the Creative Commons Attribution 4.0 License.
the Creative Commons Attribution 4.0 License.
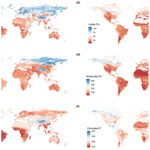
Global patterns and drivers of phosphorus fractions in natural soils
Xianjin He
Laurent Augusto
Bruno Ringeval
Ying-Ping Wang
Julian Helfenstein
Yuanyuan Huang
Most phosphorus (P) in soils is unavailable for direct biological uptake, as it is locked within primary or secondary mineral particles, adsorbed to mineral surfaces, or immobilized inside of organic material. Deciphering the composition of different P forms in soil is critical for understanding P bioavailability and its underlying dynamics. However, widely used global estimates of different soil P forms are based on a dataset containing few measurements in which many regions or soil types are unrepresented. This poses a major source of uncertainty in assessments that rely on these estimates to quantify soil P constraints on biological activity controlling global food production and terrestrial carbon balance. To address this issue, we consolidated a database of six major soil P “forms” containing 1857 entries from globally distributed (semi-)natural soils and 11 related environmental variables. These six different forms of P (labile inorganic P (Pi), labile organic P (Po), moderately labile Pi, moderately labile Po, primary mineral P, and occluded P) were measured using a sequential P fractionation method. As they do not represent precise forms of specific discrete P compounds in the soil but rather resemble operational pools, we will now refer to them as P pools. In order to quantify the relative importance of 11 soil-forming variables in predicting soil P pool concentrations and then make further predictions at the global scale, we trained random forest regression models for each of the P pools and captured observed variation with R2 higher than 60 %. We identified total soil P concentration as the most important predictor of all soil P pool concentrations, except for primary mineral P concentration, which is primarily controlled by soil pH and only secondarily by total soil P concentration. When expressed in relative values (proportion of total P), the model showed that soil pH is generally the most important predictor for proportions of all soil P pools, alongside the prominent influences of soil organic carbon, total P concentration, soil depth, and biome. These results suggest that, while concentration values of P pools logically strongly depend on soil total P concentration, the relative values of the different pools are modulated by other soil properties and the environmental context. Using the trained random forest models, we predicted soil P pools' distributions in natural systems at a resolution of . Our global maps of different P pools in soils as well as the pools' underlying drivers can inform assessments of the role of natural P availability for ecosystem productivity, climate change mitigation, and the functioning of the Earth system.
- Article
(5729 KB) - Full-text XML
-
Supplement
(1062 KB) - BibTeX
- EndNote
Phosphorus (P) is a key nutrient limiting plant growth across a wide range of ecosystems (Augusto et al., 2017; Elser et al., 2007; Hou et al., 2020). Soil is typically the major P source for plants in natural terrestrial ecosystems (Weihrauch and Opp, 2018). P supplied by the soil plays a vital role in determining the structures, functions, and processes in terrestrial ecosystems (Peltzer et al., 2010; Wardle et al., 2004). For example, soil P availability imposes a major constraint on plant productivity in terrestrial ecosystems worldwide (Augusto et al., 2017; Ellsworth et al., 2022; Elser et al., 2007; Hou et al., 2020, 2021) and affects modeled projections of terrestrial carbon cycle responses to climate change and increasing atmospheric carbon dioxide concentrations (Cunha et al., 2022; Fleischer et al., 2019; Goll et al., 2012). The size of soil P stocks is large compared to annual plant P requirements (Wang et al., 2018) and the amount of P stored in vegetation (Wang et al., 2018; Zhang et al., 2021). However, only a small proportion of soil P can be directly taken up by plants (Morel et al., 2014), with most P tightly sorbed to soil minerals, organic compounds, or organo-mineral complexes with a turnover time of centuries to millennia or longer (Helfenstein et al., 2020; Vitousek et al., 2010). Consequently, vegetation growth is often limited by P availability in ecosystems across the globe (Vitousek et al., 2010; Wardle et al., 2004). For these reasons, the investigation of P dynamics and P bioavailability in the soil requires the identification and separation of different soil P pools (Crews et al., 1995; Walker and Syers, 1976).
Our knowledge of the various pools of P existing in soils is largely based on soil chronosequences and climosequences that investigated how P is cycled during pedogenesis (Crews et al., 1995; Walker and Syers, 1976). These studies revealed that chemical weathering results in the release of P from primary minerals, after which it can be converted to organic P through biological uptake, sorbed to soil particles, or occluded within secondary minerals. The most commonly used procedures for the sequential fractionation of P in soils were developed by Hedley et al. (1982) and later modified by Tiessen and Moir (1993). This method exploits differences in solubility to separate different “forms” of P occurring in the soil. Though it cannot be used to identify specific discrete P compounds in the soil, this approach has proven indispensable for the study of soil P cycling and, as such, is widely used (Condron and Newman, 2011; Klotzbücher et al., 2019; Barrow et al., 2021). In addition to forming the basis for modeling soil P dynamics, these procedures yield operationally defined pools that are used to assess soil fertility and soil development (Wang et al., 2010, 2022). Several studies have called the validity of sequential extractions into question, pointing out that, while it is often assumed that pools from sequential extractions contain distinct forms of P, the reality is much more complex (Condron and Newman, 2011; Gu and Margenot, 2021; Klotzbücher et al., 2019). Nevertheless, radioisotope tracer experiments show that sequentially extracted pools have distinct P exchange behaviors that result in significantly different turnover times (Bünemann et al., 2004; Helfenstein et al., 2018, 2021; Vu et al., 2010).
Numerous studies have used data from P fractionations to explore drivers of spatial differences in soil P pools from local to global scales (e.g., Brucker and Spohn, 2019; Hou et al., 2018a; Yang and Post, 2011; Chen et al., 2015). Yang and Post (2011) compiled Hedley P pools data from 178 soil samples to explore P dynamics along a soil development gradient. Their results generally supported the conceptual model proposed by Walker and Syers (1976): the gradual decrease of primary mineral-bound P, the continual increase and eventual dominance of occluded P, and the overall decrease of total P as pedogenesis progresses. However, the conceptual model of Walker and Syers (1976) disagreed with the results of Yang and Post (2011), who found that labile Pi and moderately labile Pi (non-occluded P in Walker and Syers' model) formed a significant fraction of total P at every stage of pedogenic development. Augusto et al. (2017) compiled 1684 measurements of P pools that were taken worldwide using the Hedley fractionation method. This work found that total P content was a main factor in determining the concentrations of labile Pi and organic P pools. Almost concomitantly, Hou et al. (2018a) used a global dataset compiled from analyses of 802 soil samples to examine climate effects on the soil P cycle and P availability and found that soil labile Pi concentration decreased with increasing mean annual temperature, which was mainly due to decreasing soil organic P and primary mineral P with increasing temperature. Although those studies advanced our understanding of factors controlling the size of various soil P pools, their focus was largely contained to the effects of climatic factors or the soil weathering stage on a few select P pools, mainly labile Pi and organic P. Thus, we still lack a comprehensive understanding of the relationships between environmental drivers and the various soil P pools at a global scale.
Despite significant efforts to synthesize global Hedley soil P pool data, to our knowledge, only a single mapping of soil P fractions across natural terrestrial ecosystems exists, and this work was based on the upscaling of measurements taken from only 178 samples (Yang et al., 2013). These global estimates and associated maps of soil P pools have been used to explore global patterns of soil P supply and to estimate P availability in natural and managed systems (e.g., Ringeval et al., 2017; Sun et al., 2017). They have also been used to calibrate or initialize a range of global P models (Wang et al., 2010; Yang et al., 2014). However, the poor global coverage of the underlying data introduces significant uncertainty, potentially resulting in misinformed model predictions and assessments.
We recently developed a new global map of soil total P concentrations and explored the underlying drivers, taking advantage of improved data availability and the use of nonlinear statistical modeling (He et al., 2021). Here, we constructed a database of soil P pools in 1857 globally distributed (semi-)natural soils collected from 274 published studies, 1 order of magnitude larger than the dataset used by Yang et al. (2013) (see comparison in Fig. S1 in the Supplement). Using this database, we trained random forest models to capture observed variations in Hedley P pool concentrations at the site level with two aims: (1) to quantify the relative importance of different drivers of spatial variation in each soil P pool and (2) to develop global distribution maps of various P pools at a spatial resolution of using the calibrated random forest regression model.
2.1 Soil P fractionation terminology and procedure
In the present study, we use the word “pool” to indicate the concentrations quantified in each step during sequential fractionation and the word “proportion” to represent the size of a pool relative to total P. We try to avoid using “fraction” to describe different P forms anymore because it is easy to confuse with proportion. There is disagreement about how to interpret the different pools yielded by sequential fractionation (Gu and Margenot, 2019; Barrow et al., 2021; Klotzbücher et al., 2019; Condron and Newman, 2011; Helfenstein et al., 2020). Here, we adopt a widely used regime for understanding these pools, which correspond to different forms of soil P: the resin Pi pool represents the soil soluble Pi pool, which is immediately accessible to plants. The Pi pool can be released by ligand exchange with bicarbonate ions; this pool is available to plants and persists only for short periods (e.g., a growing season). Due to their functional similarity, the resin and Pi pools can be combined and used as an index of labile inorganic P (i.e., “available” P). The Po pool represents labile Po that can be utilized by plants after mineralization. The OH− P (Pi and Po) pools mainly indicate moderately labile P that is bound to both amorphous and crystalline Al and Fe; these pools represent P that is moderately available to plants. The 1 M HCl Pi pool represents primary mineral P that is bound to calcium and that can be utilized by plants after it is released by weathering. And other P pools, such as residual P, are the least available to plants due to their particularly low solubility.
