the Creative Commons Attribution 4.0 License.
the Creative Commons Attribution 4.0 License.
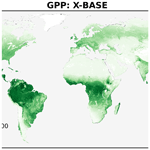
X-BASE: the first terrestrial carbon and water flux products from an extended data-driven scaling framework, FLUXCOM-X
Fabian Gans
Basil Kraft
Ulrich Weber
Kimberly Novick
Nina Buchmann
Mirco Migliavacca
Georg Wohlfahrt
Ladislav Šigut
Andreas Ibrom
Dario Papale
Mathias Göckede
Gregory Duveiller
Alexander Knohl
Lukas Hörtnagl
Russell L. Scott
Jiří Dušek
Weijie Zhang
Zayd Mahmoud Hamdi
Markus Reichstein
Sergio Aranda-Barranco
Jonas Ardö
Maarten Op de Beeck
Dave Billesbach
David Bowling
Rosvel Bracho
Christian Brümmer
Gustau Camps-Valls
Shiping Chen
Jamie Rose Cleverly
Ankur Desai
Gang Dong
Tarek S. El-Madany
Eugenie Susanne Euskirchen
Iris Feigenwinter
Marta Galvagno
Giacomo A. Gerosa
Bert Gielen
Ignacio Goded
Sarah Goslee
Christopher Michael Gough
Bernard Heinesch
Kazuhito Ichii
Marcin Antoni Jackowicz-Korczynski
Anne Klosterhalfen
Sara Knox
Hideki Kobayashi
Kukka-Maaria Kohonen
Mika Korkiakoski
Ivan Mammarella
Mana Gharun
Riccardo Marzuoli
Roser Matamala
Stefan Metzger
Leonardo Montagnani
Giacomo Nicolini
Thomas O'Halloran
Jean-Marc Ourcival
Matthias Peichl
Elise Pendall
Borja Ruiz Reverter
Marilyn Roland
Simone Sabbatini
Torsten Sachs
Marius Schmidt
Christopher R. Schwalm
Ankit Shekhar
Richard Silberstein
Maria Lucia Silveira
Donatella Spano
Torbern Tagesson
Gianluca Tramontana
Carlo Trotta
Fabio Turco
Timo Vesala
Caroline Vincke
Domenico Vitale
Enrique R. Vivoni
William Woodgate
Enrico A. Yepez
Junhui Zhang
Donatella Zona
Martin Jung
Mapping in situ eddy covariance measurements of terrestrial land–atmosphere fluxes to the globe is a key method for diagnosing the Earth system from a data-driven perspective. We describe the first global products (called X-BASE) from a newly implemented upscaling framework, FLUXCOM-X, representing an advancement from the previous generation of FLUXCOM products in terms of flexibility and technical capabilities. The X-BASE products are comprised of estimates of CO2 net ecosystem exchange (NEE), gross primary productivity (GPP), evapotranspiration (ET), and for the first time a novel, fully data-driven global transpiration product (ETT), at high spatial (0.05°) and temporal (hourly) resolution. X-BASE estimates the global NEE at −5.75 ± 0.33 Pg C yr−1 for the period 2001–2020, showing a much higher consistency with independent atmospheric carbon cycle constraints compared to the previous versions of FLUXCOM. The improvement of global NEE was likely only possible thanks to the international effort to increase the precision and consistency of eddy covariance collection and processing pipelines, as well as to the extension of the measurements to more site years resulting in a wider coverage of bioclimatic conditions. However, X-BASE global net ecosystem exchange shows a very low interannual variability, which is common to state-of-the-art data-driven flux products and remains a scientific challenge. With 125 ± 2.1 Pg C yr−1 for the same period, X-BASE GPP is slightly higher than previous FLUXCOM estimates, mostly in temperate and boreal areas. X-BASE evapotranspiration amounts to 74.7×103 ± 0.9×103 km3 globally for the years 2001–2020 but exceeds precipitation in many dry areas, likely indicating overestimation in these regions. On average 57 % of evapotranspiration is estimated to be transpiration, in good agreement with isotope-based approaches, but higher than estimates from many land surface models. Despite considerable improvements to the previous upscaling products, many further opportunities for development exist. Pathways of exploration include methodological choices in the selection and processing of eddy covariance and satellite observations, their ingestion into the framework, and the configuration of machine learning methods. For this, the new FLUXCOM-X framework was specifically designed to have the necessary flexibility to experiment, diagnose, and converge to more accurate global flux estimates.
-
Please read the editorial note first before accessing the article.
-
Article
(13321 KB)
-
Please read the editorial note first before accessing the article.
- Article
(13321 KB) - Full-text XML
- BibTeX
- EndNote
Energy, water, and carbon exchange between terrestrial surfaces and the atmosphere are key components of the Earth system and impact ecosystems, ecosystem services, weather, climate, and water availability. The exchange (or flux) can be directly observed using eddy covariance (EC) measurement systems (Baldocchi, 2019), which are installed on towers overlooking the ecosystem of interest. The EC stations typically represent an area of a few hundred square meters to a square kilometer. One key advantage of the EC methodology is the ability to provide near-continuous measurements with some records now exceeding 20 years (Pastorello et al., 2020), allowing for examination of flux variations from the order of 30 min to decades. EC systems also provide a unique perspective on the magnitude, temporal variability, and environmental sensitivity of ecosystem CO2 uptake, water use, and local climate regulation (Baldocchi, 2019; Musavi et al., 2017; Bao et al., 2022). However, while many of the most pressing scientific knowledge gaps surrounding the delicate land carbon balance and the water cycle require spatially and temporally resolved flux patterns at continental to global scales, EC observations are confined to individual locations in space and limited periods in time (Kumar et al., 2016; Papale et al., 2015). Methodologies to transcend the gap between local and global scales are needed to ultimately support societally relevant activities of building greenhouse gas monitoring systems, taking informed climate and land management actions, and verifying the effectiveness of mitigation strategies (Baldocchi and Penuelas, 2019; Bonan et al., 2011; Novick et al., 2022).
Coordinated and consolidated data collections from EC networks are invaluable for the mapping of in situ fluxes to regional and global scales. For example, EC measurements aid both the parameterization (Huang et al., 2021) and the validation (Turner et al., 2006; Heinsch et al., 2006) of mechanistic models of ecosystem productivity and land surface processes. The latter generate widely used reference data sets for terrestrial carbon cycle applications (Zhao and Running, 2010; Ukkola et al., 2022). A complementary approach to modeling terrestrial fluxes at continental and global scales is of empirical nature and links observations of explanatory variables at the EC stations, particularly meteorological and remote sensing data, to the EC fluxes via machine learning models. This upscaling concept does not prescribe any mechanistic formulations and assumes that the EC observations cover all complexities of ecosystem functioning. Based on a trained machine learning model and globally gridded input data of the explanatory variables, EC fluxes can be mapped to the global scale.
First implementations of this flux upscaling concept emerged in the early 2000s. They focused on net ecosystem exchange (NEE) of CO2 and utilized the growing EC networks in Europe (Papale and Valentini, 2003) and North America (Xiao et al., 2008). The release of the FLUXNET La Thuile Synthesis Dataset of harmonized EC data in 2007, as well as methodological improvements in the training of the machine learning models (Jung et al., 2009), led to the first global products of terrestrial CO2 and water fluxes at a monthly time step and in 0.5° grids in 2011 (Jung et al., 2011). While good agreement of flux estimates derived from complementary process-based models with the upscaled global gross photosynthetic CO2 uptake (gross primary productivity, GPP) and energy fluxes demonstrated the potential of the approach, important inconsistencies remained, in particular regarding the globally integrated NEE and its year-to-year variability (Jung et al., 2020, 2019, 2011; Zscheischler et al., 2017). A critical component of data-driven methods, including the flux upscaling methodologies described here, is understanding the related uncertainties. The multistage process of going from the actual measured values of high-frequency (10–20 Hz) measurements of three-dimensional wind components, temperature, water vapor, and CO2 concentration to the standard half-hourly, gap-filled, long-term data records from synthesis data sets such as FLUXNET has multiple associated potential biases and uncertainties. The well-documented issues of gap filling (Vekuri et al., 2023; Soloway et al., 2017; Papale et al., 2006), instrumentation errors (Zhang et al., 2023; Fratini et al., 2014; Vitale et al., 2020; Rannik et al., 2016), and energy balance non-closure (Mauder et al., 2020; Leuning et al., 2012; Stoy et al., 2013; McGloin et al., 2018; Franssen et al., 2010) will likely have an effect on the resulting gridded global data sets.
In an effort to better understand the uncertainties associated with mapping of EC fluxes to larger scales, the FLUXCOM intercomparison initiative built an ensemble of flux estimates as a type of factorial experiment (Tramontana et al., 2016; Jung et al., 2019, 2020). The ensemble consisted of multiple machine learning algorithms, meteorological forcing data, and combinations of predictor variables, resulting in 120 individual upscaled estimates per flux. These were summarized in two overall ensemble configurations, which differed in the set of predictors and spatial–temporal resolution. Apart from creating a large ensemble, the FLUXCOM evaluation included a consistent site-level cross-validation analysis as well as cross-consistency checks with terrestrial flux estimates from independent approaches, such as complementary modeling concepts or observational surrogates. From a methodological point of view, the key lessons learned from FLUXCOM were the following:
-
The overall approach seems to be primarily limited by the input information given to the machine learning algorithms rather than by the ability of the algorithm to extract the information.
-
The largest qualitative differences among flux products were related to the set of the predictor variables rather than to the choice of the machine learning method or meteorological forcing.
-
The cross-consistency checks with global independent data are essential for supplementing site-level cross-validation.
-
The largest qualitative discrepancy with independent data was a very high (strongly negative) tropical NEE that was shared among all ensemble members.
In addition to the systematic intercomparisons in FLUXCOM, the empirical upscaling concept has been implemented for a series of regional- and global-scale applications, each of them adopting disparate and individual methodological choices (e.g., Ichii et al., 2017; Yao et al., 2018; Joiner and Yoshida, 2020; Virkkala et al., 2021; Dannenberg et al., 2023; Burton et al., 2023; Zhu et al., 2024). These potentially important choices relate to data treatment (quality control, gap filling, processing pathways), ingestion (sampling, as well as matching EC and space-borne observations), and methodological configurations (machine learning methods and their training configuration, choice of predictor variables, resolution). The disparity between the setups and implementations of the empirical upscaling approach impedes any direct comparisons among products and valid conclusions as to where potential differences originate. Hence, flexibility to explore the large methodological space, as well as the ability to diagnose and evaluate global products in parallel to site-level cross-validation, is required to understand the importance of individual methodological choices and to make progress in empirical upscaling of EC fluxes.
We are developing a modeling framework that allows experimenting with many of these methodological choices. We coin this extended upscaling environment FLUXCOM-X. Compared to the FLUXCOM intercomparison initiative and the products produced therein, it follows the same overall principles. The key innovation of FLUXCOM-X is that it represents a flexibly adjustable upscaling environment to systematically explore different methodological avenues. Lessons learned from FLUXCOM and other upscaling exercises further imply striving for the enhancement of the information content of the training data with aspects related to coverage and quality of EC measurements as well as complementarity, completeness, and quality of predictor variables. The flexibility of the way FLUXCOM-X is implemented will considerably reduce the latency with which innovations in the related fields of machine learning and space-based Earth observations as well as novel EC data can find their way to empirical flux upscaling. This in turn allows faster progress towards more accurate and fit-for-purpose global biogenic flux estimates. Here, we introduce and evaluate the initial “basic” set of products from this flexible framework, which we refer to as FLUXCOM-X-BASE products (or X-BASE for short; see Appendix A for an overview on the naming conventions).
X-BASE products were comparably generated to the original FLUXCOM ensemble using qualitatively similar predictor variables, i.e., remotely sensed vegetation indices and land surface temperatures from the Moderate Resolution Imaging Spectroradiometer (MODIS) along with meteorological variables. In contrast to FLUXCOM, the remotely sensed and the meteorological predictors are combined in the X-BASE products, and the subdaily variability is resolved. We furthermore made efforts to provide more and improved information to the machine learning models by enhancing coverage and quality of the training data and by further developing the processing of satellite predictor variables (Walther and Besnard et al., 2022). In this article, we show results for X-BASE NEE, GPP, evapotranspiration (ET), and for the first time transpiration (ETT), for the period 2001–2020 at 0.05° spatial and hourly temporal resolution. We focus here on the evaluation and cross-consistency checks of X-BASE with previous FLUXCOM products and independent data streams. Our specific objectives are the following:
-
to describe the production of X-BASE products
-
to evaluate the X-BASE setup using site-level cross-validation
-
to assess qualitative differences of global patterns compared to previous FLUXCOM products with reference to independent flux estimates where possible
-
to synthesize lessons learned from this basic exercise to guide future FLUXCOM-X developments.
X-BASE products are freely available and serve as a baseline for future FLUXCOM-X developments (see data availability statement). Any future product releases originating from FLUXCOM-X will follow the naming convention X-[specific name].
The following section gives an overview on the essential methodological implementations and data choices adopted in the generation of X-BASE products.
2.1 Eddy covariance data
Eddy covariance data consisted of 294 sites from around the world though skewed towards a higher representation of temperate forests from North America and Europe. All EC data were collected, processed, and analyzed for quality by the station teams, before being processed using state-of-the-art approaches in the ONEFlux data processing pipeline (Pastorello et al., 2020). ONEFlux provides consistent quality checks, gap filling, and carbon flux partitioning. The data included were collected between 2001–2020 and available with a CC BY 4.0 license. Based on this criterion, data for each site came from one of five different sources based on most recent availability: FLUXNET 2015 (Pastorello et al., 2020), ICOS Drought 2018 (Team and Centre, 2020), ICOS Warm Winter 2020 (Team and Centre, 2022), or the most recent AmeriFlux or ICOS release as of December 2022. Table 1 lists all sites included as well as the associated digital object identifier specific to the associated release.
(Garcia et al., 2016)(Kutzbach, 2021)(Posse et al., 2016)(Wohlfahrt et al., 2016)(Cleverly and Eamus, 2016b)(Beringer and Hutley, 2016c)(Meyer et al., 2016)(Pendall and Griebel, 2016)(Beringer and Hutley, 2016b)(Beringer and Hutley, 2016f)(Beringer and Hutley, 2016e)(Schroder et al., 2016)(Beringer and Hutley, 2016a)(Macfarlane et al., 2016)(Beringer and Hutley, 2016d)(Liddell, 2016)(Cleverly and Eamus, 2016a)(Woodgate et al., 2016)(Beringer et al., 2016b)(Beringer et al., 2016a)(Arndt et al., 2016)(Beringer and Walker, 2016)(Team and Centre, 2022)(Team and Centre, 2022)(RI, 2021)(Team and Centre, 2022)(Team and Centre, 2022)(Team and Centre, 2022)(Vourlitis et al., 2022)(Saleska, 2016)(Goulden, 2016d)(Staebler, 2022)(Knox, 2022)(Christen and Knox, 2022)(Wagner-Riddle, 2021)(McCaughey, 2016)(Black, 2021)(Amiro, 2016b)(Goulden, 2016a)(Goulden, 2016b)(Goulden, 2016c)(Goulden, 2016g)(Goulden, 2016e)(Goulden, 2016f)(Black, 2016b)(Black, 2016a)(Margolis, 2016)(Amiro, 2016c)(Amiro, 2016a)(Amiro, 2016d)(Arain, 2016b)(Arain, 2016a)(Arain, 2022b)(Arain, 2016c)(Arain, 2022a)(Nouvellon, 2016)(Team and Centre, 2022)(Team and Centre, 2022)(Team and Centre, 2022)(Team and Centre, 2022)(Team and Centre, 2022)(Ammann, 2016)(Team and Centre, 2022)(Zhang and Han, 2016)(Dong, 2016)(Shi et al., 2016)(Zhou and Yan, 2016)(Chen, 2016k)(Shao, 2016b)(Tang et al., 2016)(Wang and Fu, 2016)(Shao, 2016a)(Team and Centre, 2022)(Sigut et al., 2016)(Team and Centre, 2022)(Team and Centre, 2022)(Team and Centre, 2022)(Team and Centre, 2022)(Team and Centre, 2022)(Team and Centre, 2022)(Team and Centre, 2022)(Team and Centre, 2022)(Team and Centre, 2022)(Team and Centre, 2022)(Team and Centre, 2020)(Team and Centre, 2022)(Team and Centre, 2022)(Lindauer et al., 2016)(Knohl et al., 2016)(Team and Centre, 2022)(RI, 2022)(Team and Centre, 2022)(Team and Centre, 2022)(Schneider and Schmidt, 2016)(Klatt et al., 2016)(Bernhofer et al., 2016)(Team and Centre, 2022)(Sachs et al., 2016)(Pilegaard and Ibrom, 2016)(Olesen, 2016)(RI, 2022)(Team and Centre, 2022)(Team and Centre, 2022)(Team and Centre, 2022)(Poveda et al., 2016)(Team and Centre, 2022)(Team and Centre, 2022)(Team and Centre, 2022)(Team and Centre, 2022)(Reverter et al., 2016b)(Reverter et al., 2016a)(Team and Centre, 2022)(Lohila et al., 2016)(Team and Centre, 2022)(Team and Centre, 2022)(Aurela et al., 2016a)(Team and Centre, 2022)(Team and Centre, 2022)(Aurela et al., 2016b)(RI, 2022)(Team and Centre, 2022)(Team and Centre, 2022)(RI, 2022)(Team and Centre, 2022)(Team and Centre, 2022)(Team and Centre, 2022)(Team and Centre, 2022)(Berbigier and Loustau, 2016)(RI, 2022)(Team and Centre, 2022)(Ourcival, 2016)(RI, 2022)(Team and Centre, 2022)(Valentini et al., 2016b)(RI, 2022)(Hansen, 2016)(Lund et al., 2016b)(Lund et al., 2016a)(Team and Centre, 2022)(Team and Centre, 2022)(Team and Centre, 2022)(RI, 2022)(Sabbatini et al., 2016c)(Sabbatini et al., 2016a)(Sabbatini et al., 2016b)(Matteucci, 2016)(Team and Centre, 2022)(Valentini et al., 2016a)(Gruening et al., 2016b)(Cescatti et al., 2016)(Team and Centre, 2022)(RI, 2022)(Team and Centre, 2022)(Spano et al., 2016)(Manca and Goded, 2016)(Team and Centre, 2022)(Valentini et al., 2016c)(Papale et al., 2016)(Team and Centre, 2022)(Gruening et al., 2016a)(Team and Centre, 2022)(Kotani, 2016b)(Kotani, 2016a)(Yepez and Garatuza, 2021)(Kosugi and Takanashi, 2016)(Dolman et al., 2016a)(Team and Centre, 2020)(Wolf et al., 2016b)(Wolf et al., 2016a)(Griffis and Roman, 2021)(Merbold et al., 2016)(Dolman et al., 2016b)(Team and Centre, 2022)(Team and Centre, 2022)(Belelli et al., 2016)(Ardö et al., 2016)(Team and Centre, 2022)(Team and Centre, 2022)(Team and Centre, 2020)(Team and Centre, 2022)(Team and Centre, 2022)(Team and Centre, 2022)(Christensen, 2016)(Boike et al., 2016)(Tagesson et al., 2016)(Billesbach et al., 2022)(Billesbach et al., 2016b)(Billesbach et al., 2016a)(Biraud et al., 2022)(Torn, 2016b)(Torn, 2016a)(Zona and Oechel, 2016a)(Euskirchen, 2022b)(Euskirchen, 2022c)(Euskirchen, 2022d)(Euskirchen, 2022a)(Rey-Sanchez et al., 2022b)(Rey-Sanchez et al., 2022a)(Goldstein, 2016)(Huggins, 2021)(Huggins, 2022c)(Huggins, 2022a)(Huggins, 2022b)(Chen and Chu, 2016b)(Desai, 2022a)(Desai, 2022c)(Desai, 2022d)(Desai, 2022b)(Bowling, 2016)(Oikawa, 2021)(Massman, 2016)(Massman, 2022)(Meyers, 2016b)(Forsythe et al., 2021)(Goslee, 2022)(Munger, 2016)(Liu et al., 2022)(Hollinger, 2022)(Matamala, 2016)(Euskirchen et al., 2022a)(Euskirchen et al., 2022b)(Zona and Oechel, 2016b)(Vivoni and Perez-Ruiz, 2022)(Brunsell, 2022a)(Brunsell, 2022b)(Drake and Hinkle, 2016a)(Drake and Hinkle, 2016b)(Hinkle, 2022)(Meyers, 2016a)(Fares, 2016)(Desai, 2016c)(Novick and Phillips, 2022)(Wood and Gu, 2022)(Law, 2016c)(Law, 2022)(Law, 2016a)(Law, 2016e)(Law, 2016d)(Law, 2016b)(Litvak, 2021)(Sturtevant et al., 2016)(Torn and Dengel, 2021)(Blanken et al., 2022)(Suyker, 2022)(Suyker, 2016b)(Suyker, 2016a)(Silveira, 2021)(Bohrer, 2021)(Bohrer and Kerns, 2022)(Chen et al., 2016)(Desai, 2016d)(Kobayashi and Suzuki, 2016)(Flerchinger, 2022c)(Baker et al., 2022)(Baker and Griffis, 2022a)(Baker and Griffis, 2021)(Baker and Griffis, 2022b)(Flerchinger and Reba, 2022)(Flerchinger, 2022a)(Flerchinger, 2022b)(Kurc, 2022)(Scott, 2016a)(Scott, 2016b)(Shortt et al., 2022)(Kusak et al., 2022)(Ewers and Pendall, 2016)(Desai, 2016b)(Baldocchi and Ma, 2016)(Valach et al., 2021)(Sturtevant et al., 2022)(Chamberlain et al., 2022)(Sanchez et al., 2016)(Valach et al., 2022)(Baldocchi, 2016)(Bohrer, 2022)(Gough et al., 2016)(Gough et al., 2022)(Baldocchi et al., 2016)(Desai, 2016a)(Chen and Chu, 2016a)(Scott, 2016d)(Chen, 2016g)(Chen, 2016e)(Chen, 2016j)(Chen, 2016b)(Chen, 2016d)(Chen, 2016a)(Chen, 2016h)(Chen, 2016i)(Chen, 2016c)(Chen, 2016f)(Litvak, 2022)(Scott, 2016c)(Network, 2022)Meteorological data measured at each site consisted of incoming shortwave radiation, air temperature, and vapor pressure deficit, of which all data were gap-filled using the marginal distribution sampling method (Reichstein et al., 2005), as well as the computed potential shortwave incoming radiation (top-of-atmosphere theoretical maximum radiation) for every hour. Carbon dioxide flux data consisted of gap-filled net ecosystem exchange (NEE, variable USTAR threshold 50th percentile i.e., NEE_VUT_50) and the corresponding gross primary productivity (GPP, nighttime partitioning method after Reichstein et al., 2005). Water flux data consisted of evapotranspiration (ET, no energy balance correction), which was converted from the latent energy and transpiration estimates based on the transpiration estimation algorithm (TEA; Nelson et al., 2018; Nelson, 2021). The TEA estimates transpiration based on the relationship between GPP and ET under conditions where surface evaporation and soil evaporation are expected to be minimal, accounting for residual non-transpiration evaporation. Note that estimates of GPP and transpiration are not direct measurements but instead are based on statistical relationships localized to each station and time period and thus contain their own assumptions and uncertainties. All data were aggregated to a common hourly time resolution, an overview of which can be found in Table 2.
