the Creative Commons Attribution 4.0 License.
the Creative Commons Attribution 4.0 License.
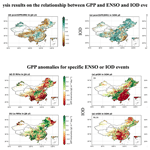
Distinct impacts of the El Niño–Southern Oscillation and Indian Ocean Dipole on China's gross primary production
Ran Yan
Weimin Ju
Xiuli Xing
Meirong Wang
Jingye Tan
Xunmei Wang
Hengmao Wang
Fei Jiang
Gross primary production (GPP), a crucial component in the terrestrial carbon cycle, is strongly influenced by large-scale circulation patterns. This study explores the influence of the El Niño–Southern Oscillation (ENSO) and Indian Ocean Dipole (IOD) on China's GPP, utilizing long-term GPP data generated by the Boreal Ecosystem Productivity Simulator (BEPS). Partial correlation coefficients between GPP and ENSO reveal substantial negative associations in most parts of western and northern China during the September–October–November (SON) period of ENSO development. These correlations shift to strongly positive over southern China in December–January–February (DJF) and then weaken in March–April–May (MAM) in the following year, eventually turning generally negative over southwestern and northeastern China in June–July–August (JJA). In contrast, the relationship between GPP and IOD basically exhibits opposite seasonal patterns. Composite analysis further confirms these seasonal GPP anomalous patterns. Mechanistically, these variations are predominantly controlled by soil moisture during ENSO events (except MAM) and by temperature during IOD events (except SON). Quantitatively, China's annual GPP demonstrates modest positive anomalies in La Niña and negative IOD years, in contrast to minor negative anomalies in El Niño and positive IOD years. This outcome is due to counterbalancing effects, with significantly larger GPP anomalies occurring in DJF and JJA. Additionally, the relative changes in total GPP anomalies at the provincial scale display an east–west pattern in annual variation, while the influence of IOD events on GPP presents an opposing north–south pattern. We believe that this study can significantly enhance our understanding of specific processes by which large-scale circulation influences climate conditions and, in turn, affects China's GPP.
- Article
(9330 KB) - Full-text XML
-
Supplement
(5006 KB) - BibTeX
- EndNote
Vegetation photosynthesis, a pivotal physiological process affecting the terrestrial carbon cycle, predominantly governs variations in the net biome productivity (NBP), surpassing the impact of total ecosystem respiration (Piao et al., 2020; Wang et al., 2022, 2018). Gross primary production (GPP) represents the total amount of carbon dioxide assimilated by plants per unit time through the photosynthetic processes, acting as a crucial carbon flux in mitigating anthropogenic CO2 emissions (Gough, 2011; Houghton, 2007). However, despite evident long-term increasing trends in GPP, primarily attributed to CO2 fertilization (Ryu et al., 2019; Schimel et al., 2015; Yang et al., 2022), it also shows regional and global interannual variations. These variations are largely linked to climate fluctuations driven by ocean–atmosphere interactions and the teleconnections (Wang et al., 2021b; Ying et al., 2022). To date, the impact of such teleconnections on China's GPP remains insufficiently documented.
The El Niño–Southern Oscillation (ENSO) exerts a significant influence on the global terrestrial carbon cycle, which is the dominant mode of interannual climate variability (Bauch, 2020; Kim et al., 2017; Wang et al., 2016, 2018; Zeng et al., 2005). Within this context, GPP typically assumes a leading role in shaping the response of terrestrial carbon sinks to ENSO events (Ahlstrom et al., 2015; Wang et al., 2018; Zhang et al., 2018). Global patterns reveal a negative GPP anomaly of approximately −1.08 Pg C yr−1 during El Niño years, contrasting a positive GPP anomaly of about 1.63 Pg C yr−1 in La Niña years (Zhang et al., 2019). However, the impact of ENSO on GPP exhibits significant regional differences. At present, while existing research studies have predominantly focused on the response of tropical GPP to ENSO, studies specific to China are relatively limited. Liu et al. (2014) highlighted the effects of ENSO on crop growth in North China, and Li et al. (2021) demonstrated that the response of GPP to El Niño varies with the phase of the Pacific Decadal Oscillation (PDO) in the eastern China.
ENSO is not the sole global climatic oscillation influencing the terrestrial carbon cycle. Another significant player is the Indian Ocean Dipole (IOD), a tropical coupled ocean–atmosphere mode (Saji et al., 1999), which also affects the terrestrial carbon cycling by modulating the climate circulations (Wang et al., 2022, 2020, 2021b; Yan et al., 2023). Research indicates that IOD events can influence precipitation in China, with effects lasting from the year of the event through the subsequent summer (Zhang et al., 2022a). Zhang et al. (2022b) also proved that extreme positive IOD (pIOD) events in 2019 affected the precipitation in summer 2020 in eastern China and proposed that the summer precipitation in the following year was mainly affected by IOD in northern China, while it was affected by ENSO in the Yangtze River basin. Additionally, a prior study explored the influence of the extreme pIOD event in 2019 on GPP anomalies across the Indian Ocean rim countries. It suggested a conspicuous negative GPP anomaly occurred in eastern China during the September–October–November (SON) (Wang et al., 2021b).
The primary objective of this study was to comprehensively assess the impact of ENSO and IOD events on GPP in China. To this end, we initially employed partial correlation analysis to elucidate the relationship between GPP and climate anomalies, specifically soil moisture and temperature, induced by ENSO and IOD events across various seasons. The analysis utilized historical long-term GPP data spanning from 1981 to 2021, simulated by the Boreal Ecosystem Productivity Simulator (BEPS) model. The aim was to get a preliminary understanding of the influence exerted by ENSO and IOD. Furthermore, composite analysis was adopted to illustrate the actual responses during distinct events, including individual ENSO and IOD occurrences. The ensuing discussion will delve into the analysis results on national, regional, and provincial scales.
2.1 Datasets used
The sea surface temperature (SST) dataset was derived from the NOAA's monthly Extended Reconstructed Sea Surface Temperature version 5 (ERSSTv5) (Huang et al., 2017). It is generated on a 2° × 2° grid, using statistical methods to enhance spatial completeness. Commencing from January 1854 to the present, the monthly SST data include anomalies computed with respect to a 1971–2000 monthly climatology.
Meteorological data were adopted from ECMWF Reanalysis v5 (ERA5)-Land monthly averaged data with 0.1° × 0.1° grids, including 2 m surface air temperature (TAS) and volumetric soil moisture (SM) during the period from 1981 to 2021 (Muñoz, 2019). ERA5-Land was created by replaying the land component of the ECMWF ERA5 climate reanalysis at a higher resolution compared to ERA5. The reanalysis combines model data with global observations into a consistent dataset based on the laws of physics. The original soil moisture data were divided into four layers based on different surface depths. These layers were depth-weighted and then aggregated into the average soil moisture to a depth of 289 cm (m3 m−3).
GPP spanning from 1981 to 2021 was simulated by the BEPS model, featuring a horizontal resolution of 0.0727° × 0.0727°. The BEPS model, originally developed for Canadian boreal ecosystems, has been reconstructed for GPP simulations on the global scale (Chen et al., 1999; Chen et al., 2012). BEPS is a process-based model driven by the satellite-observed leaf area index (LAI) and foliage clumping index (Ω), meteorological data, land cover types, soil texture, and CO2 concentration to simulate the daily carbon flux of terrestrial ecosystems (Chen et al., 2019; Liu et al., 1997). The input data used to drive GPP in this study include the ERA5 meteorological data (Hersbach et al., 2023), GLOBMAP LAI product (Liu et al., 2012), Land Cover Classification System (LCCS) generated by the Food and Agriculture Organization (FAO) of the United Nations (Friedl and Sulla-Menashe, 2019), Harmonized World Soil Database v1.2 from FAO (Fischer et al., 2008), and CO2 concentration based on the Global Monitoring Laboratory from NASA (Lan et al., 2022).
Notably, BEPS distinguishes itself from other models through the organic combination of remote sensing data and mechanistic modeling. It produces simulation datasets for GPP, net primary productivity (NPP), and evapotranspiration (ET). Key features of BEPS include the incorporation of a sunlit–shaded leaf stratification strategy (Norman, 1982). The model calculates canopy-level photosynthesis by summing the GPP of sunlit and shaded leaves (Chen et al., 1999).
where Asun and Ashade represent the amount of photosynthesis at per sunlit and shaded leaf, respectively; LAIsun and LAIshade represent the canopy-level sunlit and shaded LAI, respectively; Ω is the foliage clumping index indicating the influence of foliage clustering on radiation transmission; and θ is the solar zenith angle.
