0.7 ‰ for δ2HS, ± 0.6 ‰ for d-excessS, ± 6 per meg for 17O-excessS) (m–p), the isotope composition of atmospheric water vapor (± 0.2 ‰ for δ18OV, ± 1.8 ‰ for δ2HV, ± 0.9 ‰ for d-excessV, ± 14 per meg for 17O-excessV), and (q–t) stomatal conductance (gs) (± 100 mmol m−2 s−1) (u–x). Colored curves show the isotope composition of leaf water predicted by the C–G steady-state model combined with the mixing equation (Eq. 4) (i) when assuming leaf temperatures to be equal to atmospheric temperature (i–l, orange), (ii) when assuming leaf temperatures to be 2 ∘C lower than atmospheric temperature (i–l, light blue), and (iii) when estimating atmospheric water vapor from isotope equilibrium with source water (irrigation water) (q–t, orange).
3.3 Changes in climate averages, grass height, silicification rate, and triple oxygen isotope composition of phytolith assemblages
Table 2 shows daily and daytime climate averages, maximum grass height, silicification rate, ratio of long-cell to short- and long-cell phytoliths, and the triple oxygen isotope composition of phytoliths for the three regrowth periods. Daily average Tair is from 9 to 22 ∘C, and daily average RH is from 64 % to 81 %. Daytime average Tair is about 2.4 ∘C higher than the daily average. Daytime average RH is about 8 % lower than the daily average. Daily averages of Tplot are similar to Tair, so that RH approximates h (cf. Sect. 2.4). During daytime, averages of RH and h differ by 1 %–4 % due to ΔTleaf-air ranging from −1.1 to 0.3 ∘C. Daytime average h is 61 % in spring and summer and 76 % in autumn. The average soil water content is always higher than 0.20 ± 0.05 L L−1, whatever the regrowth, supporting that the grass plot is always well-watered, and that water stress is excluded.
Grass height increases exponentially during spring regrowth and linearly during summer regrowth (Fig. A5). During the autumn regrowth, the grass height increases only in the first month of the regrowth and stabilizes thereafter (Fig. A5). The silicification rate (from 2.7 to 5.9 SiO2 g−1 d−1) and the proportion of silicified long cell phytoliths (from 30 % to 70 % of short and long cells) increase with the number of regrowth periods, without any correlation with RH or h that varied little from one regrowth to another (Table 2). The O and 17O-excess of the grass leaf phytoliths are similar in spring and summer (36.2 ± 0.5 ‰ and −260 ± 5 per meg, respectively; Table 2) and slightly different in autumn (34.3 ‰ and −234 per meg, respectively). These isotope values fall within the range of values observed in previous growth chamber calibrations (Alexandre et al., 2018, 2019; Outrequin et al., 2021). The 17O-excessphyto coincides with the lower range of values reported for phytoliths extracted from soils in western and central Africa (Alexandre et al., 2018).
3.4 Relationship between the 17O-excess of grass phytoliths and leaf water
Numerous studies have investigated the temperature-dependent isotope fractionation between amorphous and/or biogenic silica and their formation water () with variable results (e.g., O'Neil and Clayton, 1964; Knauth and Epstein, 1976; Shemesh et al., 1992; Brandriss et al., 1998; Hu and Clayton, 2003; Dodd, 2011, and many more). Here we use temperature-dependent obtained for the diatom–water pair by Dodd and Sharp (2010) (1.0326 at 20 ∘C). The triple oxygen isotope exponent between silica and water () linking to (17α = 18αθ) has been defined as 0.524 ± 0.0002 for the 5–35 ∘C temperature range (Cao and Liu, 2011; Sharp et al., 2016). However, a different value of 0.522 ± 0.001 was consistently obtained for phytoliths, reproducible regardless of bio-climatic constraints (Outrequin et al., 2021). Using this apparent , we calculated the triple oxygen isotope compositions of the formation water (FW) in equilibrium with the phytolith samples obtained from the three regrowths (Fig. 5). The reconstructed triple oxygen isotope composition of FW is close to that estimated for daytime average climate conditions of the three regrowths using the C–G model combined with the mixing equation (Eq. 4) (Fig. 5). The differences are lower than 1.8 ‰ and 33 per meg for O and 17O-excess, respectively. Using the same , but of 0.524, the 17O-excess of FW is largely underestimated by 35–60 per meg compared to model predictions (Fig. 5).
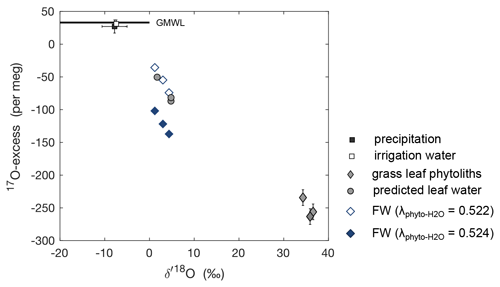
Figure 517O-excess vs. O of amount-weighted annual average precipitation, average irrigation water, and the measured isotope composition of phytoliths extracted from F. arundinacea grass leaves harvested on 20 May 2021 (spring), 27 August 2021 (summer), and 23 November 2021 (autumn). Also shown are the formation water (FW) predicted using temperature-dependent from Dodd and Sharp (2010) and of 0.522 or 0.524, and the isotope composition of bulk leaf water predicted by the C–G model for steady-state conditions combined with the mixing equation (Eq. 4) using average daytime boundary conditions for the three regrowth periods (Table 2). Error bars represent analytical precisions (see methods section), except for precipitation, for which the amount-weighted standard deviation is indicated.
4.1 Parameters responsible for discrepancies between observed and predicted isotope compositions of grass leaf water
Overall agreement between the observed and predicted leaf water δ18O and 17O-excess trends from one sampling day to another shows that the C–G steady-state model combined with the two-pool mixing equation (Eq. 4) is appropriate for estimating seasonal scale variations in the triple oxygen isotope composition of grass leaf water at midday. The two-pool mixing model also correctly reproduces the trends in triple oxygen isotope evolution of leaf water during daytime, although observed and predicted values diverge little when transpiration is maximal in the early afternoon (Fig. 3). As shown by the sensitivity tests, ΔTleaf-air contributes largely to model uncertainty (Fig. 4). Assumptions on Tleaf equal to Tair can explain the discrepancies between predicted and observed isotope values often reported in the literature. In the present case, Tleaf was indirectly measured using Tplot, and large misestimation of Tleaf (> 2 ∘C) is unlikely. Part of the small model–data discrepancies in the afternoon on 15 June 2021 can result from RH measured at 60 cm above the ground next to the grass plot being lower than RH surrounding the grass leaf canopy, due to intense soil evaporation. Another bias may come from misestimation of the unevaporated water pool f that can drive large variations in the triple oxygen isotope composition of leaf water, as shown by the sensitivity tests. The value of 0.2 chosen for f in the present study is at the lower limit of previously reported values selected for grass species (0.2–0.4; Hirl et al., 2019; Barbour et al., 2021). Considering a value for f of 0.4 instead of 0.2 would minimize the discrepancy between observed and predicted δ18O of leaf water for the samples taken in the afternoon on 15 June 2021 (Fig. 4). Some studies suggested that f may increase with increasing transpiration, due to increased advection of unevaporated xylem water, known as the Péclet effect (Farquhar and Lloyd, 1993; Cuntz et al., 2007). In contrast to a recent isotope study that found no evidence for the Péclet effect in grass leaves (Hirl et al., 2019), the data from the 24 h monitoring show a significant positive correlation (R2 = 0.49) between transpiration and the difference between observed and predicted δ18O values of leaf water. Considering the Péclet effect (Eq. 5) instead of a simple mixing significantly reduces model–data discrepancies by 50 %–80 % and leads to deviations between predicted and observed δ18O and 17O-excess of grass leaf water in the afternoon on 15 June 2021 that are lower than 1.1 ‰, and 12 per meg, respectively (Table S5). The Péclet effect can thus explain that the observed triple oxygen isotope composition of leaf water varies less than predicted when transpiration is high.
In agreement with previous studies on δ18O and δ2H (Farquhar and Cernusak, 2005; Cernusak et al., 2016), a non-steady-state model is used to reproduce the trends in isotope evolution of leaf water at night when stomatal conductance and transpiration are low. Our results confirm the applicability of this model for the triple oxygen isotope composition of leaf water. In addition, the model–data comparison shows the advantage of 17O-excess over d-excess in detecting isotope non-steady state in leaf water on a diurnal scale. Figure 6a illustrates the 17O-excess vs. O evolution of leaf water from the beginning to the end of the night when transpiration is too low to reach the isotope steady state. RH of 96 ± 2 % persisting between 03:00 and 07:00 (LT) on 15 June 2021 drives the theoretical isotope steady-state values to the upper end of the predicted trend on Fig. 6a. However, due to the long leaf water residence time, the observed leaf water isotope composition evolves only slowly towards these values without reaching them. This is well captured by the concave curvature of the non-steady-state prediction (Fig. 6a). In contrast, linearity of evaporation trends in the d-excess vs. δ18O space challenges the differentiation between isotope steady-state and non-steady-state conditions, as illustrated in Fig. 6b.
