the Creative Commons Attribution 4.0 License.
the Creative Commons Attribution 4.0 License.
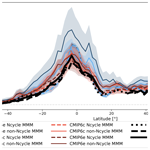
Representation of the terrestrial carbon cycle in CMIP6
Manuel Schlund
Pierre Friedlingstein
Chris D. Jones
Colin Jones
Sönke Zaehle
Veronika Eyring
Simulation of the carbon cycle in climate models is important due to its impact on climate change, but many weaknesses in its reproduction were found in previous models. Improvements in the representation of the land carbon cycle in Earth system models (ESMs) participating in the Coupled Model Intercomparison Project Phase 6 (CMIP6) include the interactive treatment of both the carbon and nitrogen cycles, improved photosynthesis, and soil hydrology. To assess the impact of these model developments on aspects of the global carbon cycle, the Earth System Model Evaluation Tool (ESMValTool) is expanded to compare CO2-concentration- and CO2-emission-driven historical simulations from CMIP5 and CMIP6 to observational data sets. A particular focus is on the differences in models with and without an interactive terrestrial nitrogen cycle. Overestimations of photosynthesis (gross primary productivity (GPP)) in CMIP5 were largely resolved in CMIP6 for participating models with an interactive nitrogen cycle but remaining for models without one. This points to the importance of including nutrient limitation. Simulating the leaf area index (LAI) remains challenging, with a large model spread in both CMIP5 and CMIP6. In ESMs, the global mean land carbon uptake (net biome productivity (NBP)) is well reproduced in the CMIP5 and CMIP6 multi-model means. However, this is the result of an underestimation of NBP in the Northern Hemisphere, which is compensated by an overestimation in the Southern Hemisphere and the tropics. Carbon stocks remain a large uncertainty in the models. While vegetation carbon content is slightly better represented in CMIP6, the inter-model range of soil carbon content remains the same between CMIP5 and CMIP6. Overall, a slight improvement in the simulation of land carbon cycle parameters is found in CMIP6 compared to CMIP5, but with many biases remaining, further improvements of models in particular for LAI and NBP is required. Models from modeling groups participating in both CMIP phases generally perform similarly or better in their CMIP6 compared to their CMIP5 models. This improvement is not as significant in the multi-model means due to more new models in CMIP6, especially those using older versions of the Community Land Model (CLM). Emission-driven simulations perform just as well as the concentration-driven models, despite the added process realism. Due to this, we recommend that ESMs in future Coupled Model Intercomparison Project (CMIP) phases perform emission-driven simulations as the standard so that climate–carbon cycle feedbacks are fully active. The inclusion of the nitrogen limitation led to a large improvement in photosynthesis compared to models not including this process, suggesting the need to view the nitrogen cycle as a necessary part of all future carbon cycle models. Possible benefits when including further limiting nutrients such as phosphorus should also be considered.
- Article
(16329 KB) - Full-text XML
-
Supplement
(367 KB) - BibTeX
- EndNote
Earth system models (ESMs) simulate the climate system by interactively coupling physical general circulation models of the atmosphere, ocean, land, and cryosphere with biogeochemical and biophysical cycles (Jones, 2020). The Coupled Model Intercomparison Project (CMIP; Meehl et al., 2000) was established to facilitate a consistent comparison between different ESMs through the use of common forcings and a uniform output structure in order to better understand past, present, and future climate. The newest phase, CMIP6 (Eyring et al., 2016a), provides a large ensemble of model simulations and includes 23 CMIP6-Endorsed Model Intercomparison Projects (MIPs) which facilitate a better analysis of specific scientific questions. Every new phase of CMIP sees additional models and improved model components, making a comparison to previous phases vital to determine if known systematic biases have been reduced and model weaknesses were identified and overcome (Eyring et al., 2019) or if increased model realism through the inclusion of additional processes introduces new biases. Increasing the process realism of models, for example, by replacing time-invariant observation-based fields with interactive prognostic ones, while having a neutral impact on the present-day performance of the model, can be viewed as a successful step in model improvement. This is particularly important for more self-consistent climate projections. Bock et al. (2020) assessed atmospheric variables of the CMIP6 ensemble and compared them to CMIP3 and CMIP5 output. They find that for temperature, precipitation, water vapor, and zonal wind speed, many long-standing biases remain in the multi-model mean, but individual models and high-resolution versions of models show some improvements for temperature and precipitation. In this study, we expand this assessment to the carbon cycle.
The carbon cycle is an important part of ESMs due to the key role of anthropogenic emissions of carbon dioxide (CO2) in driving climate change (IPCC, 2021). The land carbon cycle dominates the uncertainty in the global carbon cycle (Canadell et al., 2021). The Intergovernmental Panel on Climate Change (IPCC) Fifth Assessment Report (AR5) was largely supported by model simulations from the CMIP5 ensemble, and CMIP6 models were an important input to AR6 (IPCC, 2021). It is important to evaluate these models and document their changes compared to CMIP5. The focus of this study is on the results of the CMIP6 historical simulations that are split into simulations with prescribed greenhouse gas concentrations and those with prescribed CO2 emissions. Simulations driven by carbon emissions require an interactive carbon cycle to determine the distribution of natural and anthropogenic carbon fluxes across the land, marine, and atmospheric reservoirs instead of relying on prescribed atmospheric CO2 concentrations (Friedlingstein et al., 2014). Therefore, only emission-driven simulations have fully active climate–carbon cycle feedbacks vital for self-consistent future projections. Sanderson et al. (2024) thus petition for the use of emission-driven simulations to be prioritized in CMIP7. This leads to the further importance of the evaluation of the carbon cycle as more models will be required to implement and improve their interactive carbon cycle. An analysis of idealized 1 % CO2 increase per year (1pctCO2) simulations for CMIP5 and CMIP6 models with respect to carbon concentration and carbon–climate feedbacks has been carried out by Arora et al. (2020). They found that while these feedback parameters have not changed significantly between CMIP5 and CMIP6, the land feedback parameters are weaker for models including a nitrogen cycle coupled to the carbon cycle. Davies-Barnard et al. (2020) documented the development and inclusion of modeling of the terrestrial nitrogen cycle in ESM land surface schemes and showed how this affects the response to elevated CO2 across models. They find that these models show a more accurate response in the tropics than high-latitudes compared with observed responses. Gier et al. (2020) investigated the atmospheric CO2 concentrations for emission-driven CMIP5 and CMIP6 models and found that while CMIP6 models show an improvement in reproducing the observations compared to CMIP5, during the period of the satellite observations (2003–2014) the growth rate is overestimated, and the seasonal cycle amplitude is underestimated in both CMIP5 and CMIP6 relative to observations. Furthermore, the model spread in simulated atmospheric CO2 (∼ 45 ppmv in CMIP5, ∼ 35 ppmv in CMIP6) was found to remain many times larger than the observational uncertainty of under 1 ppmv (parts per million by volume) over this period.
Anav et al. (2013a) investigated the land and ocean carbon cycle for CMIP5 historical model simulations. While most models reproduced the main climatic variables and their seasonal evolution correctly, weaknesses were found in their ability to reproduce more specific biogeochemical fields such as a general overestimation of photosynthesis. Consequently, these were some of the main areas tackled in updating and enhancing the land carbon models of the ESMs for CMIP6, including the addition of a coupled nitrogen cycle and nitrogen limitation, which can limit the rates of carbon cycling through vegetation and soil, as well as photosynthesis updates and soil hydrology improvements (e.g., Danabasoglu et al., 2020; Delire et al., 2020; Wiltshire et al., 2021). Jones et al. (2023) drew on an expert assessment of regional carbon budgets to evaluate the terrestrial carbon cycle in CMIP6 models. They found that the multi-model mean performs well in most regions for most variables (both carbon fluxes and stocks), but individual models have strengths and weaknesses. This paper assesses the impacts of the model improvements and additions in CMIP6, especially the impact of an additional coupled nitrogen cycle, and whether they help overcome some of the weaknesses identified in CMIP5. Expanding on the analysis from Anav et al. (2013a) for CMIP5, this study uses CMIP6 concentration- and emission-driven historical simulations to compare to the land carbon cycle in CMIP5 and identify possible improvements in the newer model generation. In this study, we restrict ESM evaluation to data sets with global extent.
Section 2 describes the data used in this study, while Sect. 3 contains the analysis of different carbon cycle variables in the comparison between CMIP ensembles and observations. Long-term trends and the seasonal cycle of carbon cycle variables are considered, and the analysis is concluded with a performance metrics plot evaluating the climatological seasonal cycle of the models with different observational data sets. Section 4 summarizes the results and conclusions.
Law et al. (2017)Ziehn et al.20172020Ziehn et al. (2019a, b)Swart et al. (2019a)Swart et al. (2019e, b)Swart et al. (2019a)Swart et al. (2019d, c)Danabasoglu et al.2020Danabasoglu (2019b)Danabasoglu et al.2020Danabasoglu (2019a)Cherchi et al. (2019)Lovato and Peano2020Lovato et al. (2022)Lovato et al. (2021)Séférian et al. (2019)Séférian (2018, 2019)Döscher et al. (2022)EC-Earth Consortium2021b2021aDöscher et al. (2022)EC-Earth Consortium2019Dunne et al. (2020)Krasting et al.2018a2018bVolodin et al. (2018)Volodin et al. (2019a)Volodin et al. (2017a, b)Volodin et al. (2019b)Boucher et al. (2020)Boucher et al. (2018)Hajima et al. (2020b)Hajima et al.20192020aNeubauer et al.2019aTegen et al.2019Neubauer et al. (2019b)Mauritsen et al. (2019)Wieners et al.2019a2019bYukimoto et al. (2019a)Yukimoto et al.2019c2019bSeland et al. (2020)Seland et al. (2019b, a)Seland et al. (2020)Bentsen et al. (2019)Sellar et al. (2019)Tang et al. (2019b, a)Park et al. (2019)Park and Shin (2019)Lee et al. (2020)Lee and Liang (2020)Table 1CMIP6 models analyzed in this study. In the comments column, D stands for models including dynamic vegetation and N for models including nitrogen cycles. Models for which emission-driven simulations are also analyzed are marked in bold.
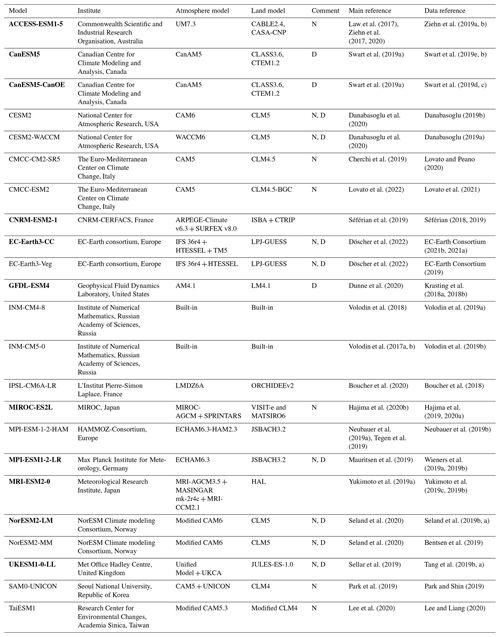
2.1 CMIP simulations
Model simulations from both CMIP Phase 6 (Eyring et al., 2016a) and Phase 5 (Taylor et al., 2012) are used, with Tables 1 and 2 listing model characteristics such as their atmosphere and land model components, in addition to their main references. A more comprehensive summary of the land model components of the CMIP models is given in Appendix A. Models were selected due to their availability on Earth System Grid Federation (ESGF) nodes for the considered variables (see the “Code and data availability” section).
This study focuses on historical simulations which aim to reproduce the observed climate since the pre-industrial times. They span 1850–2005 (CMIP5) and 1850–2014 (CMIP6). Both experiments with prescribed greenhouse gas concentrations (concentration driven; historical) and prescribed CO2 emissions (emission driven; esmHistorical and esm-hist for CMIP5 and CMIP6, respectively) are considered but evaluated separately and compared to each other. Models participating in the emission-driven simulations, marked in bold in the tables, use their interactive carbon cycle to determine the distribution of natural and anthropogenic carbon fluxes across the land, marine, and atmospheric reservoirs instead of relying on prescribed atmospheric CO2 concentrations (Friedlingstein et al., 2014).
Very few CMIP5 models had a coupled carbon–nitrogen cycle. While the BNU-ESM model included carbon–nitrogen interactions, they were turned off for the CMIP5 model simulations as the nitrogen cycle had not been fully evaluated (Ji et al., 2014). Therefore, a nitrogen cycle was included in 2 out of 18 CMIP5 models (CESM1-BGC and NorESM1-ME) which both use the CLM4 land model and in 15 out of 23 CMIP6 models spread over six different land models, with the Community Land Model (CLM) in different versions accounting for 8 CMIP6 models (v5: CESM2, CESM2-WACCM, NorESM2-LM, and NorESM2-MM; v4.5: CMCC-CM2-SR5 and CMCC-ESM2; v4: SAM0-UNICON and TaiESM1). The other land models in CMIP6 with a coupled nitrogen cycle are LPJ-GUESS (EC-Earth3-CC and EC-Earth3-Veg), JSBACH (MPI-ESM-1-2-HAM and MPI-ESM1-2-LR), CABLE+CASA-CNP (ACCESS-ESM1-5), JULES-ES (UKESM1-0-LL), and Visit-e (MIROC-ES2L). This shows a large bias towards the CLM land model in CMIP6 which needs to be considered while analyzing the multi-model mean (MMM).
To facilitate a direct comparison of CMIP5 and CMIP6 data in figures containing temporal means, only data up to 2005 representing the end of the CMIP5 historical simulations are considered. Unless stated otherwise, figures use mean data over the time period 1986–2005. Only one realization per model is used, as different ensemble members perform similarly to each other with respect to the carbon cycle (Gier et al., 2020), and using an ensemble mean would lead to an under representation of the internal variability present in individual ensemble members (Anav et al., 2013a). Multi-model means (MMMs) were computed separately for each CMIP phase and experiment combination, as well as an additional distinction between models with and without interactive nitrogen models, and are computed on the monthly gridded data for which models are regridded to a common 2°×2° grid. MMMs are not weighted according to the interdependence of the models and model components or according to their performance relative to observational products.
While we split models into groups only dependent on the presence of an interactive nitrogen cycle in this study, vegetation dynamics is another important process for ESM comparison. Models interactively simulating vegetation cover may simulate trees or grasses in the wrong areas compared to models using observational land cover maps, thus impacting variables with a strong relation to land cover, such as leaf area index (LAI) or gross primary productivity (GPP). While models with prescribed land cover may show better LAI in the present day, they cannot predict future changes in vegetation cover or their impact on regional climate and carbon processes. For reference, Tables 1 and 2 note models with dynamic vegetation with a D in the comment column.
2.2 Reference data
A large range of observations and reanalysis data sets have been used to assess model performance. These data sets are listed in Table 3, along with their main reference(s), their source, the variables used, and their temporal coverage. Both observational and reanalysis data sets will be referred to as “observations” from here on, in contrast with the results from the CMIP model simulations. The longest observational records are derived from reanalyses, while satellite observations only provide data from the late 20th century onward. Since most reference data sets do not come with observational uncertainty, a common approach is to use several reference data sets per variable where available, as noted in Seiler et al. (2022). This approach is also taken in this study.
Rödenbeck (2005)Chevallier et al. (2005, 2010)Chevallier (2013)Friedlingstein et al. (2022)Global Carbon Project (2021)Jung et al. (2019)Jung et al. (2011)Yuan et al. (2007)Liang et al. (2021)Zhu et al. (2013)Cao et al. (2023)Gibbs (2006)Wieder (2014)Hugelius et al. (2013)Table 3Reference data sets used in this study. Data sets in bold are the main reference data set, and those in italics are the alternate references for Figs. 15–17.