Table 2Fluxes to be predicted and predictor variables used in X-BASE. The units of the fluxes correspond to the native hourly resolution. Upon temporal aggregation as in some analyses in the presented results, the units may change.
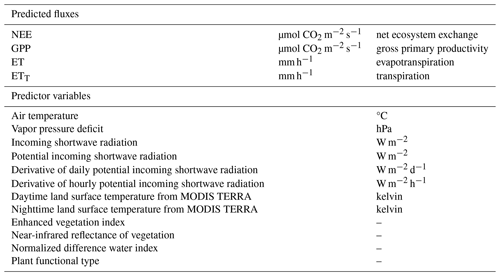
Data from the EC data set that ultimately were used for training the models varied 1214×106 site hours depending on the target variable (i.e., GPP, NEE, ET, or ETT). Training of the machine learning algorithms was only conducted on hours where all input variables passed quality control. The quality control procedure consisted of two levels, with the first being that each hour must have at least one value of good quality measured or gap-filled with confidence (i.e., at least one half hour was either 0 or 1 based on the ONEFlux _QC flags). Second, a set of consistency tests were performed on each used variable to check the consistency both among variables and across sites. As the consistency flags were based on daily aggregates of the meteorological and flux data, entire days were removed if the test indicated inconsistencies among related variables. The consistency flag also checked the relationship between variables across sites, ensuring that the relationships found across the data are coherent. A detailed explanation of these consistency flags can be found in Jung et al. (2024).
2.2 Global meteorology
For the generation of global flux maps we used hourly meteorological data from ERA5 global reanalysis products at 0.25° (Hersbach et al., 2020). Variables included air temperature at 2 m height, incoming shortwave radiation at the surface, and vapor pressure deficit (computed from relative humidity, air temperature, and surface pressure). Units were converted to correspond to the site-level measurements, which were used for training the machine learning model, and the data were regridded to a 0.05° resolution using bilinear interpolation for every hour.
2.3 Satellite Earth observation
The X-BASE products are based on measurements of the MODerate Imaging Spectroradiometer (MODIS) of surface reflectance and land surface temperature from collection 006 at daily resolution. Missing records were gap-filled consistently in both the average time series per EC station and in the global gridded data following the procedures of the FluxnetEO data version 2 (Walther and Besnard et al., 2022; Walther, 2023).
2.3.1 Spectral vegetation indices
At site level we used surface reflectance in the first seven MODIS spectral bands from the MCD43A4 c006 reflectance data set (500 m and daily, where each daily value is inverted from all valid observations within a 16 d window; Schaaf and Wang, 2015b). The spectral vegetation indices computed from the reflectance data were the enhanced vegetation index (EVI; Huete et al., 2002), the spectral reflectance of vegetation in the near-infrared (NIRv; Badgley et al., 2017), and the normalized difference water index (NDWI) with MODIS band 7 as reference (Gao, 1996). We followed the procedure of the FluxnetEO data set version 2 (Walther and Besnard et al., 2022) for data acquisition from Google Earth Engine for all pixels in a cutout of 4×4 km2 around each EC station, as well as for quality checks in terms of cloud, snow, and land cover; index values outside the defined ranges; and outliers. An iterative approach then determined both the strictness of the inversion quality of the bidirectional reflectance distribution function (BRDF, based on the MCD43A2 data, Schaaf and Wang, 2015a) and the set of pixels in a cutout that shall represent a given EC station. Appendix B1 outlines all technical details of the dynamic procedure.
Global data of BRDF-corrected surface reflectance stem from the MCD43C4 c006 data (Schaaf and Wang, 2015b), available in a climate modeling grid of 0.05° with the same temporal sampling and subject to the same removal of snow and water pixels and outlier values as at site level. The BRDF quality control of the global data followed the same dynamic approach (see Appendix B1), which maximized data availability especially in tropical regions.
2.3.2 Land surface temperature
Satellite observations of land surface temperature (LST) were based on the MODIS c006 TERRA observations, which are available every day at 1 km resolution (Wan et al., 2015). We selected the 1 km2 pixel containing a specific tower and treated the two MODIS LST data streams as independent predictor variables, which represent clear-sky LST at a specific time of the day (namely around 10:30 and 22:30 local time). Quality checks and gap filling followed the procedure described in FluxnetEO version 2 (Walther and Besnard et al., 2022).
For the global spatialization of the flux estimates, we relied on climate modeling grid LST from the MODIS TERRA data sets (Wan et al., 2015) and apply consistent quality control and imputation of missing values like at site level.
2.3.3 Land cover
Land cover information used the IGBP global vegetation classification. Site-level classification was as reported by the principal investigators. Global data were based on the yearly resolved MODIS MCD12C1 c006 product (Friedl and Sulla-Menashe, 2015). In order to ease the transition between site and global land cover classifications, an intermediate classification scheme was utilized which translated each classification into characteristics (e.g., trees, crops, needleleaf, deciduous) based on whether the classification has a specific feature (value = 1.0), might have a specific feature (value = 0.5), does not have a specific feature (value = 0.0), or is unknown (value = −1.0). A full description of this intermediate classification system can be found in Appendix B2.
2.4 Machine learning method
All X-BASE products are based on gradient-boosted regression trees using the XGBoost library (Chen and Guestrin, 2016). XGBoost is known as a robust algorithm that is able to handle a variety of variable types (numeric, Boolean, categorical). Training was conducted using a two-thirds training subsampling ratio and a 0.05 learning rate. Hyperparameters were chosen based on preliminary testing to predict eddy covariance data, and a full list of hyperparameters can be found in Appendix B3. Boosting was stopped when no model improvement (based on mean squared error of validation data) was observed for 10 consecutive rounds, and the best-performing model was stored to generate predictions. In all cases, the model reached the stopping criteria relatively quickly, with the final number of boosting rounds between 80–230, depending on the flux.
2.5 Cross-validation
All cross-validation was performed using a 10-fold, leave-site-fold-out scheme, where each fold was constructed by randomly assigning each site to a fold. Leave-site-fold-out is distinct from traditional leave-one-out cross-validation as each fold is comprised of independent sites rather than fully randomized individual points. For each round of cross-validation, eight folds were used for training, one for validation, and the remaining one as the test fold for which the actual predictions were made. The leave-site-fold-out scheme ensures that no data from the sites in the test fold were ever seen by the algorithm during training and in turn iterated such that each site was in the test set once. As eddy covariance sites are sometimes clustered in the same location (e.g., as different treatments) and can therefore be both physically closely located and not truly independent, sites are assigned to the same fold if they are less than 0.05° apart to reduce overfitting. We evaluate the accuracy of the cross-validation models by computing the Nash–Sutcliffe modeling efficiency (NSE, Nash and Sutcliffe, 1970), where a negative NSE indicates a model accuracy that is worse than a mean prediction, while a value close to 1 indicates high model accuracy. We compute the NSE for each site and for a range of temporal scales from hourly to interannual.
2.6 Upscaling
The final step to train a model to use in the final global prediction step was identical to the training in the cross-validation, with the exception that, because no test fold was required, we used 9 of the 10 folds for the training, and validation was done on the remaining fold. The final trained models (one trained model for each target flux) were then used to predict fluxes at the global scales using the associated globally gridded input variables that correspond to those used at site level, as outlined in Table 2.
2.7 Previous FLUXCOM and independent global flux estimates
We compare X-BASE with upscaling results from FLUXCOM (Jung et al., 2019, 2020). As mentioned earlier, FLUXCOM comprised an ensemble of upscaling experiments that differed in the choice of machine learning method and meteorological forcing data. The FLUXCOM ensemble was summarized in two groups of setups that shared the same predictor variables and spatiotemporal resolution: the “remote-sensing-only” setup (RS) mostly used spaceborne observations of MODIS as explanatory variables and produced flux estimates every 8 d at 0.083° resolution, while the “remote sensing plus meteorology setup” (RS + METEO) produced daily flux estimates at half-degree resolution from meteorological predictor variables and an average seasonal cycle of satellite observations (Tramontana et al., 2016; Jung et al., 2019, 2020). Comparisons to FLUXCOM RS + METEO data sets always refer to the ensemble over multiple machine learning methods for all realizations driven by the ERA5 meteorology (Hersbach et al., 2020). RS + METEO uses average seasonal cycles of MODIS c005 observations. For the FLUXCOM RS setup we use the ensemble over all machine learning methods. Please note that both the previous RS runs and the X-BASE runs presented here are driven by data from MODIS c006, but the processing has changed in some aspects such as quality control and gap filling. For clarification, an overview on FLUXCOM(-X) naming conventions is given in Appendix A.
For evaluating X-BASE NEE globally, in particular its seasonal cycle and for different regions, we used two different atmospheric inversion model products: the Orbiting Carbon Observatory-2 (OCO-2) v10 model intercomparison project (Byrne et al., 2023) and the CarboScope inversion (Rödenbeck et al., 2018) version s99oc_v2022 (Roedenbeck and Heimann, 2022). Estimates from the OCO-2 came from the LNLGIS experiment, which combines satellite-based column-averaged CO2 (XCO2) retrievals and in situ CO2 measurements as observational constraints in the assimilation and consists of 13 different ensemble members covering the period 2015–2020 with a monthly frequency and 1° spatial resolution (https://gml.noaa.gov/ccgg/OCO2_v10mip/index.php, last access: 6 May 2022). The CarboScope product consisted of a single inversion output at the same spatial resolution as OCO-2 but with a longer temporal period from 2001–2020. In each case, as the inversion products estimate net biome exchange, we subtracted from the inversions data fire emissions as estimated by the Global Fire Emissions Database, Version 4.1 (Randerson et al., 2017).
We compared temporal patterns of X-BASE GPP with the patterns in global retrievals of sun-induced chlorophyll fluorescence (SIF) from the Sentinel-5P TROPOMI instrument (Köhler et al., 2018), which under most conditions approximates the variability in GPP. For the comparison we used estimates of daily mean SIF applying a correction factor to instantaneous observations (Zhang et al., 2018) and averaged both X-BASE GPP and TROPOMI SIF to a temporal resolution of 16 d and 0.5° spatial grids for the common period April 2018 to December 2020.
X-BASE ET and ETT were cross-compared with transpiration estimates from the Global Land Evaporation Amsterdam Model (GLEAM) v3.6a (Martens et al., 2017; Miralles et al., 2011). GLEAM also utilizes satellite and reanalysis data sets but in a more physically constrained way, relying on semiempirical models such as the Priestley–Taylor (Priestley and Taylor, 1972) and Gash models (Gash, 1979). Further comparisons were made to precipitation data from GPCC (Schneider et al., 2022).
2.8 Units across scales and conversion
Given the broad range of spatial and temporal scales reported here, we utilized different units depending on each use case. All base measurements and predictions are at hourly timescale (in units of µmol CO2 m−2 s−1 for carbon fluxes and mm h−1 for water fluxes; as reported in Table 2). In the case of carbon fluxes, all timescales which are not subdaily are reported in grams of carbon per unit time and area. Globally integrated values are reported in petagrams of carbon or square kilometers of water and are aggregated using a common land area estimate per grid cell.
3.1 Cross-validation and data space
One important innovation in FLUXCOM-X compared to the previous FLUXCOM ensemble was the training database, which was larger due to an increase in both number of sites and years. Furthermore, the EC methodology has changed considerably in many aspects ranging from collection and processing to quality filtering in the last 15 years. We show here one illustrative example of the changes in the environmental space that is represented in the training samples for daily NEE: between daily vapor pressure deficit (VPD) and daily incoming shortwave radiation, the distribution of training samples was considerably broader in X-BASE compared to the RS + METEO ensemble (Fig. 1). Furthermore, the number of unique sites contributing to a certain VPD-radiation bin has increased (Fig. C1); i.e., the number of ecosystems sampled in each climatic condition has also increased. The increases were seen particularly at the margins of the distribution, i.e., for days with high VPD along the full radiation spectrum, and vice versa for days with high-radiation conditions along the full VPD spectrum. Of particular note, Figs. 1 and C1 demonstrate improved sampling across more sites with conditions of high VPD and radiation, i.e., hot and dry conditions, for X-BASE compared to RS + METEO. Overall for NEE, the number of sampled site days increased over 3-fold (552 878 to 183 216 for X-BASE and RS + METEO, respectively). Note, however, that X-BASE is modeled at an hourly instead of daily resolution, and thus the number of sampled site days should not be considered a metric of how well the feature space is sampled.
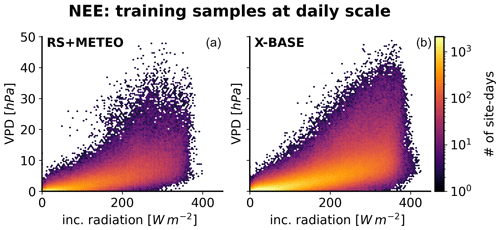
Figure 1Cross-validation sampling in meteorological space: number of site days contributing to sampling for NEE for the previous FLUXCOM RS + METEO ensemble (a) compared to the sampling of FLUXCOM-X-BASE (b) in environmental space of daily aggregated incoming shortwave radiation and VPD. Color corresponds to number of site days per bin in log scale. Only bins with at least 20 site days are shown.
The results from the 10-fold cross-validation showed an overall high performance with most fluxes and scales of variability having an NSE above 0.6 (Fig. 2). In terms of scales of variability across all fluxes, the monthly mean diel cycle (“diel”) and the daily median seasonal cycle (“seasonal”) were very regular patterns that the trained models reproduced best. Also, among-site changes (“spatial”, except for NEE) and monthly aggregated fluxes (“monthly”) were reliably predicted. Deviations from the median daily seasonality (“anom”) were only moderately reliable with NSE between 0.25 and 0.5. The XGBoost models did not succeed in accurately reproducing interannual variability of all fluxes and between-site patterns in NEE. Consistently across all scales, the net fluxes which are directly calculated (i.e., ET and even more so NEE) showed lower performance than their respective modeled gross fluxes (i.e., GPP and ETT). Note that the cross-validation results from Fig. 2 cannot be quantitatively compared to previous cross-validation results in FLUXCOM as the training data are not the same. However, qualitatively the accuracy gradient among fluxes, as well as along scales of variability, corresponded to patterns identified in FLUXCOM and in comparable empirical modeling activities (Jung et al., 2011; Tramontana et al., 2016; Virkkala et al., 2021; Dannenberg et al., 2023).
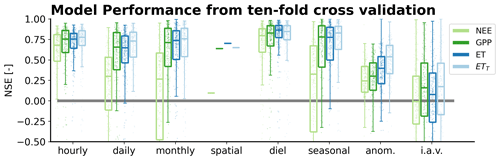
Figure 2FLUXCOM-X-BASE site-level accuracy of predicted fluxes in 10-fold leave-site-fold-out cross-validation in terms of NSE computed per site for a range of scales of variability. Scales of variability include the hourly timescale (“hourly”), daily (“daily”) and monthly (“monthly”) aggregated fluxes, between-site changes (“spatial”), monthly mean diel cycle (“diel”), daily median seasonal cycle (“seasonal”), deviations from the median daily seasonality (“anom.”), and interannual variability (“i.a.v.”). Boxes denote the range from the 25th to the 75th percentile of sites; whiskers extend 1.5 times the interquartile range from the 25th and 75th percentile of NSE across sites.
3.2 Global flux estimates
One asset of FLUXCOM-X is flexibility in the spatiotemporal resolution of the flux estimates. We produce X-BASE products at 0.05° spatial and hourly temporal resolution globally. Figure 3 illustrates the increase in spatial and temporal detail in X-BASE compared to RS (0.083°, 8-daily) and RS + METEO (0.5°, daily) using the example of NEE.
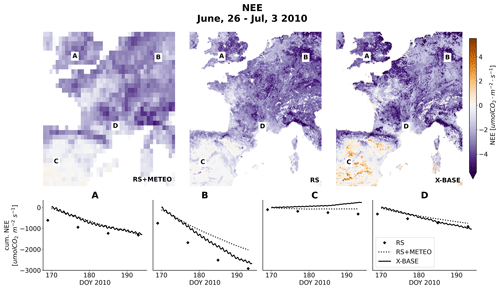
Figure 3Resolution improvements for the X-BASE products compared to RS and RS + METEO: average NEE for an 8 d period in Europe in 2010 as estimated from the RS, RS + METEO and X-BASE setups (top panel), as well as snapshots of temporal trajectories of NEE in pixels closest to selected EC station locations (A: UK-Tad, B: DE-Hai, C: ES-LM1, D: FR-Pue). Negative values of NEE denote a CO2 flux from the atmosphere to the land.
3.2.1 Net ecosystem exchange (NEE)
The X-BASE product estimates the global terrestrial NEE to be −5.75 ± 0.33 Pg C yr−1 (2001–2020), with hotspots of strong CO2 flux into the ecosystems in the tropical regions and temperate regions of North America and Europe (Fig. 4). In contrast to both RS and RS + METEO, India and some regions in central Sahel show prominent patterns of a mean CO2 flux from the ecosystems to the atmosphere in X-BASE, corresponding mostly to crop-designated areas (Fig. C2). However, X-BASE global terrestrial NEE (−5.63 Pg C yr−1) agrees well with the inversion estimates of OCO-2 (−4.12 Pg C yr−1) and CarboScope (−3.46 Pg C yr−1) over the common period (2015–2020). Here, GFED 4.1 (Randerson et al., 2017) emission estimates have been used to correct the inversion estimates for fire emissions.
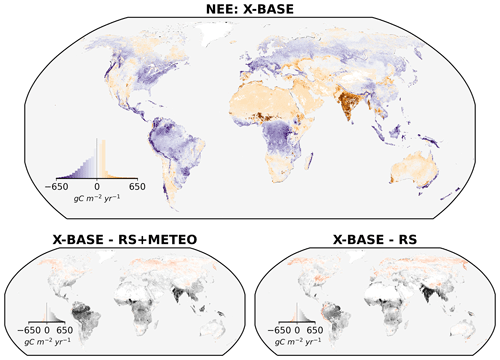
Figure 4Comparison of annually integrated NEE from X-BASE, RS + METEO with ERA5 forcing, and RS averaged over the period 2001–2020. The difference maps show the difference of the averages over 2001–2020.