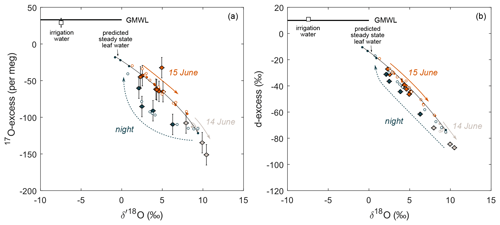
Figure 6Comparison of predicted (small circles) and observed (large circles) F. arundinacea leaf water over a 24 h period from 14–15 June 2021 in diagrams of (a) 17O-excess vs. O and (b) d-excess vs. δ18O. Filled circles indicate the steady-state model prediction (Eq. 4, Table S5); open circles indicate the non-steady-state model prediction (Eq. 6, Table S6). Colors differentiate samples collected between 19:15 and 21:45 (LT) on 14 June 2021 (gray), between 14 June 2021 at 23:30 and 15 June 2021 at 08:15 (LT) (blue), and between 10:00 and 19:00 on 15 June 2021 (orange). The black line serves as a guide of the eye for the trend in modeled isotope steady-state values. The average isotope composition of the irrigation water over the experimental period is also shown. The global meteoric water line (GMWL) is shown for comparison.
4.2 What can we learn from measurements of Tplot and triple oxygen isotope composition of atmospheric water vapor?
The sensitivity tests highlight the importance of plot-scale grass leaf temperature and the isotope composition of atmospheric water vapor for accurate prediction of the isotope composition of leaf water.
The influence of variations in Tleaf relative to Tair on the isotope composition of leaf water is two-fold. On the one hand, changes in Tleaf slightly modify the magnitude of equilibrium isotope fractionation at the liquid–vapor interface. A change of a few degrees in Tleaf is however of minor importance for the isotope composition of leaf water. In contrast, changes in ΔTleaf-air, associated with changes in Tleaf, modify the water vapor pressure ratio between the leaf and the atmosphere, i.e., h. For example, a decrease in Tleaf from 20 to 18 ∘C at constant Tair of 20 ∘C modifies h by 5 %–10 % for RH ranging from 40 % to 80 %. As h is the major driver of isotope variability in leaf water, even little variations in ΔTleaf-air can therefore significantly influence the isotope composition of leaf water (Fig. 4i–l).
Accurate measurement of Tleaf on plot scale is challenging, as Tleaf can vary considerably in space and time, particularly according to soil moisture, leaf transpiration, canopy structure and position, net radiation, elevation, and latitude (Still et al., 2019). Sufficient soil moisture supports transpiration, which generally leads to leaf cooling, i.e., Tleaf lower than Tair. On the contrary, water stress is compensated by stomata closure, which stops transpiration and increases Tleaf. In this case, Tleaf may exceed Tair, as demonstrated for irrigated vs. rain-fed crops (Siebert et al., 2014). The amplitude of ΔTleaf-air also increases with leaf size (Leuzinger and Körner, 2007). ΔTleaf-air lower than or equivalent to −2 ∘C was reported, at the ecosystem scale, for tropical forests (Rey-Sánchez et al., 2016), grasslands, or cold desert areas, whereas larger differences were reported for cold forests and warm desert areas (Blonder and Michaletz, 2018). In the present case, continuous irrigation of the grass plot sustained the transpiration, leading to a daytime Tleaf consistently near or below the daytime Tair (Figs. A3, A6). However, under natural conditions, estimation of Tleaf 2 ∘C lower than or equal to Tair may lead to significant bias in modeled leaf water isotope composition. Figure A3 shows that Tplot can be used to estimate Tleaf. The measurement of Tplot using IR radiometry as performed here is easy to set up and is strongly recommended if high accuracy is sought in the estimate of Tleaf at plot scale.
The isotope difference between source water and the atmosphere is another key determinant of the leaf water isotope composition. According to the C–G model (Eq. 2), the influence of atmospheric water vapor relative to source water becomes increasingly important with increasing h (or RH). While the isotope composition of source water can be often constrained by measurements, accurate estimates of the isotope composition of atmospheric water vapor are difficult to obtain. In the absence of direct measurements, the δ18O of atmospheric water vapor is often assumed to be in equilibrium with precipitation (e.g., Cernusak et al., 2002; Voelker et al., 2014; Bush et al., 2017; Li et al., 2017; Song et al., 2011; Flanagan and Farquhar, 2014). However, a recent comparison between modeled vapor and precipitation isotope compositions obtained from different isotope-enabled global climate models suggests that precipitation is rarely in isotope equilibrium with atmospheric water vapor (Fiorella et al., 2019). The deviation generally increases with increasing latitude. In continental areas, the δ18O of near-surface atmospheric water vapor can be lower than suggested by isotope equilibrium with precipitation due to high evaporation fluxes from lakes (Krabbenhoft et al., 1990; Benson and White, 1994). Similarly, the δ18O of atmospheric water vapor can be lower than suggested by isotope equilibrium, if precipitation is affected by sub-cloud re-evaporation, as has been reported for monsoon areas (Landais et al., 2010; Wen et al., 2010). Moreover, the equilibrium assumption is often not valid in semi-arid to arid regions, when precipitation is limited to a short period of the year and not representative for the annual average atmospheric conditions (Tsujimura et al., 2007; Voigt et al., 2021). The atmospheric water vapor record presented here supports the validity of the equilibrium assumption at the study site, for annual δ18O, δ2H, d-excess, and 17O-excess averages. The agreement remains good at the monthly scale, but significant discrepancies occur for d-excess and 17O-excess during the summer months when RH is the lowest. Sub-cloud re-evaporation of precipitation can be invoked to explain the low d-excess and 17O-excess in summer precipitation, whereas d-excess and 17O-excess of atmospheric water vapor remain stable. At the diurnal scale, primary isotope ratios of atmospheric water vapor can vary strongly, often deviating from the monthly equilibrium value. This can lead to significant model–data discrepancies (Fig. 4). On a diurnal scale, 17O-excess and d-excess of atmospheric water vapor generally agree with the monthly equilibrium water vapor at daytime, when transpiration is high, but significantly deviate at night and in the early morning. Notably, the variations in 17O-excess of atmospheric water vapor over the 24 h monitoring are low (45 per meg) compared to its large variability observed in leaf water (120 per meg) (cf. Tables 1 and S5). In comparison, δ18O shows much higher variability in atmospheric water vapor (5 ‰) compared to leaf water (8 ‰) (cf. Tables 1 and S5).
4.3 Does the 17O-excess of grass leaf phytoliths reflect daily or daytime RH?
The triple oxygen isotope composition of bulk grass leaf phytoliths is influenced by their distribution along the leaf blade in relation to the leaf water isotope gradient and to silicification patterns (Alexandre et al., 2019; Outrequin et al., 2021). The triple oxygen isotope gradient along grass leaf blades can be predicted by a string-of-lakes model (Alexandre et al., 2019). However, the triple oxygen isotope composition of the bulk grass leaf water is independent of grass leaf length and predictable by the C–G model combined with the mixing equation (Eq. 4) (Alexandre et al., 2019) or a Péclet effect. The bulk phytolith FW integrates the whole grass elongation period and is thus different from the sampled bulk leaf waters that only represent a snapshot in time. Short cells are among the first cell types to be silicified, sometimes even before the leaf transpires (Motomura et al., 2004; Kumar et al., 2017). The process is metabolically controlled and does not depend on the transpiration rate. Long cell silicification occurs in a second step in relation to transpiration (Motomura et al., 2004; Kumar et al., 2017). Moreover, in grass leaves, the epidermal cells are produced at the base of the leaf and pushed upward during the growth. Hence, epidermal cells along the leaf blade gather phytoliths that were formed at short and long distances relative to the leaf base, i.e., at isotopically low and high evaporative conditions, respectively. The combination of these two processes likely causes the apparent to be lower than the established (= 0.524; Sharp et al., 2016) (Outrequin et al., 2021). The consistency of equal to 0.522 ± 0.001 observed in this study and in previous calibrations (Outrequin et al., 2021) supports that the silicification patterns are systematic and similar for different climate conditions.