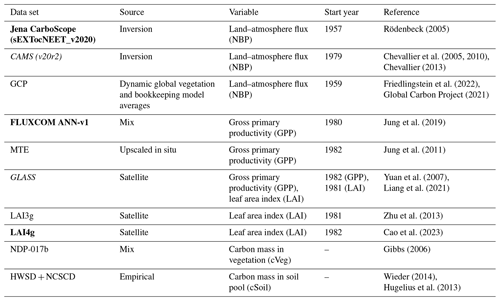
For the leaf area index (LAI), we use the LAI3g product (Zhu et al., 2013) that provides global monthly gridded data starting in the year 1981. It has been generated using an artificial neural network based on data from the Advanced Very High Resolution Radiometer (AVHRR) and the Moderate Resolution Imaging Spectroradiometer (MODIS). Furthermore, we also use the newly released GIMMS LAI4g data set (Cao et al., 2023), which is based on the same satellite data as LAI3g but employs a newer Normalized Differential Vegetation Index (NDVI) data set base which removes the effects of the satellite orbital drift and AVHRR sensor degradation which plagued many other LAI data sets. Furthermore, LAI4g uses a large number of high-quality Landsat LAI samples to increase the spatiotemporal consistency of the data set. Last, the Global Land Surface Satellite (GLASS; Liang et al., 2021) is a product suite with 12 products and from which we employ both the leaf area index (LAI) and gross primary productivity (GPP) products. Similar to LAI3g and LAI4g, GLASS is based on data obtained from AVHRR and MODIS. As newer GLASS data products only use MODIS and thus start from 2000, this paper uses a previous GLASS version (v4.0) which includes AVHRR data and thus starts in 1981 for LAI and 1982 for GPP, respectively. GLASS LAI uses general regression neural networks trained on preprocessed reflectance data of an entire year to estimate the 1-year LAI profile for each pixel. The LAI product is one of the variables used to estimate GLASS GPP with an eddy covariance light use efficiency model. Both GLASS products are available on a 0.05° grid with a frequency of 8 d.
Another GPP product, MTE (Jung et al., 2011), provides global monthly gridded data starting in 1982. It uses an upscaling of data from the FLUXNET eddy covariance tower network based on the model tree ensemble (MTE) approach. Similarly, the FLUXCOM product (Jung et al., 2019) is also based on an upscaling of FLUXNET site level observations but additionally incorporates a larger variety of machine learning methods and also includes remote sensing (from MODIS) and meteorological data. Here, we use a global monthly gridded version of FLUXCOM (starting in 1980) from the RS+METEO setup. Due to the assumption of an unchanging average CO2 level, both MTE and FLUXCOM data are known to have an unrealistic non-existent trend (0.01 PgC yr−2 globally) (Anav et al., 2015). Thus, trend analysis on GPP should exclude these data sets.
The main data set for the land–atmosphere carbon flux (net biome productivity (NBP)) is the Jena CarboScope (version sEXTocNEET_v2020) product (Rödenbeck, 2005), which provides global daily gridded data starting from the year 1957. This data set provides surface–atmosphere CO2 fluxes based on atmospheric measurements calculated from an atmospheric transport inversion. The inversion used here (NEE-T inversion) involves a regression of interannual net ecosystem exchange (NEE) anomalies against air temperature anomalies (T). In total, Jena CarboScope uses data from 156 atmospheric measurement sites distributed across the entire globe. The alternative data set for the land–atmosphere carbon flux is a further inversion product from the Copernicus Atmosphere Monitoring Service (CAMS; Chevallier et al., 2005, 2010; Chevallier, 2013). CAMS provides global gridded data on a monthly resolution starting in 1979 (other temporal resolutions are also available). The inversion product we use here (v20r2) is based on surface measurements from more than 100 sites. A third data set used for comparing the global annual mean NBP is the Global Carbon Project (GCP; Friedlingstein et al., 2022), which estimates the global carbon budget using several observations and models. It provides estimates for emissions from fossil fuel combustion and industrial processes, emissions from land use change, the atmospheric CO2 growth rate, ocean sink, land sink, cement carbonation sink, and the budget imbalance from combining all these terms. The land–atmosphere carbon flux for GCP has to be calculated by subtracting the land use change emissions from the residual land sink. The land sink was obtained from averaging the results from 17 dynamic global vegetation models (DGVMs) which reproduce the observed mean total land uptake of the 1990s and is given with an uncertainty of ± 0.5 PgC yr−1 on average. The land use change emissions are estimated from the average of three bookkeeping models with an uncertainty of ± 0.7 PgC yr−1, making it one of the only data sets with direct estimations for uncertainties.
For each of the remaining carbon cycle variables, only a single reference data set is taken into account. For the vegetation and soil carbon pools, the NDP-017b (Gibbs, 2006) and HWSD + NCSCD (Wieder, 2014; Hugelius et al., 2013) products are used, respectively. Both data sets provide global gridded annual data for the single year of 2000. NDP-017b uses an updated database that extends the methodology of Olson et al. (1985), who developed a global carbon stock map of above- and belowground biomass using 20 years of field investigations, consultations, and literature analysis and the more up-to-date land cover conditions of the Global Land Cover Database (GLC2000). The Harmonized World Soil Database (HWSD) uses large volumes of regional and national soil information to create an empirical data set that provides soil parameter estimates for topsoil (0–30 cm) and subsoil (30–100 cm). Similar to Varney et al. (2022), we combine the HWSD data set with the Northern Circumpolar Soil Carbon Database (NCSCD; Hugelius et al., 2013) to complement the HWSD data in the polar region. It uses data on soil order coverage to calculate soil organic carbon content and mass with 1778 pedon data. Wherever overlap between the two data sets occurs, the NCSCD data are chosen.
2.3 ESMValTool
The analysis in this paper was produced using the Earth System Model Evaluation Tool (ESMValTool) version 2 (Righi et al., 2020; Eyring et al., 2020; Lauer et al., 2020; Weigel et al., 2021; Schlund et al., 2023). ESMValTool is an open-source community diagnostics and performance metrics tool which has been developed to routinely evaluate ESMs contributing to CMIP and compare them with other ESMs, predecessor versions, as well as observations. Since its first release (Eyring et al., 2016b), ESMValTool has been updated for increased performance in its core functionality to deal with the increased data volume of CMIP6 and now features full traceability and reproducibility through provenance, as well as new and updated diagnostics and metrics, which can be applied to many models and variables. Available diagnostics cover a large range of scientific topics and are described in three papers. They include large-scale diagnostics for quasi-operational and comprehensive evaluation of ESMs (Eyring et al., 2020), diagnostics for extreme events, regional model and impact evaluation and analysis (Weigel et al., 2021), and diagnostics for emergent constraints and analysis of future projections (Lauer et al., 2020). A new suite of recipes has been developed to cover the work of this study, as well as some improvements on previous diagnostics for the carbon cycle available in ESMValTool. This facilitates the evaluation of the carbon cycle in future studies, including the analysis of upcoming CMIP7 simulations that can be easily compared to CMIP5 and CMIP6 to assess improvements.
General climate variables, such as temperature and precipitation have a large influence on the carbon cycle. It is therefore important to assess how well these variables are simulated by the ESMs. If they are well reproduced but carbon cycle variables are not, then it is likely due to a poor representation of processes specific to the carbon cycle, while a poor performance in the physical variables makes an attribution of the cause of poor performance in the carbon cycle variables more difficult. The CMIP5 and CMIP6 ensembles have been assessed compared to observations by Flato et al. (2013) and Eyring et al. (2021), respectively. A detailed analysis was also done by Bock et al. (2020), and references therein, who compare the surface temperature, pressure, precipitation, radiation, and clouds of CMIP3, CMIP5, and CMIP6 historical simulations for annual means. The CMIP6 models show better correlations for these variables than the CMIP5 models for all parameters, with smaller improvements for variables such as temperature which were already well represented in previous CMIP phases. However, the model spread is not significantly reduced but instead largely remains the same. Here, we expand the analysis to the carbon cycle. However, it should be mentioned that many carbon cycle processes are affected by physical variables on much smaller timescales, such as the timing of precipitation throughout the day or if surface temperatures falling below the freezing point at any time of the day may impact the growth of plants more than their monthly means suggest. This study uses monthly mean data, which does not resolve many of these important events and thus does not investigate the impact of physical variables further, as any analysis would still be lacking many possible impacts of sub-frequency effects. Future studies using higher-frequency data should put more emphasis also on the physical drivers of carbon processes, as well as the role of extreme events.
3.1 Leaf area index
The leaf area index (LAI) is the ratio of one-sided leaf area per unit of ground area (Anav et al., 2013a) as a measure of the canopy structure. Models use LAI to calculate the photosynthetic uptake of the total canopy, also known as gross primary productivity (GPP). While LAI is an important building block for the carbon cycle, it was also one of the weaknesses of the carbon cycle in the CMIP5 ensemble and tended to be overestimated (Anav et al., 2013a, b).
Carbon uptake by land follows a pronounced seasonal cycle, with CO2 removed from the atmosphere through plant photosynthesis and released back through plant and soil respiration. With LAI describing the canopy structure and more plants thriving in summer, it is strongly linked to the seasonal cycle of atmospheric CO2. The seasonal cycles for LAI for CMIP5 and CMIP6 MMMs for concentration- and emission-driven simulations are shown in Fig. 1 and split into models with (Ncycle) and without interactive nitrogen cycle (non-Ncycle), as well as different regional means, namely global, Northern Hemisphere (20–90° N; NH), Southern Hemisphere (20–90° S; SH), and tropics (20° N–20° S). From here on, concentration-driven simulations will be denoted by c, such as CMIP5c and CMIP6c, and emission-driven simulations by e (CMIP5e and CMIP6e). The BNU-ESM and MRI-ESM1 CMIP5 models were removed from the MMM due to featuring an unrealistically high mean LAI, almost doubling the LAI of the reference data and the other models in the SH and the tropics. A common mask is applied to all data sets, which includes all missing values in any data set to allow for direct comparison between the models and reference data sets. This increases the LAI compared to unmasked regional means, as missing values are more common in desert and mountainous regions with low LAI (Fig. 3). The chosen reference data sets LAI3g, LAI4g, and GLASS agree well across all regions, which was to be expected, as they are all based on the same raw satellite data from AVHRR and MODIS. Xiao et al. (2017) found that the GLASS product outperformed other products, which included LAI3g, when compared to LAI from high-resolution reference maps. As such, GLASS, which is also the reference data set with the largest coverage, will be considered the main reference data set for our analysis of LAI.
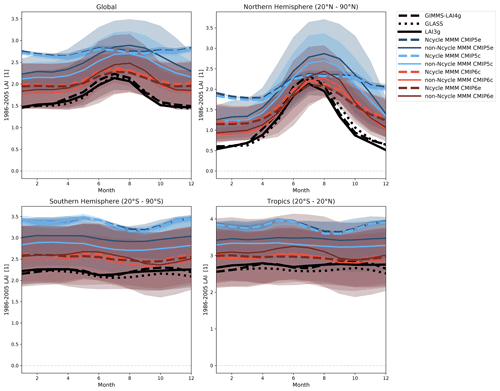
Figure 1Seasonal cycle of leaf area index (LAI) for a climatological mean of 1986–2005 for different regional averages, namely global, Northern Hemisphere (20–90° N), Southern Hemisphere (20–90° S), and tropics (20° S–20° N). The reference data sets (LAI3g, solid line; LAI4g, dashed line; GLASS, dotted line) are shown in black, while the MMMs for the different phase–experiment combinations are denoted by blue for CMIP5 and red for CMIP6, with darker colors for the emission-driven simulations (dark blue for CMIP5e; dark red for CMIP6e) and lighter colors for concentration-driven simulations (light blue for CMIP5c; light red for CMIP6c). MMMs derived from models with coupled nitrogen cycle (Ncycle) are dashed, while solid lines represent MMMs of models without a coupled nitrogen cycle (non-Ncycle). The shading represents the standard deviation of the MMMs, with vertical hatching for models without and horizontal hatching for models with coupled nitrogen cycle. For comparison with the reference data, which contain many missing values, a common mask was applied to all data sets, removing values where any data set is missing a value.
There is a strong seasonal cycle in the NH, dominated by high-latitude vegetation in Eurasia and North America. The NH seasonal cycle is the dominant contribution to the global mean due to the higher relative land fraction in the higher latitudes of the Global North compared to the Global South. All models overestimate LAI in all regions, but the CMIP6 models reproduce the reference data better than the CMIP5 models. According to Anav et al. (2013b), the overestimation in the mid-latitudes is likely partly due to a wet bias and its control on soil moisture, a saturation of satellite instrumentation, and missing parameterizations of disturbances. The annual mean precipitation wet bias is minimally reduced in CMIP6 (Bock et al., 2020), and new parameterizations such as nutrient limitations through interactive nitrogen cycle have been introduced in some models, leading to a reduced LAI in the CMIP6 MMMs compared to CMIP5. The seasonal cycle in the NH is reproduced, but while the CMIP5 non-Ncycle models reproduce the amplitude well with a positive offset of approximately 0.7 m2 m−2, the CMIP6 non-Ncycle models are better at reproducing the peak value. Both CMIP5 Ncycle models (CESM1-BGC and NorESM1-ME) use the CLM4 land model with known issues regarding LAI, such as underestimating LAI in dry regions due to elevated CO2 and overestimating LAI in moist regions (Lee et al., 2013), as well as an unrealistically strong nitrogen limitation (Wieder et al., 2019), hindering plant growth. This leads to these models showing a larger overestimation in LAI in both the Southern Hemisphere and tropic regions dominated by moist rainforests. Additionally, the seasonal cycle amplitude is strongly reduced in the NH, while the mean LAI is larger than for the reference data sets. Both CMIP phases show a weakness in simulating the end of the growing season, shown by the later decline in LAI in winter, which also leads to a smaller seasonal cycle amplitude, consistent with the findings of Park and Jeong (2021). The drawdown in autumn signifying the end of the growing season is smaller in Ncycle models compared to non-Ncycle models. The differences between the concentration- and emission-driven simulations are small, with models participating in both simulations having very similar results (individual models not shown). Larger differences occurring here and in later analysis between the concentration- and emission-driven simulations are likely due to the different subset of models in the historical simulations and not due to the experiment design.
Figure 2 shows the mean and trend of LAI averaged over 1986–2005 and depicts phase–experiment simulations with one type of marker each for a better overview. Exact numbers for all data sets are found in the Supplement (Tables S1–2). The errors given in the tables refer to the standard deviation of the mean as a measure for the interannual variability (IAV), while the standard error in the trend is the error in the linear regression calculating the trend. For the individual reference data, LAI3g and LAI4g agree well in mean and trend, while GLASS agrees with their mean but shows a significantly higher trend in all regions, leading to the large trend error bar. Xiao et al. (2017) analyzed the trend of several LAI products for different biome types for 1982–2011 and found GLASS to have significantly higher trends in savannahs and shrubs compared to LAI3g but lower trends in deciduous broadleaf forests and evergreen needleleaf forests, and even a negative trend for deciduous needleleaf forests, while grasses, cereal crops, and evergreen broadleaf forest trends are similar for GLASS and LAI3g. This results in larger trend differences in the SH (0.009 m2 m−2 yr−1) and tropics (0.010 m2 m−2 yr−1) than the NH (0.003 m2 m−2 yr−1). The MMM global means show a significant overestimation compared to the reference data, with the overestimation of the CMIP5 MMMs of 0.7 m2 m−2 reduced by half for CMIP6 MMMs, as previously seen in Fig. 1. This large improvement for the CMIP6 models is not related to the difference between Ncycle and non-Ncycle models, as their LAI MMM global means are comparable for CMIP6. Due to the large difference in trends in the reference data sets, the global mean trends of all CMIP MMMs lie within the range of the reference data. The model trends range between slightly negative and strong positive. Unlike the mean, the LAI trend does not show a strong difference between CMIP phases or any other grouping method we employed. The CMIP6 models only show a slightly smaller range in trend compared to the CMIP5 models, but more CMIP5 models have a lower trend compared to the reference data sets than CMIP6 models.
