the Creative Commons Attribution 4.0 License.
the Creative Commons Attribution 4.0 License.
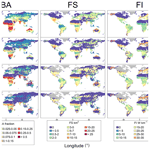
The response of wildfire regimes to Last Glacial Maximum carbon dioxide and climate
Iain Colin Prentice
Sandy P. Harrison
Climate and fuel availability jointly control the incidence of wildfires. The effects of atmospheric CO2 on plant growth influence fuel availability independently of climate, but the relative importance of each in driving large-scale changes in wildfire regimes cannot easily be quantified from observations alone. Here, we use previously developed empirical models to simulate the global spatial pattern of burnt area, fire size, and fire intensity for modern and Last Glacial Maximum (LGM; ∼ 21 000 ka) conditions using both realistic changes in climate and CO2 and sensitivity experiments to separate their effects. Three different LGM scenarios are used to represent the range of modelled LGM climates. We show large, modelled reductions in burnt area at the LGM compared to the recent period, consistent with the sedimentary charcoal record. This reduction was predominantly driven by the effect of low CO2 on vegetation productivity. The amplitude of the reduction under low-CO2 conditions was similar regardless of the LGM climate scenario and was not observed in any LGM scenario when only climate effects were considered, with one LGM climate scenario showing increased burning under these conditions. Fire intensity showed a similar sensitivity to CO2 across different climates but was also sensitive to changes in vapour pressure deficit (VPD). Modelled fire size was reduced under LGM CO2 in many regions but increased under LGM climates because of changes in wind strength, dry days (DDs), and diurnal temperature range (DTR). This increase was offset under the coldest LGM climate in the northern latitudes because of a large reduction in VPD. These results emphasize the fact that the relative magnitudes of changes in different climate variables influence the wildfire regime and that different aspects of climate change can have opposing effects. The importance of CO2 effects imply that future projections of wildfire must take rising CO2 into account.
- Article
(3198 KB) - Full-text XML
-
Supplement
(2058 KB) - BibTeX
- EndNote
Climate influences the occurrence of wildfires both through fire weather, which affects the probability of wildfire start and spread, and the long-term establishment of vegetation which is strongly controlled by temperature and precipitation (Bradstock, 2010; Pausas and Ribeiro, 2013). It has been suggested that current climate change, driven by increasing atmospheric CO2 levels, will increase wildfire risk in many regions through increased fuel dryness whilst potentially reducing wildfire risk in some regions due to decreasing fuel availability (e.g. Abatzoglou et al., 2019; Bowman et al., 2020; Harrison et al., 2021; Rogers et al., 2020). However, atmospheric CO2 levels also affect fuel loads independently of climate through physiological effects on photosynthesis, which cascade into plant growth rates (Bond et al., 2003; Bond and Midgley, 2012; Kgope et al., 2010). Much emphasis has been placed on recent and future changes in fire weather (see e.g. Abatzoglou et al., 2019; Betts et al., 2015; Flannigan et al., 2013; Jolly et al., 2015). However, increases in atmospheric CO2 concentrations promote vegetation productivity, thus altering fuel availability and loads, as well as affecting fuel types through e.g. woody thickening (Buitenwerf et al., 2012; Donohue et al., 2013; Knorr et al., 2016; Martin Calvo et al., 2014; Martin Calvo and Prentice, 2015; Pausas, 2014). Fuel properties have different effects on different aspects of the fire regime, with fire size being strongly constrained by fuel continuity and with fire intensity being limited by fuel loads (Archibald et al., 2013; Haas et al., 2022). Thus, CO2-induced changes in vegetation properties will most likely affect these aspects of wildfire regimes differently.
One reason the impact of CO2 on wildfires is poorly constrained is the difficulty of isolating it based on observations alone. Satellite records only span ∼ 25 years, a relatively short period to monitor the effect of changing CO2 levels on the vegetation properties that influence wildfires. Furthermore, changes in atmospheric CO2 levels and climate are temporally correlated, and since both affect vegetation, it is difficult to attribute changes in observations to one or the other. An alternative approach is to use process-based fire-enabled vegetation models which explicitly account for the physiological effects of CO2 and can be used to examine the temporal and spatial patterns of wildfires under different conditions. Process-based models have been used to examine the impact of climate and atmospheric CO2 changes on both vegetation and wildfire at the Last Glacial Maximum (LGM; 21 000 years ago) (Martin Calvo et al., 2014; Martin Calvo and Prentice, 2015). The LGM is a useful out-of-sample experiment since the climate forcing is of similar magnitude to the change expected by the end of the century in high-end scenarios, though it is of opposite sign (Kageyama et al., 2021). The LGM had a generally colder and drier climate than today, with CO2 levels of ∼ 185 ppm. Palaeorecords show reduced vegetation productivity and forest cover (Harrison and Prentice, 2003; Kaplan et al., 2016; Moreno et al., 2018), and ice core and sedimentary charcoal records indicate reduced biomass burning globally (Albani et al., 2018; Harrison et al., 2022; Marlon et al., 2016; Rubino et al., 2015). Although this reduction could reflect the colder and drier conditions, model experiments suggests that low CO2 also played a crucial role. Experiments using the coupled biogeography and biogeochemistry model BIOME4 (Kaplan et al., 2003) showed that it was necessary to include the direct effect of CO2 to simulate observed global and regional reductions in forest cover during the Glacial (Bragg et al., 2013; Harrison and Prentice, 2003). Similarly, Martin Calvo et al. (2014) showed that low CO2 was necessary to simulate the observed reduction of biomass burning in LGM experiments using the LPX (Land Surface Processes and eXchanges) fire-enabled vegetation model.
In this analysis, we use three empirical models (Haas et al., 2022) to explore the relative importance of climate and of CO2 to the global spatial patterns of burnt area, fire size, and fire intensity. We performed two experiments under realistic modern CO2 and climate conditions (MOD climate/MOD CO2 and LGM climate/LGM CO2). We also performed two counterfactual sensitivity experiments to quantify the sensitivity of each wildfire property to climate and CO2 independently (MOD climate/LGM CO2 and LGM climate/MOD CO2). Comparisons to LGM charcoal records from the Reading Palaeofire Database (RPD) (Harrison et al., 2022) were used to examine which experiments provided the most realistic spatial patterns.
Haas et al. (2022) developed empirical models of the global spatial patterns of burnt area (BA), fire size (FS), and fire intensity (FI) using generalized linear modelling (GLM) of modern observations. Here we use these models to simulate the global spatial patterns of burnt area (BA), fire size (FS), and fire intensity (FI) under four climate and CO2 scenarios (Fig. 1). We used two realistic scenarios: (a) MOD climate and CO2 conditions and (b) LGM climate and CO2. We ran two sensitivity experiments (a) combining MOD climate/LGM CO2 and (b) combining LGM climate/MOD CO2 levels. The empirical models use climate, vegetation, topography, lightning ignitions, land cover, road density, and human population density as predictors to represent the environmental controls on each of the wildfire properties.
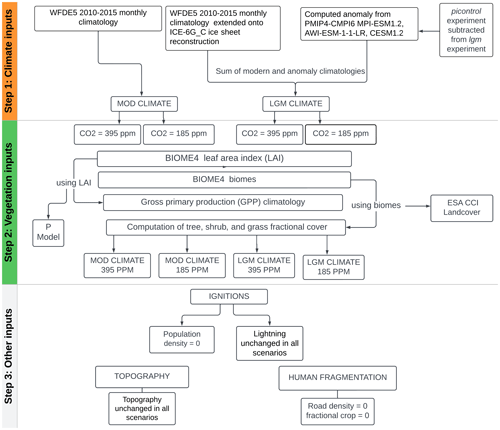
Figure 1Flowchart of the method to obtain each of the four scenarios: MOD climate/MOD CO2, LGM climate/LGM CO2, MOD climate/LGM CO2, and LGM climate/LGM CO2.
Modern (MOD) climate data (daily temperature (T), daily precipitation (P), photosynthetic photon flux density (PPFD), monthly wind speeds (wind), vapour pressure deficit (VPD), monthly specific humidity (huss), cloud cover (cld), monthly pressure (Pa)) were obtained from the WFDE5 bias-adjusted ERA5 database (Cucchi et al., 2020) for 2010 to 2015. The number of monthly dry days (DDs) (days with ≤ 1 mm of precipitation); the monthly diurnal temperature range (DTR) (daily maximum temperature – daily minimum temperature); and the monthly vapour pressure deficit (VPD), which is a function of specific humidity, temperature, and pressure, were all calculated following the methodology in Haas et al. (2022). Seasonal climatologies were derived for all variables eliminating inter-annual variability. For each grid cell, values from the month with (on average) the maximum number of DDs, the largest DTR, and the highest VPD were selected. Wind speed value was taken from the hottest month of the year (determined from the WFDE5 2 m air temperature; Cucchi et al., 2020). For lightning, the mean value over the seasonal climatology was selected. A seasonality predictor to account for wet vs. dry seasons was constructed by dividing the range of monthly values from the seasonal DD climatology by the mean value of all 12 months. Expanded ice sheets in North America, Fennoscandia, Greenland, and Antarctica resulted in global sea levels ∼ 120 m lower than today at the LGM. The modern climate data were extrapolated out onto the exposed shelves using the ICE-6G_C (Peltier et al., 2015) boundary conditions and a nearest-neighbour approach from the GeoInterpolation package in R.