The relationship between 17O-excessphyto and RH was previously investigated in two growth chamber experiments where F. arundinacea was grown under different conditions of RH (40 %–60 %–80 %) and Tair (20–24–28 ∘C) (Alexandre et al., 2018; Outrequin et al., 2021). The parameters were set constant for more than 10 d, without day–night cycles. Differences in δ18O values between source water and atmospheric water vapor were set to 0 ‰ in the first experiment (Alexandre et al., 2018) and to 10 ‰ in the second experiment (Outrequin et al., 2021). The two equations obtained from these experiments were statistically similar (Outrequin et al., 2021). Linear regression through both datasets (n = 16) gives
Here, under natural conditions, we investigate whether the RH obtained from Eq. (7) reflects daytime or daily conditions. RH values reconstructed from 17O-excessphyto obtained for the three regrowths applying Eq. (7) are closer to daytime averages (underestimation of RH by 4 ± 4 %) than to daily averages (underestimation of RH by 12 ± 5 %) (Fig. 7a, Table 2).
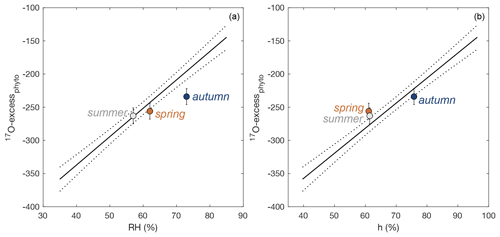
Figure 7Observed 17O-excessphyto vs. average daytime (a) relative humidity (RH), and (b) water vapor pressure ratio between the leaf and the atmosphere (h), for regrowth periods in spring, summer, and autumn. The growth chamber calibration lines with 95 % confidence interval (Eqs. 6, 7) are shown for comparison.
At night, low stomatal conductance and transpiration measured on F. arundinacea likely hamper the silicification due to cell water saturation relative to silica formation during daytime transpiration, explaining that daytime RH determines 17O-excessphyto (Fig. 7). Further, the low stomatal conductance of grasses observed at night causes its leaf water to deviate from isotope steady state. Hence, the 17O-excess of grass leaf water at night remains close to daytime values (Fig. 6). The low amount of phytoliths that may form overnight thus introduces little bias to the 17O-excessphyto of the phytolith sample. Nighttime stomatal conductance, however, can vary across biomes, depending among others on plant functional types and soil moisture (Tobin and Kulmatiski, 2018; Resco de Dios et al., 2019). A recent data compilation reported that tropical trees show the highest stomatal conductance at night, followed by desert species (Resco de Dios et al., 2019). The lowest stomatal conductance was found for non-tropical evergreen angiosperms including Mediterranean species. Therefore, for a given case, the magnitude of nighttime vs. daytime transpiration must be assessed to determine whether the RH reconstructed from 17O-excessphyto reflects day and night or only daytime conditions.
RH estimated from 17O-excessphyto can be biased by variations in ΔTleaf-air. This is because the isotope composition of leaf water is not directly determined by RH, but rather the water vapor pressure ratio between the leaf and the atmosphere, i.e., h (cf. Eq. 2). As discussed in Sect. 4.2, ΔTleaf-air of −2 ∘C leads to h values that are 5 %–10 % higher than RH. The calibration line obtained from growth chamber experiments is calibrated for ΔTleaf-air of −2 ∘C (Outrequin et al., 2021). The lower ΔTleaf-air ranging from −1.1 to 0.3 ∘C observed in our study can explain the general underestimation of RH reconstructed from the calibration line (cf. Fig. 7a). The effect of ΔTleaf-air can be removed when considering h instead of RH. We used the same datasets from the growth chamber experiments as for RH (Alexandre et al., 2018; Outrequin et al., 2021; n = 16) to obtain a relationship between 17O-excessphyto and h, assuming that Tleaf was 2 ∘C lower than Tair:
h values reconstructed from 17O-excessphyto obtained for the three regrowths applying Eq. (8) are in good agreement with corresponding observed daytime averages (Fig. 7b, Table 2). The deviations between reconstructed and measured daytime h values (1 ± 5 %) are lower than for RH −4 ± 4 %). However, the difference is insignificant considering the uncertainty on the reconstructed values (4 %). A small amplitude of ΔTleaf-air, as observed in the present study (< 1.1 ∘C), has thus little impact on the RH estimates from 17O-excessphyto. However, the possibility of larger amplitude, especially in the case of cold forests or warm desert areas, should be considered when interpreting 17O-excessphyto in terms of RH.
4.4 Future tracks for reconstruction of past RH from 17O-excess of phytoliths extracted from soils
Assessing the relationship between 17O-excessphyto and RH is crucial for accurate reconstructions using phytolith assemblages extracted from sediments, which are supplied by soil phytoliths from the catchment area. Soil phytoliths likely represent several decades of phytolith production. The limited variation of 17O-excess in meteoric water (Aron et al., 2021; Surma et al., 2021) and atmospheric water vapor and its insensitivity of 17O-excessphyto to temperature make it a powerful indicator of RH. The results of the present study reveal that grass leaf phytoliths record daytime RH under the studied eco-climatic conditions but emphasize that daytime vs. nighttime stomatal conductance and ΔTleaf-air need to be considered when interpreting 17O-excessphyto in terms of RH. In soils, the accurate interpretation of 17O-excessphyto is further complicated by the mixture of phytoliths from transpiring (leaves, inflorescences) and non-transpiring plant tissues (stems). As previously reported, grass stem phytoliths contribute to less than 10 % dry weight of the above-ground grass silica content (Webb and Longstaffe, 2002; Ding et al., 2008; Alexandre et al., 2019). A simple calculation shows that this contribution should increase 17O-excessphyto of grass phytolith assemblages extracted from soils by less than 20 per meg relative to an only grass leaf blade phytolith sample, biasing RH estimates obtained from Eq. (7) by less than 5 % towards higher values.
When tree phytoliths contribute to soil phytolith assemblages, globular granulate phytoliths are abundant (Alexandre et al., 2011, 2018; Aleman et al., 2012). This phytolith type is assumed to form in the non-transpiring secondary xylem of the wood (Collura and Neumann, 2017). However, investigation of phytolith assemblages extracted from soils under different vegetation types, including grass savannas, wooded savannas, and enclosed savannas developed under similar RH conditions, shows the same range of 17O-excessphyto values in agreement with the 17O-excessphyto vs. RH relationship obtained from the growth chamber calibration (Alexandre et al., 2018). This suggests that the FW of the globular granulate phytoliths can be affected by evaporation and calls for further investigation of its anatomical origin.
17O-excess provides useful insights into evaporation processes at the soil–plant–atmosphere interface as it varies little in rainfall and atmospheric water vapor at the annual scale. In this study, a model–data approach was used to interpret the diurnal and seasonal evolution of the triple oxygen isotope composition of F. arundinacea bulk grass leaf water. The C–G steady-state model associated with a two-pool mixing equation reliably predicts the triple oxygen isotope composition of grass leaf water during daytime, when all model-relevant parameters are measured. The few model–data discrepancies (up to 4 ‰, 9 ‰, and 34 per meg for δ18O, d-excess, and 17O-excess, respectively) are likely related to differences between Tplot and actual Tleaf, variations in the fraction of the unevaporated water pool with changes in transpiration (i.e., Péclet effect), and/or slight differences between measured RH close to the grass plot and actual RH right around the grass leaves. Deviations of the isotope composition of leaf water from steady state at night are well captured by a non-steady-state model. These deviations from steady state can also be identified in the 17O-excess vs. O system, whereas this is not the case in the d-excess vs. δ18O system. This example shows that measuring the triple oxygen isotope composition of leaf water contributes to a better understanding of water exchange at the soil–plant–atmosphere interface.
The ability to measure the grass Tleaf showed that ΔTleaf-air is a key determinant of the isotope composition of leaf water. Under the study conditions, it is close to −2 ∘C at midday, which is in line with the ΔTleaf-air previously observed on F. arundinacea in climate-controlled growth chambers (Alexandre et al., 2019). To gain further insights into this parameter and its variability according to vegetation and climate types, we recommend IR radiometer measurements with spatial coverage as carried out in the present study.
The first continuous record of atmospheric water vapor including δ17O measurement at a natural site presented here shows that although δ17O, δ18O, and δ2H are highly variable at the daily scale, assuming isotope equilibrium between precipitation and atmospheric water vapor is reasonable for these first-order parameters at monthly and annual scales. The second-order parameters (d-excess and 17O-excess) vary little at the daily, monthly, and annual scales and are always close to the equilibrium values estimated from precipitation. Further records of the triple oxygen isotope composition of the atmospheric water vapor, facilitated by the use of laser spectrometers, and precipitation will help to generalize this result.