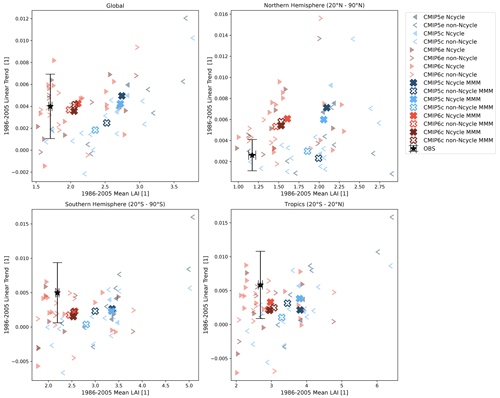
Figure 2Mean and trend of LAI computed over 1986–2005 for different regions, namely global, Northern Hemisphere (20–90° N), Southern Hemisphere (20–90° S), and tropics (20° S–20° N). The mean of the reference data sets (LAI3g, LAI4g, and GLASS) is denoted by a black star (“”), with error bars for the standard deviation. Models for phase–experiment combinations are shown with a single symbol each, with the blue smaller than (“<”) sign for CMIP5 and the red greater than (“>”) sign for CMIP6, while darker colors show emission-driven simulations, and lighter colors show concentration-driven simulations, as well as Ncycle models being denoted with a filled symbol. MMMs are depicted with crosses (“x”) in the color assigned to their phase–experiment combination.
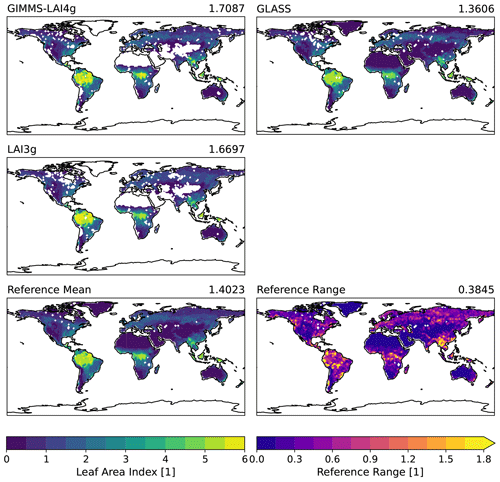
Figure 3Global maps of LAI averaged over 1986–2005 for all reference data sets, as well as the mean and range between lowest and highest values per grid cell of the reference data sets.
The other regions reflect these global MMM comparisons as well. CMIP6 means are closer to the reference data than CMIP5 in all regions, although still overestimating LAI, and agree well with each other no matter the experiment or Ncycle status. The only exception is the NH CMIP6c Ncycle MMM, which shows a larger mean than the other three CMIP6 groupings. This is due to the CMCC-ESM2 and CMCC-CM2-SR5 models, which show a much higher LAI in the NH compared to the reference data but fit well in the other regions. These two models use the CLM4.5 land model, which Li et al. (2022) found to have a far longer peak growing season and to overestimate LAI in boreal forests compared to MODIS reference data, consistent with our results. The CLM5 models (CESM2, CESM2-WACCM, NorESM2-LM, and NorESM2-MM) perform much better in the mean LAI than CLM4.5 in the NH but are still overestimating LAI compared to the reference data. The trends in the NH for the reference data are on the lower end of a large model range. Only the CMIP5 non-Ncycle MMMs agree with the reference data, while the other MMMs show a larger trend than the reference data but are comparable to each other. CMIP6 MMM LAI mean and trend values agree well with the reference data in the tropics and the SH, while the CMIP5 MMMs overestimate the mean by ≈ 0.7 m2 m−2 in the SH and ≈ 0.6 m2 m−2 in the tropics for non-Ncycle, as well as ≈ 1.2 m2 m−2 in the SH and ≈ 1.1 m2 m−2 in the tropics for Ncycle MMMs, but show a similar trend to LAI3g, LAI4g, and CMIP6 MMMs. The larger mean LAI in the CLM4 (CMIP5 Ncycle MMMs) can be traced back to the overestimation of LAI in the moist regions mentioned before. Some models show a significant negative trend in LAI in the SH and the tropics, resulting in a globally negative trend even with a positive trend in the NH.
Maps of the LAI reference data are found in Fig. 3 but without the common mask to see the different coverages. Coverage of the different reference data sets varies a lot due to different quality control criteria and algorithms, with most missing values found in desert or mountainous regions such as the Sahara and the Himalayas. Additionally, a mean of the reference data and the range of the reference data per grid cell is shown, along with the global mean of the values in the upper-right corner. GLASS has a larger coverage over desert and mountainous regions, which are regions with low plant coverage and thus low LAI, resulting in a lower global mean LAI of 1.36 m2 m−2 compared to 1.67 and 1.71 m2 m−2 from LAI3g and LAI4g, respectively. This underlines the importance of the common mask used for Figs. 1 and 2 to obtain comparable results. The different data sets show the same pattern of the LAI distribution, with the absolute values ranging between 1 and 6 m2 m−2, while the differences are below 2 m2 m−2, with the largest difference occurring in tropical rain forests and northern high latitudes – the regions with the largest absolute LAI values. For a grid cell bias comparison of the different model groupings (Fig. 4), a combined reference data set was computed as the mean of the other reference data sets. Due to the different coverages, some areas are only calculated from the GLASS data, while others are an average of all three data sets. The range of values per grid cell going into the combined data set is plotted in the lower right of Fig. 3. The largest difference occurs in the areas with larger LAI, i.e., the tropical rainforests and then boreal forests, with a global mean average range of 0.38 m2 m−2. For the MMM bias maps shown in Fig. 4, hatching is added where the MMMs agree with the reference mean within the MMM standard deviation. CMIP5 Ncycle MMMs show the issue of CLM4 in overestimating LAI in wet regions, with LAI in tropical rainforests almost doubling the reference value, while drier regions show a significant negative bias. While this results in a global mean bias of 0.66 to 0.67 m2 m−2, smaller than 0.89 m2 m−2 for CMIP5e non-Ncycle MMMs, it is still the worst-performing model grouping when considering a grid cell basis due to its extreme biases in both directions. The hatching showing the agreement can be ignored in this case, as only two models contributed to the MMM standard deviation. The CMIP5e non-Ncycle MMM shows a strong overestimation across the northern latitudes besides Greenland. This can be attributed to the GFDL-ESM2G and GFDL-ESM2M models, which are known to have established coniferous trees in areas which should contain tundra or cold deciduous trees, as in its vegetation spin-up only coniferous trees are allowed to grow in cold regions, but grasses or deciduous trees which would have a lower LAI are not (Anav et al., 2013b). While the GFDL models show this problem in both CMIP5c and CMIP5e, due to the larger number of MMMs contributing to the concentration-driven simulations, their effect is reduced. In CMIP6, GFDL-ESM4 still has a large positive LAI bias throughout these areas, but it is significantly reduced compared to its CMIP5 predecessor. The second prominent overestimation is around the tropical rainforests, where models like BNU-ESM and MRI-ESM1, and to a lesser extent, the GFDL models also extend the LAI hotspot to larger areas around it compared to the reference mean. In CMIP5c, HadGEM2-CC and HadGEM2-ES also show this overestimation. CMIP5c non-Ncycle MMM shows no special bias patterns, but instead a general overestimation in almost all areas with hatching is present throughout the globe. CMIP6 MMMs show a reduced mean bias of less than half the CMIP5 overestimation, with almost no pronounced patterns and a bias reduction in all areas, with the largest improvements found in the northern high latitudes. The largest bias is in Southeast Asia for CMIP6c Ncycle MMMs, which can be tracked to the CMCC-ESM2 and CMCC-CM2-SR5 models, and this makes the mean bias of the CMIP6c Ncycle MMM higher than that of CMIP6c non-Ncycle MMM. Otherwise, the bias pattern looks similar for CMIP6 Ncycle and non-Ncycle models.
While CMIP6 LAI has improved compared to CMIP5, especially a significantly reduced mean bias, a general overestimation of LAI remains, along with issues of correctly reproducing the length of the growing season in the Northern Hemisphere and a large model spread in mean and trend LAI. Neither the introduction of an interactive nitrogen cycle nor the comparison between emission- and concentration-driven simulations shows large differences in the overall quality of the CMIP6 simulations for LAI.
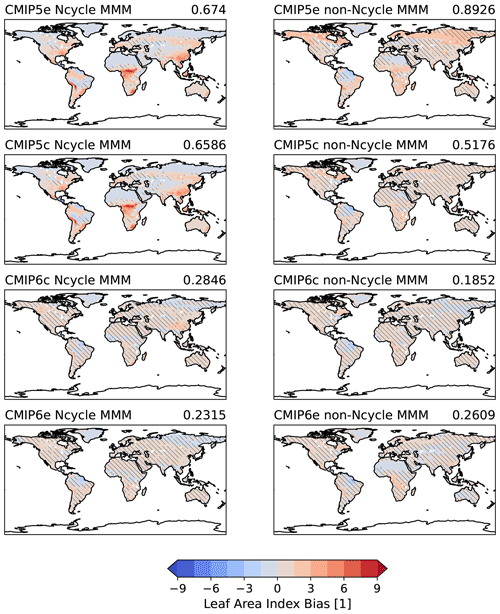
Figure 4Global maps of LAI bias for 1986–2005 with respect to the reference data set mean shown in Fig. 3. The panels show the MMMs of the models with (left) and without (right) coupled nitrogen cycle for the different phase-experiment combinations. The hatching represents the areas where the MMM of the models and reference mean agree within the MMM standard deviation.
3.2 Gross primary productivity
Gross primary productivity (GPP) represents the CO2 uptake on land due to photosynthesis. This was one of the biggest weaknesses of the CMIP5 ensemble, with most models overestimating photosynthesis, as well as leaf area index (Anav et al., 2013a). The seasonal cycle of GPP (Fig. 5) shows good agreement between the GLASS and MTE reference data, while the FLUXCOM data show a lower GPP in all regions, as well as a shorter growing season in the Northern Hemisphere. All models reproduce a similar shape of the NH seasonal cycle to the GLASS and MTE data in both model generations and experiments. As found in Anav et al. (2013a), the CMIP5 non-Ncycle models overestimate GPP in all regions, while the CMIP5 Ncycle models strongly underestimate the peak of the seasonal cycle in the NH. CMIP6 models perform better than CMIP5, but while the CMIP6 non-Ncycle models still overestimate the GPP peak in summer similarly to CMIP5 for the NH, the Ncycle models show a very good agreement for both CMIP6c and CMIP6e. Nitrogen limitation is stronger in northern latitudes through boreal forests and tundra (Du et al., 2020) compared to tropical and subtropical forests, which are more limited by phosphorus. However, from the CMIP models used in this study, only ACCESS-ESM1-5 includes an interactive phosphorus cycle. It therefore makes sense that Ncycle models show a decreased GPP compared to non-Ncycle models in the NH and are closer to the reference data. The model spread remains large in CMIP6, despite being smaller for Ncycle models, which is denoted by horizontal hatching. Following LAI, there is no strong and discernible seasonal cycle in the SH or the tropics, but the CMIP6 models are closer to the mean GPP than the CMIP5 models, with lower values for Ncycle models.
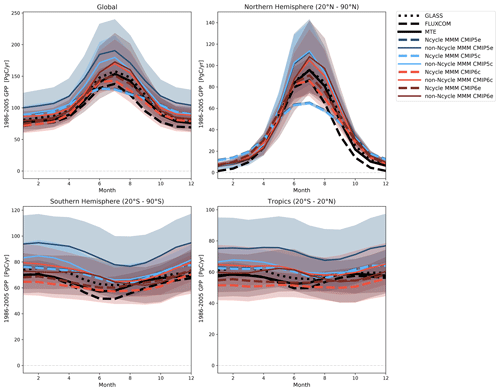
Figure 5As in Fig. 1 but for gross primary production using GLASS, FLUXCOM, and MTE reference data. Additionally, the regional GPP is calculated as the area-weighted sum instead of the mean of the grid cells used for LAI.
The temporal mean and linear trend of the spatial sums for GPP during the time period 1986–2005 is shown in Fig. 6. MTE and FLUXCOM data are known to have an unrealistic non-existent trend (0.01 PgC yr−2 globally) due to the assumption of an unchanging average CO2 level (Anav et al., 2015) in these data sets. As such, the model trend should not be compared to the trend of these two reference data sets, and we have omitted these data sets from the calculation of the reference trend. The mean and trend for GPP of all regions of these data sets, along with all other numerical values from the plot, can be found in Tables S3–S4. The trend of GLASS is closely linked to the high trend of LAI GLASS, which is one of the main influences on GPP. The reference data sets agree well with respect to the mean GPP, with the largest difference being a lower mean for FLUXCOM in the NH. The models show a large range, with MRI-ESM1 CMIP5e as an outlier, which shows an even larger mean GPP in all regions. The CMIP5 models are on the higher side of this range, with the Ncycle CMIP5 models much lower due to their underestimation in the NH as seen in Fig. 5. The CMIP6 Ncycle MMMs agree very well with the reference data, while the CMIP6 non-Ncycle MMMs show a larger mean GPP. The global trend of GLASS is positive, which is well matched by the non-Ncycle CMIP6c MMM with the other MMMs showing a smaller trend. In the NH, more CMIP5 models overestimate the mean GPP than CMIP6 models. The MMMs span a large range, with the Ncycle MMMs showing a lower mean GPP than the non-Ncycle MMMs, which are overestimating GPP compared to the reference data. While the trends of the models are centered around the GLASS trend, there is a large model range with outliers for MRI-ESM2-0 in both its CMIP6c and CMIP6e runs. The CMIP6 non-Ncycle MMM shows a higher trend than the other MMMs due to the MRI-ESM2-0 outliers. In the SH mean GPP, the CMIP6 MMMs match the reference data well, with lower values for Ncycle than for non-Ncycle MMMs. The CMIP5 MMMs are slightly above these, with the non-Ncycle CMIP5e having a much larger value due to the outliers of MRI-ESM1 and FIO-ESM, which both have values well above 100 PgC yr−1. The MMMs underestimate the trend compared to the reference data. The distribution in the tropics is very similar to the SH. The reference data trend is underestimated by the MMMs. In summary, the Ncycle MMM shows a better performance than the non-Ncycle MMM in the NH, while it shows a slight underestimation in the tropics and a similar performance in the SH. The model spread over the trend in GPP stays similar throughout the model generations, with the mean trend being largely consistent with the GLASS reference data set in all regions but underestimated everywhere but in the NH.
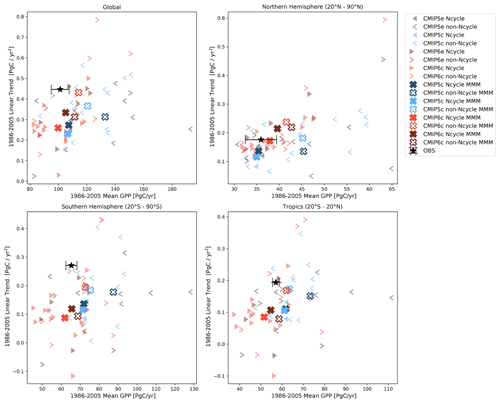
Figure 6As in Fig. 2 but for gross primary production using GLASS, FLUXCOM, and MTE reference data.