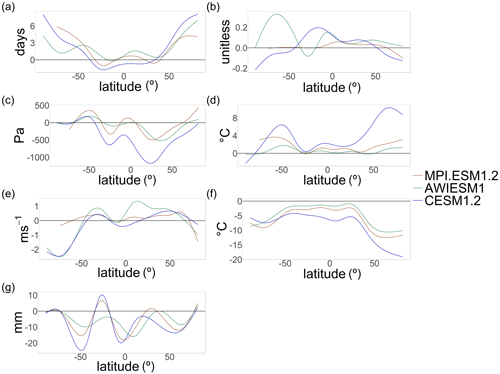
Figure 2Latitudinal distribution of the LGM-MOD climate anomalies for MPI.ESM1.2 (orange), AWI-ESM1.2 (pink), and CESM1.2 (brown) for (a) the maximum number of dry days, (b) the seasonality of dry days, (c) maximum monthly VPD, (d) maximum monthly DTR, (e) maximum monthly mean wind speeds, (f) mean monthly temperature, and (g) mean monthly total precipitation. The zero-intercept line represents no change between LGM and MOD climate, with negative values representing lower values at the LGM and positive values representing higher values at the LGM.
LGM climate data were obtained from three models participating in the Palaeoclimate Modelling Intercomparison Project (PMIP) contribution to the sixth phase of the Coupled Model Intercomparison Project (CMIP6), namely AWI-ESM-1-1-LR (short name: AWIESM1) (Lohmann et al., 2020; Sidorenko et al., 2015), MPI_ESM1.2 (Mauritsen et al., 2019), and CESM1.2 (Li et al., 2013; Tierney et al., 2020), to represent a range of LGM climates (Fig. 2). A seasonal climatology was derived for each climate variable from the PMIP picontrol experiment (pre-industrial conditions, PI) and the PMIP lgm experiment of the PMIP4 and CMIP6 simulations. The difference between the PI and LGM values (LGM-PI climate anomalies) were calculated and added to the MOD climatology (LGM-MOD climate anomalies) (see Fig. 1). We use the term climate anomalies to refer to the difference between the MOD climatology for each climate variable and the computed bias-adjusted LGM climatology for the same variable, consistently with the PMIP4 protocol (Kageyama et al., 2021). The use of anomalies is designed to minimize the impact of systematic model biases on the derived climate. This approach provided three LGM climate scenarios, resulting in 12 experiments for BA, FS, and FI.
We obtained MOD and LGM vegetation and gross primary production (GPP) using the coupled biogeography and biogeochemistry model BIOME4 (Kaplan et al., 2003) and a simple optimality based model of GPP, the P Model (Wang et al., 2017; Stocker et al., 2020). BIOME4 was used to simulate biome distribution with modern-day climate data (T, P, cld), setting CO2 levels to 395 ppm (the annual mean from 2010–2015) and 185 ppm in turn. LGM biome distributions were simulated using the three different LGM scenarios, again setting CO2 levels to 395 and 185 ppm. We derived mean fractional tree, shrub, and grass cover for each of these 12 experiments using the mean values for each biome from ESA CCI (Climate Change Initiative) Land Cover (Li et al., 2018). We also calculated fAPAR (fraction of absorbed photosynthetically active radiation) for each experiment from the leaf area index (LAI) computed by BIOME4 and obtained the fractional cover of C4 plants (see Sect. S1 in the Supplement). We computed global monthly C3 and C4 photosynthesis using the P model using appropriate combinations of climate (T, VPD, ppfd, and Pa), BIOME4-derived fAPAR, and CO2 concentration for the MOD and LGM scenarios (see Fig. 1). Total GPP was calculated as follows:
with and representing monthly C3 and C4 GPP values from the P model and representing the fractional C4 cover from BIOME4 (see Table 1).
This approach led to estimates of total BA, median FS, and median FI under modern conditions of similar magnitudes to the original GLM models and other global estimates (Andela et al., 2019; Humber et al., 2019) (Table 2).
Topographic and lightning variables were assumed not to change between the LGM and the present day. We used modern values, extrapolated out onto the exposed shelves, for the LGM experiments. The GLMs (Haas et al., 2022) include predictors associated with human activity, specifically human population density, road density, and cropland cover. Population density is used as a measure of potential human ignitions, and road density and cropland cover are used as measures of landscape fragmentation. Including these anthropogenic predictors in the GLM models was found to be essential to capture the global drivers of the observed spatial patterns of wildfires (Haas et al., 2022). This is because modern fire regimes are influenced by human activity at a global scale (e.g. Marlon et al., 2008; Bowman et al., 2020; Harrison et al., 2021). However, although the practice of foraging for plants by some hunter-gatherer communities at the LGM has been shown (Liu et al., 2013), we presume that there was no large-scale agriculture (or road networks) at the LGM. Additionally, information about pre-agricultural population sizes is limited and highly uncertain (see e.g. A. N. Williams et al., 2013; Gautney and Holliday, 2015), and though some regional models of human population do exist (Tallavaara et al., 2015), a reliable global product is not yet available. To avoid confounding effects due to the high uncertainty of human impacts on global wildfire regimes, we decided to exclude these anthropogenic predictors in all the experiments by setting them to 0. This ensured that differences between the experiments were driven solely by climate and CO2. We performed sensitivity analysis to examine the impact of setting human predictors to 0 under modern and LGM conditions (see Sect. S2). Whilst BA and FS increase in the modern sensitivity analyses (especially in areas with high road density and cropland density such as Europe and India), the effect was negligible for FI, highlighting the sensitivity of BA and FS to human activity. Under LGM conditions, the effect of including human population was negligible for all three fire properties. This reflects the slight and localized human impact on the natural landscape at the LGM (Black et al., 2007; Fuller et al., 2014; Portenga et al., 2016).
When modelled GPP values were 0, BA, FS, and FI were automatically set to 0. Modelled BA values smaller than 0.001 were assumed to imply no burning; thus, under these conditions, FS and FI were also assumed to be 0 since both GLM models were trained on data of existing fires (see Sect. S3).
The resulting BA, FS, and FI anomalies refer to the difference between the MOD climate/MOD CO2 experiment and the three other experiments since each experiment is considered to represent the long-term average spatial pattern for each fire property under the set experimental conditions. We used the sensitivity experiments to quantify the separate effects of CO2 and climate on BA, FS, and FI independently. We then used the realistic experiments to identify which predictors were driving the largest change between MOD and the three LGM scenarios by excluding one predictor at a time from the GLM models, re-running the LGM experiments, and identifying which excluded variable caused the greatest change in the BA, FS, and FI MOD-LGM anomalies in each grid cell. Comparing these results to the BA, FS, and FI MOD-LGM anomalies of the full GLM models allowed us to determine whether the predictor was responsible for an increase or a decrease in BA, FS, and FI.
We also compared the spatial patterns of BA, FS, and FI with sedimentary charcoal data from the Reading Palaeofire Database (RPD; Harrison et al., 2022). Sedimentary charcoal records provide a record of fire activity but may reflect changes in both burnt area or completeness of combustion (Power et al., 2008), so this comparison allowed us firstly to establish which of the fire regime properties was most closely reflected in these records and secondly which of the scenarios produced the most realistic patterns of burning. Model outputs and the charcoal records were re-gridded to the coarsest resolution of the three climate models (2.5∘ × 1.875∘ resolution). We calculated the number of correctly predicted BA, FS, or FI anomalies (same sign within a given grid cell), separating positive and negative BA, FS, or FI anomalies to assess the rate of false positives and false negatives for each scenario and each LGM climate scenario.
Global BA was substantially reduced compared to the realistic MOD scenario under all three realistic LGM scenarios, decreasing by 72 % for the coldest CESM1.2 LGM scenario, 62 % for the MPI-ESM1.2 LGM scenario, and 41 % for the warmest AWIESM1 LGM scenario (see Fig. 3). The largest decreases were observed in sub-Saharan Africa (excluding the tropical regions), as well as in northern Australia and the Indian subcontinent (MPI-ESM1.2 and CESM1.2 LGM scenarios). Some increases in BA were observed in Alaska (MPI-ESM1.2 and AWIESM1 LGM scenarios), as well as in southeast Asia, Indonesia, Papua New Guinea, and the northern tip of Australia. Increases in Somalia and Central America were also observed (MPI-ESM1.2 and AWIESM1 LGM scenarios). The number of grid cells (excluding ice-covered cells) in which no burning occurred was 3 times higher in the MPI-ESM1.2 and AWIESM1 LGM scenarios and 4 times higher in the CESM1.2 LGM scenario compared to the realistic MOD scenario. This was driven by the expansion of desert and tundra biomes at the LGM. The Arabian plate, the Middle East, inland China, and Australia, as well as the tips of South America and Africa, saw burning reduced to 0. Nearly all burning above 60∘ N was excluded, except for that in Alaska under the MPI-ESM1.2 and AWIESM1 LGM scenarios, with the exclusion extending down to 50∘ N for the CESM1.2 LGM scenario (see Sect. S3).
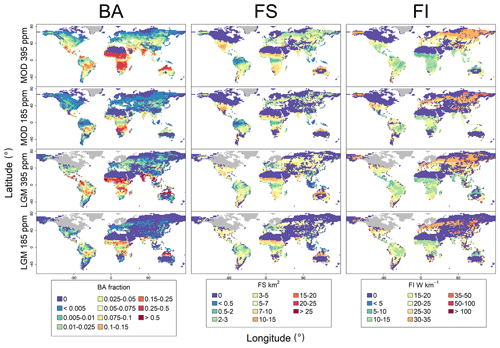
Figure 3Experiments for BA, FS, and FI for MPI-ESM1.2 LGM scenario (MOD 395 ppm and LGM 185 ppm represent the realistic modern-day simulation and LGM simulation, whilst MOD 185 ppm and LGM 395 ppm represent the CO2 and climate sensitivity experiments respectively. The ice is shown in grey). The other experiments can be found in Sect. S3.