Comparison with OCO-2 and CarboScope inversions also indicates a substantial improvement of the global mean seasonal cycle of NEE (Fig. 5) in X-BASE compared to RS and RS + METEO. The systematic bias present in RS and RS + METEO has essentially disappeared in X-BASE. The shape, and in particular the amplitude, of the global NEE seasonal cycle of X-BASE is more consistent with the inversions. The larger and more realistic seasonal cycle amplitude of global NEE in X-BASE originates primarily from improved and increased amplitudes in boreal regions. Interestingly, X-BASE suggests slightly larger NEE seasonal cycle amplitudes in temperate regions compared to the inversions. In seasonally dry regions, the timing of maximum uptake is consistent between X-BASE and inversions, while the peak of maximum net release is larger and delayed in the inversions. In Australia, the peak of CO2 release to the atmosphere at the end of the year present in both inversions is not evident in X-BASE, which instead shows a relatively consistent CO2 flux to the atmosphere throughout the year. In tropical regions, the patterns of seasonal variations are qualitatively consistent between X-BASE and the previous RS and RS + METEO products. The seasonal patterns in tropical regions are relatively weak overall and seem inconsistent between the inversions and X-BASE as well as among the inversions.
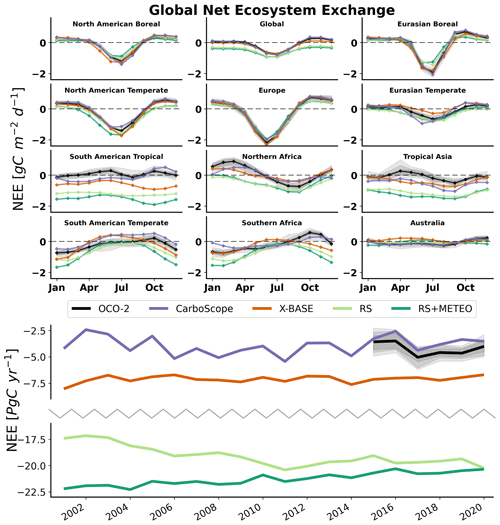
Figure 5Seasonal and interannual variability of global NEE. Comparison of mean seasonal cycles (calculated over the common time period, 2015–2020) and interannual variability (2001–2020) of NEE estimated from CarboScope and OCO-2 inversions as well as FLUXCOM-X-BASE, FLUXCOM RS + METEO, and RS outputs. All products were integrated with a common mask that removes sparsely vegetated arid regions not predicted by RS and RS + METEO.
As shown in Fig. 5, the X-BASE product shows the same large underestimation of globally integrated NEE interannual variance as the previous RS and RS + METEO products. In terms of temporal trends, the X-BASE products show almost no change in annual NEE in time, which is in contrast to the RS + METEO (slight positive trend) and RS (slight negative trend) and more consistent with the CarboScope inversions (Table C2). However, as interannual variability was poorly reproduced even in the cross-validation (Fig. 2), trends in the X-BASE products should be taken with caution and interpreted with careful scrutiny.
3.2.2 Gross primary productivity (GPP)
X-BASE estimates the globally integrated GPP at 124.7 ± 2.1 Pg C yr−1 on average in the time period 2001–2020. Globally integrated GPP over vegetated areas (RS and RS + METEO do not have estimates for non-vegetated areas) was approximately equal for X-BASE (121.9 ± 2.1 Pg C yr−1) and RS + METEO (121.6 ± 0.4 Pg C yr−1) but considerably higher than RS (113.2 ± 1.8 Pg C yr−1) over the same period. In terms of regional patterns, X-BASE GPP consistently exceeds both RS + METEO and RS in temperate, boreal, and most subtropical ecosystems but is lower in sparsely vegetated (semi-)arid regions like southwestern North America as well as southeastern Asian croplands (Fig. 6). This qualitatively consistent pattern is only broken in the humid tropics, where X-BASE GPP is higher than RS but lower than RS + METEO.
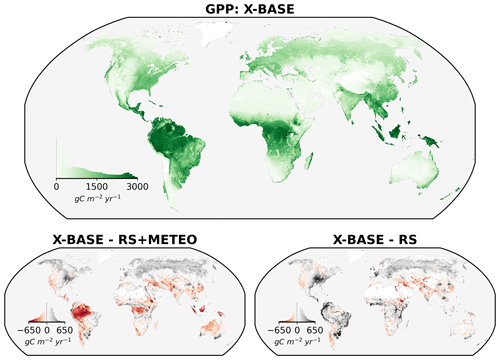
Figure 6Comparison of annually integrated GPP from X-BASE, RS + METEO with ERA5 forcing, and RS averaged over the period 2001–2020. The difference maps show the difference of the averages over 2001–2020.
Comparing the estimated trend over the last 2 decades, X-BASE GPP has a clear increasing linear trend of 0.34 Pg C yr−1, which is slightly higher than the trend in RS (0.25 Pg C yr−1; Table C2). In contrast, the RS + METEO product shows nearly no trend in annual GPP. The increases in both the X-BASE and RS products may be related to increases in surface greenness coming from variability in the remote sensing forcing data which are interannually dynamic in both products, whereas the remote sensing data were not interannually dynamic in the RS + METEO product, which instead used only the mean seasonal cycle of the remote sensing data. The magnitude of between-year changes in globally integrated X-BASE GPP is 0.575 Pg C yr−1 over the years 2001–2020, which is about twice as large as RS + METEO (0.248 Pg C yr−1) but only half the magnitude estimates in the RS setup (1.02 Pg C yr−1; Table C2).
We further compared the temporal trajectory in GPP estimates against TROPOMI SIF as an independent proxy for GPP dynamics (Fig. 7) at a temporal resolution of 16 d. The temporal variability of X-BASE GPP strongly agrees with that in TROPOMI SIF, with squared Spearman correlation values (denoted as R2) of the time series above 0.85 across most of the vegetated land surface (Fig. 7a). The only exceptions are regions with no or very small variability in both GPP and SIF, such as in evergreen tropical ecosystems in South America, Africa, and southeastern Asia; sparsely to non-vegetated areas due to aridity (e.g., Mexican and African deserts); or cold conditions (e.g., Canadian and Siberian subpolar regions). In inner Australia, despite being sparsely vegetated, variability between years is expected in GPP due to precipitation increases during La Niña years, which is however not reflected in the squared correlations. R2 values for the deviations from the average seasonality (again computed with a temporal resolution of 16 d) show the same qualitative spatial patterns (Fig. 7b) but are overall lower with R2 values between 0.55 and 0.8. Anomalies of X-BASE GPP and SIF agree best in eastern European temperate forests as well as in grassy and shrub ecosystems in eastern South America.
Comparison of the level of agreement of SIF and X-BASE with that of SIF and RS and RS + METEO, respectively, illustrates that X-BASE and RS GPP estimates have comparable consistency both for the time series (global area weighted mean R2 values of 0.72 and 0.73, respectively) and anomalies (global mean R2 values of 0.64 and 0.66, respectively). In contrast, the R2 between RS + METEO and SIF is lower in both cases (R2 values of 0.66 for the time series and 0.58 for anomalies). X-BASE GPP shows a higher agreement with SIF than RS both in terms of the actual trajectory and anomalies in evergreen tropical forests with no or only a very short dry season in the Amazon and Africa, as well as in fully humid parts of southeastern Asia (Fig. 7c, d). Improvements in X-BASE GPP compared to RS are also consistent in the very continental and polar tundra areas in eastern Siberia, northern Canada, and Alaska. Conversely, in arid steppe climates globally, X-BASE GPP variability agrees less with SIF than does RS GPP. X-BASE GPP variability is consistently and widespread much more similar to the variability in TROPOMI SIF than RS-METEO GPP. Increases in R2 for X-BASE compared to RS + METEO are most pronounced in arid to semiarid ecosystems (large parts of the Caatinga and Gran Chaco regions in South America, steppe regions in Mexico, southern and eastern Africa, Australia, and central Siberia) as well as in global crop regions, especially for the deviations from the seasonality (albeit with the magnitude of R2 change being quite variable between regions; Fig. 7e, f).
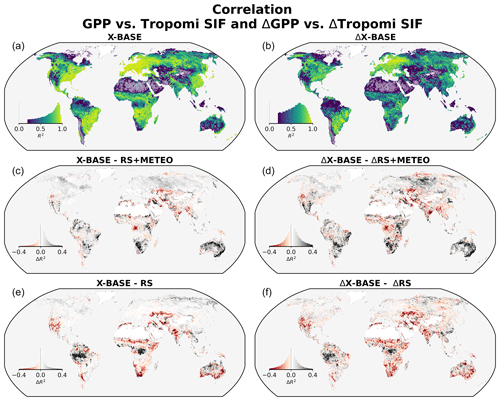
Figure 7Similarity of temporal patterns between GPP estimates and TROPOMI SIF observations: R2 (computed as the square of the Spearman correlation) between X-BASE GPP and TROPOMI SIF (Köhler et al., 2018) for the actual time series at a temporal resolution of 16 d (a) and anomalies from the median seasonality in both variables (b). Panels (c), (d), (e), and (f) compare the agreement between X-BASE GPP and TROPOMI SIF to the agreement between FLUXCOM GPP and TROPOMI SIF, where (c) and (d) refer to TROPOMI SIF and GPP from the RS + METEO setup, and (e) and (f) refer to the RS setup. All comparisons are done for time series with a resolution of 16 d for the common time period April 2018 to December 2020. SIF observations have been applied a correction factor to estimate daily average SIF before aggregation. Semitransparent areas mark pixels in which the correlation of at least one of the data sets is negative.
3.2.3 Water vapor fluxes
Globally integrated ET amounts to 74.7×103 ± 0.9×103 km3 yr−1 for 2001–2020 (Table C1) for X-BASE, with the highest rates in the tropics (Fig. 8). Comparison of global totals for vegetated areas only (where all products give outputs) shows similar values for X-BASE (68.9×103 ± 0.9×103 km3 yr−1), GLEAM (70.9×103 ± 0.9×103 km3 yr−1), and RS + METEO (68.3×103 ± 0.3×103 km3 yr−1) ET estimates, while the RS ET is more than 11 % higher (78.5×103 ± 0.5×103 km3 yr−1; Table C1). Particularly in evergreen tropical ecosystems, X-BASE estimates a considerably lower ET than GLEAM, RS + METEO, and RS (Fig. 8). Furthermore, in the temperate and high latitudes of the Northern Hemisphere, annually integrated X-BASE ET is consistently lower than the other estimates, though the magnitude of the bias is smaller than in the tropical regions. The pattern is only reversed with higher X-BASE ET in the semiarid and arid ecosystems of the lower and middle latitudes, especially with respect to annual ET in RS + METEO and GLEAM.
Comparison to precipitation estimates shows that X-BASE ET greatly exceeds precipitation inputs over large areas, indicating a strong overestimation of X-BASE ET in many arid regions with sparse vegetation (e.g., the Sahara region; Fig. C3). While transport of water both laterally (i.e., runoff from other areas) and from deeper groundwater could cause ET to exceed precipitation inputs in some areas, the extent of area where ET exceeds precipitation (e.g., the entire Sahara region) and the magnitude of the excess ET (over 3 times precipitation inputs) indicates a major bias in these areas and is likely due to a lack of EC data in similar ecosystems. As a rough estimate, constraining the X-BASE estimates with precipitation (see Appendix C5) suggests about 4–6×103 km3 yr−1 of water is overestimated globally.
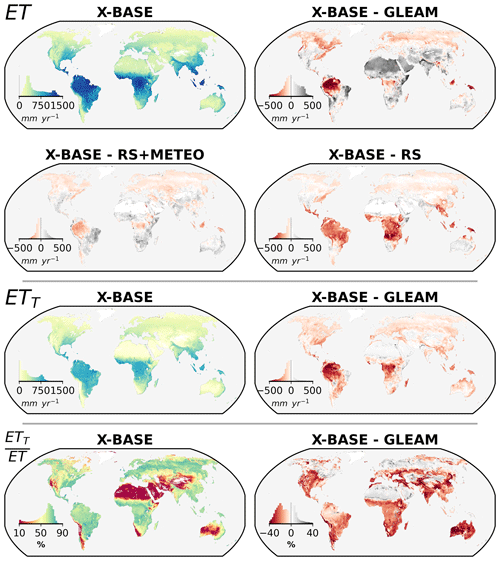
Figure 8Comparison of evaporative flux estimates ET, ETT, and ETT ET from X-BASE and its difference with RS, RS + METEO, and GLEAM. ETT is compared in the case of GLEAM but is unavailable in the previous FLUXCOM ensembles.
The globally integrated ETT amounts to 42.6×103 ± 1.0×103 km3 yr−1 (2001–2020) in X-BASE, resulting in an average ratio of transpiration to total evaporation of 57.0 % ± 0.6 % (Table C1). In contrast to ET, the ETT estimates from X-BASE do not commonly exceed precipitation estimates (Fig. C3), which could indicate that because the water vapor flux is more tightly coupled with vegetation, the model is able to distinguish that no vegetation corresponds with no transpiration, which is not generally the case for non-transpiration evaporation. The RS and RS + METEO products did not produce ETT estimates, so the comparison is limited to GLEAM (50.7×103 ± 0.6×103 km3 yr−1), which estimates ETT on average 17 % higher than X-BASE, with strong contributions from the evergreen tropics. Only in single semiarid regions, such as the northernmost Sahel as well as large parts of the South American Caatinga and Chaco regions, is this pattern reversed (Fig. 8).
Spatially, X-BASE-estimated ETT ET exceeds 50 % in the majority of areas, with the highest values seen in the higher-latitude regions of Europe and Asia, as well as in subtropical ecosystems (Fig. 8). Arid regions with sparse vegetation show the lowest ETT ET overall, with values generally below 20 %. With 71.4 % ± 0.6 % over global vegetated surfaces, GLEAM attributes about 10 % more of its ET to ETT than does X-BASE (Table C1). Regionally, this difference can even reach up to 40 %, with the only exception being boreal forests and very dry ecosystems in the Sahel, the Arabian Peninsula, and central Asia (Fig. 8).
Trends in ET, ETT, and ETT ET are positive and exceed the trends seen in all other estimates over the years 2001–2020. Conversely, the magnitude of interannual changes in X-BASE ET, ETT, and ETT ET is mostly less than half the variability in GLEAM (Table C2). Low interannual changes are common to the RS and RS + METEO ET as well.
Figure 9 shows the temporal correlation at 16-daily temporal scale using GLEAM as a reference, showing overall high values of squared correlation between X-BASE and GLEAM ET and ETT (top and bottom left). Notable exceptions with low correlations are areas with low variability in ET such as the arc of deforestation, very dry areas, and tropical evergreen ecosystems in Africa. Compared to RS + METEO and RS (middle panels, left column in Fig. 9), X-BASE ET temporal patterns are more similar to GLEAM ET in many areas, especially so in areas north of the arc of deforestation and parts of tropical evergreen areas in central Africa and southeastern Asia. Conversely, X-BASE ET agrees less well with GLEAM than RS or RS + METEO in the arc of deforestation itself, the eastern parts of the Amazon basin, and dry areas. The deviations from the mean annual cycle in ET and ETT (right column) show overall lower correlations than the actual time series, with the highest agreement between GLEAM and X-BASE in large parts of the Amazon forest and central European ecosystems. X-BASE ET anomalies are much more strongly correlated with GLEAM ET than either RS or RS + METEO everywhere except for most (semi-)arid regions.
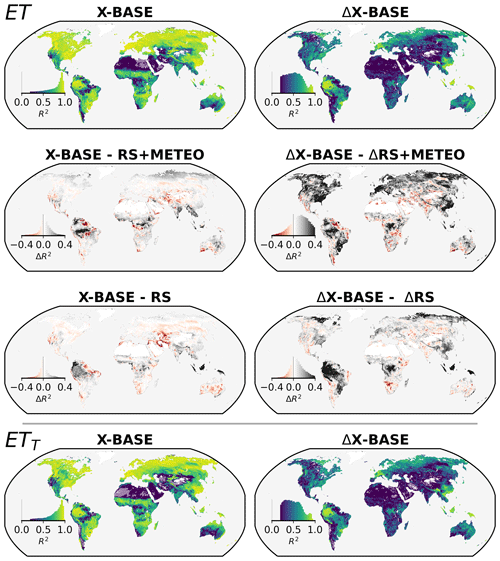
Figure 9Similarity of temporal patterns of X-BASE and GLEAM terrestrial water fluxes in comparison to those from FLUXCOM: R2 (computed as the square of the Pearson correlation) between X-BASE ET and GLEAM ET for the actual time series (left column) and anomalies from the median seasonality (right column). The middle panels compare the agreement between X-BASE ET and GLEAM ET to the agreement between FLUXCOM ET and GLEAM ET. The bottom panel shows the squared correlations between X-BASE and GLEAM ETT but no comparisons to FLUXCOM because FLUXCOM did not include ETT. All comparisons are done for time series with a resolution of 16 d and 0.05° for the years 2001–2020. Semitransparent areas mark pixels in which the correlation of at least one of the data sets is negative.
We have presented qualitative and quantitative comparisons of the X-BASE products with previous FLUXCOM estimates and independent data sets where possible. Statements on the main causes of (dis-)agreements in the magnitude and spatiotemporal variability in the flux estimates remain speculative as detailed analyses would require further investigation in the form of experiments, which is beyond the scope of this article. In the following we discuss the prominent changes in NEE compared to FLUXCOM as a key scientific highlight in X-BASE and outline potential ways forward to tackle persistent challenges in empirical upscaling.
4.1 Higher consistency of NEE with atmospheric carbon cycle constraints
Although FLUXCOM-X follows the same fundamental approach as FLUXCOM, we find a substantial improvement of the magnitude of the annually integrated NEE of FLUXCOM-X-BASE over previous FLUXCOM products (Jung et al., 2020) when compared to independent estimates from atmospheric inversions. The mean global X-BASE NEE of −5.75 Pg C yr−1 is slightly smaller than the inferred NEE of −3.92 Pg C yr−1 (corrected for fire emissions based on GFED 4.1) from CarboScope. The remaining difference could easily be explained by carbon sources such as aquatic evasion and volatile organic compounds that are included in the atmospherically based estimate but not in eddy-covariance-based FLUXCOM (see Jung et al., 2020, and Zscheischler et al., 2017, for further discussion).
The improved global NEE of FLUXCOM-X-BASE originates most likely from enhanced quality of eddy covariance measurements in the training resulting from years of consistent improvements in instrumentation, method development, and quality assurance from the point of measurement to the synthesis data sets. Previous upscaling-based NEE products of Jung et al. (2011, 2020); Bodesheim et al. (2018) – all based on the La Thuile FLUXNET data set but varying with respect to machine learning methods, predictor variables, and temporal resolution – consistently estimated a nearly 3-fold larger global terrestrial carbon uptake compared to X-BASE. As discussed and speculated in Jung et al. (2020), La Thuile likely contained biased NEE measurements, in particular for some tropical sites (Fu et al., 2018), and together with the sparsity of data in the tropics, these biases were propagated to unrealistic tropical and global NEE estimates. The fact that we can now reconcile bottom-up global eddy-covariance-based NEE and estimates from top-down atmospheric inversions is a major achievement of the FLUXNET community. For context, 1 Pg C yr−1 over the global vegetated area (145×106 km2) corresponds to ∼ 7 g C m2 yr−1, which marks a challenge for achieving such an accuracy of mean NEE at any one flux tower site. The lesson learned here emphasizes once more that it is crucial to control for and minimize systematic biases of in situ eddy covariance measurements (Moncrieff et al., 1996).
The improved seasonality of X-BASE NEE, in particular for boreal regions, likely also results from enhanced information in the training data due to the hourly resolution. Similar improvements were observed by Bodesheim et al. (2018), who extended RS + METEO by training on half-hourly flux observations. The hourly resolution improves the seasonal high-latitude NEE likely due to better capturing the responses to light when day length varies strongly.
4.2 New opportunities by X-BASE products
The improvements of NEE make X-BASE attractive as a data-driven biogenic prior for atmospheric inversions (Munassar et al., 2022). Moreover, its hourly resolution facilitates better integration in inversion systems due to the accounting of diurnal flux and atmospheric transport variations, while its high spatial variations can provide patterns of flux variations that cannot be resolved by atmospheric constraints alone.
For the first time, X-BASE includes a global data-driven product of ecosystem transpiration. The estimated global ETT ET ratio of 57 % is consistent with independent top-down assessments from isotope base methods (Good et al., 2015; Coenders-Gerrits et al., 2014) and past upscaling estimates (Wei et al., 2017; Schlesinger and Jasechko, 2014). The spatially and temporally high-resolution data-driven X-BASE ETT product provides a valuable complementary perspective to simulations from process-based land surface models, which show large disagreements and often indicate global ETT ET below 50 % (Berg and Sheffield, 2019; Miralles et al., 2016). This advancement opens new opportunities for large-scale studies of carbon–water relations on a diurnal timescale. The generation of the X-BASE ETT product was facilitated by the development of site-level evapotranspiration partitioning methods (Nelson et al., 2018, 2020) underlining once more the importance of advances by the FLUXNET community for Earth system science.