As for LAI, the coverage of the GLASS data is larger than for the other reference data, with missing values for FLUXCOM and MTE mainly found over the Sahara and the Himalayas (Fig. 7). GLASS shows a larger GPP in the tropical rainforests and boreal forests, explaining the larger mean GPP seen before. The reference mean data set has hardly any missing values left, and the largest differences in the data sets are at places with the highest GPP, with discrepancies in the areas bordering the hotspots of the rainforests and boreal forests. Even though the GPP bias maps (Fig. 8) for Ncycle CMIP6e MMMs have a global mean bias almost 2 orders of magnitude smaller than the CMIP6e non-Ncycle MMMs, they show the same pattern of overestimation in wet regions and underestimation in dry regions found for LAI (Fig. 4), underlining the strong influence of LAI on GPP. The CMIP5 non-Ncycle MMMs also shows similar patterns to the LAI bias maps, but the overestimation in the areas around the tropical rainforests is strongly reinforced, while the previous strong overestimation of LAI in the northern high latitudes for the non-Ncycle CMIP5e MMM is reduced. The CMIP5c non-Ncycle MMM additionally shows a strong underestimation at the northeastern coast of South America. The global mean bias for CMIP5 non-Ncycle MMMs lies at 0.36 kgC m−2 yr−1 for CMIP5e and is reduced by half for CMIP5c. This bias is further reduced to approximately 0.1 kgC m−2 yr−1 for CMIP6 non-Ncycle MMMs, which show similar bias patterns to CMIP5 non-Ncycle MMMs, but there is an overall reduction in the bias patterns, with a larger reduction in the savannah regions of Africa. The global mean bias for the CMIP6 Ncycle MMMs is further reduced to 0.001 kgC m−2 yr−1 for CMIP6e and a negative bias of −0.04 kgC m−2 yr−1 for CMIP6c. Both show a reduction in the Northern Hemisphere bias of the open shrublands, turning some into a negative bias, as well as southwest Africa, while the slight overestimation in North America remains, as well as the underestimation at the northeastern part of South America. This is summarized in Fig. 9, which shows the zonal sums of the reference data and the MMMs. Unlike the seasonal cycle and scatterplots shown before, a common mask is not applied here, but instead values are masked out if more than 15 % of the pixels over land in a latitude band are missing for a given data set. The large overestimation of non-Ncycle CMIP5e can be seen, which is reduced in non-Ncycle CMIP5c, with both cycles showing a peak slightly north of the Equator which is not seen in the reference data and which is due to the overestimation of the shrublands south of the Sahara. The CMIP6 non-Ncycle MMMs show a much better approximation across all latitudes, with a slight reduction in the bias in the NH, but they still show a significant overestimation in the tropics. This is remedied in the CMIP6 Ncycle models, which show a very good agreement with the reference data across all latitudes and now with slight underestimations at high latitudes.
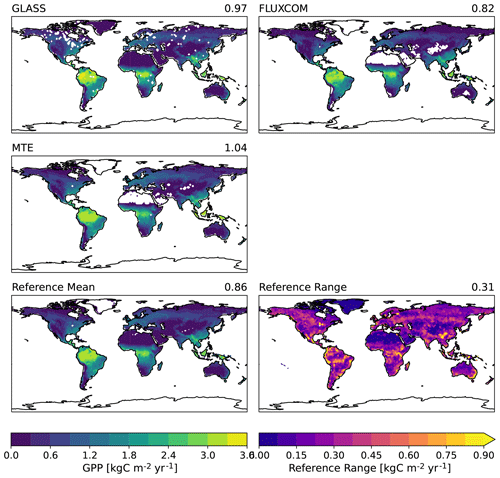
Figure 7Global maps of GPP averaged over 1986–2005 for all reference data sets (GLASS, FLUXCOM, and MTE), as well as the mean and range between lowest and highest values per grid cell of the reference data sets. The number in the top right denotes the global GPP flux.
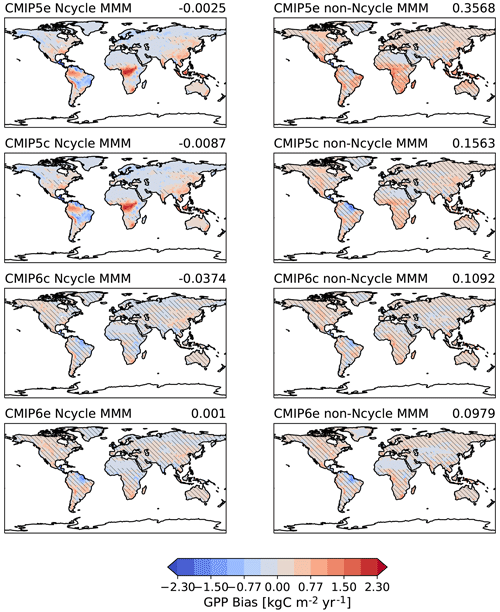
Figure 8Global maps of GPP bias for 1986–2005 with respect to the reference data set mean shown in Fig. 7. The panels show the MMMs of the models with (left) and without (right) coupled nitrogen cycle for the different phase–experiment combinations. The hatching represents the areas where the MMM of the models and reference mean agree within the MMM standard deviation, while the number in the top right denotes the global mean bias.
3.3 Land–atmosphere flux
The net carbon flux from the atmosphere into the land (net biome productivity – NBP) characterizes the balance between carbon uptake due to photosynthesis and carbon release by respiration, as well as other processes like fires and de- and afforestation. Positive values of NBP denote carbon uptake by land. The CMIP6 EC-Earth models (EC-Earth3-CC and EC-Earth3-Veg) are excluded from the MMM for NBP because they show a very strong land source in December due to the annual update of land use transitions (Döscher et al., 2022), thus influencing the seasonal cycle. For the consistency of models contributing to the MMM, these models are not only excluded from the MMM calculation of the seasonal cycle but all NBP MMMs. However, their individual data points are included in the scatterplot. MIROC-ESM and MIROC-ESM-CHEM are also removed from the MMMs due to rapidly changing fluxes both temporally and spatially. This occurs due to the stochastic dynamic vegetation model (Watanabe et al., 2011) and shows stronger effects in the tropics when combined with the land use change emission module and the high climate sensitivity. While the influences on the regional means or climatologies are smaller than for EC-Earth3, they can be seen in mean map plots very well.
The global seasonal cycle for NBP (Fig. 10) is dominated by the Northern Hemisphere, with almost no discernible cycle in the Southern Hemisphere or the tropics. There is generally a good agreement between the two inversions, but the CAMS inversions show a larger seasonal cycle in the SH and tropics of approximately 6 PgC yr−1, where CarboScope shows no clear seasonal cycle. In the NH, and due to its large contribution to the total also globally, CAMS has a higher NBP at the start of the year and NBP to a lesser degree at the end of the year, where CarboScope shows a larger negative NBP and thus a carbon sink. The models agree with the carbon sink of CarboScope in these months but have a weaker carbon sink (higher NBP) in NH autumn. The CMIP5 Ncycle MMMs have a smaller seasonal cycle amplitude compared to any of the other MMMs and the reference data, which are carried over from GPP. The other MMMs reproduce the seasonal cycle well, while the non-Ncycle CMIP6e MMM is shifted late by a month, showing possible issues with the start and end of the growing season. In the SH and tropics, where CarboScope found no significant cycle and CAMS had a slightly larger one in the tropics, the Ncycle models follow the shape and timing of the CAMS data, while the non-Ncycle models have a seasonal cycle shifted to 2 months earlier. There is no significant difference between CMIP5 and CMIP6 or between the c and e experiments in the MMM.
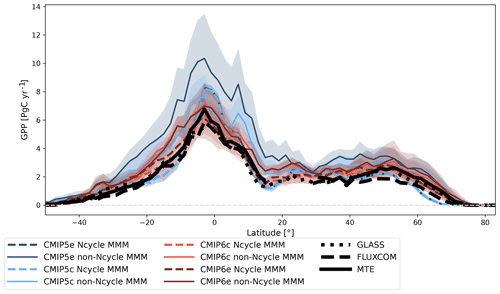
Figure 9Area-weighted zonal sums of gross primary production and the reference data sets of GLASS, FLUXCOM, and MTE. No common masking is applied, but latitudes were set to missing if more than 15 % of the land grid cells contained missing data. The hatching depicts the MMM standard deviation, with a horizontal hatching for models with an interactive nitrogen cycle and vertical hatching for models without an interactive nitrogen cycle.
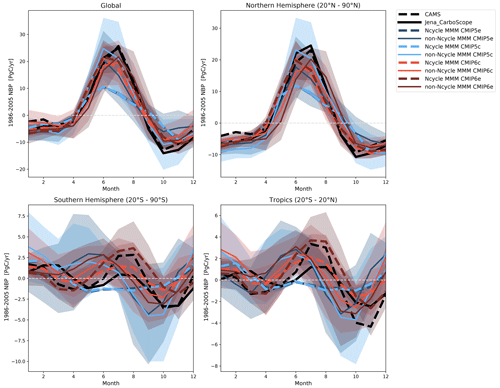
Figure 10As in Fig. 1 but for the land–atmosphere carbon flux and the reference data sets of CAMS and CarboScope.
The temporal mean and trend of spatially summed NBP are shown in Fig. 11, with specific values given in Tables S5 and S6. CAMS shows a larger mean NBP compared to CarboScope in all regions but the tropics, which is consistent with the NBP averages from Seiler et al. (2022), using this data set, who found its NBP to be larger than comparable data sets and model results. GCP as a global average is only available as a reference data set for the global panel. The models show a far larger range for the global mean NBP than the reference data, with outliers for the INM-CM CMIP6c models showing a far larger mean NBP than the other models. The Ncycle CMIP5 means have a negative mean NBP, while the other MMMs show a better agreement with the reference data. The Ncycle MMMs have significantly smaller mean NBPs than the non-Ncycle MMMs. The relatively good overall agreement of the models' global mean NBP with the reference data does not hold for the different regions. Most models and all MMMs simulate a lower carbon sink in the Northern Hemisphere when compared to the inversions, with Ncycle models generally showing a smaller mean NBP but no large discernible differences between the different groupings. Conversely, while the inversions estimate both the Southern Hemisphere and the tropics to be a slight carbon source due to deforestation, the MMMs, with the exception of the CMIP5 Ncycle, show a carbon uptake by land in these regions. The large values for the non-Ncycle CMIP6c MMM are again due to the overestimation of the INM-CM4-8 and INM-CM5-0 models, but as their mean NBP in the NH is not a large outlier, we did not remove these from the MMM. The underestimation in the NH combined with the overestimation in the SH and tropics leads to the good global agreement of the total carbon sink. This is in agreement with the findings from IPCC AR6 (Eyring et al., 2021; Canadell et al., 2021). The inclusion of a nitrogen cycle and therefore the inclusion of nitrogen limitations on CO2 fertilization was expected to address this discrepancy of the distribution of the carbon sinks (Canadell et al., 2021), but the data do not support this, as the Ncycle MMMs do not show a different performance to the non-Ncycle MMMs in CMIP6. While the models show a large range of trends, the MMMs agree well with the reference data, and this continues in the other regions as well. While N limitation is not expected to be substantial for the present day, it represents a major limitation on future land–carbon uptake (Zaehle et al., 2015), and thus, its inclusion a major advance in being able to robustly simulate future carbon balance of the terrestrial carbon cycle.
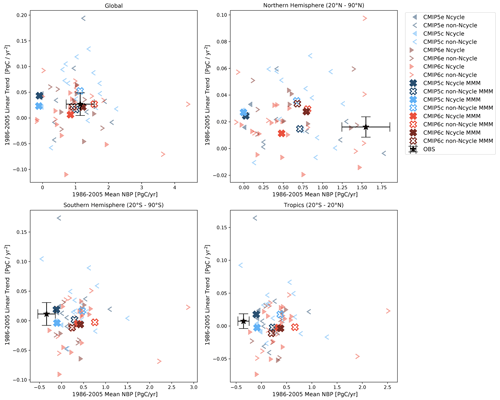
Figure 11As in Fig. 2 but for the land–atmosphere carbon flux and the reference data sets CAMS and CarboScope. The GCP data are a globally averaged time series and thus only appear in the global plot.
As seen before, the reference data sets show different means, trends, and slightly different seasonal cycles in the different regions. For a more detailed look, Fig. 12 shows maps of the reference data. In this case, the hatched area is the area where the data sets agree on the sign or within a margin of half the bin size of the contour plot. While in large parts of the globe the data sets agree in sign, there are significant differences. In North America, CAMS shows a much larger carbon sink than CarboScope, which instead shows some carbon sources along the west coast and throughout South America. In CAMS, South America is split into a much stronger carbon source in the Amazonian Rainforest and a carbon sink in the southern part. The data sets also disagree in Europe, which is a carbon source according to CAMS but a sink in CarboScope. The literature found Europe to be a carbon sink for the first part of the 21st century (Ciais et al., 2013; Reuter et al., 2014), using different reference data sets. This would support the CarboScope data set, but due to the different time frames considered, it is not definitive. CAMS also sees a carbon sink in tropical Africa, where CarboScope has a slight carbon source. In Southeast Asia, CarboScope has a large carbon sink, where CAMS shows a neutral carbon flux. The area of most agreement is in the NH as a large carbon sink, where there is no deforestation and a bit of afforestation. The Amazon region was a large carbon sink, which is becoming a source due to deforestation (Gatti et al., 2021). CAMS sees the Amazon as a strong carbon source, while CarboScope shows a smaller source with a sink in the northwestern region. Keenan and Williams (2018) found inverse models to show South America as a carbon source and a carbon sink, and it is thus a hotly debated area. Kou-Giesbrecht et al. (2023) attribute the weak agreement between CarboScope and CAMS to differences in the inversion models and atmospheric CO2 measurements used, with larger differences at latitudes with smaller land areas. Due to the difference between the observational data sets, maps depicting the bias with respect to the reference mean shown for the other variables so far have been omitted. Instead, the area-weighted zonal sums are plotted in Fig. 13 for a comparison of the MMMs with both reference data sets. The reference data sets disagree for almost all latitudes, thus making the model comparison to the reference data in these regions not very meaningful. The area where the reference data is in most agreement is in the northern high latitudes (50–80° N), where both of them show a strong carbon sink, about double that shown in both CMIP5 and CMIP6 models. While the issues in CLM4 and thus the CMIP5 Ncycle models are clearly visible (similarly to LAI and GPP), the other MMMs show similar NBP in all latitudes.
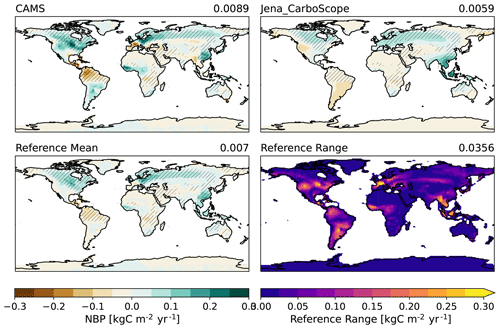
Figure 12Similar to Fig. 7 but for the land–atmosphere carbon flux. Additionally, the forward-slash hatching symbolizes areas where the reference data sets agree on the sign or where the difference is smaller than the size of one bin of the contour plot.
3.4 Carbon stocks
Another large uncertainty in CMIP5 was the amount of carbon stored in soil and vegetation. This leads to large uncertainties in land use change emissions, which are important for quantifying cumulative emissions, as well as climate mitigation strategies (Friedlingstein et al., 2023). Varney et al. (2024) investigated the carbon–climate feedbacks of soil and vegetation carbon and found soil carbon to be the dominant response of the land surface, highlighting the need to reduce the uncertainty in carbon storage to better quantify future changes in the climate system. Figure 14 shows a scatterplot of global vegetation carbon against the combined soil and litter carbon. Note that some models (CanESM5-CanOE CMIP6c, GFDL-ESM4 CMIP6e, INM-CM4-8 CMIP6c, INM-CM5-0 CMIP6c, FIO-ESM CMIP5e, CanESM2 CMIP5c, and inmcm4 CMIP5c) did not have data on the ESGF nodes for soil or vegetation carbon and are thus missing from the carbon stock analysis. BNU-ESM CMIP5c and CMIP5e show a far larger vegetation carbon than the other models and are thus removed in the calculation of the mean. Additionally, CLM5 and thus CESM2, CESM2-WACCM, NorESM2-LM, and NorESM2-MM include a full vertical soil profile. For these models, the cSoilAbove1m variable is used for better comparison with the other models, as done in Varney et al. (2022).