The measured values of 17O-excessphyto and daytime RH fit well with the 17O-excessphyto vs. RH equation established from previous growth chamber experiments (Alexandre et al., 2018; Outrequin et al., 2021). However, we emphasize that the magnitude of nighttime stomatal conductance and transpiration needs to be assessed in each study individually to evaluate if RH reconstructed from 17O-excessphyto reflects daily or daytime conditions. Small ΔTleaf-air values of less than 2 ∘C as observed in the present study have little impact on the RH estimates from 17O-excessphyto. However, larger ΔTleaf-air as common in cold forests or warm desert vegetation should be considered when reconstructing RH using 17O-excessphyto in these contexts. The insights gained from this study provide important tracks for the interpretation of 17O-excess of phytoliths accumulated in soils and sediments in terms of RH. The study also confirms the consistency of and for grasses, which implies that the distribution of phytoliths along grass leaf blades is virtually invariant. This also opens perspectives for reconstructing past changes in leaf water isotope composition from the triple oxygen isotope composition of fossil grass phytolith assemblages recovered from buried soils and sediments, e.g., useful for land–surface model and data comparisons.
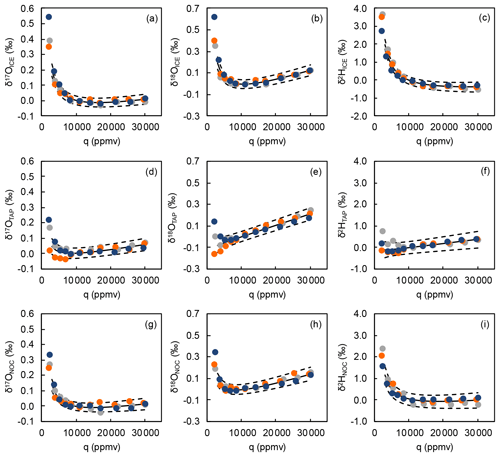
Figure A1Water mixing ratio dependencies of δ17O, δ18O, and δ2H normalized to the isotope composition measured at a water mixing ratio (q) of 10 000 ppmv for the three water standards (a–c ICE (δ18O = −26.85 ‰), d–f NOC (δ18O = −16.91 ‰), g–i TAP (δ18O = −8.64 ‰)). Mixing ratio dependency calibrations were performed on 26 May 2021 (gray), 20 October 2021 (orange), and 05 January 2022 (blue). Solid and dashed lines show mean and 1 standard deviation of the mixing ratio dependency function.
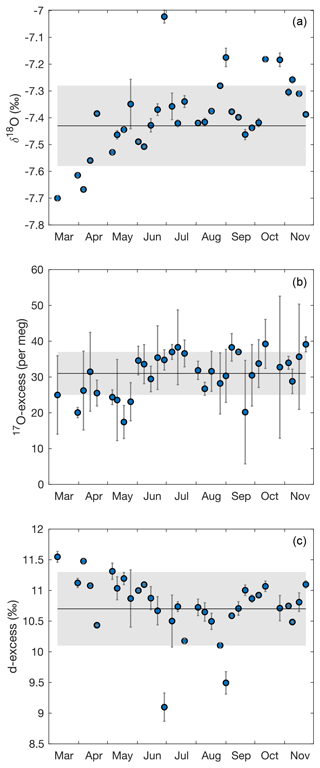
Figure A2Evolution of (a) δ18O, (b) 17O-excess and (c) d-excess of the irrigation water from March to November 2021. Each data point represents the average isotope composition of the irrigation water over the period between two samples. Error bars are 1 standard deviation (SD). The solid lines and the gray shaded areas indicate mean and SD of the isotope composition of irrigation water averaged over all samples.
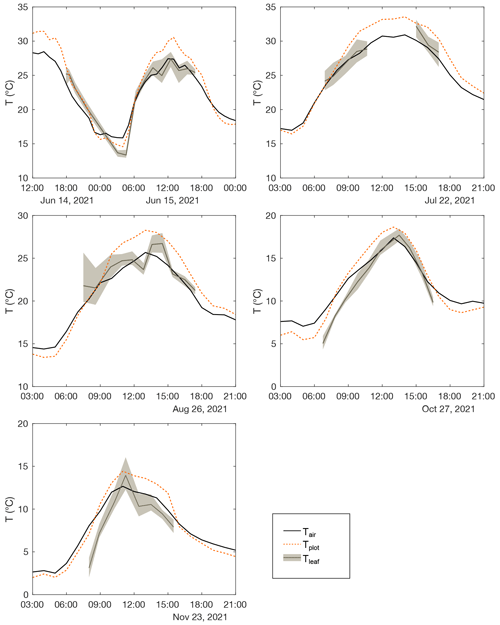
Figure A3Diurnal evolution of atmospheric temperature (Tair), plot-scale grass leaf temperature (Tplot), and mean and 1 standard deviation of leaf temperature measurements on single leaves using the Optris IR thermometer (Tleaf) measured on field days between April and November 2021.
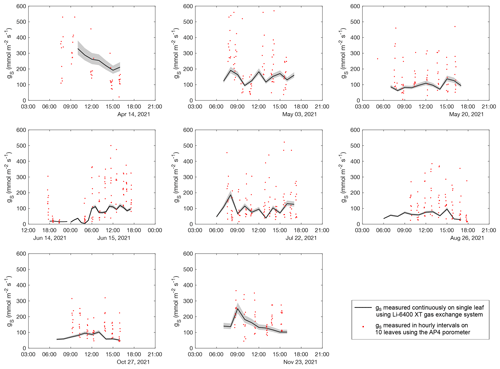
Figure A4Diurnal evolution of stomatal conductance (gs) measured on field days between April and November 2021. Black lines show gs of a single grass leaf measured continuously over the day using the LI-COR gas exchange system in hourly resolution. Red points represent gs of different grass leaves measured with the AP4 porometer.
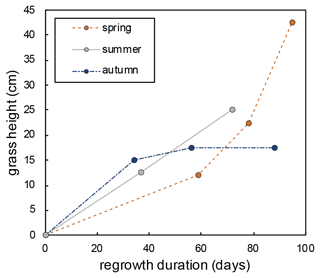
Figure A5Evolution of the grass height over the regrowth duration from 17 February–20 May 2021 (spring), from 15 June–27 August 2021 (summer), and from 27 August–23 November 2021 (autumn).
Data are available within the article or its Supplement. Additional data (e.g., LI-COR measurement data) will be made available on request. Meteorological data can be accessed from the COOPERATE database (Reiter et al., 2015).
The supplement related to this article is available online at: https://doi.org/10.5194/bg-20-2161-2023-supplement.
AA conceptualized the project and acquired financial support. AA, CV, CVC, IR, JPO, and CP designed the experiments and carried out fieldwork. CV, AA, JCM, CVC, CS, HM, JA, and JPO performed laboratory analyses. CV and AA prepared the manuscript with contributions from all co-authors.
The contact author has declared that none of the authors has any competing interests.
Publisher’s note: Copernicus Publications remains neutral with regard to jurisdictional claims in published maps and institutional affiliations.
We acknowledge Martine Couapel for her help with the field experiments. We thank Nicholas Devert and Sabrina Dubois for performing leaf water extractions at ISPA, Bordeaux, France. We thank Michael Staubwasser, Daniel Herwartz, and Mohammed El-Shenawy for permission and support during leaf water triple oxygen and hydrogen isotope analyses in the Stable Isotope Laboratory at the University of Cologne, Germany. This study was conducted in the framework of the HUMI-17 project supported by the ANR (ANR-17-CE01-0002-01), CNRS FR3098 ECCOREV, LABEX OT-Med, and OSU-Pytheas. It benefited from the CNRS resources allocated to the French ECOTRONS Research Infrastructure, from the Occitanie Region and FEDER investments as well as from the state allocation “Investissement d'Avenir” AnaEE-France ANR-11-INBS-0001. We also thank the editor (Cindy De Jonge), Marco Lehmann, and one anonymous reviewer for their useful comments, which contributed to improving the manuscript.
This research has been supported by the ANR (ANR-17-CE01-0002-01) and benefited from AnaEE-France ANR-11-INBS-0001. The O3HP platform benefited from the state allocation “Investissement d'Avenir” AnaEE-France ANR-11-INBS-0001 as well as financial and human resource support from the IMBE (UMR7263 CNRS, AMU, IRD, UA), OHP (UAR3470 CNRS), and ECCOREV (FR3098 CNRS).
This paper was edited by Cindy De Jonge and reviewed by Marco Lehmann and one anonymous referee.
Aemisegger, F., Spiegel, J. K., Pfahl, S., Sodemann, H., Eugster, W., and Wernli, H.: Isotope meteorology of cold front passages: A case study combining observations and modeling, Geophys. Res. Lett., 42, 5652–5660, https://doi.org/10.1002/2015GL063988, 2015.
Aleman, J., Leys, B., Apema, R., Bentaleb, I., Dubois, M. A., Lamba, B., Lebamba, J., Martin, C., Ngomanda, A., Truc, L., Yangakola, J. M., Favier, C., and Bremond, L.: Reconstructing savanna tree cover from pollen, phytoliths and stable carbon isotopes, J. Veg. Sci., 23, 187–197, https://doi.org/10.1111/j.1654-1103.2011.01335.x, 2012.