In addition to the improvements and opportunities that X-BASE brings through the enhancement in site-level training data quality and quantity as well as the higher spatiotemporal resolution, we find that some key issues previously identified in FLUXCOM (Tramontana et al., 2016; Jung et al., 2020; Bodesheim et al., 2018) persist in X-BASE. These include (1) the insufficient representation of water-related effects, (2) the limited predictability of the spatial patterns of mean NEE, and (3) severe limitations with respect to the variability between years and over decades. In the following, we outline what we consider the most promising ways forward to tackle these (partly interrelated) challenges in future developments beyond X-BASE: novel additional predictor variables, more in situ observations (especially for some key ecosystems), and innovative approaches to constraining machine learning models.
The persistent challenge and importance of capturing water effects on land–atmosphere flux variations is illustrated by the overestimation of mean ET in very dry, sparsely vegetated areas (Fig. C3), as well as by the poorer consistency of NEE seasonality with inversions in water-limited regions (Fig. 5). For GPP temporal patterns we find that X-BASE shows improved agreement with SIF in water-limited regions compared to RS + METEO (Fig. 7), which is likely because X-BASE uses concomitantly changing remote sensing observations opposed to a mean seasonal cycle only in RS + METEO. However, X-BASE shows deteriorated agreement with SIF when compared to RS, even though X-BASE was trained on hourly flux observations with improved coverage of dry conditions (Fig. 1). This decrease in performance indicates clearly the importance and uncertainty related to the predictor variable set for capturing water-related effects. Uncertainties due to water limitation effects are also seen in the divergence of ET and ETT estimates in semiarid regions such as the Sahel and South American Caatinga (Figs. 8 and 9). Thus, there is considerable potential for advancements by including remote-sensing-based predictors on soil moisture, subdaily varying land surface temperature from geostationary satellites, SIF, and vegetation optical depth. Here, key challenges reside in achieving a sensible integration of flux observations with footprints that are much smaller than corresponding Earth observation products on the one hand and an integration of disparate Earth observation products with each other on the other hand. This is one research priority for further FLUXCOM-X developments.
Missing predictor variables is likely also a main reason for the limited skill of predicting between-site variability of mean NEE (Fig. 2), which can depend on legacy effects of disturbances and management that are not accounted for. Novel and complementary Earth observation products that characterize ecosystem structure and states related to biomass and canopy heights from SAR and lidar should help raise the accuracy of FLUXCOM-X-based mean NEE in future efforts. Also high-resolution Earth observation products such as from Landsat may help to better resolve spatial details. X-BASE shows a prominent pattern of carbon flux to the atmosphere in subtropical and crop-dominated regions of India and the Sahel, which emphasizes the need to improve in situ data coverage for agricultural systems, especially outside the temperate zone, and to include important metadata to better characterize these ecosystems and their site history. Despite the greatly reduced overall bias of mean NEE, we emphasize that X-BASE products are premature for diagnosing spatial variations of mean NEE.
The representation of longer-term dynamics remains an area with opportunity for improvements in X-BASE. Interannual variability is still poorly reproduced in cross-validation (Fig. 2), particularly for NEE, which is likely not only due to the complexity of processes shaping interannual variations but also due to temporal discontinuities in flux tower time series related to changes in instrumentation and factors like management (Jung et al., 2024) that are not accounted for. The complexities of relying on field-deployed instrumentation, together with the uncertainties related to linking satellite and flux data, cause poor signal-to-noise ratios and may impede good cross-validation results for interannual variability. Globally, comparisons of X-BASE with inversions reveal an underestimated interannual variance and a poor correlation for global NEE interannual variability (Fig. 5 and Table C2). Interestingly, X-BASE GPP shows improved correspondence with SIF anomalies compared to RS + METEO, especially in water-limited regions (Fig. 7), while no such improvement is evident for global NEE, which is likely due to the compensatory water effects in the global NEE signal (Jung et al., 2017). That a comparison of RS + METEO runs with different meteorological forcing data showed the weakest correspondence with inversion interannual variability when using ERA5 (Jung et al., 2020) explains the substantially better correlations of RS + METEO with inversions for NEE interannual variability in earlier studies (Jung et al., 2017, 2020), and it may also explain the poor correlation with X-BASE. Thus, testing whether alternative meteorological forcing data can improve global NEE interannual variability for X-BASE is an important next step. It remains unclear at this point whether accurate interannual variations at site level and globally can be achieved by the FLUXCOM approach in the near future. Additional constraints beyond FLUXNET such as atmospheric CO2 measurements (Upton et al., 2023) or theoretical considerations in the form of hybrid (Reichstein et al., 2019) or deep learning models (Camps‐Valls et al., 2021) are promising, and we will foster such endeavors.
We presented X-BASE, a new set of global high-resolution data-driven products of land–atmosphere fluxes from the FLUXCOM approach. This represents a cornerstone of our developments of the FLUXCOM-X framework designed to explore and mitigate current limitations to upscaling from site to global scale. Improvements of the eddy covariance data facilitated reconciling estimates of global terrestrial net carbon exchange from X-BASE with top-down atmospheric inversions and allowed for the first time the generation of a global data-driven estimate of ecosystem transpiration. Beyond fostering all activities to enhance quality and coverage of available flux tower observations, most promise for future advancements by FLUXCOM-X relates to the synergistic exploitation of complementary satellite data streams to better capture effects related to water, site history, and management. This will be challenging as it requires developing strategies and methodologies to better integrate in situ flux observations and spaceborne Earth observations with very heterogeneous acquisition properties and with spatial resolutions that are often very coarse compared to flux tower footprints. The recent de-orbiting of the TERRA spacecraft requires employing alternative satellite missions where practical issues of data acquisition and conceptual issues related to temporal consistency and reduced overlap with FLUXNET records pose imminent challenges. With FLUXCOM-X we have prepared the ground for tackling these challenges, which can facilitate up-to-date and accurate flux estimates and thereby contribute to increased understanding of the Earth system in the future.
B1 Dynamic quality control and cutout size
The conditions in the pixels around a given EC station should best represent the conditions of the land surface in the area where the actual fluxes originate from. Given that the actual flux footprints are not generally available or computable for lack of critical information, we assume that the pixel containing the actual EC station (the “tower pixel”) is most representative of the dynamics of the area of influence on a tower. However, data availability and quality in the tower pixel are often insufficient. An iterative approach therefore selects both the cutout size and the strictness of the BRDF inversion quality from within defined bounds in a way that maximizes data availability and that ensures representativeness of the spatially averaged time series for the given site at the same time. In more detail, we start with a strict criterion for BRDF inversion quality (BRDF_Albedo_Band_Quality_Bandx flag in MCD43A2 ≤2, meaning only full inversions). Then, three options regarding the cutout size are considered:
-
A cutout option only considers the tower pixel, i.e., the single pixel closest to the tower location.
-
Those 20 % of pixels within 4×4 km2 around a tower that are best correlated with the tower pixel are linearly regressed against the tower pixel and subsequently spatially averaged.
-
The 25 % of pixels within a 4×4 km2 area that are closest to the tower are averaged with the inverse of the distance to the tower as weight.
Table B1Land cover intermediary classification encoding for MCD12C1 classifications where the classification has a specific feature (value = 1.0), might have a specific feature (value = 0.5), does not have a specific feature (value = 0.0), or is unknown (value = −1.0).
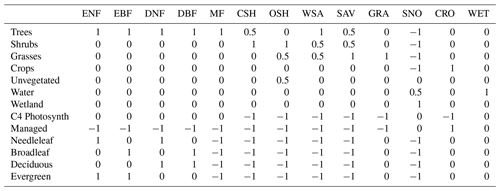
The criteria for selection between options A–C are based on the number of available good-quality observations n in the resulting spatial average time series per site as follows:
if (n_A >= 60 %) and (n_B <= 70 %), select A; elif (n_A >= 60 %) and (n_B >= 70 %), select B; elif (n_A < 60 %) and (n_A > 15 %), select B; else select C.
If after the previous steps still less than 40 % of good-quality observations outside of snow-covered times are available in the resulting average time series for a given site and index, the BRDF inversion quality threshold is relaxed to also allow magnitude inversions (MCD43A2 BRDF inversion quality flag ≤3), and the procedure to select the pixels contributing to the average described above is repeated. Consequently, the size of the area that a MODIS reflectance time series represents varies between sites and so does the BRDF inversion quality.
For the global gridded MODIS data, the BRDF inversion quality is consistently selected as ≤2 or ≤3 based on the number of available good-quality observations in a pixel.
B2 Details on the treatment of land cover information
Land cover information was passed through an intermediary classification system both to act as an encoding mechanism and to allow for arbitrary links between classification schemes. Rather than simple true/false classification for each category, different attributes are classified based on whether the classification has a specific feature (value = 1.0), might have a specific feature (value = 0.5), does not have a specific feature (value = 0.0), or is unknown (value = −1.0). In the specific case of the MCD12C1 classification scheme, the conversion is as shown in Table B1.
B3 Overview of XGBoost hyperparameters used
C1 Additional cross-validation results
C3 Global magnitude of all fluxes
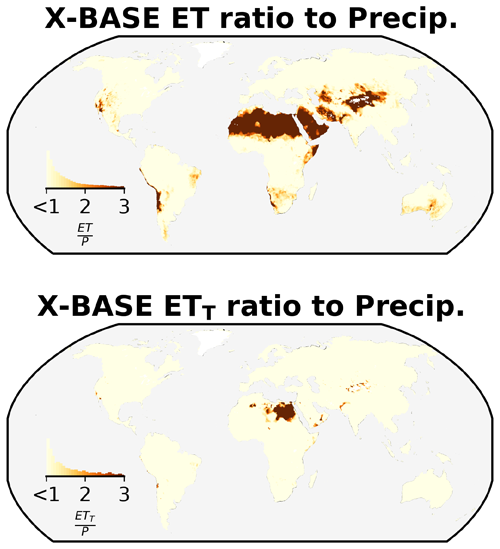
Figure C3Potential ET overestimation based on the ratio of estimated ET to precipitation from the Global Precipitation Climatology Centre (GPCC; Schneider et al., 2022).
Table C1Global magnitude of all fluxes. The first column is the globally integrated flux for all areas including sparsely vegetated dry areas from 2001–2020. The second column includes a common mask, which removes sparsely vegetated areas that are not computed for the RS and RS + METEO products. Values reported after the ± correspond to the standard deviation across years.
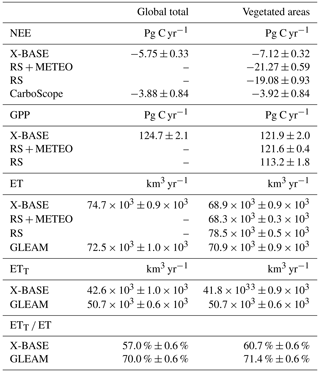
C4 Linear trends and interannual variability for all fluxes
C5 Potential overestimation of ET in dryland areas
Maps in Fig. C3 show the extent to which ET and ETT exceed precipitation as the ratio of the total of each flux to total precipitation (from GPCC Schneider et al., 2022). Overall, X-BASE ET largely exceeds precipitation in most dry, sparsely vegetated areas, indicating overestimation. In contrast, ETT does not show such extensive overestimation, limited instead to only smaller regions of the Sahara.
The amount of overestimation of X-BASE ET can be roughly estimated by replacing areas where annual ET exceeds precipitation inputs with the corresponding annual precipitation inputs for each grid cell, i.e., replacing areas where the ET precipitation ratio is more than a threshold with the precipitation rather than the estimated ET. Using thresholds from 1.25 to 2.5 gives an excess of ET (i.e., original ET minus precipitation corrected) from 3.9×103 to 6.1×103 km3 yr−1.
All FLUXCOM-X-BASE data covering the years 2001–2021 (this article only shows 2001–2020) are available as aggregated NetCDF file formats, to ease data handling for common use cases, from the ICOS Carbon Portal (https://doi.org/10.18160/5NZG-JMJE, Nelson et al., 2023). Furthermore, the full-resolution data are accessible in Zarr format and in a publicly available object store provided by the German Climate Computing Center (Deutsches Klimarechenzentrum, DKRZ). Instructions on how to access all data, as well as the full data set, can be found at the associated repository (https://gitlab.gwdg.de/fluxcom/fluxcomxdata, Nelson, 2023).
JAN, SW, and MJ wrote the manuscript, and all authors contributed to the editing and structuring of it. JAN, SW, FG, BK, and ZH wrote the code, which constitutes the modeling environment FLUXCOM-X, and structured it according to discussions with MJ, UW, GD, and MM. Editorial input was provided by AI, AK, AK, AS, BB, BK, DB, DP, FT, GCV, GD, GN, GW, JA, JC, JD, KI, KMK, KN, LH, LS, MK, MM, MR, NB, RLS, SS, TT, and WZ. All other authors contributed through their work at one or more eddy covariance stations, the acquisition of funding, setup and maintenance, preprocessing and quality control, administration, and their participation in regional or global networks.
At least one of the (co-)authors is a member of the editorial board of Biogeosciences. The peer-review process was guided by an independent editor, and the authors also have no other competing interests to declare.
Publisher’s note: Copernicus Publications remains neutral with regard to jurisdictional claims made in the text, published maps, institutional affiliations, or any other geographical representation in this paper. While Copernicus Publications makes every effort to include appropriate place names, the final responsibility lies with the authors.
We would like to thank the broader eddy covariance community, including FLUXNET and the associated regional networks, particularly the European Integrated Carbon Observation System (ICOS) and AmeriFlux. We also acknowledge the contributions of Andrej Varlagin and colleagues to these efforts. We thank the team at the ICOS Carbon Portal for their support in publishing the FLUXCOM-X data sets, with great thanks in particular to Ute Karstens and Zois Zogopoulos. We also thank Brendan Byrne and colleagues of Jet Propulsion Laboratory, California Institute of Technology, Pasadena, CA, USA, for use of the OCO-2 data.
The work of Sophia Walther, Jacob A. Nelson, and Martin Jung was funded in part by the European Union's Horizon 2020 research and innovation program (grant nos. 776186 CHE, 776810 VERIFY, 958927 CoCO2, 820852 E-SHAPE). Sophia Walther acknowledges funding from a European Space Agency Living Planet Fellowship in the project Vad3e mecum as well as the CCI LST project (4000123553/18/I-NB). Gregory Duveiller and Zayd Mahmoud Hamdi acknowledge funding from the European Space Agency in the Sen4GPP project (4000134598/21/I-NB). Simone Sabbatini and Gregory Duveiller acknowledge Horizon Europe funding (Open-Earth-Monitor Cyberinfrastructure project, 101059548). Donatella Zona acknowledges NSF award numbers 2149988 and 1932900. Anne Klosterhalfen and Alexander Knohl acknowledge funding by the German Federal Ministry of Education and Research (BMBF) as part of the European Integrated Carbon Observation System (ICOS), by the Deutsche Forschungsgemeinschaft (INST 186/1118-1 FUGG) and by the Ministry of Lower Saxony for Science and Culture (DigitalForst: Niedersächsisches Vorab (ZN 3679)). Leonardo Montagnani acknowledges funding provided by Forest Services, Autonomous Province of Bolzano. Enrico A. Yepez acknowledges that MX-Tes is part of the MexFlux regional network. Funding for the Swiss sites is greatly acknowledged from various sources: from the EU project SUPER-G (contract no. 774124), the SNF projects M4P (40FA40_154245), DiRad (146373), InnoFARM (407340_172433), CoCo (200021_197357), ICOS-CH (20FI21_148992, 20FI20_173691, 20FI20_198227), and InsuranceGrass (100018L_200918); from NESTLE via the ETH foundation (DONA); and from the ETH Board and from ETH Zurich (project FEVER ETH-27 19-1). Funding for US-BZB, US-BZF, US-BZo, and US-BZB was provided by National Science Foundation grant nos. DEB LTREB 1354370 and 2011257, DEB-0425328, DEB-0724514, and DEB-0830997, as well as funding by the US Geological Survey Climate R&D program. Bonanza Creek Long Term Experimental Research station provided lab space and equipment. US-ICs and US-ICt were supported by grants from the Arctic Observatory Program of the National Science Foundation (grant nos. 1936752, 1503912, 1107892). Sergio Aranda-Barranco acknowledges projects PID2020-117825GB-C21 and PID2020-117825GB-C22 funded by MCIN/AEI/10.13039/501100011033, as well as support by the FPU grant by the Ministry of Universities of Spain (REF: FPU19/01647). SE-Deg, SE-Svb, and SE-Ros acknowledge funding from the Swedish Research Council and contributions from research institutes to the Swedish Integrated Carbon Observation System (ICOS-Sweden) Research Infrastructure and the Swedish Infrastructure for Ecosystem Science (SITES). Torbern Tagesson was funded by the Swedish National Space Agency (SNSA Dnr 2021-00144) and FORMAS (Dnr. 2021-00644). William Woodgate is supported by an Australian Research Council DECRA Fellowship (DE190101182). Ivan Mammarella acknowledges funding from Academy of Finland (N-PERM 341349), ICOS-Finland UH, and EU projects (GreenFeedBack 101056921, LiWeFor). Domenico Vitale acknowledges the Integrated Carbon Observation System – Research Infrastructure (ICOS ERIC, https://www.icos-cp.eu/, last access: 3 October 2024) and the ICOS ETC funding from the Italian Ministry of Research. Mathias Göckede was supported by the European Research Council (ERC) under the European Union's Horizon 2020 research and innovation program (grant agreement no. 951288, Q-Arctic). The DE-Geb site received funds within the ICOS Germany preparatory and implementation phase by the Federal Ministry of Education and Research and is supported by the Ministry of Digital and Traffic through ICOS station contributions as well as by the Ministry of Food and Agriculture covering operational costs. Giacomo A. Gerosa thanks the Catholic University of Brescia for continuous supporting the research station of Bosco Fontana (ICOS station IT-Bft). Ladislav Šigut acknowledges support by the Ministry of Education, Youth and Sports of CR within the CzeCOS program (grant number LM2023048) and the AdAgriF project (CZ.02.01.01/00/22_008/0004635). Gustau Camps-Valls, Markus Reichstein, Basil Kraft, and Gregory Duveiller acknowledge funding by the European Research Council (ERC) Synergy Grant “Understanding and modeling the Earth System with Machine Learning (USMILE)” under the European Union's Horizon 2020 research and innovation program (grant agreement no. 855187). Ankur Desai acknowledges the US Department of Energy American Network Management Project award to the ChEAS core site cluster (US-PFa, US-WCr, US-Syv, US-Los), Wisconsin Potato and Vegetable Growers Association, and WI Department of Natural Resources (US-CS*), and NSF grant nos. 1822420, 2313772 (US-PFa). Marilyn Roland and Bert Gielen acknowledge the Research Foundation Flanders (FWO) for the support of ICOS research infrastructure in Flanders, Belgium. Dario Papale thanks the support of the ITINERIS – Italian Integrated Environmental Research Infrastructures System project (IR0000032) funded by NextGenerationEU Mission 4.2.3.1. Timo Vesala acknowledges ICOS-Finland (University of Helsinki) and Flagship funding (grant no. 337549). Torsten Sachs acknowledges that the DE-Zrk site relies on infrastructure of the Terrestrial Environmental Observatories Network (TERENO) supported by a Helmholtz Young Investigators Grant (VH-NG-821). Elise Pendall acknowledges Australian Terrestrial Ecosystem Research Network, as part of the National Cooperative Research Infrastructure System. Sara Knox was also supported by an NSERC Discovery Grant (RGPIN-2019-04199) and an Alliance Grant (ALLRP 555468-20). Hideki Kobayashi acknowledges ArCSII no. JPMXD1420318865 (US-Prr). Stefan Metzger acknowledges the National Ecological Observatory Network, which is a program sponsored by the National Science Foundation and operated under cooperative agreement by Battelle. This material is based in part on work supported by the National Science Foundation through the NEON Program. Bernard Heinesch and Caroline Vincke acknowledge the Service Public de Wallonie (SPW-DGO6) for the support of ICOS research infrastructure in Wallonia, Belgium.
This research has been supported by the National Science Foundation (grant nos. 2149988, 1932900, DEB LTREB 1354370, 2011257, DEB-0425328, DEB-0724514, DEB-0830997, 1936752, 1503912, 1107892, 1822420, and 2313772), the Deutsche Forschungsgemeinschaft (grant no. INST 186/1118-1 FUGG), the Niedersächsische Ministerium für Wissenschaft und Kultur (grant no. ZN 3679), the Horizon 2020 (grant nos. 774124, 951288, 855187, 958927, 776810, 820852, and 776186), the Schweizerischer Nationalfonds zur Förderung der Wissenschaftlichen Forschung (grant nos. 40FA40_154245, 146373, 407340_172433, 200021_197357, 100018L_200918, 20FI21_148992, 20FI20_173691, and 20FI20_198227), the ETH Zürich Foundation (grant no. DONA), the Eidgenössische Technische Hochschule Zürich (grant no. FEVER ETH-27 19-1), the Agencia Estatal de Investigación (grant nos. PID2020-117825GB-C21 and PID2020-117825GB-C22), the Ministerio de Universidades (grant no. FPU19/01647), the Swedish National Space Agency (grant no. 2021-00144), the Australian Research Council (grant no. DE190101182), the Research Council of Finland (grant nos. N-PERM 341349 and 337549), the Ministerstvo Školství, Mládeže a Tělovýchovy (grant no. LM2023048), the Ministry of Education, Culture, Sports, Science and Technology (grant no. JPMXD1420318865), the Helmholtz Association (grant no. VH-NG-821), the Natural Sciences and Engineering Research Council of Canada (grant nos. RGPIN-2019-04199 and ALLRP 555468-20), the European Space Agency (grant nos. Vad3e mecum, 4000134598/21/I-NB, and 4000123553/18/I-NB), the Horizon Europe Framework Programme, Horizon Europe Excellent Science (grant nos. 101059548, 20FI20_173691, 101079192, and 101056921), and the Vetenskapsrådet (grant no. 2021-00644).