To integrate data from studies that use different interpretations, we consider a set of six simplified P pools (Fig. 1): labile Pi, labile Po, moderately labile Pi, moderately labile Po, primary mineral P, and occluded P. Labile Pi includes the resin Pi and Pi pools; labile Po and moderately labile Po are organic pools extracted by carbonate and NaOH, respectively; moderately labile Pi is the NaOH Pi fraction; primary mineral P represents the 1 M HCl Pi pool; and occluded P includes any remaining P (Hou et al., 2018b).
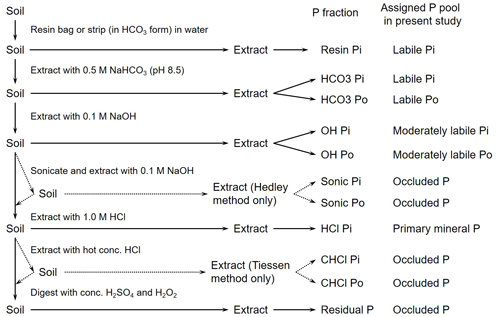
Figure 1Flow chart of soil P fractionation. The flow chart follows the procedures outlined by Hedley et al. (1982) and Tiessen and Moir (1993). Redrawn according to Hou et al. (2018b).
We collected, filtered, and processed soil P pool data (see Sect. 2.2.) from the literature (Sect. S1 data source references in the Supplement). First, we added all measured P pools together to calculate total soil P, unless at least one pool had a missing value. In this case, we used the measured value of total soil P presented in that paper instead. Second, if phosphate was extracted using deionized water before the resin P extraction step, the labile Pi pool includes both resin and aqueous P. If the extraction procedure began by using a sodium bicarbonate solution instead of resin P, we classified Pi as labile Pi. Third, the labile Po pool and the moderately labile Po pool represent the -extracted Po and NaOH-extracted pools, respectively. The raw data contained other organic P pools (e.g., Po extracted by sonication and NaOH or by hot, concentrated HCl), which we included as part of occluded P. Fourth, if occluded P was not reported, we calculated this pool's concentration by subtracting the sum of the five other pools from total P.
2.2 Data source and processing
We collected soil P pool data by aggregating all the publications that cited either one of two main references dedicated to Hedley's method (Hedley et al., 1982; Tiessen and Moir, 1993). We included all studies that reported data from (semi-)natural soils that supported primary vegetation or that had been reforested with a stand older than 10 years and no documented history of P fertilization. We excluded observations taken from pot experiments, mine zones, and intertidal zones, as P pools in these soils could be affected by factors different from those influencing (semi-)natural soils. Despite our best efforts, we cannot rule out that our database includes data collected from soils affected by undocumented anthropogenic activities in the past (e.g., P fertilization occurring before reforestation), particularly in western Europe and the eastern USA (e.g., De Schrijver et al., 2012). All data were collected at the plot scale. For data that included replicates within a plot or soil layer, average values were calculated.
To compile our database, we first combined the two existing global databases (Augusto et al., 2017; Hou et al., 2018b). Detailed information about the methods used to construct these datasets can be found in the original publications. We extracted observations from these two databases by selecting only unfertilized, uncultivated, and (semi-)natural soils. This yielded 1684 observations from 182 studies from the dataset developed by Augusto et al. (2017) and 802 observations from 99 studies from the dataset developed by Hou et al. (2018b). Next, we removed 375 duplicates, after which our dataset contained 2111 observations from 245 studies (Fig. S2). Because we use total soil P concentration as a predictor of soil P pools, we removed data that did not include total soil P (calculated as the sum of P pools or measured by a separate method) or that did not identify the concentration of at least one pool (e.g., labile Pi, labile Po, moderately labile Pi, moderately labile Po, primary mineral P, or occluded P). In this step, 816 observations were removed, resulting in a dataset that included 1295 observations from 178 studies.
Next, we added additional observations by compiling data from literature published after 2016, the final year included in the database compiled by Hou et al. (2018b). We used Google Scholar to search for studies published between 2016 and 8 August 2021 that referenced either Hedley et al. (1982) or Tiessen and Moir (1993). This search returned 701 publications citing Hedley et al. (1982) and 245 citing Tiessen and Moir (1993). From this set, we selected studies that presented soil P data collected using the fractionation method for (semi-)natural soils. The resulting 562 observations from 96 studies were added to our final dataset, which includes a total of 1857 observations collected from 729 sites from 274 studies (Sect. S1).
In addition to soil P pool concentration and site coordinates, our dataset contains site characteristics including climate variables (i.e., mean annual temperature (MAT), mean annual precipitation (MAP), and potential biome), soil physicochemical properties (e.g., soil organic carbon concentration (SOC), soil clay and sand content, and soil pH), and elevation (Table 1). The potential biome was identified using a global map of potential natural biomes (i.e., the global distribution of biomes that would exist in the absence of human activity) (Hengl et al., 2018). This categorization includes seven ecosystem types, including tropical forest, temperate forest, boreal forest, grassland, savanna, desert, and tundra. We did not include a parent material type because it can be inferred from soil total P concentration and other soil properties (e.g., soil texture and pH) (Augusto et al., 2017; He et al., 2021). Because soil age was rarely reported, we used USDA soil order identity as a proxy for three age classes: slightly, intermediately, and strongly weathered (Smeck, 1985; Yang et al., 2013). Among the 12 USDA soil orders, Entisols, Inceptisols, Histosols, Andisols, and Gelisols are classified as slightly weathered soils. Alfisols, Mollisols, Aridisols, and Vertisols are classified as intermediately weathered soils. Oxisols, Ultisols, and Spodosols are classified as strongly weathered soils (Yang et al., 2013; Smeck, 1985). Given that atmospheric P inputs are small (0.3 kg P ha yr−1, on average) compared to soil P stocks (Mahowald et al., 2008; Wang et al., 2015) and are also highly uncertain over timescales relevant to soil development, we do not consider atmospheric inputs to be a predictor of P pools. As such, we did not include this information in our dataset. We extracted data from each publication as available. In cases in which relevant information was not reported, we extracted the missing data from gridded datasets (Table S1 in the Supplement) based on the geographic coordinates of the study sites.
Table 1Summary of training data used to predict soil P pool concentrations. P10 and P90 indicate percentile ranks of 10 % and 90 %, respectively. Proportions from literature (PFL) and proportions from gridded maps (PFGMs) indicate proportions of measurements from the literature and extracted from global gridded maps, respectively.
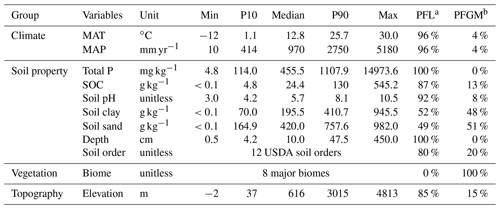
MAT: mean annual temperature; MAP: mean annual precipitation; SOC: soil organic carbon. a PFL: proportion from literature. b PFGM: proportion from gridded map. PFL and PFGM indicate proportions of measurements from literature and extracted from global gridded maps, respectively.
In random forest modeling, correlated predictors can be substituted for each other so that the importance of correlated predictors will be shared, making each predictor's estimated importance smaller than its true value (Strobl et al., 2008). Thus, we did not include the soil total nitrogen content, as it is strongly correlated with SOC (), nor did we include the aridity index as it is strongly correlated with MAP (). We also did not include rarely reported variables that were included in the referenced studies (e.g., soil extractable aluminum and iron concentrations).
2.3 Statistical modeling
All statistical analyses and plotting were performed in the R environment (v. 4.0.2) (R Core Team, 2018).
The database includes some extreme values in each P pool. These values were likely observed in exceptional geological contexts (Porder and Ramachandran, 2013) or in special soils (e.g., very young volcanic soils). We included these extreme values in the shared version of the dataset. However, these values were excluded from data used in model training, as the extremely high values could have a large influence on modeled relationships between soil P pools and predictors. To this end, we only included values falling in the interval between 1 % and 99 % (Table 2). As we only generate predictions in the top 100 cm depth, the training of the model was done using observations in 0–100 cm.
Table 2Statistical summary of P pools in global (semi-)natural soils. Results are based on our database of collected sites. P1, P10, P25, P75, P90, and P99 indicate percentile ranks of 1 %, 10 %, 25 %, 75 %, 90 %, and 99 %, respectively.