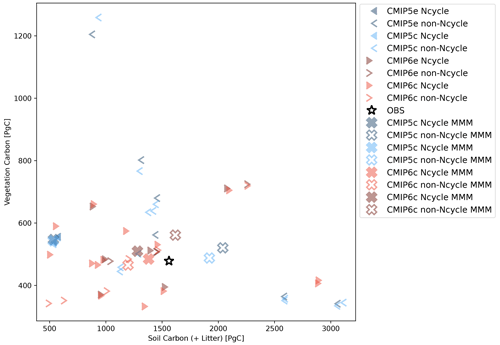
Figure 14Scatterplot of global mean vegetation and soil carbon over 1986–2005 for models, with observations from NDP (vegetation carbon) and HWSD + NCSCD (soil carbon). As in Fig. 2, filled symbols denote models with the nitrogen cycle.
The large spread in the global carbon stocks still remains in CMIP6, as shown in Fig. 14 with values for each data set listed in Table S7. In CMIP5, vegetation carbon was spread between 335 PgC (MPI-ESM-LR CMIP5c) and 802 PgC (GFDL-ESM2M CMIP5e), with the outlier of BNU-ESM even reaching values above 1200 PgC. The spread has only marginally been reduced in CMIP6 from a range of 333 PgC (EC-Earth3-Veg CMIP6c) to 724 PgC (CNRM-ESM2-1 CMIP6e). The reference data are at a value of 478 PgC, in the lower range of the models, with the MMMs ranging between 465 PgC (non-Ncycle CMIP6c) and 547 (Ncycle CMIP5e). The spread in soil carbon is even larger, with a CMIP5 range from 513 PgC (CESM1-BGC CMIP5c) up to 3092 PgC (MPI-ESM-MR CMIP5c). The overestimation by MPI-ESM is due to its decomposition parameterization depending on soil moisture and showing maxima in continental dry lands. In CMIP6 MPI-ESM1-2, the soil carbon model was changed to YASSO, which simulates more plausible soil carbon content (Mauritsen et al., 2019). The spread in soil carbon was not significantly reduced in CMIP6 with a range from 514 PgC (GFDL-ESM4 CMIP6c) to 2913 PgC (CMCC-ESM2 CMIP6c), with the reference value for HWSD + NCSCD at 1561 PgC. The CMIP5 Ncycle models have a soil carbon on the lower end of the range, consistent with the CMIP6 models TaiESM1 and SAM0-UNICON which also use CLM4. The CMIP5 Ncycle MMMs are on the very low end of the range, with 532 and 534 PgC for CMIP5 and CMIP5e, respectively, while the other MMMs are closer to the reference data and range between 1197 PgC for non-Ncycle CMIP6c and 2040 PgC for non-Ncycle CMIP5e. While the CMIP6 Ncycle MMMs are closer to the reference data, no significant improvement due to the inclusion of the interactive nitrogen cycle can be seen when considering the whole spread of the models. This is consistent with Wang et al. (2022), who found that changing models from C to CN coupling often result in lowered ecosystem storage but due to different parameterizations simulate similar carbon pools. Varney et al. (2022) suggest that much of the uncertainty in carbon stocks is due to the simulation of below-ground processes; this is backed up by the differences in soil carbon being much greater than in GPP and thus implicating differences in simulated residence times (Carvalhais et al., 2014; Todd-Brown et al., 2014). For a more in-depth discussion, we would like to refer to dedicated studies, such as Varney et al. (2022) and Wei et al. (2022). Furthermore, Varney et al. (2023) found that while the CMIP6 future soil carbon projections have a lower model spread compared to CMIP5, the structure of soil carbon models within CMIP6 ESMs has likely contributed towards this reduction.
3.5 Overall model performance
Here we assess the overall performance of CMIP5 and CMIP6 models with respect to carbon cycle variables. Figures 15 and 16 show a portrait plot similar to Gleckler et al. (2008) for NBP, GPP, and LAI for all four considered regions (global, Northern Hemisphere, Southern Hemisphere, and the tropics), with the normalized relative root mean square difference in the model simulations to reference data sets. Most models have similar scores when compared to the different observations for GPP and LAI, showing that the inter-model spread in CMIP6 is larger than the observational uncertainty in these variables. For NBP, however, models can have different scores to the considered reference data, which is due to the difference in the reference data found in the previous sections. CMIP6 models on average perform much better than CMIP5 models, with models that had a predecessor in CMIP5 improving on their CMIP5 performance in almost all variables, such as GFDL and IPSL in all variables and CESM and NorESM in LAI, with the exception of CanESM, which shows a reduced performance for NBP in CMIP6. Large improvements can be found in all variables going from CMIP5 to CMIP6, especially in LAI, with the exception of the models using the older CLM4 land component (SAM0-UNICON and TaiESM1) in CMIP6 and GPP, which were previously identified as weaknesses in CMIP5. Only the MRI-ESM2-0 model shows a bad performance in both these variables. As mentioned before, dynamic vegetation in models plays a large role in their ability to simulate variables directly related to it, with models interactively simulating vegetation cover (labeled D in Tables 1 and 2), showing a below-average score for LAI and GPP root mean square difference (RMSD).
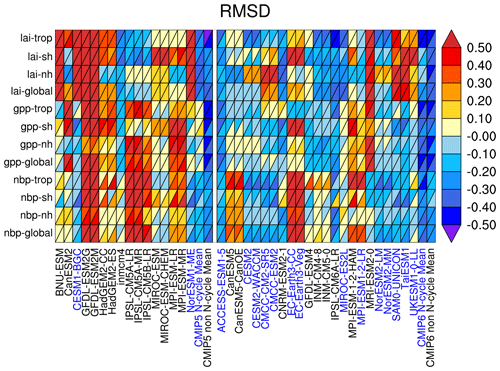
Figure 15Normalized relative space–time root mean square difference (RMSD) of the climatological seasonal cycle with respect to reference observations for CMIP5 (left) and CMIP6 (right) concentration-driven simulations compared to reference data sets. The normalization is done relative to the ensemble median of both CMIP5 and CMIP6 models, with positive values (red) denoting a higher RMSD and thus worse performance, while negative values (blue) denote a lower RMSD than the ensemble median and thus a better performance. The considered time periods depend on the start of the reference data (see Table 3) and end in 2005 to accommodate the end of the CMIP5 data. When using two observational references, a diagonal split is introduced, with the default reference data set being shown on the lower right, while the alternate data set is used for the top-left triangle. The default and alternate reference data sets are marked in Table 3 and are as follows: LAI with LAI4g (main) and GLASS (alt), GPP with FLUXCOM (main) and GLASS(alt), and NBP with CarboScope (main) and CAMS (alt). Models with a nitrogen cycle are marked with blue labels. MMMs for both Ncycle and non-Ncycle models were added, with the models which were excluded from MMMs due to various issues, as stated in the previous sections, also removed from the MMM here. Note that the MMMs were calculated on the climatologies prior to the calculation of the RMSDs, so over- and underestimations can cancel each other out. This is the standard for the performance metric plot implemented in ESMValTool and kept for consistency.
Models which were remarked upon in the previous sections as having good or bad agreement with the observations in specific areas, such as the NBP problems in December for EC-Earth3 have RMSDs that reflect these statements, making this metric a well-suited measure for overall performance. Most models have similar RMSDs in the different regions, with the global value reflecting a mean of the different regions. There does not seem to be a qualitative difference between Ncycle and non-Ncycle models as a whole, but the MMMs perform better than any individual model. The better performance of the MMMs is mathematically expected as long as the assumption that both observations and model simulations draw from the same distribution holds true (Christiansen, 2018). The global NBP, LAI, and GPP are also found in Fig. 42 of Chap. 3 of the IPCC AR6 (Eyring et al., 2021), which showed not only carbon cycle variables but also other land, ocean and atmosphere variables averaged over 1980–1999 for comparison across models from CMIP3 to CMIP6. Their results are compared to reference data from JMA-TRANSCOM for NBP, LAI3g for LAI, and MTE and FLUXCOM for GPP. As, other than NBP, these reference sets are the same as the ones considered in this paper, the results are also the same. For NBP, despite the different data set, the performance of the models is very similar to the one found for CAMS, our alternative data set. The ILAMB benchmark used in Chapter 5 of the IPCC AR6 (Canadell et al., 2021) also comes to the conclusion of the model having improved from CMIP5 to CMIP6. No qualitative difference can be found between models that have both emission-driven and concentration-driven simulations compared to models with only concentration-driven simulations, and models with both simulations have similar RMSDs in both. This indicates that carbon exchanges are well simulated in these models as the freely evolving fluxes are comparable to results with prescribed atmospheric concentrations.
Centered pattern correlations for these variables and regions are shown in Fig. 17, with a score of 1 meaning the perfect similarity of a model to the reference data, while a value of 0 signifies no relationship. For GPP and LAI, the reference data sets show a very good similarity of above 0.9, while for NBP the differences in the references highlighted in Fig. 12 are highlighted through a small correlation of up to 0.3, with a high anti-correlation in the Southern Hemisphere. Due to this, the precise value of the correlation coefficient between models and reference data set is not a good measure, but it can be seen that the models show a large spread. For GPP, the CMIP6 performance in the tropics is vastly improved, with even higher correlation values for Ncycle models. Other than in the NH, the CMIP5 models show a large spread in correlation values, which has reduced for CMIP6. The correlation distribution for LAI is similar to GPP, with the highest correlation values found in the tropics and globally, but the difference between Ncycle and non-Ncycle models is not as prominent. These overall performance plots underline the specific conclusions from the separate sections above.
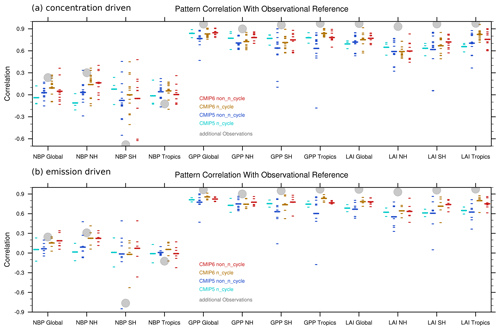
Figure 17Centered pattern correlations between models and reference data sets for annual mean climatology for concentration-driven (a) and emission-driven (b) CMIP5 and CMIP6 models split into Ncycle and non-Ncycle models. A score of 1 denotes the perfect similarity of a model to the reference data, while a value of 0 signifies no relationship. The longer lines denote the MMM, while the grey circle shows the similarity of the alternate data set to the main reference data set. Main and alternate observations are the same as in Fig. 15.
To be able to have confidence in future model projections of climate change, Earth system models first need to show the ability to simulate observed climatologies and trends of the carbon cycle in the present-day climate. In the Coupled Model Intercomparison Project Phase 5 (CMIP5; Taylor et al., 2012), several weaknesses of the simulated carbon cycle were found, such as a general overestimation of photosynthesis and a wide range of values for carbon stocks, which became one of the main areas of focus for improvement for some model groups (Delire et al., 2020). In this study, we have analyzed the land carbon cycle of models participating in the Coupled Model Intercomparison Project Phase 6 (CMIP6; Eyring et al., 2016a) to investigate whether these weaknesses were improved in the newer model generation, with a special focus on differences arising due to inclusion of an interactive terrestrial nitrogen cycle in some of the CMIP6 models. Concentration- and emission-driven simulations from CMIP5 and CMIP6 models were compared to reference data sets, with 2 out of 18 CMIP5 models and 15 out of 23 CMIP6 models including carbon–nitrogen interactions. We assessed the means, trends, and seasonal cycles of the leaf area index (LAI), the gross primary productivity (GPP), and the land–atmosphere carbon flux (NBP). We furthermore looked at land carbon stocks to see if the large range of values simulated in CMIP5 was reduced in CMIP6.
In general, CMIP6 models show a better performance across all assessed land carbon cycle variables to differing degrees, and no significant differences between the concentration-driven and emission-driven simulation were found in the considered variables that cannot be explained by the number of different models. While there is a bias towards the CLM land component in the CMIP6 models, the different versions (4, 4.5, and 5) do not perform the same, and thus these versions can be seen as independent components for the multi-model mean.
The leaf area index was a weakness of the CMIP5 simulation, as its seasonal cycle was not well captured, and its absolute value was generally overestimated. While the peak of the climatological seasonal cycle of LAI is much better reproduced in CMIP6, the amplitude of the seasonal cycle is weaker in CMIP6 compared to observations due to a weaker drawdown in winter. Thus, LAI should remain an area of focus for future model development. Mean LAI is much better reproduced in CMIP6, while the range of trends in the observations is large enough to cover most models for both CMIP5 and CMIP6. It should be noted that due to correlations between parameters, there are often tradeoffs for the better reproduction of one variable. In CLM5, such a tradeoff had to be weighed between biases for GPP and LAI against high plant functional type (PFT) survivability rates (Lawrence et al., 2019). Therefore, looking at one variable separately instead of the whole model performance can lead to wrong conclusions about the model's ability of reproducing the carbon cycle, depending on which choices were made in the tuning. Similarly, models interactively simulating vegetation cover perform worse in the evaluation of present-day LAI compared to models using observationally derived land cover maps due to simulating trees and grasses in the wrong areas. However, paleo-observations show that large changes in climate, such as during the Paleocene–Eocene Thermal Maximum, have a significant impact on vegetation and its global distribution (e.g., McInerney and Wing, 2011). Therefore, only models with dynamic instead of prescribed vegetation can self-consistently account for future changes in vegetation and the impact of these changes on climate and carbon processes in future projections.
One of the largest improvements due to the inclusion of an interactive nitrogen cycle was seen in GPP, where the CMIP6 nitrogen cycle models were able to capture the seasonal cycle in the Northern Hemisphere well, which was previously overestimated. Beside the improvements in the NH, bias patterns in the tropics showing larger GPP overestimations bordering tropical rainforests are reduced in CMIP6 models, with some of these biases wholly removed in the multi-model mean of the CMIP6 models with interactive nitrogen cycle.
The land carbon sink is underestimated in the Northern Hemisphere, regardless of the CMIP phase or the inclusion of the nitrogen cycle. The models compensate for this by simulating a larger carbon sink in the tropics and the Southern Hemisphere for a global average close to the observed value. An improvement is seen in CMIP6 in capturing the amplitude of the seasonal cycle, which is controlled by carbon uptake through photosynthesis in the growth season and carbon release by respiration. This improvement can largely be attributed to the improved seasonal cycle of GPP.
The large range of soil and vegetation carbon was another large weakness of CMIP5, with inter-model differences of 900 PgC for vegetation carbon and 2500 PgC for soil carbon. This range has not significantly decreased in CMIP6, and it remains an area for improvement.
While we find a significant improvement through the inclusion of the nitrogen cycle for photosynthesis, the effects are reduced for the leaf area index and the land–atmosphere carbon flux. Despite similar NBP values for models with and without interactive nitrogen cycle, models without interactive nitrogen overestimate carbon fertilization, leading to large differences in atmospheric carbon content for future scenario simulations (Kou-Giesbrecht and Arora, 2023). Therefore, the inclusion of further limiting nutrients like phosphorus is important, as they will likely have substantial impacts on future carbon uptake (Yang et al., 2023). Model performance overall has improved from CMIP5 to CMIP6, even with the added complexity introducing more degrees of freedom into the models, as also found in the latest IPCC report (Eyring et al., 2021; Canadell et al., 2021). This is a positive outlook for the future, as many aspects have to be considered when increasing model complexity, such as a need to adjust the existing parameterizations after model structural changes from carbon-only to carbon–nitrogen coupling. Without such adjustments, lowered ecosystem carbon storage simulated by models with N processes would lead to an underestimation of carbon pools (Wang et al., 2022). The increased overall model performance confirms results from the individual model groups that found improved performance in carbon cycle variables compared to previous model configurations, with the biggest improvements seen in LAI and GPP (Ziehn et al., 2017; Danabasoglu et al., 2020). Many areas requiring improvement remain, such as simulated carbon stocks, which saw no significant reduction in the simulated range between CMIP5 and CMIP6, or the inclusion of more nutrient limitations like an interactive phosphorus cycle. The improvement of the carbon cycle in the models since CMIP5 is a step in the right direction for a better understanding and a more accurate simulation of future trends. Based on our analysis, due to the small differences between historical concentration- and emission-driven simulations, despite the increased process realism, we recommend ESMs in future CMIP phases to be based on emission-driven simulations to fully account for climate–carbon feedbacks in future projections, supporting the message from Sanderson et al. (2024). Similarly, due to the significant improvements in GPP with the inclusion of an interactive nitrogen cycle and no detrimental change in the present-day evaluation of any carbon cycle variable, we suggest that the nitrogen cycle should be seen as a necessary part of carbon cycle models in the future.