This paper was edited by Andrew Feldman and reviewed by two anonymous referees.
Amiro, B.: FLUXNET2015 CA-SF2 Saskatchewan – Western Boreal, Forest Burned in 1989, [data set], https://doi.org/10.18140/flx/1440047, 2016a. a
Amiro, B.: FLUXNET2015 CA-Man Manitoba – Northern Old Black Spruce (Former BOREAS Northern Study Area), [data set], https://doi.org/10.18140/flx/1440035, 2016b. a
Amiro, B.: FLUXNET2015 CA-SF1 Saskatchewan – Western Boreal, Forest Burned in 1977, [data set], https://doi.org/10.18140/flx/1440046, 2016c. a
Amiro, B.: FLUXNET2015 CA-SF3 Saskatchewan – Western Boreal, Forest Burned in 1998, [data set], https://doi.org/10.18140/flx/1440048, 2016d. a
Ammann, C.: FLUXNET2015 CH-Oe1 Oensingen Grassland, [data set], https://doi.org/10.18140/flx/1440135, 2016. a
Arain, M.: AmeriFlux FLUXNET-1F CA-TPD Ontario – Turkey Point Mature Deciduous, [data set], https://doi.org/10.17190/amf/1881567, 2022a. a
Arain, M.: AmeriFlux FLUXNET-1F CA-TP3 Ontario – Turkey Point 1974 Plantation White Pine, [data set], https://doi.org/10.17190/amf/1881566, 2022b. a
Arain, M. A.: FLUXNET2015 CA-TP2 Ontario – Turkey Point 1989 Plantation White Pine, [data set], https://doi.org/10.18140/flx/1440051, 2016a. a
Arain, M. A.: FLUXNET2015 CA-TP1 Ontario – Turkey Point 2002 Plantation White Pine, [data set], https://doi.org/10.18140/flx/1440050, 2016b. a
Arain, M. A.: FLUXNET2015 CA-TP4 Ontario – Turkey Point 1939 Plantation White Pine, [data set], https://doi.org/10.18140/flx/1440053, 2016c. a
Ardö, J., El Tahir, B. A., and ElKhidir, H. A. M.: FLUXNET2015 SD-Dem Demokeya, [data set], https://doi.org/10.18140/flx/1440186, 2016. a
Arndt, S., Hinko-Najera, N., and Griebel, A.: FLUXNET2015 AU-Wom Wombat, [data set], https://doi.org/10.18140/flx/1440207, 2016. a
Aurela, M., Lohila, A., Tuovinen, J.-P., Hatakka, J., Rainne, J., Mäkelä, T., and Lauria, T.: FLUXNET2015 FI-Lom Lompolojankka, [data set], https://doi.org/10.18140/flx/1440228, 2016a. a
Aurela, M., Tuovinen, J.-P., Hatakka, J., Lohila, A., Mäkelä, T., Rainne, J., and Lauria, T.: FLUXNET2015 FI-Sod Sodankyla, [data set], https://doi.org/10.18140/flx/1440160, 2016b. a
Badgley, G., Field, C. B., and Berry, J. A.: Canopy Near-Infrared Reflectance and Terrestrial Photosynthesis, Sci. Adv., 3, e1602244, https://doi.org/10.1126/sciadv.1602244, 2017. a
Baker, J. and Griffis, T.: AmeriFlux FLUXNET-1F US-Ro5 Rosemount I18_South, [data set], https://doi.org/10.17190/amf/1818371, 2021. a
Baker, J. and Griffis, T.: AmeriFlux FLUXNET-1F US-Ro4 Rosemount Prairie, [data set], https://doi.org/10.17190/amf/1881589, 2022a. a
Baker, J. and Griffis, T.: AmeriFlux FLUXNET-1F US-Ro6 Rosemount I18_North, [data set], https://doi.org/10.17190/amf/1881590, 2022b. a
Baker, J., Griffis, T., and Griffis, T.: AmeriFlux FLUXNET-1F US-Ro1 Rosemount-G21, G21 [data set], https://doi.org/10.17190/amf/1881588, 2022. a
Baldocchi, D.: FLUXNET2015 US-Twt Twitchell Island, [data set], https://doi.org/10.18140/flx/1440106, 2016. a
Baldocchi, D. and Ma, S.: FLUXNET2015 US-Ton Tonzi Ranch, [data set], https://doi.org/10.18140/flx/1440092, 2016. a
Baldocchi, D. and Penuelas, J.: The Physics and Ecology of Mining Carbon Dioxide from the Atmosphere by Ecosystems, Glob. Change Biol., 25, 1191–1197, 2019. a
Baldocchi, D., Ma, S., and Xu, L.: FLUXNET2015 US-Var Vaira Ranch-Ione, [data set], https://doi.org/10.18140/flx/1440094, 2016. a
Baldocchi, D. D.: How Eddy Covariance Flux Measurements Have Contributed to Our Understanding of Global Change Biology, Glob. Change Biol., 26, 242–260, 2019. a, b
Bao, S., Wutzler, T., Koirala, S., Cuntz, M., Ibrom, A., Besnard, S., Walther, S., Šigut, L., Moreno, A., Weber, U., Wohlfahrt, G., Cleverly, J., Migliavacca, M., Woodgate, W., Merbold, L., Veenendaal, E., and Carvalhais, N.: Environment-Sensitivity Functions for Gross Primary Productivity in Light Use Efficiency Models, Agr. Forest Meteorol., 312, 108708, https://doi.org/10.1016/j.agrformet.2021.108708, 2022. a
Belelli, L., Papale, D., and Valentini, R.: FLUXNET2015 RU-Ha1 Hakasia Steppe, [data set], https://doi.org/10.18140/flx/1440184, 2016. a
Berbigier, P. and Loustau, D.: FLUXNET2015 FR-LBr Le Bray, [data set], https://doi.org/10.18140/flx/1440163, 2016. a
Berg, A. and Sheffield, J.: Evapotranspiration Partitioning in CMIP5 Models: Uncertainties and Future Projections, J. Clim., 32, 2653–2671, 2019. a
Beringer, J. and Hutley, L.: FLUXNET2015 AU-Fog Fogg Dam, [data set], https://doi.org/10.18140/flx/1440124, 2016a. a
Beringer, J. and Hutley, L.: FLUXNET2015 AU-DaP Daly River Savanna, [data set], https://doi.org/10.18140/flx/1440123, 2016b. a
Beringer, J. and Hutley, L.: FLUXNET2015 AU-Ade Adelaide River, [data set], https://doi.org/10.18140/flx/1440193, 2016c. a
Beringer, J. and Hutley, L.: FLUXNET2015 AU-RDF Red Dirt Melon Farm, Northern Territory, [data set], https://doi.org/10.18140/flx/1440201, 2016d. a
Beringer, J. and Hutley, L.: FLUXNET2015 AU-Dry Dry River, [data set], https://doi.org/10.18140/flx/1440197, 2016e. a
Beringer, J. and Hutley, P. L.: FLUXNET2015 AU-DaS Daly River Cleared, [data set], https://doi.org/10.18140/flx/1440122, 2016f. a
Beringer, J. and Walker, J.: FLUXNET2015 AU-Ync Jaxa, [data set], https://doi.org/10.18140/flx/1440208, 2016. a
Beringer, J., Cunningham, S., Baker, P., Cavagnaro, T., MacNally, R., Thompson, R., and McHugh, I.: FLUXNET2015 AU-Whr Whroo, [data set], https://doi.org/10.18140/flx/1440206, 2016a. a
Beringer, J., Hutley, L., McGuire, D., and U, P.: FLUXNET2015 AU-Wac Wallaby Creek, [data set], https://doi.org/10.18140/flx/1440127, 2016b. a
Bernhofer, C., Grünwald, T., Moderow, U., Hehn, M., Eichelmann, U., and Prasse, H.: FLUXNET2015 DE-Spw Spreewald, [data set], https://doi.org/10.18140/flx/1440220, 2016. a
Billesbach, D., Bradford, J., and Torn, M.: FLUXNET2015 US-AR2 ARM USDA UNL OSU Woodward Switchgrass 2, 2 [data set], https://doi.org/10.18140/flx/1440104, 2016a. a
Billesbach, D., Bradford, J., and Torn, M.: FLUXNET2015 US-AR1 ARM USDA UNL OSU Woodward Switchgrass 1, [data set], https://doi.org/10.18140/flx/1440103, 2016b. a
Billesbach, D., Kueppers, L., Torn, M., and Biraud, S.: AmeriFlux FLUXNET-1F US-A32 ARM-SGP Medford Hay Pasture, [data set], https://doi.org/10.17190/amf/1881568, 2022. a
Biraud, S., Fischer, M., Chan, S., and Torn, M.: AmeriFlux FLUXNET-1F US-ARM ARM Southern Great Plains Site-Lamont, [data set], https://doi.org/10.17190/amf/1854366, 2022. a
Black, T.: AmeriFlux FLUXNET-1F CA-LP1 British Columbia – Mountain Pine Beetle-Attacked Lodgepole Pine Stand, [data set], https://doi.org/10.17190/amf/1832155, 2021. a
Black, T. A.: FLUXNET2015 CA-Obs Saskatchewan – Western Boreal, Mature Black Spruce, [data set], https://doi.org/10.18140/flx/1440044, 2016a. a
Black, T. A.: FLUXNET2015 CA-Oas Saskatchewan – Western Boreal, Mature Aspen, [data set], https://doi.org/10.18140/flx/1440043, 2016b. a
Blanken, P., Monson, R., Burns, S., Bowling, D., and Turnipseed, A.: AmeriFlux FLUXNET-1F US-NR1 Niwot Ridge Forest (LTER NWT1), [data set], https://doi.org/10.17190/amf/1832155, 2022. a
Bodesheim, P., Jung, M., Gans, F., Mahecha, M. D., and Reichstein, M.: Upscaled diurnal cycles of land–atmosphere fluxes: a new global half-hourly data product, Earth Syst. Sci. Data, 10, 1327–1365, https://doi.org/10.5194/essd-10-1327-2018, 2018. a, b, c
Bohrer, G.: AmeriFlux FLUXNET-1F US-ORv Olentangy River Wetland Research Park, [data set], https://doi.org/10.17190/amf/1832164, 2021. a
Bohrer, G.: AmeriFlux FLUXNET-1F US-UM3 Douglas Lake, [data set], https://doi.org/10.17190/amf/1881596, 2022. a
Bohrer, G. and Kerns, J.: AmeriFlux FLUXNET-1F US-OWC Old Woman Creek, [data set], https://doi.org/10.17190/amf/1871142, 2022. a
Boike, J., Westermann, S., Lüers, J., Langer, M., and Piel, K.: FLUXNET2015 SJ-Blv Bayelva, Spitsbergen, [data set], https://doi.org/10.18140/flx/1440242, 2016. a
Bonan, G. B., Lawrence, P. J., Oleson, K. W., Levis, S., Jung, M., Reichstein, M., Lawrence, D. M., and Swenson, S. C.: Improving Canopy Processes in the Community Land Model Version 4 (CLM4) Using Global Flux Fields Empirically Inferred from FLUXNET Data, J. Geophys. Res., 116, G02014, https://doi.org/10.1029/2010JG001593, 2011. a
Bowling, D.: FLUXNET2015 US-Cop Corral Pocket, [data set], https://doi.org/10.18140/flx/1440100, 2016. a
Brunsell, N.: AmeriFlux FLUXNET-1F US-KFS Kansas Field Station, [data set], https://doi.org/10.17190/amf/1881585, 2022a. a
Brunsell, N.: AmeriFlux FLUXNET-1F US-KLS Kansas Land Institute, [data set], https://doi.org/10.17190/amf/1854367, 2022b. a
Burton, C. A., Renzullo, L. J., Rifai, S. W., and Van Dijk, A. I. J. M.: Empirical Upscaling of OzFlux Eddy Covariance for High-Resolution Monitoring of Terrestrial Carbon Uptake in Australia, 2023. a
Byrne, B., Baker, D. F., Basu, S., Bertolacci, M., Bowman, K. W., Carroll, D., Chatterjee, A., Chevallier, F., Ciais, P., Cressie, N., Crisp, D., Crowell, S., Deng, F., Deng, Z., Deutscher, N. M., Dubey, M. K., Feng, S., García, O. E., Griffith, D. W. T., Herkommer, B., Hu, L., Jacobson, A. R., Janardanan, R., Jeong, S., Johnson, M. S., Jones, D. B. A., Kivi, R., Liu, J., Liu, Z., Maksyutov, S., Miller, J. B., Miller, S. M., Morino, I., Notholt, J., Oda, T., O'Dell, C. W., Oh, Y.-S., Ohyama, H., Patra, P. K., Peiro, H., Petri, C., Philip, S., Pollard, D. F., Poulter, B., Remaud, M., Schuh, A., Sha, M. K., Shiomi, K., Strong, K., Sweeney, C., Té, Y., Tian, H., Velazco, V. A., Vrekoussis, M., Warneke, T., Worden, J. R., Wunch, D., Yao, Y., Yun, J., Zammit-Mangion, A., and Zeng, N.: National CO2 budgets (2015–2020) inferred from atmospheric CO2 observations in support of the global stocktake, Earth Syst. Sci. Data, 15, 963–1004, https://doi.org/10.5194/essd-15-963-2023, 2023. a
Camps‐Valls, G., Tuia, D., Zhu, X. X., and Reichstein, M.: Deep Learning for the Earth Sciences: A Comprehensive Approach to Remote Sensing, Climate Science, and Geosciences, Wiley, 1 Edn., 2021. a
Cescatti, A., Marcolla, B., Zorer, R., and Gianelle, D.: FLUXNET2015 IT-La2 Lavarone2, [data set], https://doi.org/10.18140/flx/1440235, 2016. a
Chamberlain, S., Oikawa, P., Sturtevant, C., Szutu, D., Verfaillie, J., and Baldocchi, D.: AmeriFlux FLUXNET-1F US-Tw3 Twitchell Alfalfa, [data set], https://doi.org/10.17190/amf/1881594, 2022. a
Chen, J.: FLUXNET2015 US-Wi5 Mixed Young Jack Pine (MYJP), [data set], https://doi.org/10.18140/flx/1440059, 2016a. a
Chen, J.: FLUXNET2015 US-Wi3 Mature Hardwood (MHW), [data set], https://doi.org/10.18140/flx/1440057, 2016b. a
Chen, J.: FLUXNET2015 US-Wi8 Young Hardwood Clearcut (YHW), [data set], https://doi.org/10.18140/flx/1440062, 2016c. a
Chen, J.: FLUXNET2015 US-Wi4 Mature Red Pine (MRP), [data set], https://doi.org/10.18140/flx/1440058, 2016d. a
Chen, J.: FLUXNET2015 US-Wi1 Intermediate Hardwood (IHW), [data set], https://doi.org/10.18140/flx/1440055, 2016e. a
Chen, J.: FLUXNET2015 US-Wi9 Young Jack Pine (YJP), [data set], https://doi.org/10.18140/flx/1440063, 2016f. a
Chen, J.: FLUXNET2015 US-Wi0 Young Red Pine (YRP), [data set], https://doi.org/10.18140/flx/1440055, 2016g. a
Chen, J.: FLUXNET2015 US-Wi6 Pine Barrens #1 (PB1), [data set], https://doi.org/10.18140/flx/1440060, 2016h. a
Chen, J.: FLUXNET2015 US-Wi7 Red Pine Clearcut (RPCC), [data set], https://doi.org/10.18140/flx/1440061, 2016i. a
Chen, J.: FLUXNET2015 US-Wi2 Intermediate Red Pine (IRP), [data set], https://doi.org/10.18140/flx/1440056 2016j. a
Chen, J. and Chu, H.: FLUXNET2015 US-WPT Winous Point North Marsh, [data set], https://doi.org/10.18140/flx/1440117, 2016a. a
Chen, J. and Chu, H.: FLUXNET2015 US-CRT Curtice Walter-Berger Cropland, [data set], https://doi.org/10.18140/flx/1440117, 2016b. a
Chen, J., Chu, H., and Noormets, A.: FLUXNET2015 US-Oho Oak Openings, [data set], https://doi.org/10.18140/flx/1440088, 2016. a
Chen, S.: FLUXNET2015 CN-Du2 Duolun_grassland (D01), [data set], https://doi.org/10.18140/flx/1440140, 2016k. a
Chen, T. and Guestrin, C.: XGBoost, in: Proceedings of the 22nd ACM SIGKDD International Conference on Knowledge Discovery and Data Mining, ACM, 2016. a
Christen, A. and Knox, S.: AmeriFlux FLUXNET-1F CA-DBB Delta Burns Bog, [data set], https://doi.org/10.17190/amf/1881565, 2022. a
Christensen, T.: FLUXNET2015 SJ-Adv Adventdalen, [data set], https://doi.org/10.18140/flx/1440241, 2016. a
Cleverly, J. and Eamus, D.: FLUXNET2015 AU-TTE Ti Tree East, [data set], https://doi.org/10.18140/flx/1440205, 2016a. a
Cleverly, J. and Eamus, D.: FLUXNET2015 AU-ASM Alice Springs, [data set], https://doi.org/10.18140/flx/1440194, 2016b. a
Coenders-Gerrits, A. M. J., van der Ent, R. J., Bogaard, T. A., Wang-Erlandsson, L., Hrachowitz, M., and Savenije, H. H. G.: Uncertainties in Transpiration Estimates, Nature, 506, E1–E2, 2014. a
Dannenberg, M. P., Barnes, M. L., Smith, W. K., Johnston, M. R., Meerdink, S. K., Wang, X., Scott, R. L., and Biederman, J. A.: Upscaling dryland carbon and water fluxes with artificial neural networks of optical, thermal, and microwave satellite remote sensing, Biogeosciences, 20, 383–404, https://doi.org/10.5194/bg-20-383-2023, 2023. a, b
Desai, A.: FLUXNET2015 US-WCr Willow Creek, [data set], https://doi.org/10.18140/flx/1440091, 2016a. a
Desai, A.: FLUXNET2015 US-Syv Sylvania Wilderness Area, [data set], https://doi.org/10.18140/flx/1440091, 2016b. a
Desai, A.: FLUXNET2015 US-Los Lost Creek, [data set], https://doi.org/10.18140/flx/1440076, 2016c. a
Desai, A.: FLUXNET2015 US-PFa Park Falls/WLEF, [data set], https://doi.org/10.18140/flx/1440089, 2016d. a
Desai, A.: AmeriFlux FLUXNET-1F US-CS1 Central Sands Irrigated Agricultural Field, 2022a. a
Desai, A.: AmeriFlux FLUXNET-1F US-CS4 Central Sands Irrigated Agricultural Field, [data set], https://doi.org/10.17190/amf/1881576, 2022b. a
Desai, A.: AmeriFlux FLUXNET-1F US-CS2 Tri County School Pine Forest, [data set], https://doi.org/10.17190/amf/1881577 2022c. a
Desai, A.: AmeriFlux FLUXNET-1F US-CS3 Central Sands Irrigated Agricultural Field, [data set], https://doi.org/10.17190/amf/1881578, 2022d. a
Dolman, H., Hendriks, D., Parmentier, F.-J., Marchesini, L. B., Dean, J., and Van Huissteden, K.: FLUXNET2015 NL-Hor Horstermeer, [data set], https://doi.org/10.18140/flx/1440091, 2016a. a
Dolman, H., Van Der Molen, M., Parmentier, F.-J., Marchesini, L. B., Dean, J., Van Huissteden, K., and Maximov, T.: FLUXNET2015 RU-Cok Chokurdakh, [data set], https://doi.org/10.18140/flx/1440182, 2016b. a
Dong, G.: FLUXNET2015 CN-Cng Changling, [data set], https://doi.org/10.18140/flx/1440209, 2016. a
Drake, B. and Hinkle, R.: FLUXNET2015 US-KS1 Kennedy Space Center (Slash Pine), [data set], https://doi.org/10.18140/flx/1440209, 2016a. a
Drake, B. and Hinkle, R.: FLUXNET2015 US-KS2 Kennedy Space Center (Scrub Oak), [data set], https://doi.org/10.18140/flx/1440075, 2016b. a
Euskirchen, E.: AmeriFlux FLUXNET-1F US-BZo Bonanza Creek Old Thermokarst Bog, [data set], https://doi.org/10.17190/amf/1881571, 2022a. a
Euskirchen, E.: AmeriFlux FLUXNET-1F US-BZB Bonanza Creek Thermokarst Bog, [data set], https://doi.org/10.17190/amf/1881569, 2022b. a
Euskirchen, E.: AmeriFlux FLUXNET-1F US-BZF Bonanza Creek Rich Fen, [data set], https://doi.org/10.17190/amf/1881570, 2022c. a
Euskirchen, E.: AmeriFlux FLUXNET-1F US-BZS Bonanza Creek Black Spruce, [data set], https://doi.org/10.17190/amf/1881572, 2022d. a
Euskirchen, E., Shaver, G., and Bret-Harte, S.: AmeriFlux FLUXNET-1F US-ICs Imnavait Creek Watershed Wet Sedge Tundra, [data set], https://doi.org/10.17190/amf/1871138, 2022a. a