Alexandre, A., Basile-Doelsch, I., Sonzogni, C., Sylvestre, F., Parron, C., Meunier, J. D., and Colin, F.: Oxygen isotope analyses of fine silica grains using laser-extraction technique: Comparison with oxygen isotope data obtained from ion microprobe analyses and application to quartzite and silcrete cement investigation, Geochim. Cosmochim. Ac., 70, 2827–2835, https://doi.org/10.1016/j.gca.2006.03.003, 2006.
Alexandre, A., Bouvet, M., and Abbadie, L.: The role of savannas in the terrestrial Si cycle: A case-study from Lamto, Ivory Coast, Glob. Planet. Change, 78, 162–169, https://doi.org/10.1016/j.gloplacha.2011.06.007, 2011.
Alexandre, A., Crespin, J., Sylvestre, F., Sonzogni, C., and Hilbert, D. W.: The oxygen isotopic composition of phytolith assemblages from tropical rainforest soil tops (Queensland, Australia): Validation of a new paleoenvironmental tool, Clim. Past, 8, 307–324, https://doi.org/10.5194/cp-8-307-2012, 2012.
Alexandre, A., Landais, A., Vallet-Coulomb, C., Piel, C., Devidal, S., Pauchet, S., Sonzogni, C., Couapel, M., Pasturel, M., Cornuault, P., Xin, J., Mazur, J. C., Prié, F., Bentaleb, I., Webb, E., Chalié, F., and Roy, J.: The triple oxygen isotope composition of phytoliths as a proxy of continental atmospheric humidity: Insights from climate chamber and climate transect calibrations, Biogeosciences, 15, 3223–3241, https://doi.org/10.5194/bg-15-3223-2018, 2018.
Alexandre, A., Webb, E., Landais, A., Piel, C., Devidal, S., Sonzogni, C., Couapel, M., Mazur, J., Pierre, M., Prié, F., Vallet-Coulomb, C., Outrequin, C., and Roy, J.: Effects of leaf length and development stage on the triple oxygen isotope signature of grass leaf water and phytoliths: insights for a proxy of continental atmospheric humidity, Biogeosciences, 16, 4613–4625, https://doi.org/10.5194/bg-16-4613-2019, 2019.
Aron, P. G., Levin, N. E., Beverly, E. J., Huth, T. E., Passey, B. H., Pelletier, E. M., Poulsen, C. J., Winkelstern, I. Z., and Yarian, D. A.: Triple oxygen isotopes in the water cycle, Chem. Geol., 565, 120026, https://doi.org/10.1016/j.chemgeo.2020.120026, 2021.
Barbeta, A., Burlett, R., Martín-Gómez, P., Fréjaville, B., Devert, N., Wingate, L., Domec, J. C., and Ogée, J.: Evidence for distinct isotopic compositions of sap and tissue water in tree stems: consequences for plant water source identification, New Phytol., 233, 1121–1132, https://doi.org/10.1111/nph.17857, 2022.
Barbour, M. M., Loucos, K. E., Lockhart, E. L., Shrestha, A., McCallum, D., Simonin, K. A., Song, X., Griffani, D. S., and Farquhar, G. D.: Can hydraulic design explain patterns of leaf water isotopic enrichment in C3 plants?, Plant Cell Environ., 44, 432–444, https://doi.org/10.1111/pce.13943, 2021.
Barkan, E. and Luz, B.: High precision measurements of 17O/16O and 18O 16O ratios in H2O, Rapid Commun. Mass Sp., 19, 3737–3742, https://doi.org/10.1002/rcm.2250, 2005.
Barkan, E. and Luz, B.: Diffusivity fractionations of HO HO and HO HO in air and their implications for isotope hydrology, Rapid Commun. Mass Sp., 21, 2999–3005, https://doi.org/10.1002/rcm.3180, 2007.
Belviso, S., Reiter, I. M., Loubet, B., Gros, V., Lathière, J., Montagne, D., Delmotte, M., Ramonet, M., Kalogridis, C., Lebegue, B., Bonnaire, N., Kazan, V., Gauquelin, T., Fernandez, C., and Genty, B.: A top-down approach of surface carbonyl sulfide exchange by a Mediterranean oak forest ecosystem in southern France, Atmos. Chem. Phys., 16, 14909–14923, https://doi.org/10.5194/acp-16-14909-2016, 2016.
Benson, L. V. and White, J. W. C.: Stable isotopes of oxygen and hydrogen in the Truckee River-Pyramid Lake surface-water system, 3. Source of water vapor overlying Pyramid Lake, Limnol. Oceanogr., 39, 1945–1958, https://doi.org/10.4319/lo.1994.39.8.1945, 1994.
Blonder, B. and Michaletz, S. T.: A model for leaf temperature decoupling from air temperature, Agr. Forest. Meteorol., 262, 354–360, https://doi.org/10.1016/j.agrformet.2018.07.012, 2018.
Bögelein, R., Thomas, F. M., and Kahmen, A.: Leaf water 18O and 2H enrichment along vertical canopy profiles in a broadleaved and a conifer forest tree, Plant Cell Environ., 40, 1086–1103, https://doi.org/10.1111/pce.12895, 2017.
Brandriss, M. E., O'Neil, J. R., Edlund, M. B., and Stoermer, E. F.: Oxygen isotope fractionation between diatomaceous silica and water, Geochim. Cosmochim. Ac., 62, 1119–1125, https://doi.org/10.1016/S0016-7037(98)00054-4, 1998.
Bremond, L., Alexandre, A., Peyron, O., and Guiot, J.: Grass water stress estimated from phytoliths in West Africa, J. Biogeogr., 32, 311–327, https://doi.org/10.1111/j.1365-2699.2004.01162.x, 2005.
Buhay, W. M., Edwards, T. W. D., and Aravena, R.: Evaluating kinetic fractionation factors used for reconstructions from oxgen and hydrogen isotope ratios in plant water and cellulose, Geochim. Cosmochim. Ac., 60, 2209–2218, https://doi.org/10.1016/0016-7037(96)00073-7, 1996.
Bush, R. T., Berke, M. A., and Jacobson, A. D.: Plant Water δD and δ18O of Tundra Species from West Greenland, Arct. Antarct. Alp Res., 49, 341–358, https://doi.org/10.1657/AAAR0016-025, 2017.
Cao, X. and Liu, Y.: Equilibrium mass-dependent fractionation relationships for triple oxygen isotopes, Geochim. Cosmochim. Ac., 75, 7435–7445, https://doi.org/10.1016/j.gca.2011.09.048, 2011.
Cernusak, L. A., Pate, J. S., and Farquhar, G. D.: Diurnal variation in the stable isotope composition of water and dry matter in fruiting Lupinus angustifolius under field conditions, Plant Cell Environ., 25, 893–907, https://doi.org/10.1046/j.1365-3040.2002.00875.x, 2002.
Cernusak, L. A., Barbour, M. M., Arndt, S. K., Cheesman, A. W., English, N. B., Feild, T. S., Helliker, B. R., Holloway-Phillips, M. M., Holtum, J. A. M., Kahmen, A., Mcinerney, F. A., Munksgaard, N. C., Simonin, K. A., Song, X., Stuart-Williams, H., West, J. B., and Farquhar, G. D.: Stable isotopes in leaf water of terrestrial plants, Plant Cell Environ., 39, 1087–1102, https://doi.org/10.1111/pce.12703, 2016. Chapligin, B., Meyer, H., Friedrichsen, H., Marent, A., Sohns, E., and Hubberten, H.-W.: A high-performance, safer and semi-automated approach for the δ18O analysis of diatom silica and new methods for removing exchangeable oxygen, Rapid Commun. Mass Sp., 24, 2655–2664, https://doi.org/10.1002/rcm.4689, 2010.
Collura, L. V. and Neumann, K.: Wood and bark phytoliths of West African woody plants, Quaternary Int., 434, 142–159, https://doi.org/10.1016/j.quaint.2015.12.070, 2017.
Corbineau, R., Reyerson, P. E., Alexandre, A., and Santos, G. M.: Towards producing pure phytolith concentrates from plants that are suitable for carbon isotopic analysis, Rev. Palaeobot. Palynol., 197, 179–185, https://doi.org/10.1016/j.revpalbo.2013.06.001, 2013.
Craig, H. and Gordon, L. I.: Deuterium and oxygen-18 variations in the ocean and the marine atmosphere, in: Stable Isotopes in Oceanographic Studies and Paleotemperatures, edited by: Tongiorgi, E., Spoleto, Consiglio Nazionale Delle Ricerche, Laboratorio di Geologia Nucleare, Pisa, Italy, 9–130, 1965.
Crespin, J., Alexandre, A., Sylvestre, F., Sonzogni, C., Paillès, C., and Garreta, V.: IR laser extraction technique applied to oxygen isotope analysis of small biogenic silica samples, Anal. Chem., 80, 2372–2378, https://doi.org/10.1021/ac071475c, 2008.