While a summary of the model components for each model can be found in Tables 1 and 2, some special characteristics of the carbon cycle in the land surface components are given below. As several ESMs use the same land model, the sections are listed by the land model instead of the ESM. If different versions of the same component were used in different CMIP models, then the difference between the versions, as well as the models using each version, are explained.
A1 CABLE + CASA-CNP
The Community Atmosphere–Biosphere Land Exchange model (CABLE; Kowalczyk et al., 2013) version 2.4 is a land surface model coupled to the biogeochemistry module Carnegie–Ames–Stanford Approach carbon cycle model with nitrogen and phosphorus cycles (CASA-CNP; Wang et al., 2010) used in ACCESS-ESM1-5 (Ziehn et al., 2020). CASA-CNP and thus ACCESS-ESM1.5 is the only model in this study to include a phosphorus cycle coupled to the land carbon–nitrogen cycle. A sensitivity study of allowable emissions to nutrient limitation found a reduction in the land carbon uptake by 35 %–40 % with nitrogen limitation and a further 20 %–30 % reduction with nitrogen and phosphorus limitation on the carbon cycle (Zhang et al., 2013), showing the importance of nutrient limitation.
A simple land use scheme accounts for the annual net change in the vegetation tile fractions of each grid cell, which consider 10 vegetated and 3 non-vegetated surfaces. Three live and six dead carbon pools are modeled. Leaf area index (LAI) in ACCESS-ESM1-5 is calculated from a specific leaf area and the size of the leaf carbon pool, while phenology is prescribed. In the CMIP5 model ACCESS-ESM1, which is not considered in this paper due to a lack of variables on the ESGF, LAI was significantly higher than observations, mainly due to an overestimation of LAI in the Northern Hemisphere, despite a significant underestimation of LAI in the tropics. To better match the observations, two parameters were adjusted for ACCESS-ESM1-5, namely one PFT-specific parameter used in the parameterization for the maximum carboxylation rate, and thus related to the nitrogen cycle, and one global parameter related to the daytime leaf respiration rate. Further changes to the model since CMIP5 include the conservation of land carbon, which was not conserved in CMIP5, and the inclusion of wetland tiles in the biogeochemistry calculation and the removal of a spin-up condition which ensured a minimum nitrogen and phosphorus level in soil pools.
A2 CLASS + CTEM
The land component in the Canadian Earth System Model (CanESM) is divided into the physical part represented by the Canadian Land Surface Scheme (CLASS; Verseghy, 1991, 2000; Verseghy et al., 1993) and the biogeochemical processes as simulated by the Canadian Terrestrial Ecosystem Model (CTEM; Arora, 2003; Arora and Boer, 2003, 2005). In the CMIP5 model (CanESM2; Arora et al., 2011), version 2.7 of CLASS was used, while the CMIP6 models CanESM and CanESM-CanOE (Swart et al., 2019a) employ CLASS v3.6. While neither version includes a nitrogen cycle, a parameter representing terrestrial photosynthesis downregulation is included to simulate the effect of nutrient constraints. This parameter is increased in CanESM5 compared to the previous version, CanESM2, resulting in a higher land carbon uptake in CanESM5. Four PFTs are considered in CLASS, while CTEM increases the number to nine PFTs so that phenology can be simulated prognostically. LAI is dynamically simulated, and three live and two dead carbon pools are considered. Added features since CanESM2 include dynamic wetlands and their diagnostic methane emissions.
A3 CLM
The Community Land Model (CLM; UCAR, 2020) is the most commonly used land model in this study, with 11 models across CMIP5 and CMIP6 using 3 different versions of it. For the CMIP5 models, CLM3.5 (Oleson et al., 2008) was used by FIO-ESM, and CLM4 (Lawrence et al., 2011) was used in CESM1-BGC and NorESM1-ME. In CMIP6, CLM4 is used for SAM0-UNICON and TaiESM, which mainly adapted the CESM1 configuration (Lee et al., 2020). CMCC-CM2-SR5 and CMCC-ESM2 use CLM4.5 (Koven et al., 2013), while the newest version, CLM5 (Lawrence et al., 2019), is part of CESM2, CESM2-WACCM, NorESM2-LM, and NorESM2-MM.
While in CLM3.5 the nitrogen limitation was merely represented by a downregulation factor, CLM4 introduced the coupled carbon–nitrogen cycle. Further improvements in CLM4 included transient land cover change modeling, changes to the PFT distribution, and more realistic modeling of permafrost regions. To reduce biases found in CLM4 such as low soil carbon stocks and unrealistic values for GPP and LAI in several regions such as a stark overestimation in the tropics, several parameterizations were changed in CLM4.5. Modifications were made to the canopy processes, including co-limitations on photosynthesis and photosynthetic parameters. Newly introduced features included a vertically resolved soil biogeochemistry with vertical mixing of soil carbon and nitrogen and a more realistic distribution of biological fixation over the year. The structure of the litter and soil carbon and nitrogen pools was adapted to the century model, and 13C and 14C carbon isotopes were introduced.
Finally, in CLM5, many major components of the land model were updated, with a focus on a better representation of land use and land cover change, as well as a more mechanistic treatment of key processes. Changes included a stronger soil moisture control on decomposition, the use of 13C and 14C isotopes for crops, and several changes to the nitrogen cycle and its impact on photosynthesis. Flexible plant C : N ratios were introduced to eliminate instantaneous downregulation of photosynthesis, leaf nitrogen was optimized in the form of the leaf use of nitrogen for assimilation (LUNA; Ali et al., 2016) model, and a model handling the fixation and uptake of nitrogen (FUN; Shi et al., 2016) was included. With respect to the land use and land cover aspect of the model, land unit weights are no longer fixed during the simulation, and the transient PFT distribution was updated. CLM5 considers 22 live and 7 dead carbon pools, as well as 22 PFTs.
A4 CoLM + BNU-DGVM
The Common Land Model (CoLM; Dai et al., 2003), which shares an initial version with CLM but was then developed separately, is the land model component for the CMIP5 model BNU-ESM in the CoLM2005 version. CoLM includes a photosynthesis–stomatal conductance model for sunlit and shaded leaves separately. While carbon–nitrogen cycle interactions were included in the model, they were turned off for the CMIP5 simulations due to not being fully evaluated at the time (Ji et al., 2014).
A5 HAL
The land model for the MRI models in both its CMIP5 version MRI-ESM1 and the CMIP6 version MRI-ESM2-0 is the Hydrology, Atmosphere, and Land surface model (HAL; Hosaka, 2011). It consists of three submodels called SiByl (vegetation), with grass and canopy vegetation layers; SNOWA (snow); and SOILA (soil), with 14 soil layers in the CMIP5 experiments.
A6 ISBA-CTRIP
The land component for the CNRM-ESM2-1 model is presented by the Interaction Soil–Biosphere–Atmosphere (ISBA) land surface model and the total runoff integrating pathways (CTRIP) river routing model (Decharme et al., 2019; Delire et al., 2020). ISBA-CTRIP simulates plant physiology, leaf phenology, carbon allocation and turnover, wild fires, and carbon cycling through litter and soil (Séférian et al., 2019). Land use processes are prescribed instead of simulated, while land cover changes are used to represent anthropogenic disturbances. While the model does not include an interactive nitrogen cycle, its effects are included through an artificial downregulation of photosynthesis and a reduced specific leaf area with increasing CO2 concentration. There are 6 live and 7 dead carbon pools considered, along with 16 PFTs (Gibelin et al., 2008). Changes since the previous version used in CNRM-ESM-1 include improvements to the photosynthetic and autotrophic respiration schemes.
CTRIP includes carbon leaching through the soil and subsequent transport of dissolved organic carbon to the ocean. As chemical species such as dissolved inorganic carbon are not included, the air–water carbon exchange in the river routing model CTRIP cannot be computed. This leads to a carbon cycle which is not fully bounded.
A7 JSBACH
JSBACH is the land component of the MPI-ESM model, with version 3.2 used for MPI-ESM1.2 (Mauritsen et al., 2019). In the previous version, parameters in the model for the decomposition of dead organic matter were tuned to reproduce the historical atmospheric CO2 concentrations, with soil and litter carbon stocks merely being the result of this tuning. In version 3.2, decomposition is handled by the YASSO model (Tuomi et al., 2011), based on litter and soil data and resulting in no unconstrained parameters. YASSO simulates four fast soil carbon pools and one slow pool. A total of 18 dead carbon pools is considered due to a different application based on the woody and non-woody origins, as well as above- and belowground decomposition. Additionally, 3 live carbon pools (natural vegetation, crops, and pasture) and 13 PFTs are simulated by JSBACH, while permafrost carbon is not considered. The dynamical vegetation component interacts with the land use changes, modifying the land use data set to conform to the JSBACH setup. JSBACH3.2 includes an interactive terrestrial nitrogen cycle (Goll et al., 2017) driven by the nitrogen demand of the carbon cycle. Further adjustments in v3.2 include the change in carbon timescales in wood pools to be PFT-specific.
A8 JULES
The Joint UK Land Environment Simulator (JULES; Best et al., 2011; Clark et al., 2011) is the land model for the CMIP5 models HadGEM2-CC and HadGEM2-ES with the terrestrial carbon cycle, following the Top-down Representation of Interaction of Foliage and Flora Including Dynamics (TRIFFID; Cox, 2001) dynamic vegetation scheme. The CMIP6 model UKESM1-0-LL (Sellar et al., 2019) employs JULES version 5.0.
Improvements in the version used for UKESM1-0-LL include the introduction of nitrogen cycling, as well as developments to plant physiology and functional types and land use. In this model, nitrogen controls biomass and the leaf area index within TRIFFID, thus only indirectly affecting photosynthetic capacity, as well as limiting the decomposition of litter into soil carbon. For better agreement with observations, global total GPP was tuned down through a reduction in the quantum efficiency of photosynthesis. Furthermore, crop and pasture areas were separated, and a harvest carbon flux was introduced. UKESM1 has four soil carbon pools, nine natural PFTs – increased from five in prior versions – and four PFTs for crop and pasture.
A9 LM
The GFDL Land Model (LM; Anderson et al., 2004) is used in the GFDL Earth System Model with the CMIP5 models GFDL-ESM2G and GFDL-ESM2M (Dunne et al., 2012, 2013), using version 2.0, and the CMIP6 model GFDL-ESM4, employing version 4.1 (Dunne et al., 2020). Neither version includes an interactive nitrogen cycle.
Improvements since CMIP5 include updated soil types in the CORPSE model (Sulman et al., 2014, 2019), hydrology, and radiation, as well as the inclusion of a new fire model FINAL (Rabin et al., 2018), with daily computations instead of previously annual figures and a new model for vegetation dynamics through the perfect plasticity approximation (PPA; Weng et al., 2015). LM4.1 includes 6 live carbon pools for leaves, fine roots, heartwood, sapwood, seeds, and nonstructural carbon, and there are 20 vertical soil levels split into separate fast and slow pools and pools for soil microbes and microbial products. Six PFTs are included, representing C3 grass, C4 grass, tropical trees, temperate deciduous trees, and cold evergreen trees. Land use is accounted for through annual wood harvesting, crop planting and harvesting, pasture grazing, and newly included rangelands.
A10 LPJ-GUESS
The Lund–Potsdam–Jena General Ecosystem Simulator (LPJ-GUESS; Smith et al., 2014) in combination with the Hydrology Tiled ECMWF Scheme for Surface Exchanges over Land (H-TESSEL; Balsamo et al., 2009) is the land model used in the EC-Earth models EC-Earth3-CC and EC-Earth3-Veg (Döscher et al., 2022). The model described as “CC” additionally includes ocean biogeochemistry (PISCES) and atmospheric composition for CO2 (TM5), letting it perform CO2 emission-driven simulations.
H-TESSEL solves the energy and water balance at the land surface, while vegetation types and vegetation coverage are interactively provided by the coupled LPJ-GUESS, which includes an interactive nitrogen cycle. Compared to the common area-based vegetation schemes, the interactive coupling of LPJ-GUESS to an atmospheric model should improve realism on longer timescales (Döscher et al., 2022). LPJ-GUESS includes 10 L pools, seven vegetation carbon pools, and five soil carbon pools. Wildfires, disturbances, and land use change are simulated on a yearly time step and distributed evenly throughout the year to conserve carbon mass. Land use change dynamics are considered together with a crop module (Lindeskog et al., 2013), including five crop functional types. Three types of plant phenology – evergreen, seasonal deciduous, and stress deciduous – are considered, with only the latter two being simulated with an explicit phenological cycle. Seasonal deciduous PFTs have a fixed growing season length of 210 d, while the growing season for stress deciduous PFTs is determined by a threshold for water stress.
A11 MATSIRO + SEIB-DGVM/VISIT-e
The Minimal Advanced Treatments of Surface Interaction and RunOff (MATSIRO; Takata et al., 2003) is the physical land model for the MIROC-ESM family – MIROC-ESM and MIROC-ESM-CHEM (with coupled atmospheric chemistry) for CMIP5 (Watanabe et al., 2011) and MIROC-ES2L for CMIP6 (Hajima et al., 2020b) – which consists of a single-canopy layer, three snow layers, and six soil layers down to a depth of 14 m. For the CMIP6 version, a physically based parameterization for snow distribution and snow-derived wetlands was added. Biogeochemistry in MIROC-ESM and MIROC-ESM-CHEM is simulated by the spatially explicit individual-based dynamic global vegetation model (SEIB-DGVM; Sato et al., 2007). It includes 13 PFTs split into 2 for grass and 11 for trees, as well as 2 organic carbon pools. Light capture competition among trees is explicitly modeled instead of parameterized.
MIROC-ES2L for CMIP6 uses the Vegetation Integrative SImulator for Trace gases model (VISIT; Ito and Inatomi, 2012), with changes for coupling to the ESM (adding the “-e” suffix), such as including leaf nitrogen concentrations, and thus limitations to enable fully coupled climate–carbon–nitrogen projections, and land use change processes to get more use out of new land use change (LUC) forcing data sets, such as using five types of land cover. The model does not simulate explicit dynamic vegetation. Three vegetation carbon pools (leaf, stem, and root) are dynamically regulated and have constant turnover rates to three litter and three soil pools. In total, 12 vegetation types are considered. A daily time step is used for the land ecosystem and land biogeochemistry.
A12 ORCHIDEE
The Organising Carbon and Hydrology In Dynamic Ecosystems (ORCHIDEE; Krinner et al., 2005; Boucher et al., 2020) land model is used in the IPSL models, with version 1 for the CMIP5 models IPSL-CM5A-LR and IPSL-CM5A-MR and version 2 in the CMIP6 model IPSL-CM5B-LR. The model considers 15 PFTs, as well as 8 vegetation carbon, 4 L carbon, and 3 soil carbon pools. Plant and soil carbon fluxes are computed every 15 min (the same as the atmospheric physics time step), while slow processes like soil and litter carbon dynamics are computed daily instead. The CMIP5 model used a 2-layer bucket model for its soil hydrology, while in CMIP6 an 11-layer soil hydrology scheme is employed. Photosynthesis is parameterized based on the common Farquhar and Collatz schemes for C3 and C4, respectively. Nutrient limitation in CMIP6 is introduced through downregulation using a logarithmic function of the CO2 concentration.
A13 Other: INMCM
The carbon cycle module for INMCM (Volodin, 2007) includes a single soil carbon pool. The most important changes with respect to inmcm4 for the CMIP6 models lie in the atmospheric component of the model, as well as some upgrades to the oceanic component, but there are no changes to the carbon cycle (Volodin et al., 2017b).