The accuracy of carbon flux products simulated by BEPS has been validated in previous studies (Chen et al., 2019; He et al., 2021). We also used the measured site data from ChinaFLUX (https://chinaflux.org/, last access: October 2022; Yu et al., 2016) and the National Tibetan Plateau/Third Pole Environment Data Center (Li et al., 2013) (Table S1 in the Supplement) to assess the performance of BEPS-simulated GPP (Fig. S1 in the Supplement). Our analysis reveals a high consistency between simulated and observed GPP, with an average R2 of 0.77 (p < 0.05) and an average root mean square error (RMSE) of 1.70 . In addition, the global terrestrial GPP from FluxSat product version 2.2 (Joiner et al., 2018) was also used to assess the reliability of BEPS GPP. FluxSat GPP is obtained by using the light-use efficiency (LUE) framework based on Moderate Resolution Imaging Spectroradiometer (MODIS) satellite data, eliminating the dependency on other meteorological input data. The comparison between BEPS GPP and FluxSat GPP data revealed a robust agreement, with a correlation coefficient (r) of 0.63 (p < 0.05) and a RMSE of 1.1 Pg C yr−1 (Fig. S2). These consistencies underscore the reliability of the BEPS GPP data in capturing terrestrial carbon flux dynamics.
2.2 Anomaly calculation
To calculate anomalies, we first removed the long-term climatology to eliminate the seasonal cycle. Subsequently, we subtracted the 7-year running average for each grid to eliminate the decadal oscillation and long-term trends for all the variables. Further, refinement involved smoothing the derived GPP and climate anomalies using a 3-month running average to remove the intra-seasonal variability. For consistency, the BEPS-simulated GPP data were resampled to 0.1° × 0.1°. To align with this, non-vegetated areas in the climate data were masked according to the resampled BEPS GPP, ensuring uniformity in spatial representation.
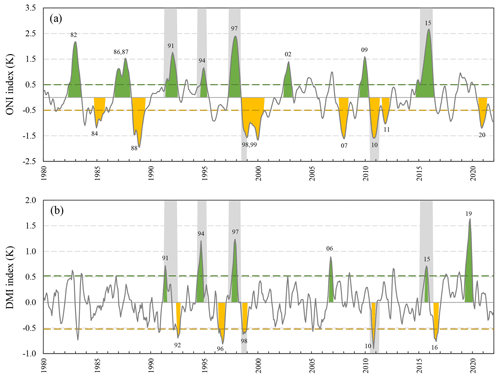
Figure 1Time series of the Oceanic Niño Index (ONI) (a) and the Dipole Mode Index (DMI) (b) from 1980 to 2022. The positive phase events (El Niño and positive Indian Ocean Dipole, pIOD) are filled in green, and the negative phase events (La Niña and negative IOD, nIOD) are filled in yellow, and the events are also labeled with a two-digit year. The dashed green and dashed yellow lines represent the positive and negative thresholds for the El Niño–Southern Oscillation (ENSO) and IOD, respectively. The gray background indicates years with the simultaneous ENSO and IOD events.
2.3 Definition of climate events
The Oceanic Niño Index (ONI) is used to define ENSO events (Fig. 1a) and represents the 3-month running mean SST anomaly in the Niño 3.4 region (5° N–5° S, 120–170° W; https://www.cpc.ncep.noaa.gov/products/analysis_monitoring/ensostuff/nino_regions.shtml, last access: October 2022). The positive phase of an ENSO event (El Niño) is characterized by the ONI exceeding +0.5 K for five consecutive overlapping 3-month periods. Conversely, the negative phase of an ENSO event (La Niña) occurs when the ONI is below −0.5 K for five consecutive overlapping 3-month periods. The severity of the event can be further categorized into weak (0.5–0.99), moderate (1.00–1.49), strong (1.50–1.99), and extremely strong (≥ 2.00) based on the absolute value of the ONI. To qualify for a specific rating, an event should meet or exceed a threshold for at least three consecutive overlapping 3-month periods.
Moreover, the Dipole Mode Index (DMI) is employed to identify IOD events (Saji et al., 1999). The DMI is calculated from SST differences between the western equatorial Indian Ocean (10° S–10° N, 50–70° E) and the southeastern equatorial Indian Ocean (10° S–0° N, 90–110° E) (Fig. 1b). Given the short duration of IOD events with a tendency to peak during SON, the standard deviation of SON DMI (0.52 K from 1981 to 2021) is used as the criterion for identifying IOD events. A positive phase IOD (pIOD) event is defined when the absolute value of DMI is greater than or equal to 1 standard deviation (0.52 K) for three consecutive 3-month periods. Additionally, a strong pIOD event is identified if the DMI value exceeds 2 standard deviations (1.04 K).
2.4 Partial correlation analysis
To comprehensively assess the impacts of ENSO and IOD on GPP, while accounting for the influence of other events, partial correlation analysis (pcor) was employed, following the previous studies (Saji and Yamagata, 2003; Wang et al., 2021b). The definition of pcor for x and y, controlling for z, is given by
where ryx is the correlation of the dependent variable y and the explanatory variable x (e.g., DMI), and the same is true for ryz and ryx. The two-tailed Student t test was used to calculate the statistical significance of each pixel result:
where n and k are the number of samples and conditioned variables, respectively.
2.5 Composite analysis
When enumerating the years of ENSO and IOD events, we retained all the years of IOD events and ENSO events above the moderate intensity. Individual events and compound events were categorized and summarized in Table 1. In this study, a compound event refers to the simultaneous occurrence of ENSO and IOD, primarily El Niño and pIOD and La Niña and negative IOD (nIOD). IOD typically peaked in September–October–November (SON, year 0), while ENSO peaked in December(year 0)–January(year 1)–February(year 1) (DJF), and the influence of the two events could extend until the summer of the following year. Therefore, we selected four seasons from SON to June–July–August (JJA) in the following year for composite analysis in this study. In addition, the year 1991 was excluded due to the strong eruption of Mount Pinatubo, which had a large impact on the global carbon cycle (Mercado et al., 2009).
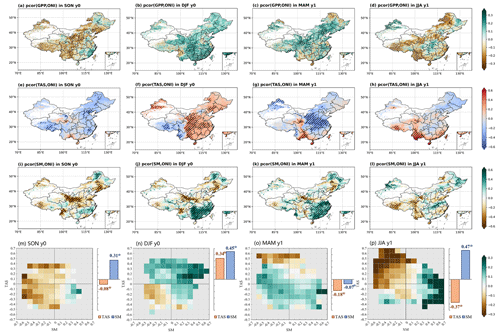
Figure 2Spatial patterns of partial correlation coefficients (pcor) between ONI and gross primary productivity (GPP) (a–d), surface air temperature (TAS) (e–h), and soil moisture (SM) (i–l) in different seasons, controlling for the effect of DMI. Hatched areas represent significance at p≤ 0.05 based on the two-tailed Student t test. (m–p) Heatmaps represent the relationships of the pcor patterns among GPP, TAS, and SM, and bar charts illustrate the pattern correlations of these pcor values between GPP and TAS and SM on the national scale for each season. We here use seasonal average temperature as a mask to exclude regions with temperatures below zero, thereby minimizing the influence of phenology on GPP. Notably, asterisks (∗) in the bar charts denote significance at p < 0.05.
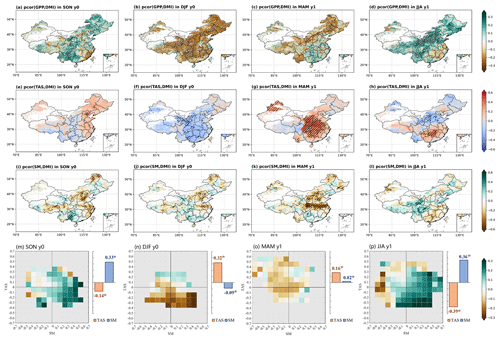
Figure 3Same as Fig. 2 but for DMI, controlling for the effect of ONI.
3.1 Historical relationship between GPP and ENSO
We analyzed the pcor patterns between GPP and climate anomalies across different events using long time series data (Figs. 2 and 3). Following this, we calculated pattern correlation coefficients between the GPP and climate pcor patterns, aiming to investigate the varying impacts of key climate drivers (TAS and SM) on photosynthesis across different seasons (Figs. 2m–p and 3m–p).
Figure 2 reveals notable seasonal variations in the pcor patterns between GPP, related climate anomalies, and ONI in December–January–February (DJF) when ENSO peaked, controlling for the effect of DMI in September–October–November (SON) when IOD peaked. During SON, significant negative pcor between GPP and ONI is observed in regions including the Tibetan Plateau, southwestern China, the Loess Plateau, and Liaoning province (Fig. 2a). Clearly, this pattern aligns closely with the pcor pattern between soil moisture and ONI (Fig. 2a and i). The pattern correlation analysis between GPP and both TAS and SM underscores the dominance of SM in influencing GPP anomalies, indicated by a correlation coefficient of 0.31 (p < 0.05). This finding suggests that the soil moisture deficit induced by El Niño largely inhibits vegetation photosynthesis during this season (Fig. 2m).