Globally, there was a large decrease in global median FS and FI when considering all grid cells (not covered in ice) because of the overall global reduction in burning (see Fig. 3). Under all three LGM scenarios, global median FS and FI were reduced to 0 compared to ∼ 5 km2 for FS and 40 W km−1 for FI. However, when excluding grid cells in which no burning occurred, global median FS increased compared to the realistic MOD scenario (by ∼ 16 % under the two less conservative scenarios (MPI-ESM1.2 and AWIESM1) and by 12 % under the CESM1.2 LGM scenario). The main increases in FS occurred in Central America, Amazonia, tropical Africa, the Indian subcontinent, Europe, and Asia between 30 and 60∘ N (except for CESM1.2, which had very few positive FS anomalies). The largest reductions were observed in North America, southern Australia, the Middle East, and the rest of Eurasia. Global median FI also increased in regions that were burning under two of the LGM scenarios – by 11 % under the CESM1.2 LGM scenario and by 4 % under the MPI.ESM1.2 LGM scenario. Under the AWIESM1 LGM scenario, global median FI decreased by 2 % even when excluding grid cells that were not burning. Despite this, changes in FI were spatially consistent across all three LGM scenarios, with increases in FI occurring primarily across the American and African continents, as well as in the Mediterranean Basin and Europe, and with decreases occurring in Asia and inland Australia.
Under low CO2 levels with MOD climate (MOD climate/LGM CO2), global BA decreased by ∼ 70 % under all three LGM scenarios (72 % for CESM1.2 and AWIESM1, 73 % for MPI-ESM1.2). Despite larger global decreased BA compared to the realistic LGM scenarios, the number of grid cells in which no burning occurred was only 1.7 times higher for the MPI-ESM1.2 and AWIESM1 LGM scenarios and 1.5 times higher for the CESM1.2 LGM scenario compared to the realistic MOD scenario. The spatial pattern was consistent across all three LGM scenarios, with very few grid points showing a positive BA anomaly relative to the MOD experiment. Though FS increased slightly under this sensitivity experiment when burning did occur, this increase was concentrated in the tropical regions of South America and Africa (mainly Amazonia), except in the case of AWIESM1, where increases were observed across Eurasia. In burning grid cells, global median FI increased by ∼ 15 %–18 % in this sensitivity experiment (18 % for MPI-ESM1.2 and CESM1.2 and 15 % for AWIESM1). This spatial pattern was also consistent with BA, with very few negative FI anomalies, except for regions ∼ 20–30∘ N and ∼ 20–30∘ S.
Under MOD CO2 and LGM climate, BA decreased by 41 % compared to the MOD experiment for the CESM1.2 LGM scenario and by 4 % for the MPI-ESM1.2 LGM scenario, but it increased by 48 % for the AWIESM1 LGM scenario, showing a strong sensitivity to climate. The number of grid cells in which no burning occurred was of similar amplitude to the previous sensitivity experiment for the MPI-ESM1.2 and AWIESM1 LGM scenarios (∼ 1.8 times higher compared to the realistic MOD scenario) but was much higher for the CESM1.2 LGM scenario (∼ 3.5 increase). When burning occurred, the global median FS increased under all LGM scenarios by 17 % for CESM1.2, 25 % for MPI-ESM1.2, and 23 % for AWIESM1. These increases were concentrated in tropical Africa, central America, and Russia, with decreases shown in North America and South Africa. Global median FI also increased under this sensitivity experiment by 2 %–3 % for AWIESM1 and MPI-ESM1.2 but decreased by 5 % for the CESM1.2 LGM scenario, with decreases concentrated in Eurasia and North America.
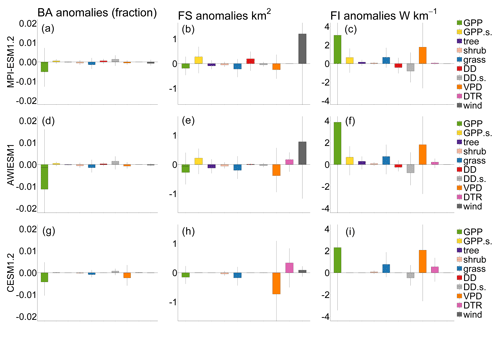
Figure 4Boxplots showing relative importance of each predictor (GPP – gross primary production; GPP.s. – GPP seasonality; tree – tree cover; shrub – shrub cover; grass – grass cover; DDs – dry days; DD.s. – dry-day seasonality; VPD – vapour pressure deficit; DTR – diurnal temperature range; wind – wind speed) in driving the BA, FS, or FI anomaly between the MOD 395 ppm and LGM 185 ppm experiment. For each grid cell common to both experiments (on modern-day continental shelves and masking the LGM ice sheets), the predictor which caused the largest change in the anomaly between the two experiments when it was excluded from the GLM model was retained; it is the change in anomaly that is shown here. This was taken as an indicator of the relative importance of that predictor in driving the observed change for (d–f) the AWIESM1 LGM scenario, (a–c) the MPI-ESM-1.2 LGM scenario, and (g–i) the CESM1.2 LGM scenario. A positive anomaly indicates that the variable caused an increase in BA, FS, or FI at the LGM, and a negative anomaly indicates that the variable caused a decrease in BA, FS, or FI at the LGM.
Reductions in BA between the MOD and LGM scenarios were driven primarily by changes in GPP; grass cover; VPD; and, to a lesser extent, dryness (dry days (DDs) and dry-day seasonality (DD.s)). Changes in FI were driven by changes in GPP and VPD, with changes in GPP seasonality also leading to increased FI in inland regions, reflecting both changes in climate and CO2 levels for BA and FI. Increased FS was largely driven by increased wind speeds; DDs and diurnal temperature range (DTR), reflecting a strong climate effect; and GPP seasonality. Decreases in FS were driven by changes in GPP and grass cover, as well as by VPD under the CESM1.2 LGM scenario and DTR under the AWIESM1 LGM scenario (Fig. 4). Changes in GPP and grass cover were responsible for the largest reductions in burning, with these vegetation effects being concentrated across Africa and much of Eurasia (see Fig. 5). In Amazonia, changes in DDs were the most important factor, reducing BA and FS (except for MPI-ESM1.2, which saw increased FS driven by DDs). Increased BA in western Alaska was driven by GPP in the MPI-ESM-1.2 and AWIESM1 LGM scenarios. Increased BA in tropical regions was driven by grass cover, GPP, and DD changes. Changes in VPD across the northern latitudes, especially of North America and Europe, led to decreased BA in the most conservative CESM1.2 LGM scenario. FS decreased across the Americas and Eurasia in the CESM1.2 LGM scenario because of low VPD values, which reduced the occurrence of burning and offset the increases caused by wind speed and DTR in the other two LGM scenarios. Low values of VPD drove increases in FI across eastern North America, South America, western Africa, and southeast Asia.
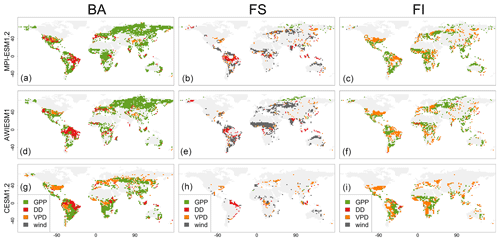
Figure 5Map showing selection of four variables (GPP in green, DDs in red, VPD in orange, and wind in grey) responsible for some of the most important grid cell drivers in reducing BA and increasing FS and FI for (d–f) the AWIESM1 LGM scenario, (a–c) the MPI-ESM1.2 LGM scenario, and (g–i) the CESM1.2 LGM scenario. Maps of most important grid cell drivers for all variables and all experiments can be found in Sect. S3.
Comparing the spatial patterns of the simulated BA anomalies with charcoal-based reconstructions of the sign of changes in biomass burning (RPD; Harrison et al., 2022) showed that the best overall match occurred when both the climate and CO2 effect were considered, with a success rate of ∼ 39 %–45 % depending on the climate scenario. The MPI-ESM1.2 and AWIESM1 LGM scenarios produced the best overall matches. None of the MOD climate/LGM CO2 experiments identified any of the positive BA anomalies shown by the charcoal records. The LGM climate/MOD CO2 experiments identified around half (∼ 10 %–17 %) of the negative BA anomalies identified by the realistic experiment (17 %–20 %) and the MOD climate/LGM CO2 sensitivity experiment and only performed marginally better than the realistic experiment in identifying the positive BA anomalies (Table 3). Thus, although this sensitivity experiment produced a similar overall agreement with the reconstructions as the LGM climate/LGM CO2 simulations, only the realistic scenarios produced similar success rates for both the negative and positive BA anomalies. Climate change alone produced too few negative anomalies matches; CO2 changes alone resulted in no positive anomaly matches.

Figure 6Comparison of BA anomalies between the experiment outputs from the MPI-ESM1.2 LGM scenario with charcoal records from the Reading Palaeofire Database (RPD) for (a) the realistic LGM experiment (b) the LGM climate/MOD CO2 sensitivity experiment and (c) the MOD climate/LGM CO2 sensitivity experiment The modelled positive LGM-MOD anomalies are shown in red and LGM-MOD negative anomalies in blue. Dotted red (positive anomaly) and blue (negative anomaly) points show the location of the RPD records for the LGM. The LGM ice sheets are shown in dark blue.