Euskirchen, E., Shaver, G., and Bret-Harte, S.: AmeriFlux FLUXNET-1F US-ICt Imnavait Creek Watershed Tussock Tundra, [data set], https://doi.org/10.17190/amf/1871138, 2022b. a
Ewers, B. and Pendall, E.: FLUXNET2015 US-Sta Saratoga, [data set], https://doi.org/10.18140/flx/1440115, 2016. a
Fares, S.: FLUXNET2015 US-Lin Lindcove Orange Orchard, [data set], https://doi.org/10.18140/flx/1440107, 2016. a
Flerchinger, G.: AmeriFlux FLUXNET-1F US-Rwf RCEW Upper Sheep Prescibed Fire, [data set], https://doi.org/10.17190/amf/1881591, 2022a. a
Flerchinger, G.: AmeriFlux FLUXNET-1F US-Rws Reynolds Creek Wyoming Big Sagebrush, [data set], https://doi.org/10.17190/amf/1881592, 2022b. a
Flerchinger, G.: AmeriFlux FLUXNET-1F US-Rms RCEW Mountain Big Sagebrush, [data set], https://doi.org/10.17190/amf/1881587, 2022c. a
Flerchinger, G. and Reba, M.: AmeriFlux FLUXNET-1F US-Rwe RCEW Reynolds Mountain East, [data set], https://doi.org/10.17190/amf/1871143, 2022. a
Forsythe, J., Kline, M., and O’Halloran, T.: AmeriFlux FLUXNET-1F US-HB1 North Inlet Crab Haul Creek, [data set], https://doi.org/10.17190/amf/1832160, 2021. a
Franssen, H. H., Stöckli, R., Lehner, I., Rotenberg, E., and Seneviratne, S.: Energy balance closure of eddy-covariance data: A multisite analysis for European FLUXNET stations, Agr. Forest Meteorol., 150, 1553–1567, https://doi.org/10.1016/j.agrformet.2010.08.005, 2010. a
Fratini, G., McDermitt, D. K., and Papale, D.: Eddy-covariance flux errors due to biases in gas concentration measurements: origins, quantification and correction, Biogeosciences, 11, 1037–1051, https://doi.org/10.5194/bg-11-1037-2014, 2014. a
Friedl, M. and Sulla-Menashe, D.: MCD12C1 MODIS/Terra+Aqua Land Cover Type Yearly L3 Global 0.05Deg CMG V006, https://doi.org/10.5067/MODIS/MCD12C1.006, 2015. a
Fu, Z., Gerken, T., Bromley, G., Araújo, A., Bonal, D., Burban, B., Ficklin, D., Fuentes, J. D., Goulden, M., Hirano, T., Kosugi, Y., Liddell, M., Nicolini, G., Niu, S., Roupsard, O., Stefani, P., Mi, C., Tofte, Z., Xiao, J., Valentini, R., Wolf, S., and Stoy, P. C.: The Surface-Atmosphere Exchange of Carbon Dioxide in Tropical Rainforests: Sensitivity to Environmental Drivers and Flux Measurement Methodology, Agr. Forest Meteorol., 263, 292–307, 2018. a
Gao, B.-C.: NDWI—A Normalized Difference Water Index for Remote Sensing of Vegetation Liquid Water from Space, Remote Sens. Environ., 58, 257–266, 1996. a
Garcia, A., Di Bella, C., Houspanossian, J., Magliano, P., Jobbágy, E., Posse, G., Fernández, R., and Nosetto, M.: FLUXNET2015 AR-SLu San Luis, [data set],https://doi.org/10.18140/flx/1440191, 2016. a
Gash, J. H. C.: An Analytical Model of Rainfall Interception by Forests, Q. J. Roy. Meteorol. Soc., 105, 43–55, 1979. a
Goldstein, A.: FLUXNET2015 US-Blo Blodgett Forest, [data set], https://doi.org/10.18140/flx/1440068, 2016. a
Good, S. P., Noone, D., and Bowen, G.: Hydrologic Connectivity Constrains Partitioning of Global Terrestrial Water Fluxes, Science, 349, 175–177, 2015. a
Goslee, S.: AmeriFlux FLUXNET-1F US-HWB USDA ARS Pasture Sytems and Watershed Management Research Unit- Hawbecker Site, [data set], https://doi.org/10.17190/amf/1881582, 2022. a
Gough, C., Bohrer, G., and Curtis, P.: FLUXNET2015 US-UMB Univ. Of Mich. Biological Station, [data set], https://doi.org/10.18140/flx/1440093, 2016. a
Gough, C., Bohrer, G., and Curtis, P.: AmeriFlux FLUXNET-1F US-UMd UMBS Disturbance, [data set], https://doi.org/10.17190/amf/1881597, 2022. a
Goulden, M.: FLUXNET2015 CA-NS2 UCI-1930 Burn Site, [data set], https://doi.org/10.18140/flx/1440037, 2016a. a
Goulden, M.: FLUXNET2015 CA-NS3 UCI-1964 Burn Site, [data set], https://doi.org/10.18140/flx/1440037, 2016b. a
Goulden, M.: FLUXNET2015 CA-NS4 UCI-1964 Burn Site Wet, [data set], https://doi.org/10.18140/flx/1440039, 2016c. a
Goulden, M.: FLUXNET2015 BR-Sa3 Santarem-Km83-Logged Forest, [data set], https://doi.org/10.18140/flx/1440033, 2016d. a
Goulden, M.: FLUXNET2015 CA-NS6 UCI-1989 Burn Site, [data set], https://doi.org/10.18140/flx/1440041, 2016e. a
Goulden, M.: FLUXNET2015 CA-NS7 UCI-1998 Burn Site, [data set], https://doi.org/10.18140/flx/1440042, 2016f. a
Goulden, M.: FLUXNET2015 CA-NS5 UCI-1981 Burn Site, [data set], https://doi.org/10.18140/flx/1440040, 2016g. a
Griffis, T. and Roman, T.: AmeriFlux FLUXNET-1F PE-QFR Quistococha Forest Reserve, [data set], https://doi.org/10.17190/amf/1832157, 2021. a
Gruening, C., Goded, I., Cescatti, A., Manca, G., and Seufert, G.: FLUXNET2015 IT-SRo San Rossore, [data set], https://doi.org/10.17190/amf/1832157, 2016a. a
Gruening, C., Goded, I., Cescatti, A., and Pokorska, O.: FLUXNET2015 IT-Isp Ispra ABC-IS, [data set], https://doi.org/10.18140/flx/1440234, 2016b. a
Hansen, B. U.: FLUXNET2015 GL-NuF Nuuk Fen, [data set], https://doi.org/10.18140/flx/1440222, 2016. a
Heinsch, F., Zhao, M., Running, S., Kimball, J., Nemani, R., Davis, K., Bolstad, P., Cook, B., Desai, A., Ricciuto, D., Law, B., Oechel, W., Kwon, H., Luo, H., Wofsy, S., Dunn, A., Munger, J., Baldocchi, D., Xu, L., Hollinger, D., Richardson, A., Stoy, P., Siqueira, M., Monson, R., Burns, S., and Flanagan, L.: Evaluation of Remote Sensing Based Terrestrial Productivity from MODIS Using Regional Tower Eddy Flux Network Observations, IEEE Trans. Geosci. Remote Sens., 44, 1908–1925, 2006. a
Hersbach, H., Bell, B., Berrisford, P., Hirahara, S., Horányi, A., Muñoz‐Sabater, J., Nicolas, J., Peubey, C., Radu, R., Schepers, D., Simmons, A., Soci, C., Abdalla, S., Abellan, X., Balsamo, G., Bechtold, P., Biavati, G., Bidlot, J., Bonavita, M., Chiara, G., Dahlgren, P., Dee, D., Diamantakis, M., Dragani, R., Flemming, J., Forbes, R., Fuentes, M., Geer, A., Haimberger, L., Healy, S., Hogan, R. J., Hólm, E., Janisková, M., Keeley, S., Laloyaux, P., Lopez, P., Lupu, C., Radnoti, G., Rosnay, P., Rozum, I., Vamborg, F., Villaume, S., and Thépaut, J.: The ERA5 Global Reanalysis, Q. J. Roy. Meteorol. Soc., 146, 1999–2049, 2020. a, b
Hinkle, R.: AmeriFlux FLUXNET-1F US-KS3 Kennedy Space Center (Salt Marsh), [data set], https://doi.org/10.17190/amf/1881586, 2022. a
Hollinger, D.: AmeriFlux FLUXNET-1F US-Ho2 Howland Forest (West Tower), [data set], https://doi.org/10.17190/amf/1881581, 2022. a
Huang, X., Xiao, J., Wang, X., and Ma, M.: Improving the Global MODIS GPP Model by Optimizing Parameters with FLUXNET Data, Agr. Forest Meteorol., 300, 108314, https://doi.org/10.1016/j.agrformet.2020.108314, 2021. a
Huete, A., Didan, K., Miura, T., Rodriguez, E., Gao, X., and Ferreira, L.: Overview of the Radiometric and Biophysical Performance of the MODIS Vegetation Indices, Remote Sens. Environ., 83, 195–213, 2002. a
Huggins, D.: AmeriFlux FLUXNET-1F US-CF1 CAF-LTAR Cook East, [data set], https://doi.org/10.17190/amf/1832158, 2021. a
Huggins, D.: AmeriFlux FLUXNET-1F US-CF3 CAF-LTAR Boyd North, [data set], https://doi.org/10.17190/amf/1881573, 2022a. a
Huggins, D.: AmeriFlux FLUXNET-1F US-CF4 CAF-LTAR Boyd South, [data set], https://doi.org/10.17190/amf/1881575, 2022b. a
Huggins, D.: AmeriFlux FLUXNET-1F US-CF2 CAF-LTAR Cook West, [data set], https://doi.org/10.17190/amf/1881573, 2022c. a
Ichii, K., Ueyama, M., Kondo, M., Saigusa, N., Kim, J., Alberto, M. C., Ardö, J., Euskirchen, E. S., Kang, M., Hirano, T., Joiner, J., Kobayashi, H., Marchesini, L. B., Merbold, L., Miyata, A., Saitoh, T. M., Takagi, K., Varlagin, A., Bret‐Harte, M. S., Kitamura, K., Kosugi, Y., Kotani, A., Kumar, K., Li, S., Machimura, T., Matsuura, Y., Mizoguchi, Y., Ohta, T., Mukherjee, S., Yanagi, Y., Yasuda, Y., Zhang, Y., and Zhao, F.: New Data‐driven Estimation of Terrestrial CO2 fluxes in Asia Using a Standardized Database of Eddy Covariance Measurements, Remote Sensing Data, and Support Vector Regression, J. Geophys. Res.-Biogeo., 122, 767–795, 2017. a
Joiner, J. and Yoshida, Y.: Satellite-Based Reflectances Capture Large Fraction of Variability in Global Gross Primary Production (GPP) at Weekly Time Scales, Agr. Forest Meteorol., 291, 108092, https://doi.org/10.1016/j.agrformet.2020.108092, 2020. a
Jung, M., Reichstein, M., and Bondeau, A.: Towards global empirical upscaling of FLUXNET eddy covariance observations: validation of a model tree ensemble approach using a biosphere model, Biogeosciences, 6, 2001–2013, https://doi.org/10.5194/bg-6-2001-2009, 2009. a
Jung, M., Reichstein, M., Margolis, H. A., Cescatti, A., Richardson, A. D., Arain, M. A., Arneth, A., Bernhofer, C., Bonal, D., Chen, J., Gianelle, D., Gobron, N., Kiely, G., Kutsch, W., Lasslop, G., Law, B. E., Lindroth, A., Merbold, L., Montagnani, L., Moors, E. J., Papale, D., Sottocornola, M., Vaccari, F., and Williams, C.: Global Patterns of Land-Atmosphere Fluxes of Carbon Dioxide, Latent Heat, and Sensible Heat Derived from Eddy Covariance, Satellite, and Meteorological Observations, J. Geophys. Res., 116, G00J07, https://doi.org/10.1029/2010JG001566, 2011. a, b, c, d
Jung, M., Reichstein, M., Schwalm, C. R., Huntingford, C., Sitch, S., Ahlström, A., Arneth, A., Camps-Valls, G., Ciais, P., Friedlingstein, P., Gans, F., Ichii, K., Jain, A. K., Kato, E., Papale, D., Poulter, B., Raduly, B., Rödenbeck, C., Tramontana, G., Viovy, N., Wang, Y.-P., Weber, U., Zaehle, S., and Zeng, N.: Compensatory Water Effects Link Yearly Global Land CO2 Sink Changes to Temperature, Nature, 541, 516–520, 2017. a, b
Jung, M., Koirala, S., Weber, U., Ichii, K., Gans, F., Camps-Valls, G., Papale, D., Schwalm, C., Tramontana, G., and Reichstein, M.: The FLUXCOM Ensemble of Global Land-Atmosphere Energy Fluxes, Sci. Data, 6, 74, https://doi.org/10.1038/s41597-019-0076-8, 2019. a, b, c, d
Jung, M., Schwalm, C., Migliavacca, M., Walther, S., Camps-Valls, G., Koirala, S., Anthoni, P., Besnard, S., Bodesheim, P., Carvalhais, N., Chevallier, F., Gans, F., Goll, D. S., Haverd, V., Köhler, P., Ichii, K., Jain, A. K., Liu, J., Lombardozzi, D., Nabel, J. E. M. S., Nelson, J. A., O'Sullivan, M., Pallandt, M., Papale, D., Peters, W., Pongratz, J., Rödenbeck, C., Sitch, S., Tramontana, G., Walker, A., Weber, U., and Reichstein, M.: Scaling carbon fluxes from eddy covariance sites to globe: synthesis and evaluation of the FLUXCOM approach, Biogeosciences, 17, 1343–1365, https://doi.org/10.5194/bg-17-1343-2020, 2020. a, b, c, d, e, f, g, h, i, j, k
Jung, M., Nelson, J., Migliavacca, M., El-Madany, T., Papale, D., Reichstein, M., Walther, S., and Wutzler, T.: Technical note: Flagging inconsistencies in flux tower data, Biogeosciences, 21, 1827–1846, https://doi.org/10.5194/bg-21-1827-2024, 2024. a, b
Klatt, J., Schmid, H. P., Mauder, M., and Steinbrecher, R.: FLUXNET2015 DE-SfN Schechenfilz Nord, [data set], https://doi.org/10.18140/flx/1440219, 2016. a
Knohl, A., Tiedemann, F., Kolle, O., Schulze, E.-D., Anthoni, P., Kutsch, W., Herbst, M., and Siebicke, L.: FLUXNET2015 DE-Lnf Leinefelde, [data set], https://doi.org/10.18140/flx/1440150, 2016. a
Knox, S.: AmeriFlux FLUXNET-1F CA-DB2 Delta Burns Bog 2, [data set], https://doi.org/10.17190/amf/1881564, 2022. a
Kobayashi, H. and Suzuki, R.: FLUXNET2015 US-Prr Poker Flat Research Range Black Spruce Forest, [data set], https://doi.org/10.18140/flx/1440113, 2016. a
Kosugi, Y. and Takanashi, S.: FLUXNET2015 MY-PSO Pasoh Forest Reserve (PSO), [data set], https://doi.org/10.18140/flx/1440240, 2016. a
Kotani, A.: FLUXNET2015 JP-SMF Seto Mixed Forest Site, [data set], https://doi.org/10.18140/flx/1440239, 2016a. a
Kotani, A.: FLUXNET2015 JP-MBF Moshiri Birch Forest Site, [data set], https://doi.org/10.18140/flx/1440238, 2016b. a
Kumar, J., Hoffman, F. M., Hargrove, W. W., and Collier, N.: Understanding the Representativeness of FLUXNET for Upscaling carbon Flux from Eddy Covariance Measurements, 2016. a
Kurc, S.: AmeriFlux FLUXNET-1F US-SRC Santa Rita Creosote, [data set], https://doi.org/10.17190/amf/1871145, 2022. a
Kusak, K., Sanchez, C., Szutu, D., and Baldocchi, D.: AmeriFlux FLUXNET-1F US-Snf Sherman Barn, [data set], https://doi.org/10.17190/amf/1854371, 2022. a
Kutzbach, L.: AmeriFlux FLUXNET-1F AR-TF1 Rio Moat Bog, [data set], https://doi.org/10.17190/amf/1818370, 2021. a
Köhler, P., Frankenberg, C., Magney, T. S., Guanter, L., Joiner, J., and Landgraf, J.: Global Retrievals of Solar‐Induced Chlorophyll Fluorescence With TROPOMI: First Results and Intersensor Comparison to OCO‐2, Geophys. Res. Lett., 45, e2020GL087541, https://doi.org/10.1029/2020GL087541, 2018. a, b
Law, B.: FLUXNET2015 US-Me3 Metolius-second Young Aged Pine, [data set], https://doi.org/10.18140/flx/1440080, 2016a. a
Law, B.: FLUXNET2015 US-Me6 Metolius Young Pine Burn, [data set], https://doi.org/10.18140/flx/1440099, 2016b. a
Law, B.: FLUXNET2015 US-Me1 Metolius – Eyerly Burn, [data set], https://doi.org/10.18140/flx/1440078, 2016c. a
Law, B.: FLUXNET2015 US-Me5 Metolius-first Young Aged Pine, [data set], https://doi.org/10.18140/flx/1440082, 2016d. a
Law, B.: FLUXNET2015 US-Me4 Metolius-old Aged Ponderosa Pine, [data set], https://doi.org/10.18140/flx/1440081, 2016e. a
Law, B.: AmeriFlux FLUXNET-1F US-Me2 Metolius Mature Ponderosa Pine, [data set], https://doi.org/10.17190/amf/1854368, 2022. a
Leuning, R., van Gorsel, E., Massman, W. J., and Isaac, P. R.: Reflections on the surface energy imbalance problem, Agr. Forest Meteorol., 156, 65–74, https://doi.org/10.1016/j.agrformet.2011.12.002, 2012. a
Liddell, M. J.: FLUXNET2015 AU-Rob Robson Creek, Queensland, Australia, [data set], https://doi.org/10.18140/flx/1440203, 2016. a
Lindauer, M., Steinbrecher, R., Wolpert, B., Mauder, M., and Schmid, H. P.: FLUXNET2015 DE-Lkb Lackenberg, [data set], https://doi.org/10.18140/flx/1440214 2016. a
Litvak, M.: AmeriFlux FLUXNET-1F US-Mpj Mountainair Pinyon-Juniper Woodland, [data set], https://doi.org/10.17190/amf/1832161, 2021. a
Litvak, M.: AmeriFlux FLUXNET-1F US-Wjs Willard Juniper Savannah, [data set], https://doi.org/10.17190/amf/1832161, 2022. a
Liu, H., Huang, M., and Chen, X.: AmeriFlux FLUXNET-1F US-Hn3 Hanford 100H Sagebrush, [data set], https://doi.org/10.17190/amf/1881580, 2022. a
Lohila, A., Aurela, M., Tuovinen, J.-P., Hatakka, J., and Laurila, T.: FLUXNET2015 FI-Jok Jokioinen, [data set], https://doi.org/10.18140/flx/1440159, 2016. a
Lund, M., Jackowicz-Korczyński, M., and Abermann, J.: FLUXNET2015 GL-ZaH Zackenberg Heath, [data set], https://doi.org/10.18140/flx/1440224, 2016a. a
Lund, M., Jackowicz-Korczyński, M., and Abermann, J.: FLUXNET2015 GL-ZaF Zackenberg Fen, [data set], https://doi.org/10.18140/flx/1440223, 2016b. a
Macfarlane, C., Lambert, P., Byrne, J., Johnstone, C., and Smart, N.: FLUXNET2015 AU-Gin Gingin, [data set], https://doi.org/10.18140/flx/1440199, 2016. a
Manca, G. and Goded, I.: FLUXNET2015 IT-PT1 Parco Ticino Forest, [data set], https://doi.org/10.18140/flx/1440172, 2016. a
Margolis, H. A.: FLUXNET2015 CA-Qfo Quebec – Eastern Boreal, Mature Black Spruce, [data set], https://doi.org/10.18140/flx/14400452016. a
Martens, B., Miralles, D. G., Lievens, H., van der Schalie, R., de Jeu, R. A. M., Fernández-Prieto, D., Beck, H. E., Dorigo, W. A., and Verhoest, N. E. C.: GLEAM v3: satellite-based land evaporation and root-zone soil moisture, Geosci. Model Dev., 10, 1903–1925, https://doi.org/10.5194/gmd-10-1903-2017, 2017. a
Massman, B.: FLUXNET2015 US-GBT GLEES Brooklyn Tower, [data set], https://doi.org/10.18140/flx/1440118, 2016. a
Massman, B.: AmeriFlux FLUXNET-1F US-GLE GLEES, [data set], https://doi.org/10.17190/amf/1871136, 2022. a
Matamala, R.: FLUXNET2015 US-IB2 Fermi National Accelerator Laboratory- Batavia (Prairie Site), [data set], https://doi.org/10.18140/flx/1440072, 2016. a