Cuntz, M., Ogée, J., Farquhar, G. D., Peylin, P., and Cernusak, L. A.: Modelling advection and diffusion of water isotopologues in leaves, Plant Cell Environ., 30, 892–909, https://doi.org/10.1111/j.1365-3040.2007.01676.x, 2007.
Diao, H., Schuler, P., Goldsmith, G. R., Siegwolf, R. T., Saurer, M., and Lehmann, M. M.: Technical note: On uncertainties in plant water isotopic composition following extraction by cryogenic vacuum distillation, Hydrol. Earth Syst. Sci., 26, 5835–5847, https://doi.org/10.5194/hess-26-5835-2022, 2022.
Ding, T. P., Zhou, J. X., Wan, D. F., Chen, Z. Y., Wang, C. Y., and Zhang, F.: Silicon isotope fractionation in bamboo and its significance to the biogeochemical cycle of silicon, Geochim. Cosmochim. Ac., 72, 1381–1395, https://doi.org/10.1016/j.gca.2008.01.008, 2008.
Dodd, J. P.: Oxygen isotopes in diatom silica: a new understanding of silica-water oxygen isotope fractionation in diatom frustules and an application of diatom δ18O values as a record of paleohydrologic variability in a Middle-Pleistocene lacustrine core from the Valles Caldera, New Mexico, 1–108, University of New Mexico, https://digitalrepository.unm.edu/eps_etds/17 (last access: 13 June 2023), 2011.
Dodd, J. P. and Sharp, Z. D.: A laser fluorination method for oxygen isotope analysis of biogenic silica and a new oxygen isotope calibration of modern diatoms in freshwater environments, Geochim. Cosmochim. Ac., 74, 1381–1390, https://doi.org/10.1016/j.gca.2009.11.023, 2010.
Dongmann, G., Nürnberg, H. W., Förstel, H., and Wagener, K.: On the enrichment of HO in the leaves of transpiring plants, Radiat. Environ. Biophys., 11, 41–52, https://doi.org/10.1007/BF01323099, 1974.
Farquhar, G. D. and Cernusak, L. A.: On the isotopic composition of leaf water in the non-steady state, Funct. Plant Biol., 32, 293–303, https://doi.org/10.1071/FP04232, 2005.
Farquhar, G. D. and Gan, K. S.: On the progressive enrichment of the oxygen isotopic, Plant Cell Environ., 26, 1579–1597, https://doi.org/10.1046/j.1365-3040.2003.01013.x, 2003.
Farquhar, G. D. and Lloyd, J.: Carbon and Oxygen Isotope Effects in the Exchange of Carbon Dioxide between Terrestrial Plants and the Atmosphere, in: Stable Isotopes and Plant Carbon-Water Relations, Academic Press, 47–70, https://doi.org/10.1016/B978-0-08-091801-3.50011-8, 1993.
Farquhar, G. D., Cernusak, L. A., and Barnes, B.: Heavy water fractionation during transpiration, Plant Physiol., 143, 11–18, https://doi.org/10.1104/pp.106.093278, 2007.
Farris, F. and Strain, B. R.: The effects of water-stress on leaf HO enrichment, Radiat. Environ. Biophys., 15, 167–202, https://doi.org/10.1007/BF01323264, 1978.
Fiorella, R. P., West, J. B., and Bowen, G. J.: Biased estimates of the isotope ratios of steady-state evaporation from the assumption of equilibrium between vapour and precipitation, Hydrol. Process., 33, 2576–2590, https://doi.org/10.1002/hyp.13531, 2019.
Flanagan, L. B. and Farquhar, G. D.: Variation in the carbon and oxygen isotope composition of plant biomass and its relationship to water-use efficiency at the leaf- and ecosystem-scales in a northern Great Plains grassland, Plant Cell Environ., 37, 425–438, https://doi.org/10.1111/pce.12165, 2014.
Flanagan, L. B., Comstock, J. P., and Ehleringer, J. R.: Comparison of Modeled and Observed Environmental Influences on the Stable Oxygen and Hydrogen Isotope Composition of Leaf Water in Phaseolus vulgaris L., Plant Physiol., 96, 588–596, https://doi.org/10.1104/pp.96.2.588, 1991.
Gan, K. S., Wong, S. C., Yong, J. W. H., and Farquhar, G. D.: 18O Spatial Patterns of Vein Xylem Water, Leaf Water, and Dry Matter in Cotton Leaves, Plant Physiol., 130, 1008–1021, https://doi.org/10.1104/pp.007419, 2002.
Garcin, Y., Schwab, V. F., Gleixner, G., Kahmen, A., Todou, G., Séné, O., Onana, J. M., Achoundong, G., and Sachse, D.: Hydrogen isotope ratios of lacustrine sedimentary n-alkanes as proxies of tropical African hydrology: Insights from a calibration transect across Cameroon, Geochim. Cosmochim. Ac., 79, 106–126, https://doi.org/10.1016/j.gca.2011.11.039, 2012.
Garcin, Y., Schefuß, E., Dargie, G. C., Hawthorne, D., Lawson, I. T., Sebag, D., Biddulph, G. E., Crezee, B., Bocko, Y. E., Ifo, S. A., Mampouya Wenina, Y. E., Mbemba, M., Ewango, C. E. N., Emba, O., Bola, P., Kanyama Tabu, J., Tyrrell, G., Young, D. M., Gassier, G., Girkin, N. T., Vane, C. H., Adatte, T., Baird, A. J., Boom, A., Gulliver, P., Morris, P. J., Page, S. E., Sjögersten, S., and Lewis, S. L.: Hydroclimatic vulnerability of peat carbon in the central Congo Basin, Nature, 612, 277–282, https://doi.org/10.1038/s41586-022-05389-3, 2022.
Graf, P., Wernli, H., Pfahl, S., and Sodemann, H.: A new interpretative framework for below-cloud effects on stable water isotopes in vapour and rain, Atmos. Chem. Phys., 19, 747–765, https://doi.org/10.5194/acp-19-747-2019, 2019.
Gröning, M., Lutz, H. O., Roller-Lutz, Z., Kralik, M., Gourcy, L., and Pöltenstein, L.: A simple rain collector preventing water re-evaporation dedicated for δ18O and δ2H analysis of cumulative precipitation samples, J. Hydrol., 448–449, 195–200, https://doi.org/10.1016/j.jhydrol.2012.04.041, 2012.
Grossiord, C., Buckley, T. N., Cernusak, L. A., Novick, K. A., Poulter, B., Siegwolf, R. T. W., Sperry, J. S., and McDowell, N. G.: Plant responses to rising vapor pressure deficit, New Phytol., 226, 1550–1566, https://doi.org/10.1111/nph.16485, 2020.
Helliker, B. R. and Ehleringer, J. R.: Establishing a grassland signature in veins: 18O in the leaf water of C3 and C4 grasses, P. Natl. Acad. Sci. USA, 94, 7894–7898, https://doi.org/10.1073/pnas.97.14.7894, 2000.
Helliker, B. R. and Ehleringer, J. R.: Differential 18O enrichment of leaf cellulose in C3 versus C4 grasses, Funct. Plant Biol., 29, 435–442, https://doi.org/10.1071/PP01122, 2002a.
Helliker, B. R. and Ehleringer, J. R.: Grass blades as tree rings: Environmentally induced changes in the oxygen isotope ratio of cellulose along the length of grass blades, New Phytol., 155, 417–424, https://doi.org/10.1046/j.1469-8137.2002.00480.x, 2002b.
Hirl, R. T., Schnyder, H., Ostler, U., Schäufele, R., Schleip, I., Vetter, S. H., Auerswald, K., Baca Cabrera, J. C., Wingate, L., Barbour, M. M., and Ogée, J.: The 18O ecohydrology of a grassland ecosystem – predictions and observations, Hydrol. Earth Syst. Sci., 23, 2581–2600, https://doi.org/10.5194/hess-23-2581-2019, 2019.
Holloway-Phillips, M., Cernusak, L. A., Barbour, M., Song, X., Cheesman, A., Munksgaard, N., Stuart-Williams, H., and Farquhar, G. D.: Leaf vein fraction influences the Péclet effect and 18O enrichment in leaf water, Plant Cell Environ., 39, 2414–2427, https://doi.org/10.1111/pce.12792, 2016.
Hu, G. and Clayton, R. N.: Oxygen isotope salt effects at high pressure and high temperature and the calibration of oxygen isotope geothermometers, Geochim. Cosmochim. Ac., 67, 3227–3246, https://doi.org/10.1016/S0016-7037(02)01319-4, 2003.
IPCC: Climate Change 2013: The Physical Science Basis, Contribution of Working Group I to the Fifth Assessment Report of the Intergovernmental Panel on Climate Change, edited by: Stocker, T. F., Qin, D., Plattner, G.-K., Tignor, M., Allen, S. K., Boschung, J., Nauels, A., Xia, Y., Bex, V., and Midgley, P. M., Cambridge University Press, Cambridge, United Kingdom and New York, NY, USA, 1585 pp., https://doi.org/10.1017/CBO9781107415324, 2013.