Table 2Comparison of sign in BA anomalies (between the MOD climate/MOD CO2 experiment and other three experiments respectively) at the location of each RDP (Reading Paleofire Database) charcoal-based reconstruction record. A positive anomaly represents increased biomass burning, and a negative anomaly represents decreased biomass burning. A successful identification means that the sign of the experiment anomaly and the sign of the RPD charcoal-based reconstructions are the same.
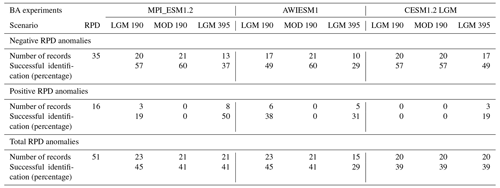
The sign of the charcoal records could reflect changes in FS or FI, as well as in BA. However, the success rates in predicting the sign of the charcoal anomalies (both positive and negative) were not as good for FS (27 %–31%) and FI (24 %–30 %) as those obtained for BA for the realistic LGM experiment. Furthermore, both FS and FI did not perform any better than BA under any experiment, with the sensitivity experiments matching the charcoal anomalies slightly better for FS and FI than under the realistic LGM experiment (see Sect. S4).
Our simulations show a global reduction in burning at the LGM but increased median fire size and intensity when burning did occur. BA, FS, and FI were all sensitive to changes in vegetation driven directly by CO2 levels alone. BA and FI were most sensitive to this effect, with the climate effect dampening the effect of CO2 alone when both are included. The largest reductions in burning occurred when only the CO2 effect was considered, although this experiment had fewer regions in which burning was excluded completely. This suggests that the reduction in burning was more spatially consistent and widespread under these conditions than when both effects were accounted for. The sensitivity of BA to CO2 is explained by the reduction in fuel availability under low-CO2 conditions, a strong constraint on burnt area. For FI, including a CO2 effect also amplified the overall global signal. This CO2 effect is most likely driven by the negative relationship between GPP and FI fitted by the empirical model. Whilst this relationship might seem counter-intuitive, it has a sound basis. The most intense fires occur in regions with a seasonal variation in productivity rather than in the most productive environments such as tropical forests (Archibald et al., 2013). High productivity can (under some climate conditions) increase the frequency of burning, which also reduces fuel loads (Rodrigues et al., 2019). Under appropriate climate conditions, there can be long-term fuel build-up in areas of low productivity that is not offset by frequent burning. All these factors help to explain why FI is not reduced at the LGM when burning occurs even though BA is. Low CO2 decreased FS, except in tropical regions, and reduced the impact of climate in the realistic scenarios. We hypothesize that this is because of decreased productivity leading to patchier vegetation and hence reduced fuel continuity, which is a factor limiting wildfire spread (Dial et al., 2022; Schertzer et al., 2015).
Changes in climate alone also affected all three modelled wildfire properties. The climate effect was larger than the CO2 effect across all models for FS, with increases in wind, DD, and DTR driving the change. BA was particularly sensitive to the amplitude of climate change: climate change alone greatly reduced BA under the coldest LGM scenario (CESM1.2), had a limited effect in the intermediate LGM scenario (MPI-ESM1.2), and increased BA in the warmest LGM scenario (AWIESM1). The amplitude of change in VPD, a measure of atmospheric moisture, relative to other climate variables was especially important in influencing overall trends. In the case of BA, large decreases in VPD under the CESM1.2 climate scenario led to much more substantial reductions, most likely due to an increase in fuel moisture. Additionally, though stronger winds and increased DTR were the main drivers of larger wildfires at the LGM, low VPD values in CESM1.2 severely limited FS and FI in the northern latitudes. VPD has been shown to influence wildfire ignition and wildfire spread (Sedano and Randerson, 2014), and our results suggest that high atmospheric moisture can inhibit fire spread. When vegetation was sufficiently abundant, however, low VPD values were key in driving intensity. Although vegetation productivity was lower at the LGM, decreased VPD may have contributed to larger fuel build-ups, thus increasing fuel loads. This highlights the sensitivity of the fire regime not just to overall climate change but to the relative amplitude of change in individual climate variables.
Our model results reproduce the global reduction of biomass burning at the LGM observed from ice cores and sedimentary charcoal records (Daniau et al., 2012; Harrison et al., 2022; Power et al., 2008; Rubino et al., 2015). Some studies have indicated the occurrence of high-intensity wildfires on the Palaeo-Agulhas Plain of South Africa and in tropical regions, northern Australia, and central China at the LGM (Kraaij et al., 2020; Power et al., 2008; Rowe et al., 2021; Ruan et al., 2020; Song et al., 2023). Our results are consistent with the trends in these regions. The LGM simulations of BA that account for both climate and CO2 appear to fit the charcoal records best. The spatial patterns of BA at the LGM were more consistent with the patterns shown by sedimentary charcoal records than FS and FI, consistent with the assumption that charcoal abundance can be used as a measure of biomass burning. The FS and FI anomaly patterns were less consistent than that of BA, suggesting that a regime of less burning but larger and more intense wildfires at the LGM could be consistent with the charcoal records. Whilst FI has been reconstructed from charcoal (e.g. Duffin, 2008; Snitker, 2018), there are currently no comparable measures that record FS or FI changes globally. Charcoal records are not available from some regions, further limiting our ability to evaluate the models, particularly in Eurasia and inland South America, where low CO2 leads to large reductions in BA that are not observed when only climate is considered.
Our results are based on simple empirical models for BA, FS, and FI. However, the inferred changes in BA are like those of Martin Calvo et al. (2014), who used the Land surface Processes and eXchanges (LPX) dynamic global vegetation model. Empirical models have been shown to perform as well as more complex process-based models in simulating burned area under modern-day conditions (Hantson et al., 2020). Thus, our conclusions about the relative impact of climate and CO2 changes on fire properties are unlikely to be adversely affected by the relative simplicity of the models used. Their simplicity facilitates running multiple scenarios and diagnosis of the factors influencing changes in wildfire properties.
The effect of human activity was not considered in this analysis, and, as such, no conclusions can be drawn on how human activity may affect these trends. Although this is a limitation, we believe it is unlikely that human activity would substantially impact the response of wildfire regimes to the changes in climate and CO2 observed here. Pre-agricultural hunter-gatherer populations used fire for land management, for example to facilitate hunting and to promote the local abundance of food plants (Bowman, 1998; Gott, 2005), although recent work indicates that the burning regimes they practised tended to reduce fire overall compared to the natural state (see e.g. Constantine et al., 2023). However, the areas suitable for hunter-gatherer populations was much reduced at the LGM by generally colder and drier climates, and hunter-gatherer populations were confined to climatically suitable refugia (see e.g. A. N. Williams et al., 2013; Blinkhorn et al., 2022). Furthermore, although the estimates of population density are highly uncertain, the LGM population of Australia was less than 5 % of the modern population, and the reduction in Africa was even larger (Gautney and Holliday, 2015). Palaeoecological evidence from Australia suggests that the use of fire by pre-agricultural hunter-gatherers had a low impact on the environment before the late Holocene (e.g. Black et al., 2007; Fuller et al., 2014; Portenga et al., 2016). Thus, it is unlikely that human activities during the LGM would have substantially increased fire or offset the impact of the changes in climate and CO2 on fire regimes. Previous studies show a weak influence of population and land use change in driving global wildfire trends prior to the 18th century (e.g. Pechony and Shindell, 2010; Bowman et al., 2020) and a sharp human-driven decline in wildfire activity since the mid-nineteenth century (e.g. Marlon et al., 2008; Wang et al., 2010). This recent reduction in global biomass burning was most likely driven by population growth and land use change leading to increased landscape fragmentation, which tends to suppress fire spread (e.g. Knorr et al., 2014; Andela et al., 2017; Harrison et al., 2021).
These results add to a growing body of literature highlighting the importance of considering not only changes in wildfire weather but also vegetation properties in projections of future wildfire regimes (e.g. Harrison et al., 2021; Kuhn-Régnier et al., 2021; Pausas and Keeley, 2021). The impact of rising CO2 levels will most likely enhance vegetation growth and litter accumulation, which are important controls on fuel availability, continuity, and load. However, climate and specifically VPD may have opposing effects to that of rising CO2 levels. Since VPD controls plant growth, increasing VPD can limit ecosystem productivity and tree growth, in turn reducing fuel loads (A. P. Williams et al., 2013). Nevertheless, VPD has also been shown to increase litter fall, thus increasing available dead fuel (De Dios et al., 2021; De Faria et al., 2017). As such, it is important to consider how temporal and spatial scales affect the response of vegetation to changing VPD (Grossiord et al., 2020). Although the trade-offs between future increases in CO2 and reductions in productivity due to higher temperatures and atmospheric dryness are not fully understood, this work highlights the importance of considering both. These effects will most likely not be evenly distributed across the globe (Gonsamo et al., 2021; Piao et al., 2020; van der Sleen et al., 2015), and CO2 effects may be more important in some regions than others. In fuel-limited ecosystems, CO2 fertilization could increase fuel loads and fuel continuity, increasing overall burnt area but also the potential for larger and more intense wildfires. This is particularly worrying in regions with anticipated decreases in atmospheric moisture, especially since evidence suggests rising VPD may only counteract a small proportion of CO2-induced plant growth (Song et al., 2022). Increased woody thickening, for example in tropical southern Asia (Kumar et al., 2021; Scheiter et al., 2020), may also alter fuel loads in regions that are likely to be vulnerable to ignition under a drier and warmer atmosphere (Clarke et al., 2022). Whilst climate variables such as DDs and DTR have also been shown to be strong controls of global wildfire regimes (e.g. Bistinas et al., 2014; Forkel et al., 2019; Kuhn-Régnier et al., 2021), this study highlights the importance of VPD relative to other climate variables in driving spatial patterns of BA, FS, and FI. This is in line with previous studies that have highlighted the important role of VPD in promoting fuel loads and fire spread (e.g. Diffenbaugh et al., 2021; Grillakis et al., 2022; Duane et al., 2021; Balch et al., 2022). Correctly projecting changes in fuels in the next century will require considering both the effect of VPD and the effects of CO2 on plant growth and fuel loads.