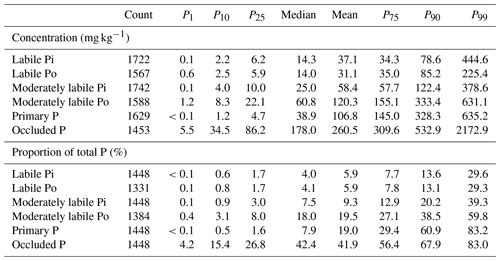
We used random forest regression models (Breiman, 2001) to predict global patterns of distribution for individual soil P pools. It is a type of ensemble learning algorithm that combines multiple decision trees to make predictions. It reduces the risk of overfitting and improves the generalization performance by using random subsets of input variables and training data. The output is the average prediction of all the trees (James et al., 2013). All models included the same 11 predictors: MAT, MAP, potential biome, total P, soil depth, SOC, soil clay and sand content, soil pH, elevation, and soil weathering stage. The random forest analysis accounts for interactions and nonlinear relationships between predictors and is appropriate for handling the multicollinearity problem in the multivariate regression (Delgado-Baquerizo et al., 2017). We performed random forest regression analysis using the R package caret by applying the embedded R package randomForest version 3.1 (Liaw and Wiener, 2002) with an automated mtry parameter. A 5-fold cross-validation was performed using the R package caret (v. 6.0-86) (Kuhn, 2020) to evaluate model performance. The mean decrease in accuracy (%IncMSE) was used to evaluate the relative importance of each variable as a predictor of a soil P pool. The mean decrease in accuracy plot shows how the accuracy of the fitted model declines with the exclusion of a predictor. The greater the decline in accuracy, the more important the variable is for prediction. In this study, the importance measure was calculated for each tree and averaged across the forest (500 trees). Our model found that all 11 variables are important for predicting pool concentrations; thus, all were used as predictors as we developed the global distribution map. Partial dependence plots are a graphical technique used in machine learning to show how the value of a particular input variable affects the predictions of a model, while holding all other input variables constant at their average values in the training data (James et al., 2013). We used the partial_dependence function in the R package edarf version 1.1.1 (Jones and Linder, 2016) to calculate the partial dependence of the response on an arbitrary dimensional set of continuous predictors from a fitted random forest model.
Finally, we applied the above trained models for each of the soil P pools to global databases of the 11 predictors to generate global predictions of each soil P pool. The gridded predictor variables used for the global prediction were all re-gridded to a spatial resolution of (the original resolution can be found in Table S1). The predict function in the ranger package (Wright and Ziegler, 2017) can compute the standard error of a predicted value. To estimate standard errors based on out-of-bag predictions, we used the infinitesimal jackknife for bagging approach (Wager et al., 2014). We did not mask croplands or other areas heavily influenced by human activity (e.g., urban areas), so pool concentrations predicted for these regions should be interpreted as the natural state prior to anthropogenic activity.
Soil depth was used as a predictor, allowing models to predict soil P pool concentration for any given depth (Hengl et al., 2017). The partial dependence plot indicated that soil P pool concentration changed with soil depth in the top 50 cm but not in deeper layers (>50 cm) (Fig. S3e). As such, we generated predictions at six standard depths for all soil P pool concentrations: 0, 10, 20, 30, 50, and 100 cm. Averages for a depth interval (e.g., 0–30 or 0–100 cm) can be derived by calculating the weighted average of the predictions within that interval (Hengl et al., 2017).
3.1 Characters of P pools in natural soils across the world
Our soil P pool database includes 1857 measurements from 729 geographically distinct sites and covers 6 continents, all major biomes, and all 12 USDA soil orders in terrestrial ecosystems (Fig. 2). The database includes pool concentrations measured in samples collected from the 0.5 cm to a depth of 450 cm, with 83 % of the measurements taken from the topsoil (0–30 cm).
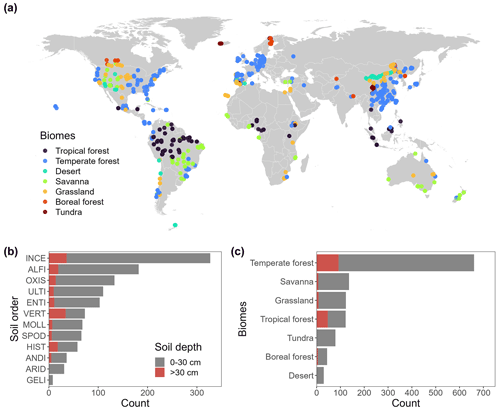
Figure 2Distribution of site-level training data (a). The database contains 1838 observations covering 12 USDA soil orders (b) and all major terrestrial biomes (c).
From the global median values (Table 2), the largest pool among the six pools considered is the occluded P, accounting for more than 40 % of the soil total P, followed by the moderately labile pools (Pi and Po mainly bound to Al and Fe), accounting for about a quarter of total P. Primary mineral P (bound to calcium) accounted for a minor proportion (7.9 %) of soil total P; labile P pools (Pi and Po) represent the smallest proportions of total P (around 4 %, respectively).
3.2 Model performance of different P pools in soils
The random forest regression models explained 62 %, 64 %, 60 %, 83 %, 76 %, and 82 % of the variance in the concentrations of labile Pi, labile Po, moderately labile Pi, moderately labile Po, primary P, and occluded P, respectively (Fig. 3). Using the importance measure (%IncMSE), we identified total P concentration as the most important predictor for concentrations of soil labile Pi, labile Po, moderately labile Pi, moderately labile Po, and occluded P and soil pH as the most important predictor for soil primary P (Fig. 3). The random forest regression models explained 48 %, 58 %, 52 %, 64 %, 80 %, and 58 % of the variance in proportions of labile Pi, labile Po, moderately labile Pi, moderately labile Po, primary P, and occluded P, respectively (Fig. S4). Based on the importance measure, soil pH is generally the most important predictor for proportions of all soil P pools, with prominent influences of total P concentration, soil organic carbon, soil depth, and biome (Fig. S4). These results suggest that, while concentration values of P pools logically strongly depend on soil total P concentration, the relative values of the different pools are modulated by other soil properties and the environmental context.
3.3 Global patterns and drivers of P pools in natural soils
Our global predictions (Fig. 4) revealed that average values across all P pools were higher in slightly weathered soils compared to those in more weathered soils (Fig. 5a), reflecting the strong effect of the initial stages of soil development on soil P depletion. While occluded P proportion increased with soil development, the proportions of labile and moderately labile P (Pi and Po) were fairly independent of soil weathering stage (Fig. 5b).
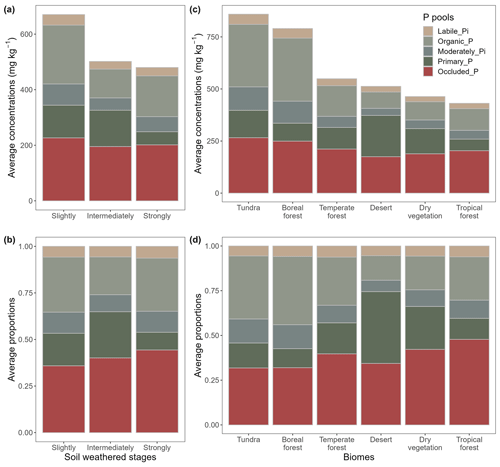
Figure 5Average concentrations of P pools and their proportions of total soil P concentration across soil weathering stages and biomes. Labile and moderately labile Po form the organic pool. The results are based on global estimates for 0–30 cm depth. Dry vegetation combines grassland and savanna biomes to simplify the figure.
Our global predictions also indicated that soil P pool concentrations varied substantially among different biomes. Lower P pool concentrations were found in warm and/or humid biomes (e.g., tropical forest and savanna), while higher P pool concentrations were found in northern cold biomes (e.g., tundra and boreal forest) (Fig. 5c). The spatial patterns of pool proportions were different from those of pool concentrations across biomes (Fig. 5d). For example, variation in the proportion of labile Pi was relatively small compared to the variation observed in labile Pi concentrations; moreover, the proportion of occluded P tended to increase in the transition from tundra and boreal forest to tropical forest and savanna (Fig. 5d). It should be noted that the mapped predictions of P pool concentrations across biomes (see Fig. 5c) are not consistent with the measured data (Fig. S5), which indicates that total soil P in tropical forests is higher than in any other biome. This result suggests a sampling bias due to overrepresentation of high total soil P sites in the tropical forest data.
Partial dependence plots (Fig. S3) and the results of Pearson correlation analysis (Fig. 6) were generally consistent. Both analyses revealed that concentrations for all six pools were significantly and positively correlated with total P concentration. SOC was significantly and negatively correlated with primary mineral P concentration but positively correlated with the other five pool concentrations. MAT and MAP were significantly and negatively correlated with concentrations of all soil P pools. Soil pH was significantly and positively correlated with primary mineral P concentration but significantly and negatively correlated with concentrations of the other five P pools. The results of Pearson correlation analysis also indicated that P pool concentrations were well correlated with each other, except for primary mineral P; this pool was negatively correlated with labile Po and not correlated with moderately labile Po concentration. The partial dependence plot indicated the variation of P pool concentrations with increasing soil depth (Fig. S3e). We found a drastic decrease of P pools with soil depth in the top 50 cm of soil, which then became relatively stable at 50–100 cm soil depth. Labile and moderately labile P (both Pi and Po) concentrations also decreased with an increase in soil depth in the top 50 cm, while primary mineral P and occluded P concentrations generally increased with soil depth.