Along with the peak of ENSO events in DJF, the pcor pattern between GPP and ONI exhibits a distinct shift from the pattern in SON. Notably, DJF showcases significant positive pcor values over large areas in southern China and weak positive pcor in North and Northeast China (Fig. 2b). During this period, soil moisture still serves as a more influential factor in driving GPP changes, reflected in a nationwide pattern correlation coefficient of 0.45 (p < 0.05) (Fig. 2n). Specifically, sufficient soil moisture during El Niño, coupled with higher winter temperatures, contributes to a substantial enhancement in GPP across southern China. In contrast, the impact is weaker in North and Northeast China due to the vegetation being in the non-growing season and localized soil water deficits (Fig. 2b, f, and j). In addition, GPP experiences inhibition in some areas of southwestern China due to low temperatures and soil drought.
Subsequently, the positive pcor of GPP decreases or even turns slightly negative from DJF to March–April–May (MAM) in southern China, primarily attributed to shifts in temperature (Fig. 2c and g). On a nationwide scale, temperature becomes the dominant factor in this period, but it exhibits a negative correlation with GPP, with a spatial correlation coefficient of −0.18 (p < 0.5). This negative correlation is mainly due to negative GPP and positive temperature in the southwest region and due to positive GPP and negative temperature in the northern region (Fig. 2c and g). Specifically, the negative pcor of GPP in southwest China is due to soil moisture shortages (Fig. 2k). In the northern region, where a large area of croplands exists (Fig. S11), human management practices may have a greater impact on GPP, particularly in the spring when the growing season begins. However, these human management practices (e.g., irrigation, fertilization, pesticide use) are not considered in the BEPS model, which could introduce significant uncertainties in simulated GPP over cropland areas. Additionally, in some grasslands of northern Hebei and parts of neighboring Inner Mongolia, GPP shows positive pcor during El Niño events, possibly due to the strong legacy effects of climatic conditions in the DJF period.
Moving into JJA, the pcor of GPP exhibits widespread negative values again (Fig. 2d). In general, during El Niño, increased soil moisture and lower temperatures greatly contribute to enhanced GPP, while drier soil moisture and higher temperatures inhibit the increase in GPP (Fig. 2p). Regionally, higher temperatures and lower soil moisture both contribute to the negative GPP anomalies over southwestern China. However, lower soil moisture predominantly curtails GPP over the Tibetan Plateau, the Yellow River basin, and northeastern Inner Mongolia. Overall, the correlation coefficients between GPP and TAS and SM in summer are comparable, with soil moisture exhibiting a slightly higher effect, represented by a correlation coefficient of 0.47 (p < 0.05), compared to a correlation coefficient of −0.37 (p < 0.05) for temperature.
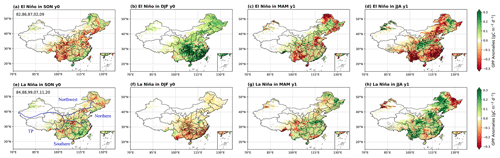
Figure 4Spatial distributions of seasonal composite GPP anomalies for ENSO events, (a–d) for El Niño and (e–h) for La Niña. The black slashes indicate areas where El Niño events differ significantly from La Niña events (p≤ 0.05) based on the Student two-sample t test. The two-digit year in the first column denotes the years used for composite analysis. Additionally, China is divided into four regions: Northwest China, the Tibetan Plateau, Northern China, and Southern China, as shown in panel (e), which is used in the following context.
3.2 Historical relationship between GPP and IOD
In comparison, the pcor patterns between GPP and DMI in SON, controlling for the effect of ONI, are nearly opposite to those between GPP and ONI (Figs. 2 and 3). In detail, GPP demonstrates significant positive pcor values with DMI in southwestern China and eastern Inner Mongolia but displays significant negative pcor with DMI in southeastern China during SON (Fig. 3a). In terms of climate drivers, during the pIOD events, for instance, wetter soil and lower temperatures both benefit the significant enhancement in GPP in southwestern China, while higher temperatures largely contribute to the enhancement in GPP over eastern Inner Mongolia. Conversely, GPP is largely inhibited by the dry conditions in southeastern China (Fig. 3e and i). Overall, soil moisture dominates the GPP anomaly in China, with a correlation coefficient of 0.33 (p < 0.05) (Fig. 3m).
In DJF, GPP exhibits widespread significant negative pcor with DMI (Fig. 3b), primarily due to the widespread negative pcor of temperature, characterized by a correlation coefficient of 0.32 (p < 0.05) (Fig. 3f and n). Moving into MAM, the significant negative pcor between GPP and DMI carried on from those in DJF but shifts to weak positive pcor in southeastern China, driven by the significant positive pcor of temperature (Fig. 3c and g). However, the significant negative pcor of soil moisture in the Jianghuai basin and North China still negates the positive effect of temperature (Fig. 3k). During this period, temperature remains the dominant factor, with a nationwide pattern correlation coefficient of 0.16 (p < 0.05) with GPP (Fig. 3o).
In JJA, the situation undergoes a change, showing the significant positive pcor of GPP over southwestern, north, and northeast China and the weak negative pcor over southeastern China (Fig. 3d). In other words, lower temperatures and gradually wetter soil are conducive to the increase in vegetation photosynthesis, but heat and dry conditions cause the weak inhibition of photosynthesis in southeastern China during the pIOD (Fig. 3p). However, unlike the ENSO event, the role of temperature is slightly higher than that of SM in the IOD event, and the correlations between GPP and TAS and SM are −0.39 and 0.36 (p < 0.05), respectively.
3.3 GPP anomalies caused by specific ENSO and IOD events
While we have elucidated the historical relationship between GPP and ENSO and IOD events through partial correlation coefficients and discussed the underlying climate drivers, we here specifically selected actual events to conduct a composite analysis. This approach aims at further comprehensive understanding of the effects of ENSO and IOD events on GPP variations in China.
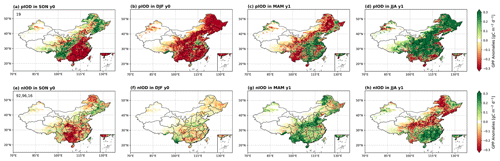
Figure 5Similar to Fig. 4 but for spatial distributions of seasonal composite GPP anomalies for IOD events, (a–d) for pIOD and (e–h) for nIOD. We did not conduct the significance test here owing to the limited samples.
3.3.1 ENSO-induced GPP anomalous patterns
The impacts of El Niño and La Niña events exhibit opposite influences on GPP with obvious seasonal variations (Fig. 4). Specifically, during SON, GPP anomalies are relatively weak, indicating some suppressions over southwestern China and north China during El Niño events, primarily attributed to dry conditions there (Figs. 4a and S4a). As ENSO peaks in DJF, GPP is significantly strengthened during El Niño events and suppressed during La Niña events, especially over southern China (Fig. 4b and f), aligning well with the patterns of pcor between GPP and ONI, controlling for the effect of DMI (Fig. 2b). Concurrently, the widespread higher temperatures and wetter soil moisture both contribute to enhanced GPP over southern China during El Niño events (Figs. S3b and S4b), while colder temperatures and drier soil moisture lead to GPP suppression there during La Niña (Figs. 2f and 3f). In MAM as ENSO weakens and vegetation starts to grow in the extratropics, the enhanced GPP over southern China in DJF during El Niño events diminishes, even transitioning into a notable GPP reduction over southwestern China, north China, and northeastern China (Fig. 4c). This transition is caused by phenological and climate changes including colder temperatures and prolonged dry conditions (Figs. S3c and S4c). The GPP pattern exhibits the opposite transition in La Niña (Fig. 4g). Moving to JJA, dry and hot conditions (Fig. S3d and S4d) lead to significant negative GPP anomalies in southeastern and southwestern China in El Niño (Fig. 4d), whereas cool and wet conditions result in positive GPP anomalies in La Niña events (Fig. 4h). Overall, GPP anomalies induced by ENSO events in DJF and JJA are more pronounced than those in SON and MAM, corresponding to the life cycle of event and vegetation growth periods, respectively. Crucially, they demonstrate distinct GPP patterns, with significant enhancements in DJF and reductions in JJA during El Niño events and reverse during La Niña events, aligning well with the pcor pattern between GPP and ONI, controlling for the effect of DMI (Fig. 4). In addition, the effect of ENSO on vegetation in southern China appears more substantial.