Our results stress the importance of accounting for the effects of CO2 on vegetation when considering how future fire regimes may evolve. Different aspects of the fire regime respond differently to changes in fuel properties. Without accounting for this crucial effect, our understanding of future risks will remain limited.
All code used in this paper is freely available for use in RStudio, the code for the GLM models is available at https://doi.org/10.6084/m9.figshare.19071044.v1 (Haas, 2023a), and the code to generate the experiments is available at https://doi.org/10.6084/m9.figshare.22285303.v2 (Haas, 2023b) and https://doi.org/10.6084/m9.figshare.22285279.v2 (Haas, 2023c).
All LGM data can be retrieved from https://esgf-node.llnl.gov/projects/cmip6/ (WCRP, 2023), and all modern data can be retrieved from references provided. The P Model documentation is available at https://doi.org/10.5281/zenodo.8366848 (Orme and Marion, 2023), and the BIOME4 documentation is available at https://pmip2.lsce.ipsl.fr/synth/biome4.shtml (last access: 26 September 2023) and https://doi.org/10.5281/zenodo.8368294 (Kaplan, 2023).
The supplement related to this article is available online at: https://doi.org/10.5194/bg-20-3981-2023-supplement.
Experiment conception, strategy, and interpretation were developed by OH, ICP, and SPH jointly. OH performed the data processing and analysis and produced the graphics and tables. OH wrote the original draft; SPH and ICP contributed to the final draft.
The contact author has declared that none of the authors has any competing interests.
Publisher's note: Copernicus Publications remains neutral with regard to jurisdictional claims in published maps and institutional affiliations.
Olivia Haas acknowledges support from the NERC Centre for Doctoral Training in Quantitative and Modelling skills in Ecology and Evolution (grant no. NE/S007415/1) and from the Leverhulme Trust through the Leverhulme Centre for Wildfires, Environment and Society (grant no. RC-2018-023). Special thanks goes to David Orme for his help with setting up BIOME4. Iain Colin Prentice acknowledges support from the European Research Council (grant no. 787203 REALM) under the European Union's Horizon 2020 research programme. Sandy P. Harrison is supported by the European Research Council (grant no. 694481 GC2.0) under the same programme. This work is a contribution to the LEMONTREE (Land Ecosystem Models based On New Theory, obseRvations and ExperimEnts) project, funded through the generosity of Eric and Wendy Schmidt by recommendation of the Schmidt Futures programme.
This research has been supported by the Natural Environment Research Council (grant no. NE/S007415/1); the Leverhulme Trust (grant no. RC-2018-023); and the H2020 Excellent Science, H2020 European Research Council (grant nos. 787203 and 694481).
This paper was edited by Petr Kuneš and reviewed by Jessica Hetzer and one anonymous referee.
Abatzoglou, J. T., Williams, A. P., and Barbero, R.: Global emergence of anthropogenic climate change in fire weather indices, Geophys. Res. Lett., 46, 326–336, https://doi.org/10.1029/2018GL080959, 2019.
Albani, S., Balkanski, Y., Mahowald, N., Winckler, G., Maggi, V., and Delmonte, B.: Aerosol-climate interactions during the Last Glacial Maximum, Current Climate Change Reports, 4, 99–114, https://doi.org/10.1007/s40641-018-0100-7, 2018.
Andela, N., Morton, D. C., Giglio, L., Chen, Y., van der Werf, G. R., Kasibhatla, P. S., DeFries, R. S., Collatz, G. J., Hantson, S., Kloster, S., and Bachelet, D.: A human-driven decline in global burned area, Science, 356, 1356–1362, https://doi.org/10.1126/science.aal4108, 2017.
Andela, N., Morton, D. C., Giglio, L., Paugam, R., Chen, Y., Hantson, S., van der Werf, G. R., and Randerson, J. T.: The Global Fire Atlas of individual fire size, duration, speed and direction, Earth Syst. Sci. Data, 11, 529–552, https://doi.org/10.5194/essd-11-529-2019, 2019.
Archibald, S., Lehmann, C. E. R., Gómez-Dans, J. L., and Bradstock, R. A.: Defining pyromes and global syndromes of fire regimes, P. Natl. Acad. Sci. USA, 110, 6442–6447, https://doi.org/10.1073/pnas.1211466110, 2013.
Balch, J. K., Abatzoglou, J. T., Joseph, M. B., Koontz, M. J., Mahood, A. L., McGlinchy, J., Cattau, M. E., and Williams, A. P.: Warming weakens the night-time barrier to global fire, Nature, 602, 442–448, https://doi.org/10.1038/s41586-021-04325-1, 2022.
Betts, R. A., Golding, N., Gonzalez, P., Gornall, J., Kahana, R., Kay, G., Mitchell, L., and Wiltshire, A.: Climate and land use change impacts on global terrestrial ecosystems and river flows in the HadGEM2-ES Earth system model using the representative concentration pathways, Biogeosciences, 12, 1317–1338, https://doi.org/10.5194/bg-12-1317-2015, 2015.
Bistinas, I., Harrison, S. P., Prentice, I. C., and Pereira, J. M. C.: Causal relationships versus emergent patterns in the global controls of fire frequency, Biogeosciences, 11, 5087–5101, https://doi.org/10.5194/bg-11-5087-2014, 2014.
Black, M. P., Mooney, S. D., and Haberle, S. G.: The fire, human and climate nexus in the Sydney Basin, eastern Australia, Holocene, 17, 469–480, https://doi.org/10.1177/0959683607077024, 2007.
Blinkhorn, J., Timbrell, L., Grove, M., and Scerri, E. M. L.: Evaluating refugia in recent human evolution in Africa, Philos. T. R. Soc. B, 377, 20200485, https://doi.org/10.1098/rstb.2020.0485, 2022.
Bond, W. J. and Midgley, G. F.: Carbon dioxide and the uneasy interactions of trees and savannah grasses, Philos. T. R. Soc. B, 367, 601–612, https://doi.org/10.1098/rstb.2011.0182, 2012.
Bond, W. J., Midgley, G. F., and Woodward, F. I.: The importance of low atmospheric CO2 and fire in promoting the spread of grasslands and savannas, Glob. Change Biol., 9, 973–998, https://doi.org/10.1046/j.1365-2486.2003.00577.x, 2003.
Bowman, D. M. J. S.: The impact of Aboriginal landscape burning on the Australian biota, New Phytol., 140, 385–410, https://doi.org/10.1046/j.1469-8137.1998.00289.x, 1998.
Bowman, D. M. J. S., Kolden, C. A., Abatzoglou, J. T., Johnston, F. H., van der Werf, G. R., and Flannigan, M.: Vegetation fires in the Anthropocene, Nature Reviews Earth and Environment, 1, 500–515, https://doi.org/10.1038/s43017-020-0085-3, 2020.
Bradstock, R. A.: A biogeographic model of fire regimes in Australia: current and future implications, Global Ecol. Biogeogr., 19, 145–158, https://doi.org/10.1111/j.1466-8238.2009.00512.x, 2010.
Bragg, F. J., Prentice, I. C., Harrison, S. P., Eglinton, G., Foster, P. N., Rommerskirchen, F., and Rullkötter, J.: Stable isotope and modelling evidence for CO2 as a driver of glacial–interglacial vegetation shifts in southern Africa, Biogeosciences, 10, 2001–2010, https://doi.org/10.5194/bg-10-2001-2013, 2013.
Buitenwerf, R., Bond, W. J., Stevens, N., and Trollope, W. S. W.: Increased tree densities in South African savannas: >50 years of data suggests CO2 as a driver, Glob. Change Biol., 18, 675–684, https://doi.org/10.1111/j.1365-2486.2011.02561.x, 2012.
Clarke, H., Nolan, R. H., de Dios, V. R., Bradstock, R., Griebel, A., Khanal, S., and Boer, M. M.: Forest fire threatens global carbon sinks and population centres under rising atmospheric water demand, Nat. Commun., 13, 7161, https://doi.org/10.1038/s41467-022-34966-3, 2022.
Constantine IV, M., Williams, A. N., Francke, A., Cadd, H., Forbes, M., Cohen, T. J., Zhu, X., and Mooney, S. D.: Exploration of the burning question: a long history of fire in eastern Australia with and without people, Fire, 6, 152, https://doi.org/10.3390/fire6040152, 2023.
Cucchi, M., Weedon, G. P., Amici, A., Bellouin, N., Lange, S., Müller Schmied, H., Hersbach, H., and Buontempo, C.: WFDE5: bias-adjusted ERA5 reanalysis data for impact studies, Earth Syst. Sci. Data, 12, 2097–2120, https://doi.org/10.5194/essd-12-2097-2020, 2020.