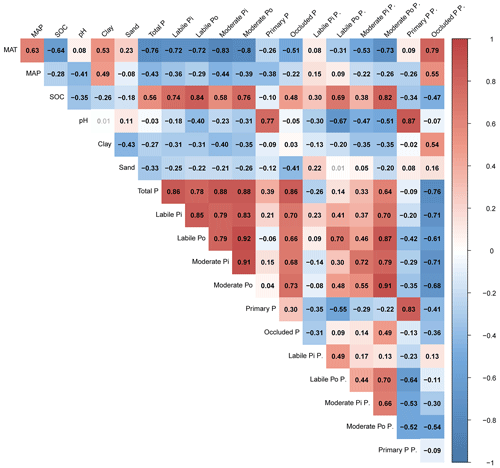
Figure 6Coefficients of Pearson correlations among proportions and concentrations of soil P pools. The results are based on the average global predictions in the top 30 cm of soils. Coefficients with P<0.001 are shown in black and bold. Labile Pi P. indicates the labile Pi proportion. The same meanings apply to the labile Po P., moderately labile Pi P., moderately labile Po P., primary P P., and occluded P P. Elevation is not included this plot, as it is not well correlated with P pool variation in our results.
As for the P pools' proportions, Pearson correlation analysis (Fig. 6) revealed that soil pH was positively correlated with the primary mineral P proportion and negatively correlated with the other five P pool proportions. Soil labile Po, moderately labile Pi, and moderately labile Po proportions decreased substantially with an increase in MAT, while the occluded P proportion increased with MAT. Soil labile Po, moderately labile Pi, and moderately labile Po proportions increased substantially with increasing total P concentration, while the soil labile Pi and occluded P proportions decreased substantially with total P concentration.
There are significant differences between our predictions and those made by Yang et al. (2013) (Fig. S6) in both the magnitude and the spatial patterns associated with most P pool concentrations. The two global estimates were only weakly to moderately correlated (Pearson correlation coefficients between 0.09 and 0.38) (Fig. 7). Yang et al.'s (2013) predictions are lower than ours for organic P, moderately labile Pi, primary mineral P, and occluded P concentrations (Table S2). Although average values for labile Pi concentrations estimated by Yang et al. (2013) were close to ours, they were only weakly correlated with each other (Pearson correlation coefficient of 0.09) (Fig. 7).
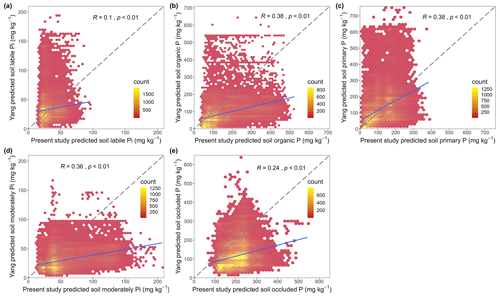
Figure 7Relationship between our predicted P fraction concentrations and Yang et al.'s predictions. Panels (a)–(e) and (f) depict correlations between both sets of predictions for soil labile Pi, organic P, primary mineral P, moderately labile Pi, and occluded P, respectively. The dashed lines indicate the 1:1 line; the blue lines indicate the regression line.
4.1 Improved mapping of different P pools in global natural soils
We trained random forest regression models using 11 variables to predict six soil P pools at different depths in (semi-)natural terrestrial ecosystems, resulting in significant improvements over earlier estimates (Yang et al., 2013). First, we used a new global map of total P concentrations in natural soils (He et al., 2021) as a predictor. Because total P is an important predictor and is highly correlated with all other P pools, a higher quality map of total soil P will also lead to improved predictions of other P pools. Further improvements in global P data availability will thus also be useful to improve maps of other P pools. Second, Yang et al. (2013) used a limited number (n=178) of measurements of Hedley P pools across soils. Our database represents a nearly 10-fold increase, which can better represent the heterogeneous conditions on Earth. Third, Yang et al. (2013) estimated P pool concentrations using total soil P concentrations, global soil order maps, and average proportions of various P pools for different soil orders. However, there are still considerable variabilities in P concentrations within any given soil order, though it could be a good predictor of P pool variation (Cross and Schlesinger, 1995; Yang and Post, 2011). Indeed, we found that soil orders were less informative than other environmental predictors. By including more predictors (e.g., SOC, climate, and soil pH), our model offers significant improvements for capturing the variation observed in soil P composition across the globe.
The above-named technical improvements have made it possible to produce more accurate maps. For example, while Yang et al.'s (2013) global predictions indicated that the highest organic P concentrations were found in the temperate zone, our maps suggest they are in boreal forest and tundra. This is more consistent with general understanding of global soil organic matter distribution (Hengl et al., 2017). Differences between our estimates of different P pools and those presented by Yang et al. (2013) have significant implications for soil P availability to vegetation. The averages and median values of Yang et al.'s (2013) predicted soil organic P, moderately labile Pi, and occluded P concentrations were substantially lower than our estimates. Evidence suggests that soil organic P and moderately labile Pi remain bioavailable on timescales of days to months (Helfenstein et al., 2020; Augusto et al., 2017; Maharjan et al., 2018), while occluded P is bioavailable in the order of years to millennia (Hou et al., 2016; Wang et al., 2007). Thus, soil P availability might be larger than previously assumed in assessments based on estimates by Yang et al. (2013) (e.g., Sun et al. 2017).
4.2 Major drivers of different P pools in natural soils
Our results indicate that global variation in soil P pools is jointly controlled by total P concentration, soil pH, soil development, climatic factors, and soil depth. Given that our models explain >48% of the variance observed in P pools (concentration and proportion), our results suggest that edaphic properties and climatic factors play significant roles in the size and composition of different soil P pools globally.
4.2.1 Effects of total soil P concentration on P pools
We found that total soil P concentration was a prominent predictor of most soil P pools at the global scale and that total P was positively correlated with all P pool concentrations and Po pool proportions. This is consistent with findings at local (Turner and Blackwell, 2013) and global (Augusto et al., 2017; Hou et al., 2018a; Harrison, 1987) scales. Total soil P is influenced by multiple soil-forming factors (e.g., parent material P concentration, climate, soil organic carbon content, and soil texture) (He et al., 2021). Thus, total soil P provides an integrated measure of factors that regulate the size of the P pools. Moreover, this result is consistent with the emerging idea of substrate-based P cycling in natural ecosystems (Lang et al., 2016, 2017): soils with high total P content are usually also associated with a large primary mineral P pool. At these P-rich sites, plant and microbial communities tend to promote P release from primary minerals, with subsequent biological and abiotic transformations resulting in high concentrations in all other P pools (Lang et al., 2016; He et al., 2021) and higher proportions of organic P (Hou et al., 2018c). In contrast, at P-poor sites, plant and microbial communities are more reliant on P recycling systems that promote the mineralization of Po by soil microbes (Achat et al., 2009; Marklein and Houlton, 2012) and the mobilization of moderately labile Pi or even occluded P (Augusto et al., 2017) to sustain the P supply. Therefore, soil P pool concentrations are expected to strongly co-vary with total soil P concentration.
4.2.2 Effects of soil pH on P pools
Consistent with previous studies (Hou et al., 2018c; Kruse et al., 2015; Oburger et al., 2011; Barrow et al., 2020), our results indicate that soil pH is an important predictor of P pool concentrations and proportions in natural soils globally. The relative importance of pH is unsurprising, since the sequential fractionation procedure is based on dissolving a soil sample in solutions of varying acidity/alkalinity. However, the observed pH effects also support the existing mechanistic understanding of the various P forms. The strong positive correlation of primary P and soil pH is expected because (1) the primary P pool is composed mainly of calcium phosphate/apatite, which is highly soluble at low pH but becomes less soluble with increasing pH, and (2) soil pH declines with soil weathering intensity (Delgado-Baquerizo et al., 2020) (e.g., the highest values of soil pH are usually found in dry regions where chemical weathering rates are limited by water availability; Slessarev et al., 2016). Both factors affect the transformation of primary mineral P to other forms.
Soil pH shows important but negative influences on the proportions of other soil P pools (i.e., proportions of labile Po, moderately labile Pi and Po, occluded P, and labile Pi). There are several possible explanations for these relationships. First, low soil pH values (<5.0) inhibit soil microbial activities and the extracellular activity of phosphatase enzymes (Aciego Pietri and Brookes, 2008; Eivazi and Tabatabai, 1977; Xu et al., 2017). Thus, in acidic soils, more organic P (i.e., labile and moderately labile Po) may accumulate than in neutral soils. Second, decreasing soil pH is associated with the accumulation of Fe and Al oxides, which leads to enhanced adsorption of P (i.e., moderately labile Pi and Po). Third, pH tends to decrease as soil weathering advances and base cations are progressively washed out (Slessarev et al., 2016). As soils weather, occluded P accumulates. Therefore, the occluded pool proportion decreases with increasing pH. Fourth, increasing soil pH is associated with enhanced adsorption of dissolved Pi to Ca and Mg, reducing the amount of labile Pi available for plants and soil microorganisms (Fink et al., 2016; Gerke, 2015). This could explain the negative relationship between soil pH and the labile Pi proportion as identified in this study. But increasing soil pH in acidic soils favors soil microbial growth and phosphatase enzyme activity, which could increase P availability. These conflicting mechanisms may be responsible for the relative low importance of predicting the spatial variation of labile Pi proportion.