3.3.2 IOD-induced GPP anomalous patterns
During the period from 1981 to 2021, we only find one independent but extreme pIOD event occurred in 2019 according to our criterion (Table 1). This extreme pIOD event extended from June to December, a longer duration compared to other IOD events. Different from ENSO, IOD basically peaks in SON. GPP anomalies induced by this extreme event align closely with the long-term pcor patterns between GPP and DMI, controlling for the effect of ONI (Fig. 3). Specifically, significant reductions in GPP occur in southeastern China in SON (Fig. 5a), predominantly due to heat stress and severe drought conditions (Figs. S5a and S6a), consistent with the findings revealed by Wang et al. (2021b). In DJF, the seasonal legacy of vegetation state (Yan et al., 2023) and prolonged droughts lead to the widespread GPP reductions (Figs. 5b and S6b), outweighing the potential positive effect of higher temperatures (Fig. S5b). Of course, the decline of GPP in southwestern China appears linked to lower temperatures (Figs. 5b and S5b). During MAM, the mitigation of soil moisture deficit and favorable higher temperatures in southern China facilitate a shift in GPP from decline to increase (Fig. 5c). In the north, persistent drought conditions notwithstanding (Fig. S6c), higher temperatures and the onset of the growing season contribute to the enhanced GPP (Fig. 5c). In JJA, increased precipitation over the Yangtze and Yellow River basins (Zhang et al., 2022) alleviates the soil moisture deficits (Fig. S6d). Coupled with the relatively lower temperatures, this leads to widespread GPP increases. Conversely, GPP suppressions in provinces south of 25° N and around the Bohai Sea are attributed to higher temperatures and soil water deficits (Figs. 5d, S5d, and S6d).
In contrast to the intense 2019 pIOD event, our composite analysis incorporates three weak nIOD events, resulting in comparatively milder anomalies. In SON, different from the pIOD event, negative GPP anomalies in nIOD mainly appear in the provinces of Guizhou, Hunan, and Guangxi (Fig. 5e), associated well with concurrent dry conditions (Fig. S6e). In DJF, although the spatial pattern of soil moisture remains largely consistent with SON (Fig. S6f), a shift from negative to positive temperature anomalies mitigates the evident GPP reductions (Fig. 5f). The ongoing soil wetting and the onset of the growing season in the Northern Hemisphere in MAM result in the increased GPP over the Yellow River basin and southwestern China (Figs. 5g, S5g, and S6g). Subsequently, in JJA, the combination of wetter soil and lower temperatures facilitates vegetation photosynthesis in southern China, while drier soil largely contributes to the reduction in GPP in north and northeastern China (Figs. 5h, S5h, and S6h).
3.3.3 National and regional total GPP anomalies
We calculated the total GPP anomaly in China and various geographic regions for each classified event on both seasonal and annual scales (Fig. 6). Regionally, the geographical divisions include Northern China, Southern China, Northwest China, and the Tibetan Plateau (Fig. 4e). Notably, the Northern China–Southern China boundary aligns closely with the 0° isotherm in January and the annual precipitation line of 800 mm. The division between the north and the northwest is determined by the annual precipitation line of 400 mm, and the Tibetan Plateau is segmented based on topographic factors.
In general, the GPP anomalies exhibit noticeable differences on the seasonal scale, while the total annual anomalies do not show a significant magnitude due to the mutual offset of positive and negative anomalies in different seasons. However, it is worth noting that our annual totals are calculated from SON in the developing year of the event to JJA in the following year. This method deviates from the traditional calendar year, and according to the conventional definition of a year, the annual anomalies induced by these events can indeed be substantial.
Specifically, taking a national perspective (Fig. 6a), GPP anomalies during the El Niño and La Niña events exhibit opposite signs in DJF and JJA, with greater magnitudes during these peak periods of the events and the most vigorous growth period of vegetation, respectively. In terms of the development process of the event, the annual anomaly of GPP is negative during El Niño, with a magnitude of −0.04 ± 0.19 Pg C yr−1, but positive during La Niña events, with a magnitude of 0.01 ± 0.37 Pg C yr−1. The asymmetry of the positive and negative phases of IOD is also evident in the total anomaly. For the pIOD event in 2019, GPP shows strong negative anomalies with values of −0.41 Pg C yr−1 in SON and −0.75 Pg C yr−1 in DJF. Conversely, it exhibits a marked positive anomaly in the following JJA, with a value of 0.85 Pg C yr−1. The annual total of the GPP anomaly is opposite for pIOD and nIOD events, showing −0.10 and 0.01 ± 0.33 Pg C yr−1, respectively. Moreover, a large standard deviation indicated that there are large uncertainties in the impact of different events, and each event has its uniqueness (Capotondi et al., 2015).
Additionally, the variation of the GPP anomaly in each region is basically consistent with that at the national scale, especially in the Southern China region. But regional differences indeed exist in the total amount of GPP anomalies, demonstrating the difference in the impact of events on different regions' GPP. Taking the 2019 extreme pIOD event as an example, the GPP showed a significant negative anomaly in the Southern China region during SON (Fig. 6c), resulting in negative anomalies in GPP at the national scale (Fig. 6a) but weak positive anomalies in the Northern China and Tibetan Plateau (TP) regions (Fig. 6b and e). Then, the GPP anomaly was close to zero in the Northern China and Southern China regions in MAM (Fig. 6b and c), while it was still a significant negative anomaly in the Northwest China and TP regions (Fig. 6d and e). Moreover, the negative annual GPP anomalies in the Southern China and Northwest China regions offset the positive anomalies of the TP and Northern China regions, resulting in a negative annual GPP anomaly at the national scale for this event.
In terms of the magnitude of GPP anomalies, they are more pronounced in the Northern China and Southern China regions, characterized by more lush vegetation, mostly less than 0.5 Pg C yr−1. Meanwhile, GPP anomalies are relatively weaker in the Northwest China and TP regions, primarily covered by grassland, generally less than 0.1 Pg C yr−1. Further, we calculate the contributions of different regions to the national total GPP anomaly in each event (Table S3), referencing an index described in the article by Ahlstrom et al. (2015), as detailed in the supplementary method. Overall, the GPP anomaly in the Southern China region dominates the national GPP variation, contributing approximately 68 % to ENSO events and 46 % to IOD events, respectively. The Northern China GPP anomaly contributes approximately 28 % to the national GPP variation in ENSO events and 39 % in IOD events. In addition, the contribution of GPP anomaly in the Northwest China and TP regions to the national GPP variation is within 10 %.
3.3.4 Relative changes in total GPP anomalies at provincial scale
We presented the spatial patterns of mean GPP anomalies from SON in the developing year to JJA in the decaying year (Fig. S7) and further calculated provincial total GPP anomalies (Fig. S8 and Table S3). Provinces with more extensive forest coverage such as Yunnan, central provinces housing the Qinling Mountains, and northeast provinces where the Greater and Lesser Khingan Mountains are situated exhibit relatively larger provincial GPP anomalies. However, differences are apparent among different events (Fig. S8). Considering differences in area and vegetation coverage across provinces, our focus centers on the relative change of GPP anomalies (Fig. 7). It is important to note that, due to different years used in composite analysis, our quantitative comparisons are limited to the same event within different provinces, while qualitative descriptions are extended to different events.
El Niño events generally induce substantial GPP changes in two main regions with a relative change of over 10 % (Fig. 7a). One region encompasses the northern coastal provinces, including Tianjin, Hebei, Shandong, and Jiangsu, while the other is situated in the western part, including Xinjiang, Tibet, and Yunnan provinces. Yunnan, rich in forest resources, bears the brunt of El Niño's impact, exhibiting a total negative GPP anomaly of −22.55 Tg C yr−1 (Table S4) and a relative change of approximately 16 %. Despite comparable relative changes in GPP for other provinces, their GPP anomalies are relatively smaller, within −5 Tg C yr−1. Notably, Xinjiang, characterized by a fragile forest steppe in the Altai and Tianshan Mountains regions, consistently demonstrates substantial relative changes in GPP during both ENSO and other events. Quantitatively, during the El Niño episode, Xinjiang witnesses a remarkable 24 % relative change in GPP, accompanied by a positive GPP anomaly of −3.82 Tg C yr−1. In contrast, during the La Niña episode, provinces with notable relative changes are mainly concentrated in the northern regions, such as Xinjiang, Inner Mongolia, Ningxia, Shanxi, and Liaoning provinces (Fig. 7b). In addition, although the influence of ENSO on GPP in the southern China is significant (Fig. 4), the total relative change through the year remains small due to the cancellation of positive and negative anomalies in different seasons.
In the pIOD classification, only the 2019 extreme event is considered, resulting in the relative change in GPP anomalies exceeding 10 % in approximately half of the provinces. Notably, Jiangxi, Fujian, Guangxi, Guangdong, and Hainan experience reductions of more than 25 % in GPP, with Jiangxi exhibiting the largest GPP anomaly of −31.50 Tg C yr−1, Conversely, Shandong, Shanxi, and Henan witness an increase of over 25 % in GPP (Fig. 7c). During nIOD events, northern provinces generally exhibit negative relative changes, while southern provinces display positive relative changes.
In summary, the relative changes in total GPP anomalies at the provincial scale exhibit an east–west pattern in annual variation. Meanwhile, the influence of IOD events on GPP presents an opposing north–south pattern.