Daniau, A.-L., Bartlein, P. J., Harrison, S. P., Prentice, I. C., Brewer, S., Friedlingstein, P., Harrison-Prentice, T. I., Inoue, J., Marlon, J. R., Mooney, S., Power, M. J., Stevenson, J., Tinner, W., Andrič, M., Atanassova, J., Behling, H., Black, M., Blarquez, O., Brown, K. J., Carcaillet, C., Colhoun, E., Colombaroli, D., Davis, B. A. S., D'Costa, D., Dodson, J., Dupont, L., Eshetu, Z., Gavin, D. G., Genries, A., Gebru, T., Haberle, S., Hallett, D. J., Horn, S., Hope, G., Katamura, F., Kennedy, L., Kershaw, P., Krivonogov, S., Long, C., Magri, D., Marinova, E., McKenzie, G. M., Moreno, P. I., Moss, P., Neumann, F. H., Norström, E., Paitre, C., Rius, D., Roberts, N., Robinson, G., Sasaki, N., Scott, L., Takahara, H., Terwilliger, V., Thevenon, F., Turner, R. B., Valsecchi, V. G., Vannière, B., Walsh, M., Williams, N., and Zhang, Y.: Predictability of biomass burning in response to climate changes, Global Biogeochem. Cy., 26, GB4007, https://doi.org/10.1029/2011GB004249, 2012.
De Dios, V. R., Hedo, J., Camprubí, À. C., Thapa, P., Del Castillo, E. M., de Aragón, J. M., Bonet, J. A., Balaguer-Romano, R., Díaz-Sierra, R., Yebra, M., and Boer, M. M.: Climate change induced declines in fuel moisture may turn currently fire-free Pyrenean mountain forests into fire-prone ecosystems, Sci. Total Environ., 797, 149104, https://doi.org/10.1016/j.scitotenv.2021.149104, 2021.
De Faria, B. L., Brando, P. M., Macedo, M. N., Panday, P. K., Soares-Filho, B. S., and Coe, M. T.: Current and future patterns of fire-induced forest degradation in Amazonia, Environ. Res. Lett., 12, 095005, https://doi.org/10.1088/1748-9326/aa69ce, 2017.
Dial, R. J., Maher, C. T., Hewitt, R. E., and Sullivan, P. F.: Sufficient conditions for rapid range expansion of a boreal conifer, Nature, 608, 546–551, https://doi.org/10.1038/s41586-022-05093-2, 2022.
Diffenbaugh, N. S., Konings, A. G., and Field, C. B.: Atmospheric variability contributes to increasing wildfire weather but not as much as global warming, P. Natl. Acad. Sci. USA, 118, e2117876118, https://doi.org/10.1073/pnas.2117876118, 2021.
Donohue, R. J., Roderick, M. L., McVicar, T. R., and Farquhar, G. D.: Impact of CO2 fertilization on maximum foliage cover across the globe's warm, arid environments, Geophys. Res. Lett., 40, 3031–3035. https://doi.org/10.1002/grl.50563, 2013.
Duane, A., Castellnou, M., and Brotons, L.: Towards a comprehensive look at global drivers of novel extreme wildfire events, Climatic Change, 165, 43, https://doi.org/10.1007/s10584-021-03066-4, 2021.
Duffin, K. I.: The representation of rainfall and fire intensity in fossil pollen and charcoal records from a South African savanna, Rev. Palaeobot. Palyno., 151, 59–71, https://doi.org/10.1016/j.revpalbo.2008.02.004, 2008.
Flannigan, M., Cantin, A. S., De Groot, W. J., Wotton, M., Newbery, A., and Gowman, L. M.: Global wildland fire season severity in the 21st century, Forest Ecol. Manage., 294, 54–61, https://doi.org/10.1016/j.foreco.2012.10.022, 2013.
Forkel, M., Andela, N., Harrison, S. P., Lasslop, G., van Marle, M., Chuvieco, E., Dorigo, W., Forrest, M., Hantson, S., Heil, A., Li, F., Melton, J., Sitch, S., Yue, C., and Arneth, A.: Emergent relationships with respect to burned area in global satellite observations and fire-enabled vegetation models, Biogeosciences, 16, 57–76, https://doi.org/10.5194/bg-16-57-2019, 2019.
Fuller, D. Q., Denham, T., Arroyo-Kalin, M., Lucas, L., Stevens, C. J., Qin, L., Allaby, R. G., and Purugganan, M. D.: Convergent evolution and parallelism in plant domestication revealed by an expanding archaeological record, P. Natl. Acad. Sci. USA, 111, 6147–6152, https://doi.org/10.1073/pnas.1308937110, 2014.
Gautney, J. R. and Holliday, T. W.: New estimations of habitable land area and human population size at the Last Glacial Maximum, J. Archaeol. Sci., 58, 103–112, https://doi.org/10.1016/j.jas.2015.03.028, 2015.
Gonsamo, A., Ciais, P., Miralles, D. G., Sitch, S., Dorigo, W., Lombardozzi, D., Friedlingstein, P., Nabel, J. E. M. S., Goll, D. S., O'Sullivan, M., Arneth, A., Anthoni, P., Jain, A. K., Wiltshire, A., Peylin, P., and Cescatti, A.: Greening drylands despite warming consistent with carbon dioxide fertilization effect, Glob. Change Biol., 27, 3336–3349, https://doi.org/10.1111/gcb.15658, 2021.
Gott, B.: Aboriginal fire management in south-eastern Australia: aims and frequency, J. Biogeogr., 32, 1203–1208, https://www.jstor.org/stable/3566388 (last access: July 2023), 2005.
Grillakis, M., Voulgarakis, A., Rovithakis, A., Seiradakis, K. D., Koutroulis, A., Field, R. D., Kasoar, M., Papadopoulos, A., and Lazaridis, M.: Climate drivers of global wildfire burned area, Environ. Res. Lett., 17, 045021, https://doi.org/10.1088/1748-9326/ac5fa1, 2022.
Grossiord, C., Buckley, T. N., Cernusak, L. A., Novick, K. A., Poulter, B., Siegwolf, R. T., Sperry, J. S., and McDowell, N. G.: Plant responses to rising vapor pressure deficit, New Phytol., 226, 1550–1566, https://doi.org/10.1111/nph.16485, 2020.
Haas, O.: Scripts and input files, figshare [data set], https://doi.org/10.6084/m9.figshare.19071044.v1, 2023a.
Haas, O.: Data for: The response of wildfire regimes to Last Glacial Maximum carbon dioxide and climate, figshare [data set], https://doi.org/10.6084/m9.figshare.22285303.v2, 2023b.
Haas, O.: R scripts to run models for: The response of wildfire regimes to Last Glacial Maximum carbon dioxide and climate, figshare [code], https://doi.org/10.6084/m9.figshare.22285279.v2, 2023c.
Haas, O., Prentice, I. C., and Harrison, S. P.: Global environmental controls on wildfire burnt area, size, and intensity, Environ. Res. Lett., 17, 065004, https://doi.org/10.1088/1748-9326/ac6a69, 2022.
Hantson, S., Kelley, D. I., Arneth, A., Harrison, S. P., Archibald, S., Bachelet, D., Forrest, M., Hickler, T., Lasslop, G., Li, F., Mangeon, S., Melton, J. R., Nieradzik, L., Rabin, S. S., Prentice, I. C., Sheehan, T., Sitch, S., Teckentrup, L., Voulgarakis, A., and Yue, C.: Quantitative assessment of fire and vegetation properties in simulations with fire-enabled vegetation models from the Fire Model Intercomparison Project, Geosci. Model Dev., 13, 3299–3318, https://doi.org/10.5194/gmd-13-3299-2020, 2020.
Harrison, S. P. and Prentice, C. I.: Climate and CO2 controls on global vegetation distribution at the last glacial maximum: analysis based on palaeovegetation data, biome modelling and palaeoclimate simulations, Glob. Change Biol., 9, 983–1004, https://doi.org/10.1046/j.1365-2486.2003.00640.x, 2003.
Harrison, S. P., Prentice, I. C., Bloomfield, K. J., Dong, N., Forkel, M., Forrest, M., Ningthoujam, R. K., Pellegrini, A., Shen, Y., and Baudena, M. Cardoso, A. W., Huss, J. C., Joshi J., Oliveras, I., Pausas, J. G., and Simpson, J. K.: Understanding and modelling wildfire regimes: an ecological perspective, Environ. Res. Lett., 16, 125008, https://doi.org/10.1088/1748-9326/ac39be, 2021.
Harrison, S. P., Villegas-Diaz, R., Cruz-Silva, E., Gallagher, D., Kesner, D., Lincoln, P., Shen, Y., Sweeney, L., Colombaroli, D., Ali, A., Barhoumi, C., Bergeron, Y., Blyakharchuk, T., Bobek, P., Bradshaw, R., Clear, J. L., Czerwiński, S., Daniau, A.-L., Dodson, J., Edwards, K. J., Edwards, M. E., Feurdean, A., Foster, D., Gajewski, K., Gałka, M., Garneau, M., Giesecke, T., Gil Romera, G., Girardin, M. P., Hoefer, D., Huang, K., Inoue, J., Jamrichová, E., Jasiunas, N., Jiang, W., Jiménez-Moreno, G., Karpińska-Kołaczek, M., Kołaczek, P., Kuosmanen, N., Lamentowicz, M., Lavoie, M., Li, F., Li, J., Lisitsyna, O., López-Sáez, J. A., Luelmo-Lautenschlaeger, R., Magnan, G., Magyari, E. K., Maksims, A., Marcisz, K., Marinova, E., Marlon, J., Mensing, S., Miroslaw-Grabowska, J., Oswald, W., Pérez-Díaz, S., Pérez-Obiol, R., Piilo, S., Poska, A., Qin, X., Remy, C. C., Richard, P. J. H., Salonen, S., Sasaki, N., Schneider, H., Shotyk, W., Stancikaite, M., Šteinberga, D., Stivrins, N., Takahara, H., Tan, Z., Trasune, L., Umbanhowar, C. E., Väliranta, M., Vassiljev, J., Xiao, X., Xu, Q., Xu, X., Zawisza, E., Zhao, Y., Zhou, Z., and Paillard, J.: The Reading Palaeofire Database: an expanded global resource to document changes in fire regimes from sedimentary charcoal records, Earth Syst. Sci. Data, 14, 1109–1124, https://doi.org/10.5194/essd-14-1109-2022, 2022.