4.2.3 Effects of climate on P pools
Our global predictions indicated negative effects of climatic factors (i.e., MAT and MAP) on the soil P concentrations, which means a decrease in soil P concentrations, as MAT increases from northern cold biomes (e.g., tundra and boreal forest) to a warm tropical biome (e.g., tropical forest) or MAP increases from arid to humid regions. These results fit well with our understanding of broad P concentration variation with increasing weathering (Walker and Syers, 1976). Also, these results are expected because the main determining factor of soil P pool concentrations (i.e., soil total P concentration) shows a similar pattern (He et al., 2021). Interestingly, we found contrasting responses of the labile Pi pool's proportions along the MAT and MAP gradients. The positive correlations between labile Pi proportion and both MAT and MAP indicated that labile Pi concentration decreased slower than the soil total P as temperature and precipitation increased. This result supported the idea that biological systems evolved to retain soil labile Pi levels despite an overall decrease in total soil P as long as climate factors are favorable for biological activity. In strongly weathered soil with limited soil P stocks but otherwise optimal growing conditions, like in warm and humid tropical forests, the mineralization of Po and mobilization of moderately labile Pi or occluded P could contribute to maintaining high levels of labile Pi due to the high soil temperature for soil enzyme kinetics and abundant carbohydrate supply from photosynthesis fueling biological activity (Vitousek, 1984; Achat et al., 2009; Chacon et al., 2006; Liptzin and Silver, 2009).
4.2.4 Effects of soil development on P pools
The variations of P concentrations and proportions across weathering stages predicted by our model partially support Walker and Syers' (1976) theory based on soil chronosequences. While our results are consistent with the expectations from Walker and Syers' (1976) theory about the increase in the proportion of occluded P that occurs at the expense of primary and organic P during soil development, our results disagree with Walker and Syers' (1976) ideas regarding the evolution of the labile Pi and moderately labile Pi pools during soil development. The evolution of occluded P is commonly explained by the increase of Al and Fe oxide minerals and the decrease of soil pH; in addition to being fixed onto Fe and Al oxides, P that is released from primary minerals or mineralized from organic matter can be occluded by being adsorbed onto mineral surfaces or precipitating in poorly soluble secondary soil minerals (Crews et al., 1995; Quesada et al., 2010; Selmants and Hart, 2010).
In the Walker and Syers model, non-occluded inorganic P increases initially to a peak value and then declines to very low levels during pedogenesis. However, our results showed that labile Pi and moderately labile Pi (non-occluded P in Walker and Syers' (1976) model) formed significant proportions of the total P throughout all soil orders across weathering stages. This could be due to the coarse classification of weathering stages in our study, which may be insufficient to characterize the end members of the range. This explanation is supported by the small proportion of primary mineral P in the slightly weathered soil and the moderate amounts of primary P remaining in strongly weathered soils. In addition, the theory of P distributions along soil development stages stems largely from relatively isolated island locations in New Zealand (Walker and Syers, 1976) and Hawaii (Crews et al. 1995). However, in most other places in the world there is higher dust deposition from surrounding land masses, which is a source of primary P to even highly weathered soils (Vogel et al., 2021). Nevertheless, the contribution of dust deposition to primary P and other forms of P in soil remains unquantified in most land areas.
4.2.5 Effects of soil depth on P pools
We found that soil P pool concentrations varied significantly with soil depth. Total soil P concentration is often higher in topsoil than in subsoil due to biological uplift, which was reported by previous studies (Jobbágy and Jackson, 2001; Porder and Chadwick, 2009). The labile and moderately labile P (in both inorganic and organic pools) concentrations were higher in the topsoil, which can also be explained by biological uplift and highly available P inputs from plants and dust to the topsoil. In contrast, the primary P and occluded P concentrations in the topsoil were lower than in the subsoil. This can be explained by the fact that topsoil tends to be more weathered and developed than the subsoil (Achat et al., 2012; Chen et al., 2021).
4.3 Limitations and prediction uncertainty
In our database, some regions were underrepresented (e.g., northern Canada, middle and northern Asia, and inner Africa), which may result in low accuracy of the predicted values in those regions. In the tropics, high P soils were overrepresented, and the accuracy of predicted values in tropical regions may be quite low. Our database contains 4 times as many observations from surface mineral soils (0–30 cm) than it does from soils deeper than 30 cm. As such, the predicted concentrations of different P pools for deep soils may suffer from larger uncertainties. Finally, large portions of variation remain unexplained by our models, especially variation in soil labile Pi concentrations and proportions (40 % and 52 % unexplained, respectively), indicating that other significant factors were not accounted for in our modeling. These factors may include microbial processes, Fe and Al oxide concentrations, plant community composition, atmospheric deposition, and soil erosion (Kruse et al., 2015; Achat et al., 2016). These limitations highlight the need for additional measurements, particularly from underrepresented regions and the subsoil, as well as measurements of closely associated variables, especially those related to labile Pi.
Here, we compiled the largest database to date of different soil P pools. Using machine learning modeling, we quantified the relative importance of multiple predictors for estimating different soil P pools and estimated these pools at the global scale. Our results indicated that the global concentrations of soil labile Pi, labile Po, moderately labile Pi, moderately labile Po, and occluded P could be generally predicted mainly by the total soil P concentration, while primary P concentration was mainly predicted by soil pH and total soil P concentration. For predicting proportions of different P pools, soil pH, and to a lesser extent soil depth, SOC and total P were the most important predictors for all P pool proportions at the global scale. In addition, our results also revealed significant effects of climate and other edaphic factors on spatial variation in P pools. We concluded that edaphic properties and climatic factors were significant predictors of soil P pools, including concentration and proportion of total P. These findings represent a significant step towards improving understanding of global variations in different soil P pools. Our global maps of predictions of different P pools will be important to improving global models of the terrestrial P cycle.
The raw datasets, data source reference, R script, and global maps generated in this study are available at https://doi.org/10.6084/m9.figshare.16988029 (He et al., 2022).
The supplement related to this article is available online at: https://doi.org/10.5194/bg-20-4147-2023-supplement.
DSG, YW, and EH designed this study. LA, EH, and XH collected the data. XH, EH, LA, DSG, BR, YW, JH, and YH discussed the analyzing methods. XH conducted the analysis and drafted the paper. All authors discussed the results and contributed to the paper.
The contact author has declared that none of the authors has any competing interests.
Publisher's note: Copernicus Publications remains neutral with regard to jurisdictional claims in published maps and institutional affiliations.
We would like to thank Joseph Elliot at the University of Kansas for his assistance with the English language and grammatical editing of the paper.
This research has been supported by the China Postdoctoral Science Foundation (grant no. 2020M673123), the National Natural Science Foundation of China (grant no. 32271644), the ANR CLAND Convergence Institute, and the EJP SOIL ICONICA project. We were also supported by a National Science Foundation Research Coordination grant (INCyTE; grant no. DEB-1754126) to investigate nutrient cycling in terrestrial ecosystems.
This paper was edited by Aninda Mazumdar and reviewed by three anonymous referees.
Achat, D. L., Bakker, M. R., and Morel, C.: Process-Based Assessment of Phosphorus Availability in a Low Phosphorus Sorbing Forest Soil using Isotopic Dilution Methods, Soil Sci. Soc. Am. J., 73, 2131–2142, https://doi.org/10.2136/sssaj2009.0009, 2009.
Achat, D. L., Augusto, L., Bakker, M. R., Gallet-Budynek, A., and Morel, C.: Microbial processes controlling P availability in forest spodosols as affected by soil depth and soil properties, Soil Biol. Biochem., 44, 39–48, https://doi.org/10.1016/j.soilbio.2011.09.007, 2012.
Achat, D. L., Pousse, N., Nicolas, M., Brédoire, F., and Augusto, L.: Soil properties controlling inorganic phosphorus availability: general results from a national forest network and a global compilation of the literature, Biogeochemistry, 127, 255–272, https://doi.org/10.1007/s10533-015-0178-0, 2016.
Aciego Pietri, J. C. and Brookes, P. C.: Relationships between soil pH and microbial properties in a UK arable soil, Soil Biol. Biochem., 40, 1856–1861, https://doi.org/10.1016/j.soilbio.2008.03.020, 2008.
Augusto, L., Achat, D. L., Jonard, M., Vidal, D., and Ringeval, B.: Soil parent material – A major driver of plant nutrient limitations in terrestrial ecosystems, Global Change Biol., 23, 3808–3824, https://doi.org/10.1111/gcb.13691, 2017.
Barrow, N. J., Debnath, A., and Sen, A.: Measurement of the effects of pH on phosphate availability, Plant Soil, 454, 217–224, https://doi.org/10.1007/s11104-020-04647-5, 2020.
Barrow, N. J., Sen, A., Roy, N., and Debnath, A.: The soil phosphate fractionation fallacy. Plant Soil, 459, 1–11, https://doi.org/10.1007/s11104-020-04476-6, 2021.
Breiman, L.: Random Forests, Mach. Learn., 45, 5–32, https://doi.org/10.1023/A:1010933404324, 2001.
Brucker, E. and Spohn, M.: Formation of soil phosphorus fractions along a climate and vegetation gradient in the Coastal Cordillera of Chile, Catena, 180, 203–211, https://doi.org/10.1016/j.catena.2019.04.022, 2019.
Bünemann, E. K., Steinebrunner, F., Smithson, P. C., Frossard, E., and Oberson, A.: Phosphorus Dynamics in a Highly Weathered Soil as Revealed by Isotopic Labeling Techniques, Soil Sci. Soc. Am. J., 68, 1645–1655, https://doi.org/10.2136/sssaj2004.1645, 2004.