4.1 The effect of compound ENSO and IOD events on China's GPP
Indeed, despite IOD events being generally considered an independent coupled ocean–atmosphere interaction (Saji et al., 1999), historical IOD events can occur in conjunction with ENSO (Ham et al., 2017; Yang et al., 2015). These combined phenomena are most notably represented by El Niño and pIOD and La Niña and nIOD events. Williams and Hanan (2011) researched the interactive effects of ENSO and IOD on African GPP, relying on an offline terrestrial biosphere model simulation. Their findings suggested that IOD could cause obvious anomalous GPP over much of Africa, capable of suppressing or even reversing ENSO signals in GPP anomalies. In addition, Yan et al. (2023) explored the interactive effects of ENSO and IOD on seasonal anomalies of tropical net land carbon flux using the TRENDYv9 multi-model simulations, revealing diverse effects in different sub-continents and seasons. We explore the anomalies of GPP in compound events based on composite analysis (Fig. 8), and the spatial patterns of soil moisture and temperature anomalies are shown in the appendix (Figs. S9 and S10).
The spatial patterns of the GPP anomalies during concurrent ENSO and IOD events differ from those in single events, although some similarities are evident. We observed that GPP anomalies during El Niño and pIOD events are generally opposite to those during La Niña and nIOD events. Here, we focus on the impacts of El Niño and pIOD events. In El Niño and pIOD events, GPP anomalies exhibit a general opposition, with enhanced vegetation photosynthesis in the southern regions and inhibited vegetation photosynthesis in the northern regions during SON. During El Niño and pIOD events, photosynthesis generally increased in the southern regions and decreased in the northern regions during SON, indicating opposing GPP anomalies across these areas. This spatial characteristic of GPP anomalies bears some resemblance to that induced by El Niño alone (Figs. 4a and 8a). Weak GPP anomalies are generally observed in DJF, with noticeable negative GPP anomalies in Guizhou and Hunan and some positive GPP anomalies in regions south of 25° N (Fig. 8b). Notably, during DJF, while significant positive GPP anomalies occur in El Niño events (Fig. 4b), simultaneous pIOD events induce significant negative GPP anomalies (Fig. 5b). When both events coincide, their impacts seem to largely counterbalance each other, resulting in a more neutral GPP anomaly. In MAM, GPP increases in northern China (Fig. 8c). Subsequently, in JJA, vegetation photosynthesis experiences a significant increase in the northern and Yunnan provinces (Fig. 8d).
It is worth noting that the impacts of compound events on China's GPP may not follow a straightforward linear superposition of the effects of two individual events. While their effects are nearly opposite when occurring separately, the positive and negative effects on GPP may not simply cancel each other out when they coincide. This complexity arises from the simultaneous occurrence of two tropical air–sea interaction modes, leading to intricate effects on mid-latitude circulations. Given the limited number of compound events, further exploration is necessary to unravel the effects of ENSO and IOD on GPP in China.
4.2 Modulation of large-scale circulations on China's GPP
China's GPP is intricately influenced by atmospheric circulations and sea surface temperature (Li et al., 2021; Ying et al., 2022). Ying et al. (2022) showed significant correlations between seasonal GPP variation in China and climate phenomena such as ENSO, Pacific Decadal Oscillation (PDO), and Arctic Oscillation (AO), based on the residual principal component analysis. Their research indicated that these identified SST and circulation factors could account for 13 %, 23 %, and 19 % of the seasonal GPP variations in spring, summer, and autumn, respectively. And Li et al. (2021) proved that the GPP response to El Niño varied with PDO phases during the growing seasons of typical El Niño years. Although both studies emphasized the impact of ENSO on China's GPP and explored the roles of PDO and AO, the IOD was notably absent from their analyses. Contrastingly, our study sheds light on the significant influence of the extreme positive phase of IOD in 2019, showing a substantial negative GPP anomaly in southeastern China during SON, aligning with findings by Wang et al. (2021b). Moreover, the integration of partial correlation and composite analysis in our study elucidates the considerable impact of IOD on China's GPP within this context. Importantly, our research underscores the temporal and spatial variability in the effects of IOD and ENSO on GPP across different seasons and regions. This complexity in ocean–atmosphere teleconnections implies that other climate oscillations, such as Polar/Eurasia and Atlantic Multidecadal Oscillation (AMO), might also contribute to influencing China's GPP (Zhu et al., 2017).
4.3 Uncertainties in BEPS simulations
The simulation of China's GPP by BEPS is subject to several sources of uncertainty inherent in the model's structure, parameterizations, processes, and input data (Chen et al., 2012, 2017; He et al., 2021a; Liu et al., 2018; Wang et al., 2021a). The leaf area index (LAI), a crucial input for the BEPS model, is derived from global remote sensing data that inherently possess uncertainties in spatial distribution and trend changes. Previous studies have highlighted significant uncertainties in simulating the carbon budget of global terrestrial ecosystems when employing different LAI remote sensing data (Chen et al., 2019; Liu et al., 2018). The foliage clumping index, which is used to separate sunlit and shaded LAI, can also cause some uncertainties in simulating GPP, because the current version of BEPS used the time-invariant satellite-derived clumping index (Chen et al., 2012). Biases in meteorological drivers, such as precipitation, can further result in considerable uncertainties in simulating the terrestrial carbon cycle. The choice of precipitation products, for instance, has been shown to yield considerable differences in simulated net land–atmosphere carbon flux (M. Wang et al., 2021). Moreover, the BEPS model, like other terrestrial biosphere models, lacks consideration for vegetation adaptability to rising CO2 concentration, potentially leading to an overestimation of the fertilization effect on GPP. In addition, the accuracy of simulations over agricultural areas is compromised in BEPS, as it only considers crops with a C3 photosynthetic pathway and overlooks C4 crops (He et al., 2017; He et al., 2021b; Ju et al., 2006). Although BEPS-simulated GPP demonstrates relatively high consistency with the measured GPP of Yingke station (CRO), located in the northwest of China, its accuracy lacks validation over the extensive farmlands in north and northeastern China where various crops are grown (Fig. S11). Agricultural operations, particularly irrigation, which can significantly impact GPP, are not considered in BEPS. He et al. (2021a) revealed extensive wetting signals over croplands in arid and semi-arid areas which exerted strong impacts on GPP and evapotranspiration simulations in BEPS after assimilating the Soil Moisture Active Passive (SMAP) soil moisture product. Furthermore, photosynthetic key parameters, such as carboxylation capacity at 25 °C (Vcmax,25), can largely determine the performance in simulating GPP. After assimilating the solar-induced chlorophyll fluorescence (SIF) from the Orbiting Carbon Observatory-2 (OCO-2) satellite to optimize Vcmax,25 of different plant functional types (PFTs) in BEPS, previous studies suggested the improvements in simulating GPP at regional and global scales to some extent (He et al., 2019; Wang et al., 2021a).
4.4 Limitations and future work
While the seasonal legacy effects of climate on subsequent vegetation have been widely confirmed (Bastos et al., 2020; Bastos et al., 2021), they were not fully accounted for in this study. During ENSO and IOD events, temperature and soil moisture vary with seasons, resulting in diverse conditions such as high temperature and drought, high temperature and wet, low temperature and drought, and low temperature and wet across different regions and seasons. Vegetation does not immediately respond to changes in climatic condition changes due to its environmental resistance and self-regulation. These legacy effects are complex and vary by region as ENSO or IOD events progress through different seasons.
Spring serves as a transitional period between the peak of the climatic event and the peak of the growing season, making it challenging to fully explain the spatial patterns of GPP anomalies in parts of northern China based on temperature and soil moisture. Higher temperatures during DJF in El Niño events (Fig. 2f) can advance the growing season, subsequently impacting vegetation in the following spring. Sanders-DeMott et al. (2020) have proved that a warm winter can enhance the photosynthetic capacity of vegetation in the subsequent spring. Additionally, Yan et al. (2023) quantified the influence of the preceding and contemporaneous climatic conditions on net ecosystem production (NEP) during the 1997/98 El Niño and pIOD compound event, showing that legacy effects can counteract or even reverse the effects of contemporaneous climatic conditions.
Additionally, temperature and water (precipitation or soil moisture) have long been regarded as the main climate factors driving interannual fluctuations of GPP or NEP (Zeng et al., 2005; Piao et al., 2013; Ahlstrom et al., 2015; Wang et al., 2016; Jung et al., 2017; Humphrey et al., 2018). However, other factors, such as vapor pressure deficit (VPD) and radiation, also play important roles. This may explain the occasional mismatch between GPP patterns and TAS/SM in certain regions in Figs. 2 and 3. Overall, although the dominant driving factors vary seasonally, TAS and SM capture GPP variations more effectively on a national scale.