Matteucci, G.: FLUXNET2015 IT-Col Collelongo, [data set], https://doi.org/10.18140/flx/1440167, 2016. a
Mauder, M., Foken, T., and Cuxart, J.: Surface-Energy-Balance Closure over Land: A Review, Bound.-Lay. Meteorol., 177, 395–426, https://doi.org/10.1007/s10546-020-00529-6, 2020. a
McCaughey, H.: FLUXNET2015 CA-Gro Ontario – Groundhog River, Boreal Mixedwood Forest, [data set], https://doi.org/10.18140/flx/1440034, 2016. a
McGloin, R., Šigut, L., Havránková, K., Dušek, J., Pavelka, M., and Sedlák, P.: Energy balance closure at a variety of ecosystems in Central Europe with contrasting topographies, Agr. Forest Meteorol., 248, 418–431, https://doi.org/10.1016/j.agrformet.2017.10.003, 2018. a
Merbold, L., Rebmann, C., and Corradi, C.: FLUXNET2015 RU-Che Cherski, [data set], https://doi.org/10.18140/flx/1440181, 2016. a
Meyer, W., Cale, P., Koerber, G., Ewenz, C., and Sun, Q.: FLUXNET2015 AU-Cpr Calperum, [data set], https://doi.org/10.18140/flx/1440195, 2016. a
Meyers, T.: FLUXNET2015 US-LWW Little Washita Watershed, [data set], https://doi.org/10.18140/flx/1440070, 2016a. a
Meyers, T.: FLUXNET2015 US-Goo Goodwin Creek, [data set], https://doi.org/10.18140/flx/1440070, 2016b. a
Miralles, D. G., Holmes, T. R. H., De Jeu, R. A. M., Gash, J. H., Meesters, A. G. C. A., and Dolman, A. J.: Global land-surface evaporation estimated from satellite-based observations, Hydrol. Earth Syst. Sci., 15, 453–469, https://doi.org/10.5194/hess-15-453-2011, 2011. a
Miralles, D. G., Jiménez, C., Jung, M., Michel, D., Ershadi, A., McCabe, M. F., Hirschi, M., Martens, B., Dolman, A. J., Fisher, J. B., Mu, Q., Seneviratne, S. I., Wood, E. F., and Fernández-Prieto, D.: The WACMOS-ET project – Part 2: Evaluation of global terrestrial evaporation data sets, Hydrol. Earth Syst. Sci., 20, 823–842, https://doi.org/10.5194/hess-20-823-2016, 2016. a
Moncrieff, J., Malhi, Y., and Leuning, R.: The Propagation of Errors in Long-Term Measurements of Land-Atmosphere Fluxes of Carbon and Water, Glob. Change Biol., 2, 231–240, 1996. a
Munassar, S., Rödenbeck, C., Koch, F.-T., Totsche, K. U., Gałkowski, M., Walther, S., and Gerbig, C.: Net ecosystem exchange (NEE) estimates 2006–2019 over Europe from a pre-operational ensemble-inversion system, Atmos. Chem. Phys., 22, 7875–7892, https://doi.org/10.5194/acp-22-7875-2022, 2022. a
Munger, J. W.: FLUXNET2015 US-Ha1 Harvard Forest EMS Tower (HFR1), [data set], https://doi.org/10.18140/flx/1440071, 2016. a
Musavi, T., Migliavacca, M., Reichstein, M., Kattge, J., Wirth, C., Black, T. A., Janssens, I., Knohl, A., Loustau, D., Roupsard, O., Varlagin, A., Rambal, S., Cescatti, A., Gianelle, D., Kondo, H., Tamrakar, R., and Mahecha, M. D.: Stand Age and Species Richness Dampen Interannual Variation of Ecosystem-Level Photosynthetic Capacity, Nat. Ecol. Evol., 1, 0048, https://doi.org/10.1038/s41559-016-0048, 2017. a
Nash, J. and Sutcliffe, J.: River Flow Forecasting Through Conceptual Models Part I – A Discussion of Principles, J. Hydrol., 10, 282–290, 1970. a
Nelson, J. A.: Jnelson18/TranspirationEstimationAlgorithm: Small Bug Fixes with Compatability, Zenodo, https://doi.org/10.5281/zenodo.5638851, 2021. a
Nelson, J. A.: FLUXCOM-X-BASE Data Access, Gitlab [data set], https://gitlab.gwdg.de/fluxcom/fluxcomxdata (last access: 3 October 2024), 2023. a
Nelson, J. A., Carvalhais, N., Cuntz, M., Delpierre, N., Knauer, J., Ogée, J., Migliavacca, M., Reichstein, M., and Jung, M.: Coupling Water and Carbon Fluxes to Constrain Estimates of Transpiration: The TEA Algorithm, J. Geophys. Res.-Biogeo., 123, 3617–3632, 2018. a, b
Nelson, J. A., Pérez‐Priego, O., Zhou, S., Poyatos, R., Zhang, Y., Blanken, P. D., Gimeno, T. E., Wohlfahrt, G., Desai, A. R., Gioli, B., Limousin, J., Bonal, D., Paul‐Limoges, E., Scott, R. L., Varlagin, A., Fuchs, K., Montagnani, L., Wolf, S., Delpierre, N., Berveiller, D., Gharun, M., Belelli Marchesini, L., Gianelle, D., Šigut, L., Mammarella, I., Siebicke, L., Andrew Black, T., Knohl, A., Hörtnagl, L., Magliulo, V., Besnard, S., Weber, U., Carvalhais, N., Migliavacca, M., Reichstein, M., and Jung, M.: Ecosystem Transpiration and Evaporation: Insights from Three Water Flux Partitioning Methods Across FLUXNET Sites, Glob. Change Biol., 26, 6916–6930, 2020. a
Nelson, J. A., Walther, S., Jung, M., Gans, F., Kraft, B., Weber, U., Hamdi, Z., Duveiller, G., and Zhang, W.: FLUXCOM-X-BASE, ICOS [data set], https://doi.org/10.18160/5NZG-JMJE, 2023. a
Network, N.: AmeriFlux FLUXNET-1F US-xBR NEON Bartlett Experimental Forest (BART), [data set], https://doi.org/10.17190/amf/1881598, 2022. a
Nouvellon, Y.: FLUXNET2015 CG-Tch Tchizalamou, [data set], https://doi.org/10.18140/flx/1440142, 2016. a
Novick, K. and Phillips, R.: AmeriFlux FLUXNET-1F US-MMS Morgan Monroe State Forest, [data set], https://doi.org/10.17190/amf/1854369, 2022. a
Novick, K. A., Metzger, S., Anderegg, W. R. L., Barnes, M., Cala, D. S., Guan, K., Hemes, K. S., Hollinger, D. Y., Kumar, J., Litvak, M., Lombardozzi, D., Normile, C. P., Oikawa, P., Runkle, B. R. K., Torn, M., and Wiesner, S.: Informing Nature‐based Climate Solutions for the United States with the Best‐available Science, Glob. Change Biol., 28, 3778–3794, 2022. a
Oikawa, P.: AmeriFlux FLUXNET-1F US-EDN Eden Landing Ecological Reserve, [data set], https://doi.org/10.17190/amf/1832159, 2021. a
Olesen, J.: FLUXNET2015 DK-Fou Foulum, [data set], https://doi.org/10.18140/flx/1440154, 2016. a
Ourcival, J.-M.: FLUXNET2015 FR-Pue Puechabon, [data set], https://doi.org/10.18140/flx/1440164, 2016. a
Papale, D. and Valentini, R.: A New Assessment of European Forests Carbon Exchanges by Eddy Fluxes and Artificial Neural Network Spatialization, Glob. Change Biol., 9, 525–535, 2003. a
Papale, D., Reichstein, M., Aubinet, M., Canfora, E., Bernhofer, C., Kutsch, W., Longdoz, B., Rambal, S., Valentini, R., Vesala, T., and Yakir, D.: Towards a standardized processing of Net Ecosystem Exchange measured with eddy covariance technique: algorithms and uncertainty estimation, Biogeosciences, 3, 571–583, https://doi.org/10.5194/bg-3-571-2006, 2006. a
Papale, D., Black, T. A., Carvalhais, N., Cescatti, A., Chen, J., Jung, M., Kiely, G., Lasslop, G., Mahecha, M. D., Margolis, H., Merbold, L., Montagnani, L., Moors, E., Olesen, J. E., Reichstein, M., Tramontana, G., Gorsel, E., Wohlfahrt, G., and Ráduly, B.: Effect of Spatial Sampling from European Flux Towers for Estimating Carbon and Water Fluxes with Artificial Neural Networks, J. Geophys. Res.-Biogeo., 120, 1941–1957, 2015. a
Papale, D., Tirone, G., Valentini, R., Arriga, N., Belelli, L., Consalvo, C., Dore, S., Manca, G., Mazzenga, F., Sabbatini, S., and Stefani, P.: FLUXNET2015 IT-Ro2 Roccarespampani 2, [data set], https://doi.org/10.18140/flx/1440175, 2016. a
Pastorello, G., Trotta, C., Canfora, E., Chu, H., Christianson, D., Cheah, Y.-W., Poindexter, C., Chen, J., Elbashandy, A., Humphrey, M., Isaac, P., Polidori, D., Reichstein, M., Ribeca, A., van Ingen, C., Vuichard, N., Zhang, L., Amiro, B., Ammann, C., Arain, M. A., Ardö, J., Arkebauer, T., Arndt, S. K., Arriga, N., Aubinet, M., Aurela, M., Baldocchi, D., Barr, A., Beamesderfer, E., Marchesini, L. B., Bergeron, O., Beringer, J., Bernhofer, C., Berveiller, D., Billesbach, D., Black, T. A., Blanken, P. D., Bohrer, G., Boike, J., Bolstad, P. V., Bonal, D., Bonnefond, J.-M., Bowling, D. R., Bracho, R., Brodeur, J., Brümmer, C., Buchmann, N., Burban, B., Burns, S. P., Buysse, P., Cale, P., Cavagna, M., Cellier, P., Chen, S., Chini, I., Christensen, T. R., Cleverly, J., Collalti, A., Consalvo, C., Cook, B. D., Cook, D., Coursolle, C., Cremonese, E., Curtis, P. S., D’Andrea, E., da Rocha, H., Dai, X., Davis, K. J., Cinti, B. D., Grandcourt, A. d., Ligne, A. D., De Oliveira, R. C., Delpierre, N., Desai, A. R., Di Bella, C. M., Tommasi, P. d., Dolman, H., Domingo, F., Dong, G., Dore, S., Duce, P., Dufrêne, E., Dunn, A., Dušek, J., Eamus, D., Eichelmann, U., ElKhidir, H. A. M., Eugster, W., Ewenz, C. M., Ewers, B., Famulari, D., Fares, S., Feigenwinter, I., Feitz, A., Fensholt, R., Filippa, G., Fischer, M., Frank, J., Galvagno, M., Gharun, M., Gianelle, D., Gielen, B., Gioli, B., Gitelson, A., Goded, I., Goeckede, M., Goldstein, A. H., Gough, C. M., Goulden, M. L., Graf, A., Griebel, A., Gruening, C., Grünwald, T., Hammerle, A., Han, S., Han, X., Hansen, B. U., Hanson, C., Hatakka, J., He, Y., Hehn, M., Heinesch, B., Hinko-Najera, N., Hörtnagl, L., Hutley, L., Ibrom, A., Ikawa, H., Jackowicz-Korczynski, M., Janouš, D., Jans, W., Jassal, R., Jiang, S., Kato, T., Khomik, M., Klatt, J., Knohl, A., Knox, S., Kobayashi, H., Koerber, G., Kolle, O., Kosugi, Y., Kotani, A., Kowalski, A., Kruijt, B., Kurbatova, J., Kutsch, W. L., Kwon, H., Launiainen, S., Laurila, T., Law, B., Leuning, R., Li, Y., Liddell, M., Limousin, J.-M., Lion, M., Liska, A. J., Lohila, A., López-Ballesteros, A., López-Blanco, E., Loubet, B., Loustau, D., Lucas-Moffat, A., Lüers, J., Ma, S., Macfarlane, C., Magliulo, V., Maier, R., Mammarella, I., Manca, G., Marcolla, B., Margolis, H. A., Marras, S., Massman, W., Mastepanov, M., Matamala, R., Matthes, J. H., Mazzenga, F., McCaughey, H., McHugh, I., McMillan, A. M. S., Merbold, L., Meyer, W., Meyers, T., Miller, S. D., Minerbi, S., Moderow, U., Monson, R. K., Montagnani, L., Moore, C. E., Moors, E., Moreaux, V., Moureaux, C., Munger, J. W., Nakai, T., Neirynck, J., Nesic, Z., Nicolini, G., Noormets, A., Northwood, M., Nosetto, M., Nouvellon, Y., Novick, K., Oechel, W., Olesen, J. E., Ourcival, J.-M., Papuga, S. A., Parmentier, F.-J., Paul-Limoges, E., Pavelka, M., Peichl, M., Pendall, E., Phillips, R. P., Pilegaard, K., Pirk, N., Posse, G., Powell, T., Prasse, H., Prober, S. M., Rambal, S., Rannik, U., Raz-Yaseef, N., Rebmann, C., Reed, D., Dios, V. R. d., Restrepo-Coupe, N., Reverter, B. R., Roland, M., Sabbatini, S., Sachs, T., Saleska, S. R., Sánchez-Cañete, E. P., Sanchez-Mejia, Z. M., Schmid, H. P., Schmidt, M., Schneider, K., Schrader, F., Schroder, I., Scott, R. L., Sedlák, P., Serrano-Ortíz, P., Shao, C., Shi, P., Shironya, I., Siebicke, L., Šigut, L., Silberstein, R., Sirca, C., Spano, D., Steinbrecher, R., Stevens, R. M., Sturtevant, C., Suyker, A., Tagesson, T., Takanashi, S., Tang, Y., Tapper, N., Thom, J., Tomassucci, M., Tuovinen, J.-P., Urbanski, S., Valentini, R., van der Molen, M., van Gorsel, E., van Huissteden, K., Varlagin, A., Verfaillie, J., Vesala, T., Vincke, C., Vitale, D., Vygodskaya, N., Walker, J. P., Walter-Shea, E., Wang, H., Weber, R., Westermann, S., Wille, C., Wofsy, S., Wohlfahrt, G., Wolf, S., Woodgate, W., Li, Y., Zampedri, R., Zhang, J., Zhou, G., Zona, D., Agarwal, D., Biraud, S., Torn, M., and Papale, D.: The FLUXNET2015 Dataset and the ONEFlux Processing Pipeline for Eddy Covariance Data, Sci. Data, 7, 225, https://doi.org/10.1038/s41597-020-0534-3, 2020. a, b, c
Pendall, E. and Griebel, A.: FLUXNET2015 AU-Cum Cumberland Plains, [data set], https://doi.org/10.18140/flx/1440196, 2016. a
Pilegaard, K. and Ibrom, A.: FLUXNET2015 DK-Eng Enghave, [data set], https://doi.org/10.18140/flx/1440153, 2016. a
Posse, G., Lewczuk, N., Richter, K., and Cristiano, P.: FLUXNET2015 AR-Vir Virasoro, [data set], https://doi.org/10.18140/flx/1440192, 2016. a
Poveda, F. D., Ballesteros, A. L., Cañete, E. P. S., Ortiz, P. S., Jiménez, M. R. M., Priego, O. P., and Kowalski, A. S.: FLUXNET2015 ES-Amo Amoladeras, [data set], https://doi.org/10.18140/flx/1440156, 2016. a
Priestley, C. H. B. and Taylor, R. J.: On the Assessment of Surface Heat Flux and Evaporation Using Large-Scale Parameters, Mon. Weather Rev., 100, 81–92, 1972. a
Randerson, J., Van der werf, G., Giglio, L., Collatz, G., and Kasibhatla, P.: Global Fire Emissions Database, Version 4.1 (GFEDv4), [data set], https://doi.org/10.3334/ornldaac/1293, 2017. a, b
Rannik, U., Peltola, O., and Mammarella, I.: Random uncertainties of flux measurements by the eddy covariance technique, Atmos. Meas. Tech., 9, 5163–5181, https://doi.org/10.5194/amt-9-5163-2016, 2016. a
Reichstein, M., Falge, E., Baldocchi, D., Papale, D., Aubinet, M., Berbigier, P., Bernhofer, C., Buchmann, N., Gilmanov, T., Granier, A., Grunwald, T., Havrankova, K., Ilvesniemi, H., Janous, D., Knohl, A., Laurila, T., Lohila, A., Loustau, D., Matteucci, G., Meyers, T., Miglietta, F., Ourcival, J.-M., Pumpanen, J., Rambal, S., Rotenberg, E., Sanz, M., Tenhunen, J., Seufert, G., Vaccari, F., Vesala, T., Yakir, D., and Valentini, R.: On the Separation of Net Ecosystem Exchange into Assimilation and Ecosystem Respiration: Review and Improved Algorithm, Glob. Change Biol., 11, 1424–1439, 2005. a, b
Reichstein, M., Camps-Valls, G., Stevens, B., Jung, M., Denzler, J., Carvalhais, N., and Prabhat: Deep Learning and Process Understanding for Data-Driven Earth System Science, Nature, 566, 195–204, 2019. a
Reverter, B. R., Perez-Cañete, E. S., and Kowalski, A. S.: FLUXNET2015 ES-Ln2 Lanjaron-Salvage Logging, [data set], https://doi.org/10.18140/flx/1440225, 2016a. a
Reverter, B. R., Perez-Cañete, E. S., and Kowalski, A. S.: FLUXNET2015 ES-LgS Laguna Seca, [data set], https://doi.org/10.18140/flx/1440225, 2016b. a
Rey-Sanchez, C., Wang, C., Szutu, D., Hemes, K., Verfaillie, J., and Baldocchi, D.: AmeriFlux FLUXNET-1F US-Bi2 Bouldin Island Corn, [data set], https://doi.org/10.18140/flx/1440225, 2022a. a
Rey-Sanchez, C., Wang, C., Szutu, D., Shortt, R., Chamberlain, S., Verfaillie, J., and Baldocchi, D.: AmeriFlux FLUXNET-1F US-Bi1 Bouldin Island Alfalfa, [data set], https://doi.org/10.17190/amf/1871134, 2022b. a
RI, I.: Ecosystem Final Quality (L2) Product in ETC-Archive Format – Release 2021-1, [data set], https://doi.org/10.18160/fzmy-pg92, 2021. a
RI, I.: Ecosystem Final Quality (L2) Product in ETC-Archive Format – Release 2022-1, [data set], https://doi.org/10.18160/pad9-hqhu, 2022. a, b, c, d, e, f, g, h, i
Roedenbeck, C. and Heimann, M.: Jena CarboScope: Atmospheric CO2 Inversion, [data set], https://doi.org/10.17871/carboscope-sextocneet_v2022, 2022. a
Rödenbeck, C., Zaehle, S., Keeling, R., and Heimann, M.: How does the terrestrial carbon exchange respond to inter-annual climatic variations? A quantification based on atmospheric CO2 data, Biogeosciences, 15, 2481–2498, https://doi.org/10.5194/bg-15-2481-2018, 2018. a
Sabbatini, S., Arriga, N., Gioli, B., and Papale, D.: FLUXNET2015 IT-CA2 Castel d’Asso2, [data set], https://doi.org/10.18140/flx/1440231, 2016a. a
Sabbatini, S., Arriga, N., Matteucci, G., and Papale, D.: FLUXNET2015 IT-CA3 Castel d’Asso 3, [data set], https://doi.org/10.18140/flx/1440232, 2016b. a
Sabbatini, S., Arriga, N., and Papale, D.: FLUXNET2015 IT-CA1 Castel d’Asso1, [data set], https://doi.org/10.18140/flx/1440230, 2016c. a
Sachs, T., Wille, C., Larmanou, E., and Franz, D.: FLUXNET2015 DE-Zrk Zarnekow, [data set], https://doi.org/10.18140/flx/1440221, 2016. a
Saleska, S.: FLUXNET2015 BR-Sa1 Santarem-Km67-Primary Forest, [data set], https://doi.org/10.18140/flx/1440032, 2016. a
Sanchez, C. R., Sturtevant, C., Szutu, D., Baldocchi, D., Eichelmann, E., and Knox, S.: FLUXNET2015 US-Tw4 Twitchell East End Wetland, [data set], https://doi.org/10.18140/flx/1440111, 2016. a
Schaaf, C. and Wang, Z.: MCD43A2 MODIS/Terra+Aqua BRDF/Albedo Quality Daily L3 Global – 500m V006, [data set], https://doi.org/10.5067/modis/mcd43a2.006, 2015a. a
Schaaf, C. and Wang, Z.: MCD43A4 MODIS/Terra+Aqua BRDF/Albedo Nadir BRDF Adjusted Ref Daily L3 Global – 500m V006, [data set], https://doi.org/10.5067/modis/mcd43a4.006, 2015b. a, b