Humber, M. L., Boschetti, L., Giglio, L., and Justice, C. O.: Spatial and temporal intercomparison of four global burned area products, Int. J. Digit. Earth, 12, 460–484, https://doi.org/10.1080/17538947.2018.1433727, 2019.
Jolly, W. M., Cochrane, M. A., Freeborn, P. H., Holden, Z. A., Brown, T. J., Williamson, G. J., and Bowman, D.: Climate-induced variations in global wildfire danger from 1979 to 2013, Nat. Commun., 6, 1–11, https://doi.org/10.1038/ncomms8537, 2015.
Kageyama, M., Harrison, S. P., Kapsch, M.-L., Lofverstrom, M., Lora, J. M., Mikolajewicz, U., Sherriff-Tadano, S., Vadsaria, T., Abe-Ouchi, A., Bouttes, N., Chandan, D., Gregoire, L. J., Ivanovic, R. F., Izumi, K., LeGrande, A. N., Lhardy, F., Lohmann, G., Morozova, P. A., Ohgaito, R., Paul, A., Peltier, W. R., Poulsen, C. J., Quiquet, A., Roche, D. M., Shi, X., Tierney, J. E., Valdes, P. J., Volodin, E., and Zhu, J.: The PMIP4 Last Glacial Maximum experiments: preliminary results and comparison with the PMIP3 simulations, Clim. Past, 17, 1065–1089, https://doi.org/10.5194/cp-17-1065-2021, 2021.
Kaplan, J. O.: jedokaplan/BIOME4: BIOME4 public release (1999) (v4.2.2), Zenodo [code], https://doi.org/10.5281/zenodo.8368294, 2023.
Kaplan, J. O., Bigelow, N. H., Prentice, I. C., Harrison, S. P., Bartlein, P. J., Christensen, T. R., Cramer, W., Matveyeva, N. V., McGuire, A. D., Murray, D. F., Razzhivin, V. Y., Smith, B., Walker, D. A., Anderson, P. M., Andreev, A. A., Brubaker, L. B., Edwards, M. E., and Lozhkin, A. V.: Climate change and Arctic ecosystems II: Modeling, palaeodata-model comparisons, and future projections, J. Geophys. Res.-Atmos., 108, 8171, https://doi.org/10.1029/2002JD002559, 2003.
Kaplan, J. O., Pfeiffer, M., Kolen, J. C. A., and Davis, B. A. S.: Large scale anthropogenic reduction of forest cover in Last Glacial Maximum Europe, PLoS One, 11, e0166726, https://doi.org/10.1371/journal.pone.0166726, 2016.
Kgope, B. S., Bond, W. J., and Midgley, G. F.: Growth responses of African savanna trees implicate atmospheric [CO2] as a driver of past and current changes in savanna tree cover, Austral Ecol., 35, 451–463, https://doi.org/10.1111/j.1442-9993.2009.02046.x, 2010.
Knorr, W., Kaminski, T., Arneth, A., and Weber, U.: Impact of human population density on fire frequency at the global scale, Biogeosciences, 11, 1085–1102, https://doi.org/10.5194/bg-11-1085-2014, 2014.
Knorr, W., Jiang, L., and Arneth, A.: Climate, CO2 and human population impacts on global wildfire emissions, Biogeosciences, 13, 267–282, https://doi.org/10.5194/bg-13-267-2016, 2016.
Kraaij, T., Engelbrecht, F., Franklin, J., and Cowling, R. M.: A fiery past: A comparison of glacial and contemporary fire regimes on the Palaeo-Agulhas Plain, Cape Floristic Region, Quaternary Sci. Rev., 235, 106059, https://doi.org/10.1016/j.quascirev.2019.106059, 2020.
Kuhn-Régnier, A., Voulgarakis, A., Nowack, P., Forkel, M., Prentice, I. C., and Harrison, S. P.: The importance of antecedent vegetation and drought conditions as global drivers of burnt area, Biogeosciences, 18, 3861–3879, https://doi.org/10.5194/bg-18-3861-2021, 2021.
Kumar, D., Pfeiffer, M., Gaillard, C., Langan, L., and Scheiter, S.: Climate change and elevated CO2 favor forest over savanna under different future scenarios in South Asia, Biogeosciences, 18, 2957–2979, https://doi.org/10.5194/bg-18-2957-2021, 2021.
Li, F., Levis, S., and Ward, D. S.: Quantifying the role of fire in the Earth system – Part 1: Improved global fire modeling in the Community Earth System Model (CESM1), Biogeosciences, 10, 2293–2314, https://doi.org/10.5194/bg-10-2293-2013, 2013.
Li, W., MacBean, N., Ciais, P., Defourny, P., Lamarche, C., Bontemps, S., Houghton, R. A., and Peng, S.: Gross and net land cover changes in the main plant functional types derived from the annual ESA CCI land cover maps (1992–2015), Earth Syst. Sci. Data, 10, 219–234, https://doi.org/10.5194/essd-10-219-2018, 2018.
Liu, L., Bestel, S., Shi, J., Song, Y., and Chen, X.: Paleolithic human exploitation of plant foods during the last glacial maximum in North China, P. Natl. Acad. Sci. USA, 110, 5380–5385, https://doi.org/10.1073/pnas.1217864110, 2013.
Lohmann, G., Butzin, M., Eissner, N., Shi, X., and Stepanek, C.: Abrupt climate and weather changes across time scales, Paleoceanogr. Paleocl., 35, e2019PA003782, https://doi.org/10.1029/2019PA003782, 2020.
Marlon, J. R., Bartlein, P. J., Carcaillet, C., Gavin, D. G., Harrison, S. P., Higuera, P. E., Joos, F., Power, M. J., and Prentice, I. C.: Climate and human influences on global biomass burning over the past two millennia, Nat. Geosci., 1, 697–702, https://doi.org/10.1038/ngeo313, 2008.
Marlon, J. R., Kelly, R., Daniau, A.-L., Vannière, B., Power, M. J., Bartlein, P., Higuera, P., Blarquez, O., Brewer, S., Brücher, T., Feurdean, A., Romera, G. G., Iglesias, V., Maezumi, S. Y., Magi, B., Courtney Mustaphi, C. J., and Zhihai, T.: Reconstructions of biomass burning from sediment-charcoal records to improve data–model comparisons, Biogeosciences, 13, 3225–3244, https://doi.org/10.5194/bg-13-3225-2016, 2016.
Martin Calvo, M. and Prentice, I. C.: Effects of fire and CO2 on biogeography and primary production in glacial and modern climates, New Phytol., 208, 987–994, https://doi.org/10.1111/nph.13485, 2015.
Martin Calvo, M., Prentice, I. C., and Harrison, S. P.: Climate versus carbon dioxide controls on biomass burning: a model analysis of the glacial–interglacial contrast, Biogeosciences, 11, 6017–6027, https://doi.org/10.5194/bg-11-6017-2014, 2014.
Mauritsen, T., Bader, J., Becker, T., Behrens, J., Bittner, M., Brokopf, R., Brovkin, V., Claussen, M., Crueger, T., Esch, M., Fast, I., Fiedler, S., Fläschner, D., Gayler, V., Giorgetta, M., Goll, D. S., Haak, H., Hagemann, S., Hedemann, C., Hohenegger, C., Ilyina, T., Jahns, T., Jimenéz-de-la-Cuesta, D., Jungclaus, J., Kleinen, T., Kloster, S., Kracher, D., Kinne, S., Kleberg, D., Lasslop, G., Kornblueh, L., Marotzke, J., Matei, D., Meraner, K., Mikolajewicz, U., Modali, K., Möbis, B., Müller, A. W., Julia, E. M. S., Nabel, J. E. M. S., Nam, C. C. W., Notz, D., Nyawira, S., Paulsen, H., Peters, K., Pincus, R., Pohlmann, H., Pongratz, J., Popp, M., Jürgen Raddatz, T., Rast, S., Redler, R., Reick, C. H., Rohrschneider, T., Schemann, V., Schmidt, H., Schnur, R., Schulzweida, U., Six, K. D., Stein, L., Stemmler, I., Stevens, B., Storch, J.-S. V., Tian, F., Voigt, A., Vrese, P., Wieners, K., Wilkenskjeld, S., Winkler, A., and Roeckner, E.: Developments in the MPI-M Earth System Model version 1.2 (MPI-ESM1.2) and its response to increasing CO2, J. Adv. Model. Earth Sy., 11, 998–1038, https://doi.org/10.1029/2018MS001400, 2019.
Moreno, P. I., Videla, J., Valero-Garcés, B., Alloway, B. V., and Heusser, L. E.: A continuous record of vegetation, fire-regime and climatic changes in northwestern Patagonia spanning the last 25,000 years, Quaternary Sci. Rev., 198, 15–36, https://doi.org/10.1016/j.quascirev.2018.08.013, 2018.
Orme, D. and Marion: ImperialCollegeLondon/pyrealm: v0.10.1 (0.10.1), Zenodo [code], https://doi.org/10.5281/zenodo.8366848, 2023.
Pausas, J. G.: Bark thickness and fire regime, Funct. Ecol., 29, 315–327, https://doi.org/10.1111/1365-2435.12372, 2014.
Pausas, J. G. and Keeley, J. E.: Wildfires and global change, Front. Ecol. Environ., 19, 387–395, https://doi.org/10.1002/fee.2359, 2021.