Finally, it is worth noting that climate factors often interact closely with one another. For example, soil moisture can influence changes in surface air temperature, and vice versa. As a result, in addition to direct effects, climate drivers may also impact vegetation through indirect pathways. Humphrey et al. (2021) discussed the direct and indirect effects of soil moisture on variations in terrestrial interannual carbon sinks – specifically, through its influence on temperature and VPD – using simulations from four Earth system models. This area of interaction warrants further investigation in future research.
In this paper, we used partial correlation coefficients and composite analysis to investigate the impacts of ENSO and IOD events on China's GPP during 1981–2021. The partial correlation results reveal that the effects of ENSO and IOD on GPP and related climate in China exhibit distinct seasonal variations and are basically opposite. Specifically, during SON, significant negative pcor between GPP and ENSO is observed over the Tibetan Plateau, southwestern China, the Loess Plateau, and Liaoning. In DJF, strongly positive pcor occurs over southern China, weakening in the subsequent MAM, albeit with some enhancements in northern Hebei and neighboring Inner Mongolia. The pcor then turns generally negative in JJA. In contrast, significant positive pcor between GPP and IOD is noted in southwestern and northeast China during SON. Subsequently, widespread negative pcor appears during DJF, persisting significantly in most western and northern regions during MAM. In JJA, the pcor becomes significantly positive in southwestern, north, and northeast China. Moreover, the correlation coefficients between GPP and climate show that GPP anomalies are primarily dominated by SM during ENSO events except for MAM, while temperature generally plays a more important role during IOD events except for SON.
The composite analysis results validate the patterns of GPP anomalies observed in the partial correlation. Generally, China's annual total GPP demonstrates modest positive anomalies in La Niña and nIOD years, contrasting with minor negative anomalies in El Niño and pIOD years. This results from the counterbalancing effects, with significantly greater GPP anomalous magnitudes in DJF and JJA. Regionally, GPP anomalies fluctuate more in the Southern China and Northern China regions. The GPP anomaly in the Southern China region dominates the national GPP variation, with the contribution of 68 % to ENSO events and 46 % to IOD events, respectively. On the provincial scale, western and northern provinces experience larger relative annual variations during ENSO events, with magnitudes exceeding 10 %, exhibiting a general east–west pattern. Conversely, provinces in southern and northern China witness larger relative changes during IOD events, showing an opposing north–south pattern. For instance, the 2019 extreme pIOD led to relative changes of over 25 % in certain provinces in the south and north.
ERA5 meteorological data are available at https://doi.org/10.24381/cds.adbb2d47 (Hersbach et al., 2023). The remote sensing GLOBMAP LAI data are available at https://doi.org/10.5281/zenodo.4700264 (Liu et al., 2021). The carbon dioxide emissions data are available at https://doi.org/10.15138/9N0H-ZH07 (Lan et al., 2022). Vegetation type data for BEPS simulations are obtained from https://doi.org/10.5067/MODIS/MCD12Q1.006 (Friedl and Sulla-Menashe, 2019). Soil texture data are available at https://data.tpdc.ac.cn/zh-hans/data/611f7d50-b419-4d14-b4dd-4a944b141175 (Fischer et al., 2008). Soil moisture and surface air temperature from ERA5-Land are available at https://doi.org/10.24381/cds.68d2bb30 (Muñoz, 2019). The sea surface temperature dataset from ERSSTv5 is available at https://doi.org/10.1175/JCLI-D-16-0836.1 (Huang et al., 2017). Eight of the 10 sites are from ChinaFLUX data available at https://chinaflux.org/ (https://doi.org/10.1007/s11442-016-1300-5, Yu et al., 2016), and two are from the National Tibetan Plateau/Third Pole Environment Data Center (https://doi.org/10.1175/BAMS-D-12-00154.1, Li et al., 2013). FluxSat GPP version 2.2 is available at https://doi.org/10.3390/rs10091346 (Joiner et al., 2018).
The supplement related to this article is available online at: https://doi.org/10.5194/bg-21-5027-2024-supplement.
JW designed the experiments. RY processed the data, carried out the analysis, and wrote the original manuscript. All the authors contributed to the writing of the paper.
The contact author has declared that none of the authors has any competing interests.
Publisher's note: Copernicus Publications remains neutral with regard to jurisdictional claims made in the text, published maps, institutional affiliations, or any other geographical representation in this paper. While Copernicus Publications makes every effort to include appropriate place names, the final responsibility lies with the authors. Regarding the maps used in this paper, please note that Figs. 2–5 and 7–8 contain disputed territories.
The calculations in this paper were performed on the computing facilities in the High Performance Computing Center (HPCC) of Nanjing University. We thank the reviewers for their constructive and useful comments.
This research has been supported by the National Natural Science Foundation of China (grant nos. 42141005 and 42475129); the Natural Science Foundation of Jiangsu Province, China (BK20221449); and the Tibet science and technology innovation base construction project (XZ202401YD0008).
This paper was edited by Sara Vicca and reviewed by Na Chen and one anonymous referee.
Ahlstrom, A., Raupach, M. R., Schurgers, G., Smith, B., Arneth, A., Jung, M., Reichstein, M., Canadell, J. G., Friedlingstein, P., Jain, A. K., Kato, E., Poulter, B., Sitch, S., Stocker, B. D., Viovy, N., Wang, Y. P., Wiltshire, A., Zaehle, S., Zeng, N.: The dominant role of semi-arid ecosystems in the trend and variability of the land CO2 sink, Science, 348, 895–899, https://doi.org/10.1126/science.aaa1668, 2015.
Bastos, A., Ciais, P., Friedlingstein, P., Sitch, S., and Zaehle, S.: Direct and seasonal legacy effects of the 2018 heat wave and drought on European ecosystem productivity, Sci. Adv., 6, eaba2724, https://doi.org/10.1126/sciadv.aba2724, 2020.
Bastos, A., Orth, R., Reichstein, M., Ciais, P., Viovy, N., Zaehle, S., Anthoni, P., Arneth, A., Gentine, P., Joetzjer, E., Lienert, S., Loughran, T., McGuire, P. C., O, S., Pongratz, J., and Sitch, S.: Vulnerability of European ecosystems to two compound dry and hot summers in 2018 and 2019, Earth Syst. Dynam., 12, 1015–1035, https://doi.org/10.5194/esd-12-1015-2021, 2021.
Bauch, M.: Chapter 15 – Impacts of extreme events on medieval societies: Insights from climate history, in: Climate Extremes and Their Implications for Impact and Risk Assessment, edited by: Sillmann, J., Sippel, S., and Russo, S., Elsevier, https://doi.org/10.1016/B978-0-12-814895-2.00015-X, 279–291, 2020.
Capotondi, A., Wittenberg, A., Newman, M., Di, L., Yu, J., Braconnot, P., Cole, J., Dewitte, B., Giese, B., Guilyardi, E., Jin, F., Karnauskas, K., Kirtman, B., Lee, T., Schneider, N., Xue, Y., and Yeh, S.: Understanding ENSO Diversity, B. Am. Meteorol. Soc., 96, 921–938, https://doi.org/10.1175/BAMS-D-13-00117.1, 2015.
Chen, J., Liu, J., Cihlar, J., and Goulden, M.: Daily canopy photosynthesis model through temporal and spatial scaling for remote sensing applications, Ecol. Model., 124, 99–119, https://doi.org/10.1016/S0304-3800(99)00156-8, 1999.
Chen, J. M., Mo, G., Pisek, J., Liu, J., Deng, F., Ishizawa, M., and Chan, D.: Effects of foliage clumping on the estimation of global terrestrial gross primary productivity, Global Biogeochem. Cy., 26, GB1019, https://doi.org/10.1029/2010GB003996, 2012.
Chen, J., Ju, W., Ciais, P., Viovy, N., Liu, R., Liu, Y., and Lu X.: Vegetation structural change since 1981 significantly enhanced the terrestrial carbon sink, Nat. Commun., 10, 4259, https://doi.org/10.1038/s41467-019-12257-8, 2019.
Chen, Z., Chen, J., Zhang, S., Zheng, X., Ju, W., Mo, G., Lu, X.: Optimization of Terrestrial Ecosystem Model Parameters Using Atmospheric CO2 Concentration Data With the Global Carbon Assimilation System (GCAS), J. Geophys. Res.-Biogeo., 122, 3218–3237, https://doi.org/10.1002/2016JG003716, 2017.
Fischer, G., Nachtergaele, F., Prieler, S., van Velthuizen, H. T., Verelst, L., Wiberg, D.: Global Agro-ecological Zones Assessment for Agriculture (GAEZ 2008) [data set], IIASA, Laxenburg, Austria and FAO, Rome, Italy, https://data.tpdc.ac.cn/zh-hans/data/611f7d50-b419-4d14-b4dd-4a944b141175 (last access: 5 November 2024), 2008.
Friedl, M., Sulla-Menashe, D.: MCD12Q1 MODIS/Terra+Aqua Land Cover Type Yearly L3 Global 500 m SIN Grid V006, NASA EOSDIS Land Processes Distributed Active Archive Center [data set], https://doi.org/10.5067/MODIS/MCD12Q1.006, 2019.