Chacon, N., Flores, S., and Gonzalez, A.: Implications of iron solubilization on soil phosphorus release in seasonally flooded forests of the lower Orinoco River, Venezuela, Soil Biol. Biochem., 38, 1494–1499, https://doi.org/10.1016/j.soilbio.2005.10.018, 2006.
Chen, C. R., Hou, E. Q., Condron, L. M., Bacon, G., Esfandbod, M., Olley, J., and Turner, B. L.: Soil phosphorus fractionation and nutrient dynamics along the Cooloola coastal dune chronosequence, southern Queensland, Australia, Geoderma, 257–258, 4–13, https://doi.org/10.1016/j.geoderma.2015.04.027, 2015.
Chen, X., Feng, J., Ding, Z., Tang, M., and Zhu, B.: Changes in soil total, microbial and enzymatic C-N-P contents and stoichiometry with depth and latitude in forest ecosystems, Sci. Total Environ., 151583, https://doi.org/10.1016/j.scitotenv.2021.151583, 2021.
Condron, L. M. and Newman, S.: Revisiting the fundamentals of phosphorus fractionation of sediments and soils, J. Soils Sediments, 11, 830–840, https://doi.org/10.1007/s11368-011-0363-2, 2011.
Crews, T. E., Kitayama, K., Fownes, J. H., Riley, R. H., Herbert, D. A., Mueller-Dombois, D., and Vitousek, P. M.: Changes in Soil Phosphorus Fractions and Ecosystem Dynamics across a Long Chronosequence in Hawaii, Ecology, 76, 1407–1424, https://doi.org/10.2307/1938144, 1995.
Cross, A. F. and Schlesinger, W. H.: A literature review and evaluation of the Hedley fractionation; applications to the biogeochemical cycle of soil phosphorus in natural ecosystems, Geoderma, 64, 197–214, https://doi.org/10.1016/0016-7061(94)00023-4, 1995.
Cunha, H. F. V., Andersen, K. M., Lugli, L. F., Santana, F. D., Aleixo, I. F., Moraes, A. M., Garcia, S., Di Ponzio, R., Mendoza, E. O., Brum, B., Rosa, J. S., Cordeiro, A. L., Portela, B. T. T., Ribeiro, G., Coelho, S. D., de Souza, S. T., Silva, L. S., Antonieto, F., Pires, M., Salomão, A. C., Miron, A. C., de Assis, R. L., Domingues, T. F., Aragão, L. E. O. C., Meir, P., Camargo, J. L., Manzi, A. O., Nagy, L., Mercado, L. M., Hartley, I. P., and Quesada, C. A.: Direct evidence for phosphorus limitation on Amazon forest productivity, Nature, 608, 558–562, https://doi.org/10.1038/s41586-022-05085-2, 2022.
Delgado-Baquerizo, M., Eldridge, D. J., Maestre, F. T., Karunaratne, S. B., Trivedi, P., Reich, P. B., and Singh, B. K.: Climate legacies drive global soil carbon stocks in terrestrial ecosystems, Sci. Adv., 3, e1602008, https://doi.org/10.1126/sciadv.1602008, 2017.
Delgado-Baquerizo, M., Reich, P. B., Bardgett, R. D., Eldridge, D. J., Lambers, H., Wardle, D. A., Reed, S. C., Plaza, C., Png, G. K., Neuhauser, S., Berhe, A. A., Hart, S. C., Hu, H., He, J., Bastida, F., Abades, S., Alfaro, F. D., Cutler, N. A., Gallardo, A., García-Velázquez, L., Hayes, P. E., Hseu, Z., Pérez, C. A., Santos, F., Siebe, C., Trivedi, P., Sullivan, B. W., Weber-Grullon, L., Williams, M. A., and Fierer, N.: The influence of soil age on ecosystem structure and function across biomes, Nat. Commun., 11, 4721–4721, https://doi.org/10.1038/s41467-020-18451-3, 2020.
De Schrijver, A., Vesterdal, L., Hansen, K., De Frenne, P., Augusto, L., Achat, D. L., Staelens, J., Baeten, L., De Keersmaeker, L., De Neve, S., and Verheyen, K.: Four decades of post-agricultural forest development have caused major redistributions of soil phosphorus fractions, Oecologia, 169, 221–234, https://doi.org/10.1007/s00442-011-2185-8, 2012.
Eivazi, F. and Tabatabai, M. A.: Phosphatases in soils, Soil Biol. Biochem., 9, 167–172, https://doi.org/10.1016/0038-0717(77)90070-0, 1977.
Ellsworth, D. S., Crous, K. Y., De Kauwe, M. G., Verryckt, L. T., Goll, D., Zaehle, S., Bloomfield, K. J., Ciais, P., Cernusak, L. A., Domingues, T. F., Dusenge, M. E., Garcia, S., Guerrieri, R., Ishida, F. Y., Janssens, I. A., Kenzo, T., Ichie, T., Medlyn, B. E., Meir, P., Norby, R. J., Reich, P. B., Rowland, L., Santiago, L. S., Sun, Y., Uddling, J., Walker, A. P., Weerasinghe, K. W. L. K., van de Weg, M. J., Zhang, Y., Zhang, J., and Wright, I. J.: Convergence in phosphorus constraints to photosynthesis in forests around the world, Nat. Commun., 13, 5005, https://doi.org/10.1038/s41467-022-32545-0, 2022.
Elser, J. J., Bracken, M. E. S., Cleland, E. E., Gruner, D. S., Harpole, W. S., Hillebrand, H., Ngai, J. T., Seabloom, E. W., Shurin, J. B., and Smith, J. E.: Global analysis of nitrogen and phosphorus limitation of primary producers in freshwater, marine and terrestrial ecosystems, Ecol. Lett., 10, 1135–1142, https://doi.org/10.1111/j.1461-0248.2007.01113.x, 2007.
Fink, J. R., Inda, A. V., Bavaresco, J., Barrón, V., Torrent, J., and Bayer, C.: Adsorption and desorption of phosphorus in subtropical soils as affected by management system and mineralogy, Soil Till. Res., 155, 62–68, https://doi.org/10.1016/j.still.2015.07.017, 2016.
Fleischer, K., Rammig, A., Kauwe, D. M. G., Walker, A. P., Domingues, T. F., Fuchslueger, L., Garcia, S., Goll, D. S., Grandis, A., Jiang, M., Haverd, V., Hofhansl, F., Holm, J. A., Kruijt, B., Leung, F., Medlyn, B. E., Mercado, L. M., Norby, R. J., Pak, B., Randow, V. C., Quesada, C. A., Schaap, K. J., Valverde-Barrantes, O. J., Wang, Y. P., Yang, X., Zaehle, S., Zhu, Q., Lapola, D. M., and Oak Ridge National Lab. ORNL: Amazon forest response to CO2 fertilization dependent on plant phosphorus acquisition, Nat. Geosci., 12, 736–741, https://doi.org/10.1038/s41561-019-0404-9, 2019.
Gerke, J.: The acquisition of phosphate by higher plants: Effect of carboxylate release by the roots. A critical review, J. Plant Nutr. Soil Sci., 178, 351–364, https://doi.org/10.1002/jpln.201400590, 2015.
Goll, D. S., Brovkin, V., Parida, B. R., Reick, C. H., Kattge, J., Reich, P. B., van Bodegom, P. M., and Niinemets, Ü.: Nutrient limitation reduces land carbon uptake in simulations with a model of combined carbon, nitrogen and phosphorus cycling, Biogeosciences, 9, 3547–3569, https://doi.org/10.5194/bg-9-3547-2012, 2012.
Gu, C. and Margenot, A. J.: Navigating limitations and opportunities of soil phosphorus fractionation, Plant Soil, 459, 13–17, https://doi.org/10.1007/s11104-020-04552-x, 2021.
Harrison, A. F.: Soil Organic Phosphorus. A Review of World Literature, CAB International, Wallingford, https://api.semanticscholar.org/CorpusID:126657539 (last access: 8 October 2023), 1987.
He, X., Augusto, L., Goll, D. S., Ringeval, B., Wang, Y., Helfenstein, J., Huang, Y., Yu, K., Wang, Z., Yang, Y., and Hou, E.: Global patterns and drivers of soil total phosphorus concentration, Earth Syst. Sci. Data, 13, 5831–5846, https://doi.org/10.5194/essd-13-5831-2021, 2021.
He, X., Augusto, L., Goll, D. S., Ringeval, B., Wang, Y.-P., Helfenstein, J., Huang, Y., and Hou, E.: Global patterns and drivers of phosphorus fractions in natural soils, figshare [data set], https://doi.org/10.6084/m9.figshare.16988029, 2022.
Hedley, M. J., Stewart, J. W. B., and Chauhan, B. S.: Changes in Inorganic and Organic Soil Phosphorus Fractions Induced by Cultivation Practices and by Laboratory Incubations, Soil Sci. Soc. Am. J., 46, 970–976, https://doi.org/10.2136/sssaj1982.03615995004600050017x, 1982.
Helfenstein, J., Tamburini, F., Von Sperber, C., Massey, M. S., Pistocchi, C., Chadwick, O. A., Vitousek, P. M., Kretzschmar, R., and Frossard, E.: Combining spectroscopic and isotopic techniques gives a dynamic view of phosphorus cycling in soil, Nat. Commun., 9, 3226, https://doi.org/10.1038/s41467-018-05731-2, 2018.