Pausas, J. G. and Ribeiro, E.: The global fire–productivity relationship, Global Ecol. Biogeogr., 22, 728–736, https://doi.org/10.1111/geb.12043, 2013.
Pechony, O. and Shindell, D. T.: Driving forces of global wildfires over the past millennium and the forthcoming century, P. Natl. Acad. Sci. USA, 107, 19167–19170, https://doi.org/10.1073/pnas.1003669107, 2010.
Peltier, W. R., Argus, D. F., and Drummond, R.: Space geodesy constrains ice age terminal deglaciation: The global ICE-6G_C (VM5a) model, J. Geophys. Res.-Sol. Ea., 120, 450–487, https://doi.org/10.1002/2014JB011176, 2015.
Piao, S., Wang, X., Park, T., Chen, C., Lian, X. U., He, Y., Bjerke, J. W., Chen, A., Ciais, P., Tømmervik, H., Nemani, R. R., and Myneni, R. B.: Characteristics, drivers and feedbacks of global greening, Nature Reviews Earth and Environment, 1, 14–27, https://doi.org/10.1038/s43017-019-0001-x, 2020.
Portenga, E. W., Rood, D. H., Bishop, P., and Bierman, P. R.: A late Holocene onset of Aboriginal burning in southeastern Australia, Geology, 44, 131–134, https://doi.org/10.1130/G37257.1, 2016.
Power, M. J., Ortiz, N., Marlon, J., Bartlein, P. J., Harrison, S. P., Mayle, F., Ballouche, A., Bradshaw, R., Carcaillet, C., Cordova, C., Mooney, S., Moreno, P., Prentice, I. C., Thonicke, K., Tinner, W., Whitlock, C., Zhang, Y., Zhao, Y., Anderson, R. S., Beer, R., Behling, H., Briles, C., Brown, K., Brunelle, A., Bush, M., Clark, J., Colombaroli, D., Chu, C. Q., Daniels, M., Dodson, J., Edwards, M. E., Fisinger, W., Gavin, D. G., Gobet, E., Hallett, D. J., Higuera, P., Horn, S., Inoue, J., Kaltenrieder, P., Kennedy, L., Kong, Z. C., Long, C., Lynch, J., Lynch, B., McGlone, M., Meeks, S., Meyer, G., Minckley, T., Mohr, J., Noti, R., Pierce, J., Richard, P., Shuman, B. J., Takahara, H., Toney, J., Turney, C., Umbanhower, C., Vandergoes, M., Vanniere, B., Vescovi, E., Walsh, M., Wang, X., Williams, N., Wilmshurst, J., and Zhang, J. H.: Changes in fire regimes since the Last Glacial Maximum: an assessment based on a global synthesis and analysis of charcoal data, Clim. Dynam., 30, 887–907, https://doi.org/10.1007/s00382-007-0334-x, 2008.
Rodrigues, M., Costafreda-Aumedes, S., Comas, C., and Vega-García, C.: Spatial stratification of wildfire drivers towards enhanced definition of large-fire regime zoning and fire seasons, Sci. Total Environ., 689, 634–644, https://doi.org/10.1016/j.scitotenv.2019.06.467, 2019.
Rogers, B. M., Balch, J. K., Goetz, S. J., Lehmann, C. E. R., and Turetsky, M.: Focus on changing fire regimes: interactions with climate, ecosystems, and society, Environ. Res. Lett., 15, 030201, https://doi.org/10.1088/1748-9326/ab6d3a, 2020.
Rowe, C., Wurster, C. M., Zwart, C., Brand, M., Hutley, L. B., Levchenko, V., and Bird, M. I.: Vegetation over the last glacial maximum at Girraween Lagoon, monsoonal northern Australia, Quaternary Res., 102, 39–52, https://doi.org/10.1017/qua.2020.50, 2021.
Ruan, Y., Mohtadi, M., Dupont, L. M., Hebbeln, D., van der Kaars, S., Hopmans, E. C., Schouten, S., Hyer, E. J., and Schefuß, E.: Interaction of fire, vegetation, and climate in tropical ecosystems: A multiproxy study over the past 22,000 years, Global Biogeochem. Cy., 34, e2020GB006677, https://doi.org/10.1029/2020GB006677, 2020.
Rubino, M., D'Onofrio, A., Seki, O., and Bendle, J. A.: Ice-core records of biomass burning, Anthropocene Review, 3, 140–162, https://doi.org/10.1177/2053019615605117, 2015.
Scheiter, S., Kumar, D., Corlett, R. T., Gaillard, C., Langan, L., Lapuz, R. S., Martens, C., Pfeiffer, M., and Tomlinson, K. W.: Climate change promotes transitions to tall evergreen vegetation in tropical Asia, Glob. Change Biol., 26, 5106–5124, https://doi.org/10.1111/gcb.15217, 2020.
Schertzer, E., Staver, A. C., and Levin, S. A.: Implications of the spatial dynamics of fire spread for the bistability of savanna and forest, J. Math. Biol., 70, 329–341, https://doi.org/10.1007/s00285-014-0757-z, 2015.
Sedano, F. and Randerson, J. T.: Multi-scale influence of vapor pressure deficit on fire ignition and spread in boreal forest ecosystems, Biogeosciences, 11, 3739–3755, https://doi.org/10.5194/bg-11-3739-2014, 2014.
Sidorenko, D., Rackow, T., Jung, T., Semmler, T., Barbi, D., Danilov, S., Dethloff, K., Dorn, W., Fieg, K., Gößling, H. F., Handorf, D., Harig, S., Hiller, W., Juricke, S., Losch, M., Schröter, J., Sein, D. V., and Wang, Q.: Towards multi-resolution global climate modeling with ECHAM6–FESOM. Part I: model formulation and mean climate, Clim. Dynam., 44, 757–780, https://doi.org/10.1007/s00382-014-2290-6, 2015.
Snitker, G.: Identifying natural and anthropogenic drivers of prehistoric fire regimes through simulated charcoal records, J. Archaeol. Sci., 95, 1–15, https://doi.org/10.1016/j.jas.2018.04.009, 2018.
Song, M., Dodson, J., Lu, F., Shi, G., and Yan, H.: A continuous paleorecord of vegetation and environmental change from Erxianyan Wetland over the past 60,000 years in central China, Palaeogeogr. Palaeocl., 613, 111399, https://doi.org/10.1016/j.palaeo.2023.111399, 2023.
Song, Y., Jiao, W., Wang, J., and Wang, L.: Increased global vegetation productivity despite rising atmospheric dryness over the last two decades, Earth's Future, 10, e2021EF002634, https://doi.org/10.1029/2021EF002634, 2022.
Stocker, B. D., Wang, H., Smith, N. G., Harrison, S. P., Keenan, T. F., Sandoval, D., Davis, T., and Prentice, I. C.: P-model v1.0: an optimality-based light use efficiency model for simulating ecosystem gross primary production, Geosci. Model Dev., 13, 1545–1581, https://doi.org/10.5194/gmd-13-1545-2020, 2020.
Tallavaara, M., Luoto, M., Korhonen, N., Järvinen, H., and Seppä, H.: Human population dynamics in Europe over the Last Glacial Maximum, P. Natl. Acad. Sci. USA, 112, 8232–8237, https://doi.org/10.1073/pnas.1503784112, 2015.
Tierney, J. E., Zhu, J., King, J., Malevich, S. B., Hakim, G. J., and Poulsen, C. J.: Glacial cooling and climate sensitivity revisited, Nature, 584, 569–573, https://doi.org/10.1038/s41586-020-2617-x, 2020.
Van der Sleen, P., Groenendijk, P., Vlam, M., Anten, N. P. R., Boom, A., Bongers, F., Pons, T. L., Terburg, G., and Zuidema, P. A.: No growth stimulation of tropical trees by 150 years of CO2 fertilization but water-use efficiency increased, Nat. Geosci., 8, 24–28, https://doi.org/10.1038/ngeo2313, 2015.
Wang, H., Prentice, I. C., Keenan, T. F., Davis, T. W., Wright, I. J., Cornwell, W. K., Evans, B. J., and Peng, C.: Towards a universal model for carbon dioxide uptake by plants, Nature Plants, 3, 734–741, https://doi.org/10.1038/s41477-017-0006-8, 2017.
Wang, Z., Chappellaz, J., Park, K., and Mak, J. E.: Large variations in Southern Hemisphere biomass burning during the last 650 years, Science, 330, 1663–1666, https://doi.org/10.1126/science.1197257, 2010.
WCRP: WCRP Coupled Model Intercomparison Project (Phase 6), WCRP [data set], https://esgf-node.llnl.gov/projects/cmip6/, last access: 26 September 2023.
Williams, A. N., Ulm, S., Cook, A. R., Langley, M. C., and Collard, M.: Human refugia in Australia during the Last Glacial Maximum and terminal Pleistocene: A geospatial analysis of the 25–12 ka Australian archaeological record, J. Archaeol. Sci., 40, 4612–4625, https://doi.org/10.1016/j.jas.2013.06.015, 2013.
Williams, A. P., Allen, C. D., Macalady, A. K., Griffin, D., Woodhouse, C. A., Meko, D. M., Swetnam, T. W., Rauscher, S. A., Seager, R., Grissino-Mayer, H. D., Dean, J. S., Cook, E. R., Gangodagamage, C., Cai, M., and McDowell, N. G.: Temperature as a potent driver of regional forest drought stress and tree mortality, Nature Climate Change Change, 3, 292–297, https://doi.org/10.1038/nclimate1693, 2013.