Gough, C.: Terrestrial Primary Production: Fuel for Life, Nature Education Knowledge, 3, 28, 2011.
Ham, Y., Choi, J., and Kug, J.: The weakening of the ENSO–Indian Ocean Dipole (IOD) coupling strength in recent decades, Clim. Dynam., 49, 249–261, https://doi.org/10.1007/s00382-016-3339-5, 2017.
He, L., Chen, J., Liu, J., Bélair, S., and Luo, X.: Assessment of SMAP soil moisture for global simulation of gross primary production, J. Geophys. Res.-Biogeo., 122, 1549–1563, https://doi.org/10.1002/2016jg003603, 2017.
He, L., Chen, J., Liu, J., Zheng, T., Wang, R., Joiner, J., Chou, S., Cheng, B., Liu, Y., and Liu, R.: Diverse photosynthetic capacity of global ecosystems mapped by satellite chlorophyll fluorescence measurements, Remote Sens. Environ., 232, 111344, https://doi.org/10.1016/j.rse.2019.111344, 2019.
He, L., Chen J., Mostovoy, G., and Gonsamo, A.: Soil Moisture Active Passive Improves Global Soil Moisture Simulation in a Land Surface Scheme and Reveals Strong Irrigation Signals Over Farmlands, Geophys. Res. Lett., 48, e2021GL092658, https://doi.org/10.1029/2021gl092658, 2021a.
He, L., Wang, R., Mostovoy, G., Liu, J., Chen, J., Shang, J., Liu, J., McNairn, H., and Powers, J.: Crop Biomass Mapping Based on Ecosystem Modeling at Regional Scale Using High Resolution Sentinel-2 Data, Remote Sens.-Basel, 13, 806, https://doi.org/10.3390/rs13040806, 2021b.
He, Q., Ju, W., Dai, S., He, W., Song, L., Wang, S., Li, X., and Mao, G.: Drought Risk of Global Terrestrial Gross Primary Productivity Over the Last 40 Years Detected by a Remote Sensing-Driven Process Model. J. Geophys. Res.-Biogeo., 126, e2020JG005944, https://doi.org/10.1029/2020JG005944, 2021.
Hersbach, H., Bell, B., Berrisford, P., Biavati, G., Horányi, A., Muñoz Sabater, J., Nicolas, J., Peubey, C., Radu, R., Rozum, I., Schepers, D., Simmons, A., Soci, C., Dee, D., Thépaut, J-N.: ERA5 hourly data on single levels from 1940 to present, Copernicus Climate Change Service (C3S) Climate Data Store (CDS) [data set], https://doi.org/10.24381/cds.adbb2d47, 2023.
Houghton, R. A.: Balancing the global carbon budget, Annu. Rev. Earth Pl. Sc., 35, 313–347, https://doi.org/10.1146/annurev.earth.35.031306.140057, 2007.
Huang, B., Thorne, P., Banzon, V., Boyer, T., Chepurin, G., Lawrimore, J., Menne, M., Smith, T., Vose, R., and Zhang, H.: Extended reconstructed Sea surface temperature, Version 5 (ERSSTv5): Upgrades, validations, and intercomparisons, J. Climate, 30, 8179–8205, https://doi.org/10.1175/JCLI-D-16-0836.1, 2017.
Humphrey, V., Zscheischler, J., Ciais, P., Gudmundsson, L., Sitch, S., and Seneviratne, SI.: Sensitivity of atmospheric CO2 growth rate to observed changes in terrestrial water storage, Nature, 560, 628–631, https://doi.org/10.1038/s41586-018-0424-4, 2018.
Humphrey, V., Berg, A., Ciais, P., Gentine, P., Jung, M., Reichstein, M., Seneviratne, SI., and Frankenberg, C.: Soil moisture–atmosphere feedback dominates land carbon uptake variability, Nature, 592, 65–69, https://doi.org/10.1038/s41586-021-03325-5, 2021.
Joiner, J., Yoshida, Y., Zhang, Y., Duveiller, G., Jung, M., Lyapustin, A., Wang, Y., and Tucker, C. J.: Estimation of Terrestrial Global Gross Primary Production (GPP) with Satellite Data-Driven Models and Eddy Covariance Flux Data, Remote Sens.-Basel, 10, 1346, https://doi.org/10.3390/rs10091346, 2018.
Ju, W., Chen J., Black T., Barr, A., Liu, J., and Chen, B.: Modelling multi-year coupled carbon and water fluxes in a boreal aspen forest, Agr. Forest Meteorol., 140, 136–151, https://doi.org/10.1016/j.agrformet.2006.08.008, 2006.
Jung, M., Reichstein, M., Schwalm, C. R., Huntingford, C., Sitch, S., Ahlstrom, A., Arneth, A., Camps-Valls, G., Ciais, P., Friedlingstein, P., Gans, F., Ichii, K., Ain, A., Kato, E., Papale, D., Poulter, B., Raduly, B., Rödenbeck, C., Tramontana, G., Viovy, N., Wang, Y. P., Weber, U., Zaehle, S., and Zeng, N.: Compensatory water effects link yearly global land CO2 sink changes to temperature, Nature, 541, 516–520, https://doi.org/10.1038/nature20780, 2017.
Kim, J., Kug J., and Jeong S.: Intensification of terrestrial carbon cycle related to El Niño-Southern Oscillation under greenhouse warming, Nat. Commun., 8, 1674, https://doi.org/10.1038/s41467-017-01831-7, 2017.
Lan, X., Tans, P., and Thoning, K. W.: Trends in globally-averaged CO2 determined from NOAA Global Monitoring Laboratory measurements, Global Monitoring Laboratory [data set], https://doi.org/10.15138/9N0H-ZH07, 2022.
Li, X., Cheng, G., Liu, S,, Xiao, Q., Ma, M., Jin, R., Che, T., Liu, Q., Wang, W., Qi, Y., Wen, J., Li, H., Zhu, G., Guo, J., Ran, Y., Wang, S., Zhu, Z., Zhou, J., Hu, X., Xu, Z.: Heihe watershed allied telemetry experimental research (HiWATER): scientific objectives and experimental design, B. Am. Meteorol. Soc., 94, 1145–1160, https://doi.org/10.1175/BAMS-D-12-00154.1, 2013.
Li, Y., Dan, L., Peng, J., Wang, J., Yang, F., Gao, D., Yang, X., and Yu, Q.: Response of Growing Season Gross Primary Production to El Niño in Different Phases of the Pacific Decadal Oscillation over Eastern China Based on Bayesian Model Averaging, Adv. Atmos. Sci., 38, 1580–1595, https://doi.org/10.1007/s00376-021-0265-1, 2021.
Liu, R., Liu, Y., and Chen, J.: GLOBMAP global Leaf Area Index since 1981 (Version 3.0), Zenodo [data set], https://doi.org/10.5281/zenodo.4700264, 2021.
Liu, J., Chen J., Cihlar, J., and Park W.: A process-based boreal ecosystem productivity simulator using remote sensing inputs, Remote Sens. Environ., 62, 158–175, https://doi.org/10.1016/S0034-4257(97)00089-8, 1997.
Liu, Y., Liu, R., and Chen, J.: Retrospective retrieval of long-term consistent global leaf area index (1981-2011) from combined AVHRR and MODIS data, J. Geophys. Res.-Biogeo.,, 117, G04003, https://doi.org/10.1029/2012JG002084, 2012.
Liu, Y., Yang X., Wang, E., and Xue, C.: Climate and crop yields impacted by ENSO episodes on the North China Plain: 1956–2006, Reg. Environ. Change., 14, 49–59, https://doi.org/10.1007/s10113-013-0455-1, 2014.
Liu, Y., Xiao, J., Ju, W., Zhu, G., Wu, X., Fan,W., Li, D., and Zhou, Y.: Satellite-derived LAI products exhibit large discrepancies and can lead to substantial uncertainty in simulated carbon and water fluxes, Remote Sens. Environ., 206, 174–188, https://doi.org/10.1016/j.rse.2017.12.024, 2018.
Mercado, L., Bellouin, N., Sitch, S., Boucher, O., Huntingford, C., Wild, M., Cox, P.: Impact of changes in diffuse radiation on the global land carbon sink, Nature, 458, 1014–1017, https://doi.org/10.1038/nature07949, 2009.
Muñoz, S. J.: ERA5-Land monthly averaged data from 1950 to present, Copernicus Climate Change Service (C3S) Climate Data Store (CDS) [data set], https://doi.org/10.24381/cds.68d2bb30, 2019.
Norman, J. M.: Simulation of microclimates, in: Biometeorology in Integrated Pest Management, edited by: Hatfield, J. and Thomason, I., Academic Press, New York, CA, 65–99, https://doi.org/10.1016/B978-0-12-332850-2.50009-8, 1982.