The code to reproduce this study is part of ESMValTool (Righi et al., 2020; Eyring et al., 2020). The corresponding code is made available in ESMValTool v2.12, with recipes to reproduce the figures found in the folder gier24bg. ESMValTool v2 is released under the Apache License, version 2.0. The latest release of ESMValTool v2 is publicly available on Zenodo at https://doi.org/10.5281/zenodo.3401363 (Andela et al., 2023a). The source code of the ESMValCore package, which is installed as a dependency of ESMValTool v2, is also publicly available on Zenodo at https://doi.org/10.5281/zenodo.3387139 (Andela et al., 2023b). ESMValTool and ESMValCore are developed on the GitHub repositories available at https://github.com/ESMValGroup (last access: 25 November 2024) and with contributions from the community being very welcome.
CMIP data are available for download via ESGF nodes (ESGF, 2024). Reference data sets have been formatted for use with ESMValTool using so-called CMORizers. When available, DOIs for all data sets contributing to this study are listed in Tables 1–3 along with their references.
The supplement related to this article is available online at: https://doi.org/10.5194/bg-21-5321-2024-supplement.
BKG led the writing and analysis of the paper. MS assisted with parts of the programming. VE supervised the study. All authors contributed to the writing of the paper.
At least one of the (co-)authors is a member of the editorial board of Biogeosciences. The peer-review process was guided by an independent editor, and the authors also have no other competing interests to declare.
Publisher’s note: Copernicus Publications remains neutral with regard to jurisdictional claims made in the text, published maps, institutional affiliations, or any other geographical representation in this paper. While Copernicus Publications makes every effort to include appropriate place names, the final responsibility lies with the authors.
We acknowledge the World Climate Research Programme (WCRP), which, through its Working Group on Coupled Modeling, coordinated and promoted CMIP6. We thank the climate modeling groups (listed in Tables 1 and 2) for producing and making available their model output, the Earth System Grid Federation (ESGF) for archiving the data and providing access, and the multiple funding agencies that support CMIP and ESGF. The computational resources of the Deutsches Klimarechenzentrum (DKRZ, Germany) were used to compute these results under project bd0854 and are gratefully acknowledged.
This research has been funded by the European Union's Horizon 2020 research and innovation programs “Climate–Carbon interactions in the Current Century” (4C; grant no. 821003) and “Earth System Models for the Future” (ESM2025; grant no. 101003536). The research for this study was additionally funded by the European Research Council (ERC) Synergy Grant “Understanding and Modeling the Earth System with Machine Learning” (USMILE) under the EU Horizon 2020 Research and Innovation programme (grant no. 855187). Veronika Eyring has also been supported by the Deutsche Forschungsgemeinschaft (DFG, German Research Foundation) through the Gottfried Wilhelm Leibniz Prize (reference no. EY 22/2-1).
The article processing charges for this open-access publication were covered by the University of Bremen.
This paper was edited by Andreas Ibrom and reviewed by Christopher Reyer and one anonymous referee.
Ali, A. A., Xu, C., Rogers, A., Fisher, R. A., Wullschleger, S. D., Massoud, E. C., Vrugt, J. A., Muss, J. D., McDowell, N. G., Fisher, J. B., Reich, P. B., and Wilson, C. J.: A global scale mechanistic model of photosynthetic capacity (LUNA V1.0), Geosci. Model Dev., 9, 587–606, https://doi.org/10.5194/gmd-9-587-2016, 2016. a
Anav, A., Friedlingstein, P., Kidston, M., Bopp, L., Ciais, P., Cox, P., Jones, C., Jung, M., Myneni, R., and Zhu, Z.: Evaluating the Land and Ocean Components of the Global Carbon Cycle in the CMIP5 Earth System Models, J. Clim., 26, 6801–6843, https://doi.org/10.1175/Jcli-D-12-00417.1, 2013a. a, b, c, d, e, f, g
Anav, A., Murray-Tortarolo, G., Friedlingstein, P., Sitch, S., Piao, S., and Zhu, Z.: Evaluation of Land Surface Models in Reproducing Satellite Derived Leaf Area Index over the High-Latitude Northern Hemisphere, Part II: Earth System Models, Remote Sens., 5, 3637–3661, https://doi.org/10.3390/rs5083637, 2013b. a, b, c
Anav, A., Friedlingstein, P., Beer, C., Ciais, P., Harper, A., Jones, C., Murray-Tortarolo, G., Papale, D., Parazoo, N. C., Peylin, P., Piao, S., Sitch, S., Viovy, N., Wiltshire, A., and Zhao, M.: Spatiotemporal patterns of terrestrial gross primary production: A review, Rev. Geophys., 53, 785–818, https://doi.org/10.1002/2015RG000483, 2015. a, b
Andela, B., Broetz, B., de Mora, L., Drost, N., Eyring, V., Koldunov, N., Lauer, A., Mueller, B., Predoi, V., Righi, M., Schlund, M., Vegas-Regidor, J., Zimmermann, K., Adeniyi, K., Arnone, E., Bellprat, O., Berg, P., Bock, L., Caron, L.-P., Carvalhais, N., Cionni, I., Cortesi, N., Corti, S., Crezee, B., Davin, E. L., Davini, P., Deser, C., Diblen, F., Docquier, D., Dreyer, L., Ehbrecht, C., Earnshaw, P., Gier, B., Gonzalez-Reviriego, N., Goodman, P., Hagemann, S., von Hardenberg, J., Hassler, B., Hunter, A., Kadow, C., Kindermann, S., Koirala, S., Lledó, L., Lejeune, Q., Lembo, V., Little, B., Loosveldt-Tomas, S., Lorenz, R., Lovato, T., Lucarini, V., Massonnet, F., Mohr, C. W., Moreno-Chamarro, E., Amarjiit, P., Pérez-Zanón, N., Phillips, A., Russell, J., Sandstad, M., Sellar, A., Senftleben, D., Serva, F., Sillmann, J., Stacke, T., Swaminathan, R., Torralba, V., Weigel, K., Roberts, C., Kalverla, P., Alidoost, S., Verhoeven, S., Vreede, B., Smeets, S., Soares Siqueira, A., and Kazeroni, R.: ESMValTool, Zenodo [data set], https://doi.org/10.5281/zenodo.8120970, 2023a. a
Andela, B., Broetz, B., de Mora, L., Drost, N., Eyring, V., Koldunov, N., Lauer, A., Predoi, V., Righi, M., Schlund, M., Vegas-Regidor, J., Zimmermann, K., Bock, L., Diblen, F., Dreyer, L., Earnshaw, P., Hassler, B., Little, B., Loosveldt-Tomas, S., Smeets, S., Camphuijsen, J., Gier, B. K., Weigel, K., Hauser, M., Kalverla, P., Galytska, E., Cos-Espuña, P., Pelupessy, I., Koirala, S., Stacke, T., Alidoost, S., Jury, M., Sénési, S., Crocker, T., Vreede, B., Soares Siqueira, A., Kazeroni, R., and Bauer, J.: ESMValCore, Zenodo [data set], https://doi.org/10.5281/zenodo.8112684, 2023b. a
Anderson, J., Balaji, V., Broccoli, A., Cooke, W., Delworth, T., Dixon, K., Donner, L., Dunne, K., Freidenreich, S., Garner, S., Gudgel, R., Gordon, C., Held, I., Hemler, R., Horowitz, L., Klein, S., Knutson, T., Kushner, P., Langenhost, A., Lau, N., Liang, Z., Malyshev, S., Milly, P., Nath, M., Ploshay, J., Ramaswamy, V., Schwarzkopf, M., Shevliakova, E., Sirutis, J., Soden, B., Stern, W., Thompson, L., Wilson, R., Wittenberg, A., and Wyman, B.: The new GFDL global atmosphere and land model AM2-LM2: Evaluation with prescribed SST simulations, J. Clim., 17, 4641–4673, https://doi.org/10.1175/JCLI-3223.1, 2004. a
Arora, V. K.: Simulating energy and carbon fluxes over winter wheat using coupled land surface and terrestrial ecosystem models, Agr. Forest Meteorol., 118, 21–47, https://doi.org/10.1016/S0168-1923(03)00073-X, 2003. a
Arora, V. K. and Boer, G. J.: A Representation of Variable Root Distribution in Dynamic Vegetation Models, Earth Interact., 7, 1 – 19, https://doi.org/10.1175/1087-3562(2003)007<0001:AROVRD>2.0.CO;2, 2003. a
Arora, V. K. and Boer, G. J.: A parameterization of leaf phenology for the terrestrial ecosystem component of climate models, Glob. Change Biol., 11, 39–59, https://doi.org/10.1111/j.1365-2486.2004.00890.x, 2005. a
Arora, V. K., Scinocca, J. F., Boer, G. J., Christian, J. R., Denman, K. L., Flato, G. M., Kharin, V. V., Lee, W. G., and Merryfield, W. J.: Carbon emission limits required to satisfy future representative concentration pathways of greenhouse gases, Geophys. Res. Lett., 38, L05805, https://doi.org/10.1029/2010gl046270, 2011. a, b
Arora, V. K., Katavouta, A., Williams, R. G., Jones, C. D., Brovkin, V., Friedlingstein, P., Schwinger, J., Bopp, L., Boucher, O., Cadule, P., Chamberlain, M. A., Christian, J. R., Delire, C., Fisher, R. A., Hajima, T., Ilyina, T., Joetzjer, E., Kawamiya, M., Koven, C. D., Krasting, J. P., Law, R. M., Lawrence, D. M., Lenton, A., Lindsay, K., Pongratz, J., Raddatz, T., Séférian, R., Tachiiri, K., Tjiputra, J. F., Wiltshire, A., Wu, T., and Ziehn, T.: Carbon–concentration and carbon–climate feedbacks in CMIP6 models and their comparison to CMIP5 models, Biogeosciences, 17, 4173–4222, https://doi.org/10.5194/bg-17-4173-2020, 2020. a
Balsamo, G., Beljaars, A., Scipal, K., Viterbo, P., van den Hurk, B., Hirschi, M., and Betts, A. K.: A Revised Hydrology for the ECMWF Model: Verification from Field Site to Terrestrial Water Storage and Impact in the Integrated Forecast System, J. Hydrometeorol., 10, 623–643, https://doi.org/10.1175/2008JHM1068.1, 2009. a
Bao, Y., Qiao, F.-L., and Song, Z.: The historical global carbon cycle simulation of FIO-ESM, Geophys. Res. Abstr., EGU2012-6834, EGU General Assembly 2012, Vienna, Austria, 2012. a
Bentsen, M., Oliviè, D. J. L., Seland, y., Toniazzo, T., Gjermundsen, A., Graff, L. S., Debernard, J. B., Gupta, A. K., He, Y., Kirkevåg, A., Schwinger, J., Tjiputra, J., Aas, K. S., Bethke, I., Fan, Y., Griesfeller, J., Grini, A., Guo, C., Ilicak, M., Karset, I. H. H., Landgren, O. A., Liakka, J., Moseid, K. O., Nummelin, A., Spensberger, C., Tang, H., Zhang, Z., Heinze, C., Iversen, T., and Schulz, M.: NCC NorESM2-MM model output prepared for CMIP6 CMIP historical, Earth System Grid Federation [data set], https://doi.org/10.22033/ESGF/CMIP6.8040, 2019. a
Best, M. J., Pryor, M., Clark, D. B., Rooney, G. G., Essery, R. L. H., Ménard, C. B., Edwards, J. M., Hendry, M. A., Porson, A., Gedney, N., Mercado, L. M., Sitch, S., Blyth, E., Boucher, O., Cox, P. M., Grimmond, C. S. B., and Harding, R. J.: The Joint UK Land Environment Simulator (JULES), model description – Part 1: Energy and water fluxes, Geosci. Model Dev., 4, 677–699, https://doi.org/10.5194/gmd-4-677-2011, 2011. a
Bock, L., Lauer, A., Schlund, M., Barreiro, M., Bellouin, N., Jones, C., Meehl, G. A., Predoi, V., Roberts, M. J., and Eyring, V.: Quantifying Progress Across Different CMIP Phases With the ESMValTool, J. Geophys. Res.-Atmos., 125, e2019JD032321, https://doi.org/10.1029/2019JD032321, e2019JD032321 2019JD032321, 2020. a, b, c
Boucher, O., Denvil, S., Levavasseur, G., Cozic, A., Caubel, A., Foujols, M.-A., Meurdesoif, Y., Cadule, P., Devilliers, M., Ghattas, J., Lebas, N., Lurton, T., Mellul, L.,Musat, I.,Mignot, J., and Cheruy, F.: IPSL IPSL-CM6A-LR model output prepared for CMIP6 CMIP historical, Earth System Grid Federation [data set], https://doi.org/10.22033/ESGF/CMIP6.5195, 2018. a
Boucher, O., Servonnat, J., Albright, A. L., Aumont, O., Balkanski, Y., Bastrikov, V., Bekki, S., Bonnet, R., Bony, S., Bopp, L., Braconnot, P., Brockmann, P., Cadule, P., Caubel, A., Cheruy, F., Codron, F., Cozic, A., Cugnet, D., D'Andrea, F., Davini, P., de Lavergne, C., Denvil, S., Deshayes, J., Devilliers, M., Ducharne, A., Dufresne, J.-L., Dupont, E., Éthé, C., Fairhead, L., Falletti, L., Flavoni, S., Foujols, M.-A., Gardoll, S., Gastineau, G., Ghattas, J., Grandpeix, J.-Y., Guenet, B., Guez, Lionel, E., Guilyardi, E., Guimberteau, M., Hauglustaine, D., Hourdin, F., Idelkadi, A., Joussaume, S., Kageyama, M., Khodri, M., Krinner, G., Lebas, N., Levavasseur, G., Lévy, C., Li, L., Lott, F., Lurton, T., Luyssaert, S., Madec, G., Madeleine, J.-B., Maignan, F., Marchand, M., Marti, O., Mellul, L., Meurdesoif, Y., Mignot, J., Musat, I., Ottlé, C., Peylin, P., Planton, Y., Polcher, J., Rio, C., Rochetin, N., Rousset, C., Sepulchre, P., Sima, A., Swingedouw, D., Thiéblemont, R., Traore, A. K., Vancoppenolle, M., Vial, J., Vialard, J., Viovy, N., and Vuichard, N.: Presentation and Evaluation of the IPSL-CM6A-LR Climate Model, J. Adv. Model. Earth Sy., 12, e2019MS002010, https://doi.org/10.1029/2019MS002010, 2020. a, b
Canadell, J., Monteiro, P., Costa, M., Cotrim da Cunha, L., Cox, P., Eliseev, A., Henson, S., Ishii, M., Jaccard, S., Koven, C., Lohila, A., Patra, P., Piao, S., Rogelj, J., Syampungani, S., Zaehle, S., and Zickfeld, K.: Global Carbon and other Biogeochemical Cycles and Feedbacks, 673–816, Cambridge University Press, Cambridge, United Kingdom and New York, NY, USA, https://doi.org/10.1017/9781009157896.007, 2021. a, b, c, d, e