IUSS Working Group WRB: World Reference Base for Soil Resources 2014, update 2015. International soil classification system for naming soils and creating legends for soil maps, World Soil Resources Reports No. 106, FAO, Rome, ISBN 978-92-5-108369-7, 2015.
Jacob, H. and Sonntag, C.: An 8-year record of the seasonal variation of 2H and 18O in atmospheric water vapour and precipitation at Heidelberg, Germany, Tellus B, 43 291–300, https://doi.org/10.1034/j.1600-0889.1991.t01-2-00003.x, 1991.
Kahmen, A., Sachse, D., Arndt, S. K., Tu, K. P., Farrington, H., Vitousek, P. M., and Dawsona, T. E.: Cellulose δ18O is an index of leaf-to-air vapor pressure difference (VPD) in tropical plants, P. Natl. Acad. Sci. USA, 108, 1981–1986, https://doi.org/10.1073/pnas.1018906108, 2011.
Kahmen, A., Schefuß, E., and Sachse, D.: Leaf water deuterium enrichment shapes leaf wax n-alkane δD values of angiosperm plants I: Experimental evidence and mechanistic insights, Geochim. Cosmochim. Ac., 111, 39–49, https://doi.org/10.1016/j.gca.2012.09.003, 2013.
Knauth, L. P. and Epstein, S.: Hydrogen and oxygen isotope ratios in nodular and bedded cherts, Geochim. Cosmochim. Ac., 40, 95–1108, https://doi.org/10.1016/0016-7037(76)90051-X, 1976.
Krabbenhoft, D. P., Bowser, C. J., Anderson, M. P., and Valley, J. W.: Estimating groundwater exchange with lakes: 1. The stable isotope mass balance method, Water Resour. Res., 26, 2445–2453, https://doi.org/10.1029/WR026i010p02445, 1990.
Kumar, S., Soukup, M., and Elbaum, R.: Silicification in grasses: Variation between different cell types, Front. Plant Sci., 8, 1–8, https://doi.org/10.3389/fpls.2017.00438, 2017.
Landais, A., Risi, C., Bony, S., Vimeux, F., Descroix, L., Falourd, S., and Bouygues, A.: Combined measurements of 17Oexcess and d-excess in African monsoon precipitation: Implications for evaluating convective parameterizations, Earth Planet Sc. Lett., 298, 104–112, https://doi.org/10.1016/j.epsl.2010.07.033, 2010.
Leaney, F. W., Osmond, C. B., Allison, G. B., and Ziegler, H.: Hydrogen-isotope composition of leaf water in C3 and C4 plants: its relationship to the hydrogen-isotope composition of dry matter, Planta, 164, 215–220, https://doi.org/10.1007/BF00396084, 1985.
Lee, X., Smith, R., and Williams, J.: Water vapour 18O 16O isotope ratio in surface air in New England, USA, Tellus B, 58, 293–304, https://doi.org/10.1111/j.1600-0889.2006.00191.x, 2006.
Leuzinger, S. and Körner, C.: Tree species diversity affects canopy leaf temperatures in a mature temperate forest, Agr. Forest. Meteorol., 146, 29–37, https://doi.org/10.1016/j.agrformet.2007.05.007, 2007.
Li, S., Levin, N. E., Soderberg, K., Dennis, K. J., and Caylor, K. K.: Triple oxygen isotope composition of leaf waters in Mpala, central Kenya, Earth Planet Sc. Lett., 468, 38–50, https://doi.org/10.1016/j.epsl.2017.02.015, 2017.
Liu, H. T., Schäufele, R., Gong, X. Y., and Schnyder, H.: The δ18O and δ2H of water in the leaf growth-and-differentiation zone of grasses is close to source water in both humid and dry atmospheres, New Phytol., 214, 1423–1431, https://doi.org/10.1111/nph.14549, 2017.
Liu, P., Liu, J., Ji, A., Reinhard, C. T., Planavsky, N. J., Babikov, D., Najjar, R. G., and Kasting, J. F.: Triple oxygen isotope constraints on atmospheric O2 and biological productivity during the mid-Proterozoic, P. Natl. Acad. Sci USA, 118, e2105074118, https://doi.org/10.1073/pnas.2105074118, 2021.
López, J., Way, D. A., and Sadok, W.: Systemic effects of rising atmospheric vapor pressure deficit on plant physiology and productivity, Glob. Change Biol., 27, 1704–1720, https://doi.org/10.1111/gcb.15548, 2021.
Loucos, K. E., Simonin, K. A., Song, X., and Barbour, M. M.: Observed relationships between leaf HO Péclet effective length and leaf hydraulic conductance reflect assumptions in Craig-Gordon model calculations, Tree Physiol., 35, 16–26, https://doi.org/10.1093/treephys/tpu110, 2014.
Luz, B. and Barkan, E.: Variations of 17O 16O and 18O 16O in meteoric waters, Geochim. Cosmochim. Ac., 74, 6276–6286, https://doi.org/10.1016/j.gca.2010.08.016, 2010.
Majoube, M.: Fractionnement en oxygène 18 et en deutérium entre l'eau et sa vapeur, J. Chim. Phys., 68, 1423–1436, https://doi.org/10.1051/jcp/1971681423, 1971.
Merlivat, L.: Molecular diffusivities of HO, HD16O, and HO in gases, J. Chem. Phys., 69, 2864–2871, https://doi.org/10.1063/1.436884, 1978.
Monteith, J.: Evaporation and environment, Symp. Soc. Exp. Biol., 19, 205–234, 1965.
Motomura, H., Fujii, T., and Suzuki, M.: Silica deposition in relation to ageing of leaf tissues in Sasa veitchii (Carrière) Rehder (Poaceae: Bambusoideae), Ann. Bot., 93, 235–248, https://doi.org/10.1093/aob/mch034, 2004.
Nogué, S., Whicher, K., Baker, A. G., Bhagwat, S. A., and Willis, K. J.: Phytolith analysis reveals the intensity of past land use change in the Western Ghats biodiversity hotspot, Quaternary Int., 437, 82–89, https://doi.org/10.1016/j.quaint.2015.11.113, 2017.
Ogée, J., Cuntz, M., Peylin, P., and Bariac, T.: Non-steady-state, non-uniform transpiration rate and leaf anatomy effects on the progressive stable isotope enrichment of leaf water along monocot leaves, Plant Cell Environ., 30, 367–387, https://doi.org/10.1111/j.1365-3040.2006.01621.x, 2007.
O'Neil, J. R. and Clayton, R. N.: Oxygen isotope geothermometry, in: Isotope and Cosmic Chemistry, edited by: Craig, H. et al., North-Holland, Amsterdam, 157–168, 1964.
Outrequin, C., Alexandre, A., Vallet-Coulomb, C., Piel, C., Devidal, S., Landais, A., Couapel, M., Mazur, J.-C., Peugeot, C., Pierre, M., Prié, F., Roy, J., Sonzogni, C., and Voigt, C.: The triple oxygen isotope composition of phytoliths, a new proxy of atmospheric relative humidity: controls of soil water isotope composition, temperature, CO2 concentration and relative humidity, Clim. Past, 17, 1881–1902, https://doi.org/10.5194/cp-17-1881-2021, 2021.
Penchenat, T., Vimeux, F., Daux, V., Cattani, O., Viale, M., Villalba, R., Srur, A., and Outrequin, C.: Isotopic Equilibrium Between Precipitation and Water Vapor in Northern Patagonia and Its Consequences on δ18O cellulose Estimate, J. Geophys. Res.-Biogeo., 125, e2019JG005418, https://doi.org/10.1029/2019JG005418, 2020.
Perry, C. C., Williams, R. J. P., and Fry, S. C.: Cell Wall Biosynthesis during Silicification of Grass Hairs, J. Plant Physiol., 126, 437–448, https://doi.org/10.1016/S0176-1617(87)80028-7, 1987.
Reiter I. M., Castagnoli G., and Rotereau A.: COOPERATE database, https://cooperate.eccorev.fr/db (last access: 8 June 2022), 2015.
Resco de Dios, V., Chowdhury, F. I., Granda, E., Yao, Y., and Tissue, D. T.: Assessing the potential functions of nocturnal stomatal conductance in C3 and C4 plants, New Phytol., 223, 1696–1706, https://doi.org/10.1111/nph.15881, 2019.
Rey-Sánchez, A. C., Slot, M., Posada, J. M., and Kitajima, K.: Spatial and seasonal variation in leaf temperature within the canopy of a tropical forest, Clim. Res., 71, 75–89, https://doi.org/10.3354/cr01427, 2016.