Helfenstein, J., Pistocchi, C., Oberson, A., Tamburini, F., Goll, D. S., and Frossard, E.: Estimates of mean residence times of phosphorus in commonly considered inorganic soil phosphorus pools, Biogeosciences, 17, 441–454, https://doi.org/10.5194/bg-17-441-2020, 2020.
Helfenstein, J., Frossard, E., Pistocchi, C., Chadwick, O., Vitousek, P., and Tamburini, F.: Soil Phosphorus Exchange as Affected by Drying-Rewetting of Three Soils From a Hawaiian Climatic Gradient, Front. Soil Sci., 1, 738464, https://doi.org/10.3389/fsoil.2021.738464, 2021.
Hengl, T., Mendes, D. J. J., Heuvelink, G. B., Ruiperez, G. M., Kilibarda, M., Blagotic, A., Shangguan, W., Wright, M. N., Geng, X., Bauer-Marschallinger, B., Guevara, M. A., Vargas, R., MacMillan, R. A., Batjes, N. H., Leenaars, J. G., Ribeiro, E., Wheeler, I., Mantel, S., and Kempen, B.: SoilGrids250m: Global gridded soil information based on machine learning, Plos One, 12, e0169748, https://doi.org/10.1371/journal.pone.0169748, 2017.
Hengl, T., Walsh, M. G., Sanderman, J., Wheeler, I., Harrison, S. P., and Prentice, I. C.: Global mapping of potential natural vegetation: an assessment of machine learning algorithms for estimating land potential, Peer J., 6, e5457, https://doi.org/10.7717/peerj.5457, 2018.
Hou, E., Chen, C., Kuang, Y., Zhang, Y., Heenan, M., and Wen, D.: A structural equation model analysis of phosphorus transformations in global unfertilized and uncultivated soils, Global Biogeochem. Cy., 30, 1300–1309, https://doi.org/10.1002/2016GB005371, 2016.
Hou, E., Chen, C., Luo, Y., Zhou, G., Kuang, Y., Zhang, Y., Heenan, M., Lu, X., and Wen, D.: Effects of climate on soil phosphorus cycle and availability in natural terrestrial ecosystems, Global Change Biol., 24, 3344–3356, https://doi.org/10.1111/gcb.14093, 2018a.
Hou, E., Tan, X., Heenan, M., and Wen, D.: A global dataset of plant available and unavailable phosphorus in natural soils derived by Hedley method, Sci. Data, 5, 180166, https://doi.org/10.1038/sdata.2018.166, 2018b.
Hou, E., Wen, D., Kuang, Y., Cong, J., Chen, C., He, X., Heenan, M., Lu, H., and Zhang, Y.: Soil pH predominantly controls the forms of organic phosphorus in topsoils under natural broadleaved forests along a 2500 km latitudinal gradient, Geoderma, 315, 65–74, https://doi.org/10.1016/j.geoderma.2017.11.041, 2018c.
Hou, E., Luo, Y., Kuang, Y., Chen, C., Lu, X., Jiang, L., Luo, X., and Wen, D.: Global meta-analysis shows pervasive phosphorus limitation of aboveground plant production in natural terrestrial ecosystems, Nat. Commun., 11, 637, https://doi.org/10.1038/s41467-020-14492-w, 2020.
Hou, E., Wen, D., Jiang, L., Luo, X., Kuang, Y., Lu, X., Chen, C., Allen, K. T., He, X., Huang, X., and Luo, Y.: Latitudinal patterns of terrestrial phosphorus limitation over the globe, Ecol. Lett., 24, 1420–1431, https://doi.org/10.1111/ele.13761, 2021.
James, G., Witten, D., Hastie, T., and Tibshirani, R.: An Introduction to Statistical Learning, Springer New York, New York, NY, https://doi.org/10.1007/978-1-4614-7138-7, 2013.
Jobbágy, E. G. and Jackson, R. B.: The Distribution of Soil Nutrients with Depth: Global Patterns and the Imprint of Plants, Biogeochemistry, 53, 51–77, https://doi.org/10.1023/A:1010760720215, 2001.
Jones, Z. M. and Linder, F. J.: edarf: Exploratory Data Analysis using Random Forests, J. Open Sour. Softw., 1, 92, https://doi.org/10.21105/joss.00092, 2016.
Klotzbücher, A., Kaiser, K., Klotzbücher, T., Wolff, M., and Mikutta, R.: Testing mechanisms underlying the Hedley sequential phosphorus extraction of soils, J. Plant Nutr. Soil Sci., 182, 570–577, https://doi.org/10.1002/jpln.201800652, 2019.
Kruse, J., Abraham, M., Amelung, W., Baum, C., Bol, R., Kühn, O., Lewandowski, H., Niederberger, J., Oelmann, Y., Rüger, C., Santner, J., Siebers, M., Siebers, N., Spohn, M., Vestergren, J., Vogts, A., and Leinweber, P.: Innovative methods in soil phosphorus research: A review, J. Plant Nutr. Soil Sci., 178, 43–88, https://doi.org/10.1002/jpln.201400327, 2015.
Kuhn, M.: caret: Classification and Regression Training, R package version 6.0-86, GitHub [code], https://github.com/topepo/caret/ (last access: 8 October 2023), 2020.
Lang, F., Bauhus, J., Frossard, E., George, E., Kaiser, K., Kaupenjohann, M., Krüger, J., Matzner, E., Polle, A., Prietzel, J., Rennenberg, H., and Wellbrock, N.: Phosphorus in forest ecosystems: New insights from an ecosystem nutrition perspective, J. Plant Nutr. Soil Sci., 179, 129–135, https://doi.org/10.1002/jpln.201500541, 2016.
Lang, F., Krüger, J., Amelung, W., Willbold, S., Frossard, E., Bünemann, E. K., Bauhus, J., Nitschke, R., Kandeler, E., Marhan, S., Schulz, S., Bergkemper, F., Schloter, M., Luster, J., Guggisberg, F., Kaiser, K., Mikutta, R., Guggenberger, G., Polle, A., Pena, R., Prietzel, J., Rodionov, A., Talkner, U., Meesenburg, H., von Wilpert, K., Hölscher, A., Dietrich, H. P., and Chmara, I.: Soil phosphorus supply controls P nutrition strategies of beech forest ecosystems in Central Europe, Biogeochemistry, 136, 5–29, https://doi.org/10.1007/s10533-017-0375-0, 2017.
Liaw, A. and Wiener, M.: Classification and Regression by randomForest, R News, 2, 18–22, 2002.
Liptzin, D. and Silver, W. L.: Effects of carbon additions on iron reduction and phosphorus availability in a humid tropical forest soil, Soil Biol. Biochem., 41, 1696–1702, https://doi.org/10.1016/j.soilbio.2009.05.013, 2009.
Maharjan, M., Maranguit, D., and Kuzyakov, Y.: Phosphorus fractions in subtropical soils depending on land use, Eur. J. Soil Biol., 87, 17–24, https://doi.org/10.1016/j.ejsobi.2018.04.002, 2018.
Mahowald, N., Jickells, T. D., Baker, A. R., Artaxo, P., Benitez-Nelson, C. R., Bergametti, G., Bond, T. C., Chen, Y., Cohen, D. D., Herut, B., Kubilay, N., Losno, R., Luo, C., Maenhaut, W., McGee, K. A., Okin, G. S., Siefert, R. L., and Tsukuda, S.: Global distribution of atmospheric phosphorus sources, concentrations and deposition rates, and anthropogenic impacts, Global Biogeochem. Cy., 22, GB4026, https://doi.org/10.1029/2008GB003240, 2008.
Marklein, A. R. and Houlton, B. Z.: Nitrogen inputs accelerate phosphorus cycling rates across a wide variety of terrestrial ecosystems, New Phytol., 193, 696–704, https://doi.org/10.1111/j.1469-8137.2011.03967.x, 2012.
Morel, C., Ziadi, N., Messiga, A., Bélanger, G., Denoroy, P., Jeangros, B., Jouany, C., Fardeau, J., Mollier, A., Parent, L., Proix, N., Rabeharisoa, L., and Sinaj, S.: Modeling of phosphorus dynamics in contrasting agroecosystems using long-term field experiments, Can. J. Soil Sci., 94, 377–387, https://doi.org/10.4141/cjss2013-024, 2014.
Oburger, E., Jones, D. L., and Wenzel, W. W.: Phosphorus saturation and pH differentially regulate the efficiency of organic acid anion-mediated P solubilization mechanisms in soil, Plant Soil, 341, 363–382, https://doi.org/10.1007/s11104-010-0650-5, 2011.
Peltzer, D. A., Wardle, D. A., Allison, V. J., Baisden, W. T., Bardgett, R. D., Chadwick, O. A., Condron, L. M., Parfitt, R. L., Porder, S., Richardson, S. J., Turner, B. L., Vitousek, P. M., Walker, J., and Walker, L. R.: Understanding ecosystem retrogression, Ecol. Monogr., 80, 509–529, https://doi.org/10.1890/09-1552.1, 2010.
Porder, S. and Chadwick, O. A.: Climate and Soil-Age Constraints on Nutrient Uplift and Retention by Plants, Ecology, 90, 623–636, https://doi.org/10.1890/07-1739.1, 2009.