Piao, S., Sitch, S., Ciais, P., Friedlingstein, P., Peylin, P., Wang, X., Ahlstrom, A., Anav, A., Canadell, J., Cong, N., Huntingford, C., Jung, M., Levis, S., Levy, PE., Li, J., Lin, X., Lomas, M., Lu, M., Luo, Y., Ma, Y., Myneni, R., Poulter, B., Sun, Z., Wang, T., Viovy, N., Zaehle, S., and Zeng, N.: Evaluation of terrestrial carbon cycle models for their response to climate variability and to CO2 trends, Glob. Change Biol., 2117–2132, https://doi.org/10.1111/gcb.12187, 2013.
Piao, S., Wang, X., Wang, K., Li, X., Bastos, A., Canadell, J., Ciais, P., Friedlingstein, P., and Sitch, S.: Interannual variation of terrestrial carbon cycle: Issues and perspectives, Glob. Change Biol., 26, 300–318, https://doi.org/10.1111/gcb.14884, 2020.
Ryu, Y., Berry J., and Baldocchi, D.: What is global photosynthesis? History, uncertainties and opportunities, Remote Sens. Environ., 223, 95–114, https://doi.org/10.1016/j.rse.2019.01.016, 2019.
Saji, N. and Yamagata, T.: Possible impacts of Indian Ocean Dipole mode events on global climate, Clim. Res., 25, 151–169, https://doi.org/10.3354/cr025151, 2003.
Saji, N., Goswami, B., Vinayachandran, P., and Yamagata, T.: A dipole mode in the tropical Indian Ocean, Nature, 401, 360–363, https://doi.org/10.1038/43855, 1999.
Sanders-DeMott, R., Ouimette, A., Lepine, L., Fogarty, S., Burakowski, E., Contosta, A., Ollinger, S.: Divergent carbon cycle response of forest and grass-dominated northern temperate ecosystems to record winter warming, Glob. Change Biol., 26, 1519–1531, https://doi.org/10.1111/gcb.14850, 2020.
Schimel, D., Stephens, B., and Fisher, J.: Effect of increasing CO2 on the terrestrial carbon cycle, P. Natl. Acad. Sci. USA, 112, 436–441, https://doi.org/10.1073/pnas.1407302112/-/DCSupplemental, 2015.
Wang, J., Zeng, N., and Wang, M.: Interannual variability of the atmospheric CO2 growth rate: roles of precipitation and temperature, Biogeosciences, 13, 2339–2352, https://doi.org/10.5194/bg-13-2339-2016, 2016.
Wang, J., Zeng, N., Wang, M., Jiang, F., Chen, J., Friedlingstein, P., Jain, A. K., Jiang, Z., Ju, W., Lienert, S., Nabel, J., Sitch, S., Viovy, N., Wang, H., and Wiltshire, A. J.: Contrasting interannual atmospheric CO2 variabilities and their terrestrial mechanisms for two types of El Niños, Atmos. Chem. Phys., 18, 10333–10345, https://doi.org/10.5194/acp-18-10333-2018, 2018.
Wang, J., Liu, Z., Zeng, N., Jiang, F., Wang, H., and Ju, W.: Spaceborne detection of XCO2 enhancement induced by Australian mega-bushfires, Environ. Res. Lett., 15, 124069, https://doi.org/10.1088/1748-9326/abc846, 2020.
Wang, J., Jiang, F., Wang, H., Qiu, B., Wu, M., He, W., Ju, W., Zhang, Y., Chen, J., and Zhou, Y.: Constraining global terrestrial gross primary productivity in a global carbon assimilation system with OCO-2 chlorophyll fluorescence data, Agr. Forest Meteorol., 304–305, 108424, https://doi.org/10.1016/j.agrformet.2021.108424, 2021a.
Wang, J., Wang, M., Kim, J., Joiner, J., Zeng, N., Jiang, F., Wang, H., He, W., Wu, M., Chen, T., Ju, W., and Chen, J.: Modulation of Land Photosynthesis by the Indian Ocean Dipole: Satellite-Based Observations and CMIP6 Future Projections, Earths Future, 9, e2020EF001942, https://doi.org/10.1029/2020ef001942, 2021b.
Wang, J., Jiang, F., Ju, W., Wang, M., Sitch, S., Arora, V., Chen, J., Goll, D., He, W., Jain, A., Li, X., Joiner, J., Poulter, B., Seferian, R., Wang, H.,Wu, M., Xiao, J., Yuan, W., Yue, X., Zaehle, S.: Enhanced India-Africa Carbon Uptake and Asia-Pacific Carbon Release Associated With the 2019 Extreme Positive Indian Ocean Dipole, Geophys. Res. Lett., 49, e2022GL100950, https://doi.org/10.1029/2022gl100950, 2022.
Wang, M., Wang, J., Cai, Q., Zeng, N., Lu, X., Yang, R., Jiang, F., Wang, H., and Ju, W.: Considerable Uncertainties in Simulating Land Carbon Sinks Induced by Different Precipitation Products, J. Geophys. Res.-Biogeo., 126, e2021JG006524, https://doi.org/10.1029/2021JG006524, 2021.
Williams, C. A. and Hanan, N. P.: ENSO and IOD teleconnections for African ecosystems: evidence of destructive interference between climate oscillations, Biogeosciences, 8, 27–40, https://doi.org/10.5194/bg-8-27-2011, 2011.
Yan, R., Wang, J., Ju, W., Goll, D., Jain, A., Sitch, S., Tian, H., Benjamin, P., Jiang, F., and Wang, H.: Interactive effects of the El Niño-Southern Oscillation and Indian Ocean Dipole on the tropical net ecosystem productivity, Agr. Forest Meteorol., 336, 109472, https://doi.org/10.1016/j.agrformet.2023.109472, 2023.
Yang, R., Wang, J., Zeng, N., Sitch, S., Tang, W., McGrath, M. J., Cai, Q., Liu, D., Lombardozzi, D., Tian, H., Jain, A. K., and Han, P.: Divergent historical GPP trends among state-of-the-art multi-model simulations and satellite-based products, Earth Syst. Dynam., 13, 833–849, https://doi.org/10.5194/esd-13-833-2022, 2022.
Yang, Y., Xie, S.-P., Wu, L., Kosaka, Y., Lau, N.-C., and Vecchi, G. A.: Seasonality and Predictability of the Indian Ocean Dipole Mode: ENSO Forcing and Internal Variability, J. Climate, 28, 8021–8036, https://doi.org/10.1175/JCLI-D-15-0078.1, 2015.
Ying, K., Peng, J., Dan, L., and Zheng, X.: Ocean–atmosphere Teleconnections Play a Key Role in the Interannual Variability of Seasonal Gross Primary Production in China, Ad v. Atmos. Sci., 39, 1329–1342, https://doi.org/10.1007/s00376-021-1226-4, 2022.
Yu, G., Ren, W., Chen, Z., Zhang, L., Wang, Q., Wen, X., He, N., Zhang, L., Fang, H., Zhu, X., Gao, Y., and Sun, X.: Construction and progress of Chinese terrestrial ecosystem carbon, nitrogen and water fluxes coordinated observation, J. Geogr. Sci. 26, 803–826, https://doi.org/10.1007/s11442-016-1300-5, 2016 (data available at: https://chinaflux.org/, last access: October 2022).
Zeng, N., Mariotti, A., and Wetzel, P.: Terrestrial mechanisms of interannual CO2 variability, Global Biogeochem Cy., 19, GB1016, https://doi.org/10.1029/2004gb002273, 2005.
Zhang, X., Wang, Y., Peng, S., Rayner, P., Ciais, P., Silver, J., Piao, S., Zhu, Z., Lu, X., Zheng, X.: Dominant regions and drivers of the variability of the global land carbon sink across timescales, Glob. Change Biol., 24, 3954–3968, https://doi.org/10.1111/gcb.14275, 2018.
Zhang, Y., Dannenberg, M., Hwang, T., and Song, C.: El Niño-Southern Oscillation-Induced Variability of Terrestrial Gross Primary Production During the Satellite Era, J. Geophys. Res.-Biogeo., 124, 2419–2431, https://doi.org/10.1029/2019jg005117, 2019.
Zhang, Y., Zhou, W., Wang, X., Chen, S., Chen, J., and Li, S.: Indian Ocean Dipole and ENSO's mechanistic importance in modulating the ensuing-summer precipitation over Eastern China, npj Clim. Atmos. Sci., 5, 48, https://doi.org/10.1038/s41612-022-00271-5, 2022.
Zhu, Z., Piao, S., Xu, Y., Bastos, A., Ciais, P., and Peng, S.: The effects of teleconnections on carbon fluxes of global terrestrial ecosystems, Geophys. Res. Lett., 44, 3209–3218, https://doi.org/10.1002/2016GL071743, 2017.