Ripullone, F., Matsuo, N., Stuart-Williams, H., Suan, C. W., Borghetti, M., Tani, M., and Farquhar, G.: Environmental effects on oxygen isotope enrichment of leaf water in cotton leaves, Plant Physiol., 146, 729–736, https://doi.org/10.1104/pp.107.105643, 2008.
Roden, J. S., Lin, G., and Ehleringer, J. R.: A mechanistic model for interpretation of hydrogen and oxygen isotope ratios in tree-ring cellulose, Geochim. Cosmochim. Ac., 64, 21–35, https://doi.org/10.1016/S0016-7037(99)00195-7, 2000.
Sharp, Z. D., Gibbons, J. A., Maltsev, O., Atudorei, V., Pack, A., Sengupta, S., Shock, E. L., and Knauth, L. P.: A calibration of the triple oxygen isotope fractionation in the SiO2-H2O system and applications to natural samples, Geochim. Cosmochim. Ac., 186, 105–119, https://doi.org/10.1016/j.gca.2016.04.047, 2016.
Shemesh, A., Charles, C. D., and Fairbanks, R. G.: Oxygen Isotopes in Biogenic Silica: Global Changes in Ocean Temperature and Isotopic Composition, Science, 256, 1434–1436, https://doi.org/10.1126/science.256.5062.1434, 1992.
Siebert, S., Ewert, F., Eyshi Rezaei, E., Kage, H., and Graß, R.: Impact of heat stress on crop yield – On the importance of considering canopy temperature, Environ. Res. Lett., 9, 044012, https://doi.org/10.1088/1748-9326/9/4/044012, 2014.
Song, X., Barbour, M. M., Saurer, M., and Helliker, B. R.: Examining the large-scale convergence of photosynthesis-weighted tree leaf temperatures through stable oxygen isotope analysis of multiple data sets, New Phytol., 192, 912–924, https://doi.org/10.1111/j.1469-8137.2011.03851.x, 2011.
Song, X., Simonin, K. A., Loucos, K. E., and Barbour, M. M.: Modelling non-steady-state isotope enrichment of leaf water in a gas-exchange cuvette environment, Plant Cell Environ., 38, 2618–2628, https://doi.org/10.1111/pce.12571, 2015.
Still, C., Powell, R., Aubrecht, D., Kim, Y., Helliker, B., Roberts, D., Richardson, A. D., and Goulden, M.: Thermal imaging in plant and ecosystem ecology: applications and challenges, Ecosphere, 10, e02768, https://doi.org/10.1002/ecs2.2768, 2019.
Surma, J., Assonov, S., Bolourchi, M. J., and Staubwasser, M.: Triple oxygen isotope signatures in evaporated water bodies from the Sistan Oasis, Iran, Geophys. Res. Lett., 42, 8456–8462, https://doi.org/10.1002/2015GL066475, 2015.
Surma, J., Assonov, S., and Staubwasser, M.: Triple Oxygen Isotope Systematics in the Hydrologic Cycle, Rev. Mineral. Geochem., 86, 401–428, https://doi.org/10.2138/rmg.2021.86.12, 2021.
Tierney, J. E., Poulsen, C. J., Montañez, I. P., Bhattacharya, T., Feng, R., Ford, H. L., Hönisch, B., Inglis, G. N., Petersen, S. V., Sagoo, N., Tabor, C. R., Thirumalai, K., Zhu, J., Burls, N. J., Foster, G. L., Goddéris, Y., Huber, B. T., Ivany, L. C., Turner, S. K., Lunt, D. J., McElwain, J. C., Mills, B. J. W., Otto-Bliesner, B. L., Ridgwell, A., and Zhang, Y. G.: Past climates inform our future, Science, 370, eaay3701, https://doi.org/10.1126/science.aay3701, 2020.
Tobin, R. L. and Kulmatiski, A.: Plant identity and shallow soil moisture are primary drivers of stomatal conductance in the savannas of Kruger National Park, PLoS One, 13, 1–17, https://doi.org/10.1371/journal.pone.0191396, 2018.
Treydte, K., Boda, S., Graf Pannatier, E., Fonti, P., Frank, D., Ullrich, B., Saurer, M., Siegwolf, R., Battipaglia, G., Werner, W., and Gessler, A.: Seasonal transfer of oxygen isotopes from precipitation and soil to the tree ring: Source water versus needle water enrichment, New Phytol., 202, 772–783, https://doi.org/10.1111/nph.12741, 2014.
Tsujimura, M., Sasaki, L., Yamanaka, T., Sugimoto, A., Li, S. G., Matsushima, D., Kotani, A., and Saandar, M.: Vertical distribution of stable isotopic composition in atmospheric water vapor and subsurface water in grassland and forest sites, eastern Mongolia, J. Hydrol., 333, 35–46, https://doi.org/10.1016/j.jhydrol.2006.07.025, 2007.
Tuthorn, M., Zech, R., Ruppenthal, M., Oelmann, Y., Kahmen, A., del Valle, H. F., Eglinton, T., Rozanski, K., and Zech, M.: Coupling δ2H and δ18O biomarker results yields information on relative humidity and isotopic composition of precipitation – a climate transect validation study, Biogeosciences, 12, 3913–3924, https://doi.org/10.5194/bg-12-3913-2015, 2015.
Vallet-Coulomb, C., Couapel, M., and Sonzogni, C.: Improving memory effect correction to achieve high precision analysis of δ17O, δ18O δ2H, 17O-excess and d-excess in water using cavity ring-down laser spectroscopy, Rapid Commun. Mass Sp., 35, e9108, https://doi.org/10.1002/rcm.9108, 2021.
Voelker, S. L., Brooks, J. R., Meinzer, F. C., Roden, J., Pazdur, A., Pawelczyk, S., Hartsough, P., Snyder, K., Plavcová, L., and Šantruček, J.: Reconstructing relative humidity from plant δ18O and δD as deuterium deviations from the global meteoric water line, Ecol. Appl., 24, 960–975, https://doi.org/10.1890/13-0988.1, 2014.
Voigt, C., Herwartz, D., Dorador, C., and Staubwasser, M.: Triple oxygen isotope systematics of evaporation and mixing processes in a dynamic desert lake system, Hydrol. Earth Syst. Sci., 25, 1211–1228, https://doi.org/10.5194/hess-25-1211-2021, 2021.
Voigt, C., Vallet-Coulomb, C., Piel, C., and Alexandre, A.: 17O-excess and d-excess of atmospheric water vapor measured by cavity ring-down spectrometry: Evidence of a matrix effect and implication for the calibration procedure, Rapid Commun. Mass Sp., 36, e9227, https://doi.org/10.1002/rcm.9227, 2022.
Wang, P., Yamanaka, T., Li, X. Y., Wu, X., Chen, B., Liu, Y., Wei, Z., and Ma, W.: A multiple time scale modeling investigation of leaf water isotope enrichment in a temperate grassland ecosystem, Ecol. Res., 33, 901–915, https://doi.org/10.1007/s11284-018-1591-3, 2018.
Webb, E. A. and Longstaffe, F. J.: The oxygen isotopic compositions of silica phytoliths and plant water in grasses: Implications for the study of paleoclimate, Geochim. Cosmochim. Ac., 64, 767–780, https://doi.org/10.1016/S0016-7037(99)00374-9, 2000.
Webb, E. A. and Longstaffe, F. J.: Climatic influences on the oxygen isotopic composition of biogenic silica in prairie grass, Geochim. Cosmochim. Ac., 66, 1891–1904, https://doi.org/10.1016/S0016-7037(02)00822-0, 2002.
Webb, E. A. and Longstaffe, F. J.: Identifying the δ18O signature of precipitation in grass cellulose and phytoliths: Refining the paleoclimate model, Geochim. Cosmochim. Ac., 70, 2417–2426, https://doi.org/10.1016/j.gca.2006.02.024, 2006.
Wen, X. F., Zhang, S. C., Sun, X., Yu, G., and Lee, X.: Water vapor and precipitation isotope ratios in Beijing, China, J. Geophys. Res.-Atmos., 115, 1–10, https://doi.org/10.1029/2009JD012408, 2010.
Yakir, D., Berry, J. A., Giles, L., and Osmond, C. B.: Isotopic heterogeneity of water in transpiring leaves: identification of the component that controls the δ18O of atmospheric O2 and CO2, Plant Cell Environ., 17, 73–80, https://doi.org/10.1111/j.1365-3040.1994.tb00267.x, 1994.
Zech, M., Mayr, C., Tuthorn, M., Leiber-Sauheitl, K., and Glaser, B.: Oxygen isotope ratios (18O 16O) of hemicellulose-derived sugar biomarkers in plants, soils and sediments as paleoclimate proxy I: Insight from a climate chamber experiment, Geochim. Cosmochim. Ac., 126, 614–623, https://doi.org/10.1016/j.gca.2013.10.048, 2014.