Porder, S. and Ramachandran, S.: The phosphorus concentration of common rocks – a potential driver of ecosystem P status, Plant Soil, 367, 41–55, https://doi.org/10.1007/s11104-012-1490-2, 2013.
Quesada, C. A., Lloyd, J., Schwarz, M., Patiño, S., Baker, T. R., Czimczik, C., Fyllas, N. M., Martinelli, L., Nardoto, G. B., Schmerler, J., Santos, A. J. B., Hodnett, M. G., Herrera, R., Luizão, F. J., Arneth, A., Lloyd, G., Dezzeo, N., Hilke, I., Kuhlmann, I., Raessler, M., Brand, W. A., Geilmann, H., Moraes Filho, J. O., Carvalho, F. P., Araujo Filho, R. N., Chaves, J. E., Cruz Junior, O. F., Pimentel, T. P., and Paiva, R.: Variations in chemical and physical properties of Amazon forest soils in relation to their genesis, Biogeosciences, 7, 1515–1541, https://doi.org/10.5194/bg-7-1515-2010, 2010.
R Core Team: R: A language and environment for statistical computing, R Foundation for Statistical Computing, Vienna, Austria, https://www.R-project.org/ (last access: 8 October 2023), 2018.
Ringeval, B., Augusto, L., Monod, H., van Apeldoorn, D., Bouwman, L., Yang, X., Achat, D. L., Chini, L. P., Van Oost, K., Guenet, B., Wang, R., Decharme, B., Nesme, T., and Pellerin, S.: Phosphorus in agricultural soils: drivers of its distribution at the global scale, Global Change Biol., 23, 3418–3432, https://doi.org/10.1111/gcb.13618, 2017.
Selmants, P. C. and Hart, S. C.: Phosphorus and soil development: Does the Walker and Syers model apply to semiarid ecosystems?, Ecology, 91, 474–484, https://doi.org/10.1890/09-0243.1, 2010.
Slessarev, E. W., Lin, Y., Bingham, N. L., Johnson, J. E., Dai, Y., Schimel, J. P., and Chadwick, O. A.: Water balance creates a threshold in soil pH at the global scale, Nature, 540, 567–569, https://doi.org/10.1038/nature20139, 2016.
Smeck, N. E.: Phosphorus dynamics in soils and landscapes, Geoderma, 36, 185–199, https://doi.org/10.1016/0016-7061(85)90001-1, 1985.
Strobl, C., Boulesteix, A., Kneib, T., Augustin, T., and Zeileis, A.: Conditional variable importance for random forests, Bmc Bioinform., 9, 307, https://doi.org/10.1186/1471-2105-9-307, 2008.
Sun, Y., Peng, S., Goll, D. S., Ciais, P., Guenet, B., Guimberteau, M., Hinsinger, P., Janssens, I. A., Peñuelas, J., Piao, S., Poulter, B., Violette, A., Yang, X., Yin, Y., and Zeng, H.: Diagnosing phosphorus limitations in natural terrestrial ecosystems in carbon cycle models, Earth's Future, 5, 730–749, https://doi.org/10.1002/2016EF000472, 2017.
Tiessen, H. and Moir, J.: Characterization of available P by sequential extraction, in: Soil Sampling and Methods of Analysis, edited by: Carter, M. R., Lewis Publishers, 75–86, https://doi.org/10.1201/9781420005271, 1993.
Turner, B. L. and Blackwell, M. S. A.: Isolating the influence of pH on the amounts and forms of soil organic phosphorus, Eur. J. Soil Sci., 64, 249–259, https://doi.org/10.1111/ejss.12026, 2013.
Vitousek, P. M.: Litterfall, Nutrient Cycling, and Nutrient Limitation in Tropical Forests, Ecology, 65, 285–298, https://doi.org/10.2307/1939481, 1984.
Vitousek, P. M., Porder, S., Houlton, B. Z., and Chadwick, O. A.: Terrestrial phosphorus limitation: mechanisms, implications, and nitrogen–phosphorus interactions, Ecol. Appl., 20, 5–15, https://doi.org/10.1890/08-0127.1, 2010.
Vogel, C., Helfenstein, J., Massey, M. S., Sekine, R., Kretzschmar, R., Beiping, L., Peter, T., Chadwick, O. A., Tamburini, F., Rivard, C., Herzel, H., Adam, C., Pradas Del Real, A. E., Castillo-Michel, H., Zuin, L., Wang, D., Félix, R., Lassalle-Kaiser, B., and Frossard, E.: Microspectroscopy reveals dust-derived apatite grains in acidic, highly-weathered Hawaiian soils, Geoderma, 381, 114681, https://doi.org/10.1016/j.geoderma.2020.114681, 2021.
Vu, D. T., Tang, C., and Armstrong, R. D.: Transformations and availability of phosphorus in three contrasting soil types from native and farming systems: A study using fractionation and isotopic labeling techniques, J. Soils Sediments, 10, 18–29, https://doi.org/10.1007/s11368-009-0068-y, 2010.
Wager, S., Hastie, T., and Efron, B.: Confidence Intervals for Random Forests: The Jackknife and the Infinitesimal Jackknife, J. Mach. Learn. Res., 15, 1625–1651,2014.
Walker, T. W. and Syers, J. K.: The fate of phosphorus during pedogenesis, Geoderma, 15, 1–19, https://doi.org/10.1016/0016-7061(76)90066-5, 1976.
Wang, R., Balkanski, Y., Boucher, O., Ciais, P., Peñuelas, J., and Tao, S.: Significant contribution of combustion-related emissions to the atmospheric phosphorus budget, Nat. Geosci., 8, 48–54, https://doi.org/10.1038/ngeo2324, 2015.
Wang, Y., Ciais, P., Goll, D., Huang, Y., Luo, Y., Wang, Y., Bloom, A. A., Broquet, G., Hartmann, J., Peng, S., Penuelas, J., Piao, S., Sardans, J., Stocker, B. D., Wang, R., Zaehle, S., and Zechmeister-Boltenstern, S.: GOLUM-CNP v1.0: a data-driven modeling of carbon, nitrogen and phosphorus cycles in major terrestrial biomes, Geosci. Model Dev., 11, 3903–3928, https://doi.org/10.5194/gmd-11-3903-2018, 2018.
Wang, Y. P., Houlton, B. Z., and Field, C. B.: A model of biogeochemical cycles of carbon, nitrogen, and phosphorus including symbiotic nitrogen fixation and phosphatase production, Global Biogeochem. Cy., 21, GB1018, https://doi.org/10.1029/2006GB002797, 2007.
Wang, Y. P., Law, R. M., and Pak, B.: A global model of carbon, nitrogen and phosphorus cycles for the terrestrial biosphere, Biogeosciences, 7, 2261–2282, https://doi.org/10.5194/bg-7-2261-2010, 2010.
Wang, Y. P., Huang, Y., Augusto, L., Goll, D. S., Helfenstein, J., and Hou, E.: Toward a Global Model for Soil Inorganic Phosphorus Dynamics: Dependence of Exchange Kinetics and Soil Bioavailability on Soil Physicochemical Properties, Global Biogeochem. Cy., 36, e2021GB007061, https://doi.org/10.1029/2021GB007061, 2022.
Wardle, D. A., Walker, L. R., Bardgett, R. D., and Sveriges, L.: Ecosystem Properties and Forest Decline in Contrasting Long-Term Chronosequences, Science, 305, 509–513, https://doi.org/10.1126/science.1098778, 2004.
Weihrauch, C. and Opp, C.: Ecologically relevant phosphorus pools in soils and their dynamics: The story so far, Geoderma, 325, 183–194, https://doi.org/10.1016/j.geoderma.2018.02.047, 2018.
Wright, M. N. and Ziegler, A.: ranger: A Fast Implementation of Random Forests for High Dimensional Data in C and R, J. Stat. Softw., 77, 1–17, https://doi.org/10.18637/jss.v077.i01, 2017.
Xu, X., Schimel, J. P., Janssens, I. A., Song, X., Song, C., Yu, G., Sinsabaugh, R. L., Tang, D., Zhang, X., and Thornton, P. E.: Global pattern and controls of soil microbial metabolic quotient, Ecol. Monogr., 87, 429–441, https://doi.org/10.1002/ecm.1258, 2017.
Yang, X. and Post, W. M.: Phosphorus transformations as a function of pedogenesis: A synthesis of soil phosphorus data using Hedley fractionation method, Biogeosciences, 8, 2907–2916, https://doi.org/10.5194/bg-8-2907-2011, 2011.
Yang, X., Post, W. M., Thornton, P. E., and Jain, A.: The distribution of soil phosphorus for global biogeochemical modeling, Biogeosciences, 10, 2525–2537, https://doi.org/10.5194/bg-10-2525-2013, 2013.
Yang, X., Thornton, P. E., Ricciuto, D. M., and Post, W. M.: The role of phosphorus dynamics in tropical forests – a modeling study using CLM-CNP, Biogeosciences, 11, 1667–1681, https://doi.org/10.5194/bg-11-1667-2014, 2014.
Zhang, Y., Guo, Y., Tang, Z., Feng, Y., Zhu, X., Xu, W., Bai, Y., Zhou, G., Xie, Z., and Fang, J.: Patterns of nitrogen and phosphorus pools in terrestrial ecosystems in China, Earth Syst. Sci. Data, 13, 5337–5351, https://doi.org/10.5194/essd-13-5337-2021, 2021.