Schlesinger, W. H. and Jasechko, S.: Transpiration in the Global Water Cycle, Agr. Forest Meteorol., 189-190, 115–117, 2014. a
Schneider, K. and Schmidt, M.: FLUXNET2015 DE-Seh Selhausen, [data set], https://doi.org/10.18140/flx/1440217, 2016. a
Schneider, U., Hänsel, S., Finger, P., Rustemeier, E., and Ziese, M.: GPCC Full Data Monthly Version 2022 at 0.25°: Monthly Land-Surface Precipitation from Rain-Gauges Built on GTS-based and Historic Data, [data set], https://doi.org/10.5676/dwd_gpcc/fd_m_v2022_025, 2022. a, b, c
Schroder, I., Zegelin, S., Palu, T., and Feitz, A.: FLUXNET2015 AU-Emr Emerald, [data set], https://doi.org/10.18140/flx/1440198, 2016. a
Scott, R.: FLUXNET2015 US-SRG Santa Rita Grassland, [data set], https://doi.org/10.18140/flx/1440114, 2016a. a
Scott, R.: FLUXNET2015 US-SRM Santa Rita Mesquite, [data set], https://doi.org/10.18140/flx/1440090, 2016b. a
Scott, R.: FLUXNET2015 US-Wkg Walnut Gulch Kendall Grasslands, [data set], https://doi.org/10.18140/flx/1440096, 2016c. a
Scott, R.: FLUXNET2015 US-Whs Walnut Gulch Lucky Hills Shrub, [data set], https://doi.org/10.18140/flx/1440097, 2016d. a
Shao, C.: FLUXNET2015 CN-Sw2 Siziwang Grazed (SZWG), [data set], https://doi.org/10.18140/flx/1440212, 2016a. a
Shao, C.: FLUXNET2015 CN-Du3 Duolun Degraded Meadow, [data set], https://doi.org/10.18140/flx/1440210, 2016b. a
Shi, P., Zhang, X., and He, Y.: FLUXNET2015 CN-Dan Dangxiong, [data set], https://doi.org/10.18140/flx/1440138, 2016. a
Shortt, R., Hemes, K., Szutu, D., Verfaillie, J., and Baldocchi, D.: AmeriFlux FLUXNET-1F US-Sne Sherman Island Restored Wetland, [data set], https://doi.org/10.17190/amf/1871144, 2022. a
Sigut, L., Havrankova, K., Jocher, G., Pavelka, M., and Janouš, D.: FLUXNET2015 CZ-BK2 Bily Kriz Grassland, [data set], https://doi.org/10.18140/flx/1440144, 2016. a
Silveira, M.: AmeriFlux FLUXNET-1F US-ONA Florida Pine Flatwoods, [data set], https://doi.org/10.17190/amf/1832163, 2021. a
Soloway, A. D., Amiro, B. D., Dunn, A. L., and Wofsy, S. C.: Carbon neutral or a sink? Uncertainty caused by gap-filling long-term flux measurements for an old-growth boreal black spruce forest, Agr. Forest Meteorol., 233, 110–121, https://doi.org/10.1016/j.agrformet.2016.11.005, 2017. a
Spano, D., Duce, P., Marras, S., Sirca, C., Arca, A., Zara, P., and Ventura, A.: FLUXNET2015 IT-Noe Arca Di Noe – Le Prigionette, [data set], https://doi.org/10.18140/flx/1440171, 2016. a
Staebler, R.: AmeriFlux FLUXNET-1F CA-Cbo Ontario – Mixed Deciduous, Borden Forest Site, [data set], https://doi.org/10.17190/amf/1854365, 2022. a
Stoy, P. C., Mauder, M., Foken, T., Marcolla, B., Boegh, E., Ibrom, A., Arain, M. A., Arneth, A., Aurela, M., Bernhofer, C., Cescatti, A., Dellwik, E., Duce, P., Gianelle, D., van Gorsel, E., Kiely, G., Knohl, A., Margolis, H., McCaughey, H., Merbold, L., Montagnani, L., Papale, D., Reichstein, M., Saunders, M., Serrano-Ortiz, P., Sottocornola, M., Spano, D., Vaccari, F., and Varlagin, A.: A data-driven analysis of energy balance closure across FLUXNET research sites: The role of landscape scale heterogeneity, Agr. Forest Meteorol., 171/172, 137–152, https://doi.org/10.1016/j.agrformet.2012.11.004, 2013. a
Sturtevant, C., Szutu, D., Baldocchi, D., Matthes, J. H., Oikawa, P., and Chamberlain, S. D.: FLUXNET2015 US-Myb Mayberry Wetland, [data set], https://doi.org/10.18140/flx/1440105, 2016. a
Sturtevant, C., Verfaillie, J., and Baldocchi, D.: AmeriFlux FLUXNET-1F US-Tw2 Twitchell Corn, [data set], https://doi.org/10.17190/amf/1881593, 2022. a
Suyker, A.: FLUXNET2015 US-Ne3 Mead – Rainfed Maize-Soybean Rotation Site, [data set], https://doi.org/10.18140/flx/1440086, 2016a. a
Suyker, A.: FLUXNET2015 US-Ne2 Mead – Irrigated Maize-Soybean Rotation Site, [data set], https://doi.org/10.17190/amf/1871140, 2016b. a
Suyker, A.: AmeriFlux FLUXNET-1F US-Ne1 Mead – Irrigated Continuous Maize Site, [data set], https://doi.org/10.18140/flx/1440085, 2022. a
Tagesson, T., Ardö, J., and Fensholt, R.: FLUXNET2015 SN-Dhr Dahra, [data set], https://doi.org/10.18140/flx/1440246, 2016. a
Tang, Y., Kato, T., and Du, M.: FLUXNET2015 CN-HaM Haibei Alpine Tibet Site, [data set], https://doi.org/10.18140/flx/1440190, 2016. a
Team, D. . and Centre, I. E. T.: Drought-2018 Ecosystem Eddy Covariance Flux Product for 52 Stations in FLUXNET-Archive Format, [data set], https://doi.org/10.18160/yvr0-4898, 2020. a, b, c, d
Team, W. W. . and Centre, I. E. T.: Warm Winter 2020 Ecosystem Eddy Covariance Flux Product for 73 Stations in FLUXNET-Archive Format, [data set], https://doi.org/10.18160/2g60-zhak, 2022. a, b, c, d, e, f, g, h, i, j, k, l, m, n, o, p, q, r, s, t, u, v, w, x, y, z, aa, ab, ac, ad, ae, af, ag, ah, ai, aj, ak, al, am, an, ao, ap, aq, ar, as, at, au, av, aw, ax, ay, az, ba, bb, bc, bd, be, bf, bg, bh, bi, bj, bk, bl, bm
Torn, M.: FLUXNET2015 US-ARc ARM Southern Great Plains Control Site- Lamont, [data set], https://doi.org/10.18140/flx/1440065, 2016a. a
Torn, M.: FLUXNET2015 US-ARb ARM Southern Great Plains Burn Site- Lamont, [data set], https://doi.org/10.18140/flx/1440064, 2016b. a
Torn, M. and Dengel, S.: AmeriFlux FLUXNET-1F US-NGB NGEE Arctic Barrow, [data set], https://doi.org/10.17190/amf/1832162, 2021. a
Tramontana, G., Jung, M., Schwalm, C. R., Ichii, K., Camps-Valls, G., Ráduly, B., Reichstein, M., Arain, M. A., Cescatti, A., Kiely, G., Merbold, L., Serrano-Ortiz, P., Sickert, S., Wolf, S., and Papale, D.: Predicting carbon dioxide and energy fluxes across global FLUXNET sites with regression algorithms, Biogeosciences, 13, 4291–4313, https://doi.org/10.5194/bg-13-4291-2016, 2016. a, b, c, d
Turner, D. P., Ritts, W. D., Cohen, W. B., Gower, S. T., Running, S. W., Zhao, M., Costa, M. H., Kirschbaum, A. A., Ham, J. M., Saleska, S. R., and Ahl, D. E.: Evaluation of MODIS NPP and GPP Products Across Multiple Biomes, Remote Sens. Environ., 102, 282–292, 2006. a
Ukkola, A. M., Abramowitz, G., and De Kauwe, M. G.: A flux tower dataset tailored for land model evaluation, Earth Syst. Sci. Data, 14, 449–461, https://doi.org/10.5194/essd-14-449-2022, 2022. a
Upton, S., Reichstein, M., Gans, F., Peters, W., Kraft, B., and Bastos, A.: Constraining Biospheric Carbon Dioxide Fluxes by Combined Top-down and Bottom-up Approaches, 2023. a
Valach, A., Shortt, R., Szutu, D., Eichelmann, E., Knox, S., Hemes, K., Verfaillie, J., and Baldocchi, D.: AmeriFlux FLUXNET-1F US-Tw1 Twitchell Wetland West Pond, [data set], https://doi.org/10.17190/amf/1832165, 2021. a
Valach, A., Kasak, K., Szutu, D., Verfaillie, J., and Baldocchi, D.: AmeriFlux FLUXNET-1F US-Tw5 East Pond Wetland, [data set], https://doi.org/10.17190/amf/1832162 2022. a
Valentini, R., Dore, S., Mazzenga, F., Sabbatini, S., Stefani, P., Tirone, G., and Papale, D.: FLUXNET2015 IT-Cpz Castelporziano, [data set], https://doi.org/10.18140/flx/1440168, 2016a. a
Valentini, R., Nicolini, G., Stefani, P., De Grandcourt, A., and Stivanello, S.: FLUXNET2015 GH-Ank Ankasa, [data set], https://doi.org/10.18140/flx/1440229, 2016b. a
Valentini, R., Tirone, G., Vitale, D., Papale, D., Arriga, N., Belelli, L., Dore, S., Manca, G., Mazzenga, F., Pegoraro, E., Sabbatini, S., and Stefani, P.: FLUXNET2015 IT-Ro1 Roccarespampani 1, [data set], https://doi.org/10.18140/flx/1440174, 2016c. a
Vekuri, H., Tuovinen, J.-P., Kulmala, L., Papale, D., Kolari, P., Aurela, M., Laurila, T., Liski, J., and Lohila, A.: A widely-used eddy covariance gap-filling method creates systematic bias in carbon balance estimates, Sci. Rep., 13, 1720, https://doi.org/10.1038/s41598-023-28827-2, 2023. a
Virkkala, A., Aalto, J., Rogers, B. M., Tagesson, T., Treat, C. C., Natali, S. M., Watts, J. D., Potter, S., Lehtonen, A., Mauritz, M., Schuur, E. A. G., Kochendorfer, J., Zona, D., Oechel, W., Kobayashi, H., Humphreys, E., Goeckede, M., Iwata, H., Lafleur, P. M., Euskirchen, E. S., Bokhorst, S., Marushchak, M., Martikainen, P. J., Elberling, B., Voigt, C., Biasi, C., Sonnentag, O., Parmentier, F. W., Ueyama, M., Celis, G., St.Louis, V. L., Emmerton, C. A., Peichl, M., Chi, J., Järveoja, J., Nilsson, M. B., Oberbauer, S. F., Torn, M. S., Park, S., Dolman, H., Mammarella, I., Chae, N., Poyatos, R., López‐Blanco, E., Christensen, T. R., Kwon, M. J., Sachs, T., Holl, D., and Luoto, M.: Statistical Upscaling of Ecosystem CO2 fluxes Across the Terrestrial Tundra and Boreal Domain: Regional Patterns and Uncertainties, Glob. Change Biol., 27, 4040–4059, 2021. a, b
Vitale, D., Fratini, G., Bilancia, M., Nicolini, G., Sabbatini, S., and Papale, D.: A robust data cleaning procedure for eddy covariance flux measurements, Biogeosciences, 17, 1367–1391, https://doi.org/10.5194/bg-17-1367-2020, 2020. a
Vivoni, E. and Perez-Ruiz, E.: AmeriFlux FLUXNET-1F US-Jo2 Jornada Experimental Range Mixed Shrubland, [data set], https://doi.org/10.17190/amf/1881584 2022. a
Vourlitis, G., Dalmagro, H., De S. Nogueira, J., Johnson, M., and Arruda, P.: AmeriFlux FLUXNET-1F BR-Npw Northern Pantanal Wetland, [data set], https://doi.org/10.17190/amf/1881584, 2022. a
Wagner-Riddle, C.: AmeriFlux FLUXNET-1F CA-ER1 Elora Research Station, [data set], https://doi.org/10.17190/amf/1832154, 2021. a
Walther, S.: README FluxnetEO V2, 2023. a
Walther, S., Besnard, S., Nelson, J. A., El-Madany, T. S., Migliavacca, M., Weber, U., Carvalhais, N., Ermida, S. L., Brümmer, C., Schrader, F., Prokushkin, A. S., Panov, A. V., and Jung, M.: Technical note: A view from space on global flux towers by MODIS and Landsat: the FluxnetEO data set, Biogeosciences, 19, 2805–2840, https://doi.org/10.5194/bg-19-2805-2022, 2022. a, b, c, d
Wan, Z., Hook, S., and Hulley, G.: MOD11C1 MODIS/Terra Land Surface Temperature/Emissivity Daily L3 Global 0.05Deg CMG V006, [data set], https://doi.org/10.5067/modis/mod11c1.006, 2015. a, b
Wang, H. and Fu, X.: FLUXNET2015 CN-Qia Qianyanzhou, [data set], https://doi.org/10.18140/flx/1440141, 2016. a
Wei, Z., Yoshimura, K., Wang, L., Miralles, D. G., Jasechko, S., and Lee, X.: Revisiting the Contribution of Transpiration to Global Terrestrial Evapotranspiration, Geophys. Res. Lett., 44, 2792–2801, 2017. a
Wohlfahrt, G., Hammerle, A., and Hörtnagl, L.: FLUXNET2015 AT-Neu Neustift, [data set], https://doi.org/10.18140/flx/1440121, 2016. a
Wolf, S., Eugster, W., and Buchmann, N.: FLUXNET2015 PA-SPs Sardinilla-Pasture, [data set], https://doi.org/10.18140/flx/1440179, 2016a. a
Wolf, S., Eugster, W., and Buchmann, N.: FLUXNET2015 PA-SPn Sardinilla Plantation, [data set], https://doi.org/10.18140/flx/1440180, 2016b. a
Wood, J. and Gu, L.: AmeriFlux FLUXNET-1F US-MOz Missouri Ozark Site, [data set], https://doi.org/10.17190/amf/1854370, 2022. a
Woodgate, W., Van Gorsel, E., and Leuning, R.: FLUXNET2015 AU-Tum Tumbarumba, [data set], https://doi.org/10.18140/flx/1440126, 2016. a
Xiao, J., Zhuang, Q., Baldocchi, D. D., Law, B. E., Richardson, A. D., Chen, J., Oren, R., Starr, G., Noormets, A., Ma, S., Verma, S. B., Wharton, S., Wofsy, S. C., Bolstad, P. V., Burns, S. P., Cook, D. R., Curtis, P. S., Drake, B. G., Falk, M., Fischer, M. L., Foster, D. R., Gu, L., Hadley, J. L., Hollinger, D. Y., Katul, G. G., Litvak, M., Martin, T. A., Matamala, R., McNulty, S., Meyers, T. P., Monson, R. K., Munger, J. W., Oechel, W. C., Paw U, K. T., Schmid, H. P., Scott, R. L., Sun, G., Suyker, A. E., and Torn, M. S.: Estimation of Net Ecosystem Carbon Exchange for the Conterminous United States by Combining MODIS and AmeriFlux Data, Agr. Forest Meteorol., 148, 1827–1847, 2008. a
Yao, Y., Li, Z., Wang, T., Chen, A., Wang, X., Du, M., Jia, G., Li, Y., Li, H., Luo, W., Ma, Y., Tang, Y., Wang, H., Wu, Z., Yan, J., Zhang, X., Zhang, Y., Zhang, Y., Zhou, G., and Piao, S.: A New Estimation of China’s Net Ecosystem Productivity Based on Eddy Covariance Measurements and a Model Tree Ensemble Approach, Agr. Forest Meteorol., 253-254, 84–93, 2018. a
Yepez, E. and Garatuza, J.: AmeriFlux FLUXNET-1F MX-Tes Tesopaco, Secondary Tropical Dry Forest, [data set], https://doi.org/10.17190/amf/1832156, 2021. a
Zhang, J. and Han, S.: FLUXNET2015 CN-Cha Changbaishan, [data set], https://doi.org/10.18140/flx/1440137, 2016. a
Zhang, W., Jung, M., Migliavacca, M., Poyatos, R., Miralles, D. G., El-Madany, T. S., Galvagno, M., Carrara, A., Arriga, N., Ibrom, A., Mammarella, I., Papale, D., Cleverly, J. R., Liddell, M., Wohlfahrt, G., Markwitz, C., Mauder, M., Paul-Limoges, E., Schmidt, M., Wolf, S., Brümmer, C., Arain, M. A., Fares, S., Kato, T., Ardö, J., Oechel, W., Hanson, C., Korkiakoski, M., Biraud, S., Steinbrecher, R., Billesbach, D., Montagnani, L., Woodgate, W., Shao, C., Carvalhais, N., Reichstein, M., and Nelson, J. A.: The effect of relative humidity on eddy covariance latent heat flux measurements and its implication for partitioning into transpiration and evaporation, Agr. Forest Meteorol., 330, 109305, https://doi.org/10.1016/j.agrformet.2022.109305, 2023. a
Zhang, Y., Xiao, X., Zhang, Y., Wolf, S., Zhou, S., Joiner, J., Guanter, L., Verma, M., Sun, Y., Yang, X., Paul-Limoges, E., Gough, C. M., Wohlfahrt, G., Gioli, B., van der Tol, C., Yann, N., Lund, M., and de Grandcourt, A.: On the Relationship Between Sub-Daily Instantaneous and Daily Total Gross Primary Production: Implications for Interpreting Satellite-Based SIF Retrievals, Remote Sens. Environ., 205, 276–289, 2018. a
Zhao, M. and Running, S. W.: Drought-Induced Reduction in Global Terrestrial Net Primary Production from 2000 Through 2009, Science, 329, 940–943, 2010. a
Zhou, G. and Yan, J.: FLUXNET2015 CN-Din Dinghushan, [data set], https://doi.org/10.18140/flx/1440139, 2016. a
Zhu, S., Quaife, T., and Hill, T.: Uniform upscaling techniques for eddy covariance FLUXes (UFLUX), Int. J. Remote Sens., 45, 1450–1476, https://doi.org/10.1080/01431161.2024.2312266, 2024. a
Zona, D. and Oechel, W.: FLUXNET2015 US-Atq Atqasuk, [data set], https://doi.org/10.18140/flx/1440067, 2016a. a
Zona, D. and Oechel, W.: FLUXNET2015 US-Ivo Ivotuk, [data set], https://doi.org/10.18140/flx/1440073, 2016b. a
Zscheischler, J., Mahecha, M. D., Avitabile, V., Calle, L., Carvalhais, N., Ciais, P., Gans, F., Gruber, N., Hartmann, J., Herold, M., Ichii, K., Jung, M., Landschützer, P., Laruelle, G. G., Lauerwald, R., Papale, D., Peylin, P., Poulter, B., Ray, D., Regnier, P., Rödenbeck, C., Roman-Cuesta, R. M., Schwalm, C., Tramontana, G., Tyukavina, A., Valentini, R., van der Werf, G., West, T. O., Wolf, J. E., and Reichstein, M.: Reviews and syntheses: An empirical spatiotemporal description of the global surface–atmosphere carbon fluxes: opportunities and data limitations, Biogeosciences, 14, 3685–3703, https://doi.org/10.5194/bg-14-3685-2017, 2017. a, b
- Abstract
- Introduction
- Data and methods
- Results
- Discussion
- Tackling persistent challenges
- Conclusions
- Appendix A: Naming conventions
- Appendix B: Details on processing of Earth observation data
- Appendix C: Additional results
- Data availability
- Author contributions
- Competing interests
- Disclaimer
- Acknowledgements
- Financial support
- Review statement
- References
Please read the editorial note first before accessing the article.
- Article
(13321 KB) - Full-text XML
- Abstract
- Introduction
- Data and methods
- Results
- Discussion
- Tackling persistent challenges
- Conclusions
- Appendix A: Naming conventions
- Appendix B: Details on processing of Earth observation data
- Appendix C: Additional results
- Data availability
- Author contributions
- Competing interests
- Disclaimer
- Acknowledgements
- Financial support
- Review statement
- References