Cao, S., Li, M., Zhu, Z., Wang, Z., Zha, J., Zhao, W., Duanmu, Z., Chen, J., Zheng, Y., Chen, Y., Myneni, R. B., and Piao, S.: Spatiotemporally consistent global dataset of the GIMMS leaf area index (GIMMS LAI4g) from 1982 to 2020, Earth Syst. Sci. Data, 15, 4877–4899, https://doi.org/10.5194/essd-15-4877-2023, 2023. a, b
Carvalhais, N., Forkel, M., Khomik, M., Bellarby, J., Jung, M., Migliavacca, M., Μu, M., Saatchi, S., Santoro, M., Thurner, M., Weber, U., Ahrens, B., Beer, C., Cescatti, A., Randerson, J. T., and Reichstein, M.: Global covariation of carbon turnover times with climate in terrestrial ecosystems, Nature, 514, 213–217, https://doi.org/10.1038/nature13731, 2014. a
Cherchi, A., Fogli, P. G., Lovato, T., Peano, D., Iovino, D., Gualdi, S., Masina, S., Scoccimarro, E., Materia, S., Bellucci, A., and Navarra, A.: Global Mean Climate and Main Patterns of Variability in the CMCC-CM2 Coupled Model, J. Adv. Model. Earth Sy., 11, 185–209, https://doi.org/10.1029/2018MS001369, 2019. a
Chevallier, F.: On the parallelization of atmospheric inversions of CO2 surface fluxes within a variational framework, Geosci. Model Dev., 6, 783–790, https://doi.org/10.5194/gmd-6-783-2013, 2013. a, b
Chevallier, F., Fisher, M., Peylin, P., Serrar, S., Bousquet, P., Bréon, F.-M., Chédin, A., and Ciais, P.: Inferring CO2 sources and sinks from satellite observations: Method and application to TOVS data, J. Geophys. Res., 110, D24309, https://doi.org/10.1029/2005JD006390, 2005. a, b
Chevallier, F., Ciais, P., Conway, T. J., Aalto, T., Anderson, B. E., Bousquet, P., Brunke, E. G., Ciattaglia, L., Esaki, Y., Fröhlich, M., Gomez, A., Gomez-Pelaez, A. J., Haszpra, L., Krummel, P. B., Langenfelds, R. L., Leuenberger, M., Machida, T., Maignan, F., Matsueda, H., Morguí, J. A., Mukai, H., Nakazawa, T., Peylin, P., Ramonet, M., Rivier, L., Sawa, Y., Schmidt, M., Steele, L. P., Vay, S. A., Vermeulen, A. T., Wofsy, S., and Worthy, D.: CO2 surface fluxes at grid point scale estimated from a global 21 year reanalysis of atmospheric measurements, J. Geophys. Res., 115, D21307, https://doi.org/10.1029/2010JD013887, 2010. a, b
Christiansen, B.: Ensemble Averaging and the Curse of Dimensionality, J. Clim., 31, 1587–1596, https://doi.org/10.1175/JCLI-D-17-0197.1, 2018. a
Ciais, P., Sabine, C., Bala, G., Bopp, L., Brovkin, V., Canadell, J., Chhabra, A., DeFries, R., Galloway, J., Heimann, M., Jones, C., Le Quéré, C., Myneni, R., Piao, S., and Thornton, P.: Carbon and Other Biogeochemical Cycles, in: Climate Change 2013: The Physical Science Basis. Contribution of Working Group I to the Fifth Assessment Report of the Intergovernmental Panel on Climate Change, edited by Stocker, T., Qin, D., Plattner, G.-K., Tignor, M., Allen, S., Boschung, J., Nauels, A., Xia, Y., Bex, V., and Midgley, P., book section 6, 465–570, Cambridge University Press, Cambridge, United Kingdom and New York, NY, USA, ISBN ISBN 978-1-107-66182-0, https://doi.org/10.1017/CBO9781107415324.015, 2013. a
Clark, D. B., Mercado, L. M., Sitch, S., Jones, C. D., Gedney, N., Best, M. J., Pryor, M., Rooney, G. G., Essery, R. L. H., Blyth, E., Boucher, O., Harding, R. J., Huntingford, C., and Cox, P. M.: The Joint UK Land Environment Simulator (JULES), model description – Part 2: Carbon fluxes and vegetation dynamics, Geosci. Model Dev., 4, 701–722, https://doi.org/10.5194/gmd-4-701-2011, 2011. a
Collins, W. J., Bellouin, N., Doutriaux-Boucher, M., Gedney, N., Halloran, P., Hinton, T., Hughes, J., Jones, C. D., Joshi, M., Liddicoat, S., Martin, G., O'Connor, F., Rae, J., Senior, C., Sitch, S., Totterdell, I., Wiltshire, A., and Woodward, S.: Development and evaluation of an Earth-System model – HadGEM2, Geosci. Model Dev., 4, 1051–1075, https://doi.org/10.5194/gmd-4-1051-2011, 2011. a, b
Cox, P. M.: Description of the TRIFFID Dynamic Global Vegetation Model, in: Hadley Centre technical note, vol. 24, Hadley Centre, Met Office, UK, 2001. a
Dai, Y., Zeng, X., Dickinson, R. E., Baker, I., Bonan, G. B., Bosilovich, M. G., Denning, A. S., Dirmeyer, P. A., Houser, P. R., Niu, G., Oleson, K. W., Schlosser, C. A., and Yang, Z.-L.: The Common Land Model, Bull. Am. Meteorol. Soc., 84, 1013–1024, https://doi.org/10.1175/bams-84-8-1013, 2003. a
Danabasoglu, G.: NCAR CESM2-WACCM model output prepared for CMIP6 CMIP historical, Earth System Grid Federation [data set], https://doi.org/10.22033/ESGF/CMIP6.10071, 2019a. a
Danabasoglu, G.: NCAR CESM2 model output prepared for CMIP6 CMIP historical, Earth System Grid Federation [data set], https://doi.org/10.22033/ESGF/CMIP6.7627, 2019b. a
Danabasoglu, G., Lamarque, J.-F., Bacmeister, J., Bailey, D. A., DuVivier, A. K., Edwards, J., Emmons, L. K., Fasullo, J., Garcia, R., Gettelman, A., Hannay, C., Holland, M. M., Large, W. G., Lauritzen, P. H., Lawrence, D. M., Lenaerts, J. T. M., Lindsay, K., Lipscomb, W. H., Mills, M. J., Neale, R., Oleson, K. W., Otto-Bliesner, B., Phillips, A. S., Sacks, W., Tilmes, S., van Kampenhout, L., Vertenstein, M., Bertini, A., Dennis, J., Deser, C., Fischer, C., Fox-Kemper, B., Kay, J. E., Kinnison, D., Kushner, P. J., Larson, V. E., Long, M. C., Mickelson, S., Moore, J. K., Nienhouse, E., Polvani, L., Rasch, P. J., and Strand, W. G.: The Community Earth System Model Version 2 (CESM2), J. Adv. Model. Earth Sy., 12, e2019MS001916, https://doi.org/10.1029/2019ms001916, 2020. a, b, c, d, e, f
Davies-Barnard, T., Meyerholt, J., Zaehle, S., Friedlingstein, P., Brovkin, V., Fan, Y., Fisher, R. A., Jones, C. D., Lee, H., Peano, D., Smith, B., Wårlind, D., and Wiltshire, A. J.: Nitrogen cycling in CMIP6 land surface models: progress and limitations, Biogeosciences, 17, 5129–5148, https://doi.org/10.5194/bg-17-5129-2020, 2020. a
Decharme, B., Delire, C., Minvielle, M., Colin, J., Vergnes, J.-P., Alias, A., Saint-Martin, D., Séférian, R., Sénési, S., and Voldoire, A.: Recent Changes in the ISBA-CTRIP Land Surface System for Use in the CNRM-CM6 Climate Model and in Global Off-Line Hydrological Applications, J. Adv. Model. Earth Sy., 11, 1207–1252, https://doi.org/10.1029/2018MS001545, 2019. a
Delire, C., Séférian, R., Decharme, B., Alkama, R., Calvet, J.-C., Carrer, D., Gibelin, A.-L., Joetzjer, E., Morel, X., Rocher, M., and Tzanos, D.: The global land carbon cycle simulated with ISBA-CTRIP: improvements over the last decade, J. Adv. Model. Earth Sy., 12, e2019MS001886, https://doi.org/10.1029/2019ms001886, 2020. a, b, c
Döscher, R., Acosta, M., Alessandri, A., Anthoni, P., Arsouze, T., Bergman, T., Bernardello, R., Boussetta, S., Caron, L.-P., Carver, G., Castrillo, M., Catalano, F., Cvijanovic, I., Davini, P., Dekker, E., Doblas-Reyes, F. J., Docquier, D., Echevarria, P., Fladrich, U., Fuentes-Franco, R., Gröger, M., v. Hardenberg, J., Hieronymus, J., Karami, M. P., Keskinen, J.-P., Koenigk, T., Makkonen, R., Massonnet, F., Ménégoz, M., Miller, P. A., Moreno-Chamarro, E., Nieradzik, L., van Noije, T., Nolan, P., O'Donnell, D., Ollinaho, P., van den Oord, G., Ortega, P., Prims, O. T., Ramos, A., Reerink, T., Rousset, C., Ruprich-Robert, Y., Le Sager, P., Schmith, T., Schrödner, R., Serva, F., Sicardi, V., Sloth Madsen, M., Smith, B., Tian, T., Tourigny, E., Uotila, P., Vancoppenolle, M., Wang, S., Wårlind, D., Willén, U., Wyser, K., Yang, S., Yepes-Arbós, X., and Zhang, Q.: The EC-Earth3 Earth system model for the Coupled Model Intercomparison Project 6, Geosci. Model Dev., 15, 2973–3020, https://doi.org/10.5194/gmd-15-2973-2022, 2022. a, b, c, d, e
Du, E., Terrer, C., Pellegrini, A. F., Ahlström, A., van Lissa, C. J., Zhao, X., Xia, N., Wu, X., and Jackson, R. B.: Global patterns of terrestrial nitrogen and phosphorus limitation, Nat. Geosci., 13, 221–226, 2020. a
Dufresne, J. L., Foujols, M. A., Denvil, S., Caubel, A., Marti, O., Aumont, O., Balkanski, Y., Bekki, S., Bellenger, H., Benshila, R., Bony, S., Bopp, L., Braconnot, P., Brockmann, P., Cadule, P., Cheruy, F., Codron, F., Cozic, A., Cugnet, D., de Noblet, N., Duvel, J. P., Ethé, C., Fairhead, L., Fichefet, T., Flavoni, S., Friedlingstein, P., Grandpeix, J. Y., Guez, L., Guilyardi, E., Hauglustaine, D., Hourdin, F., Idelkadi, A., Ghattas, J., Joussaume, S., Kageyama, M., Krinner, G., Labetoulle, S., Lahellec, A., Lefebvre, M. P., Lefevre, F., Levy, C., Li, Z. X., Lloyd, J., Lott, F., Madec, G., Mancip, M., Marchand, M., Masson, S., Meurdesoif, Y., Mignot, J., Musat, I., Parouty, S., Polcher, J., Rio, C., Schulz, M., Swingedouw, D., Szopa, S., Talandier, C., Terray, P., Viovy, N., and Vuichard, N.: Climate change projections using the IPSL-CM5 Earth System Model: from CMIP3 to CMIP5, Clim. Dynam., 40, 2123–2165, https://doi.org/10.1007/s00382-012-1636-1, 2013. a, b
Dunne, J. P., John, J. G., Adcroft, A. J., Griffies, S. M., Hallberg, R. W., Shevliakova, E., Stouffer, R. J., Cooke, W., Dunne, K. A., Harrison, M. J., Krasting, J. P., Malyshev, S. L., Milly, P. C. D., Phillipps, P. J., Sentman, L. T., Samuels, B. L., Spelman, M. J., Winton, M., Wittenberg, A. T., and Zadeh, N.: GFDL's ESM2 Global Coupled Climate-Carbon Earth System Models, Part I: Physical Formulation and Baseline Simulation Characteristics, J. Clim., 25, 6646–6665, https://doi.org/10.1175/Jcli-D-11-00560.1, 2012. a, b, c
Dunne, J. P., John, J. G., Shevliakova, E., Stouffer, R. J., Krasting, J. P., Malyshev, S. L., Milly, P. C. D., Sentman, L. T., Adcroft, A. J., Cooke, W., Dunne, K. A., Griffies, S. M., Hallberg, R. W., Harrison, M. J., Levy, H., Wittenberg, A. T., Phillips, P. J., and Zadeh, N.: GFDL's ESM2 Global Coupled Climate-Carbon Earth System Models, Part II: Carbon System Formulation and Baseline Simulation Characteristics, J. Clim., 26, 2247–2267, https://doi.org/10.1175/Jcli-D-12-00150.1, 2013. a, b, c
Dunne, J. P., Horowitz, L. W., Adcroft, A. J., Ginoux, P., Held, I. M., John, J. G., Krasting, J. P., Malyshev, S., Naik, V., Paulot, F., Shevliakova, E., Stock, C. A., Zadeh, N., Balaji, V., Blanton, C., Dunne, K. A., Dupuis, C., Durachta, J., Dussin, R., Gauthier, P. P. G., Griffies, S. M., Guo, H., Hallberg, R. W., Harrison, M., He, J., Hurlin, W., McHugh, C., Menzel, R., Milly, P. C. D., Nikonov, S., Paynter, D. J., Ploshay, J., Radhakrishnan, A., Rand, K., Reichl, B. G., Robinson, T., Schwarzkopf, D. M., Sentman, L. T., Underwood, S., Vahlenkamp, H., Winton, M., Wittenberg, A. T., Wyman, B., Zeng, Y., and Zhao, M.: The GFDL Earth System Model Version 4.1 (GFDL‐ESM 4.1): Overall Coupled Model Description and Simulation Characteristics, J. Adv. Model. Earth Sy., 12, e2019MS002015, https://doi.org/10.1029/2019ms002015, 2020. a, b
EC-Earth Consortium: EC-Earth-Consortium EC-Earth3-Veg model output prepared for CMIP6 CMIP historical, Earth System Grid Federation [data set], https://doi.org/10.22033/ESGF/CMIP6.4706, 2019. a, b
EC-Earth Consortium: EC-Earth-Consortium EC-Earth-3-CC model output prepared for CMIP6 CMIP esm-hist, Earth System Grid Federation [data set], https://doi.org/10.22033/ESGF/CMIP6.4608, 2021a. a
EC-Earth Consortium: EC-Earth-Consortium EC-Earth-3-CC model output prepared for CMIP6 CMIP historical, Earth System Grid Federation [data set], https://doi.org/10.22033/ESGF/CMIP6.4702, 2021b. a, b
ESGF: ESGF MetaGrid, https://esgf-metagrid.cloud.dkrz.de/search (last access: 25 November 2024), 2024. a
Eyring, V., Bony, S., Meehl, G. A., Senior, C. A., Stevens, B., Stouffer, R. J., and Taylor, K. E.: Overview of the Coupled Model Intercomparison Project Phase 6 (CMIP6) experimental design and organization, Geosci. Model Dev., 9, 1937–1958, https://doi.org/10.5194/gmd-9-1937-2016, 2016a. a, b, c
Eyring, V., Righi, M., Lauer, A., Evaldsson, M., Wenzel, S., Jones, C., Anav, A., Andrews, O., Cionni, I., Davin, E. L., Deser, C., Ehbrecht, C., Friedlingstein, P., Gleckler, P., Gottschaldt, K. D., Hagemann, S., Juckes, M., Kindermann, S., Krasting, J., Kunert, D., Levine, R., Loew, A., Makela, J., Martin, G., Mason, E., Phillips, A. S., Read, S., Rio, C., Roehrig, R., Senftleben, D., Sterl, A., van Ulft, L. H., Walton, J., Wang, S. Y., and Williams, K. D.: ESMValTool (v1.0) – a community diagnostic and performance metrics tool for routine evaluation of Earth system models in CMIP, Geosci. Model Dev., 9, 1747–1802, https://doi.org/10.5194/gmd-9-1747-2016, 2016b. a
Eyring, V., Cox, P. M., Flato, G. M., Gleckler, P. J., Abramowitz, G., Caldwell, P., Collins, W. D., Gier, B. K., Hall, A. D., Hoffman, F. M., Hurtt, G. C., Jahn, A., Jones, C. D., Klein, S. A., Krasting, J. P., Kwiatkowski, L., Lorenz, R., Maloney, E., Meehl, G. A., Pendergrass, A. G., Pincus, R., Ruane, A. C., Russell, J. L., Sanderson, B. M., Santer, B. D., Sherwood, S. C., Simpson, I. R., Stouffer, R. J., and Williamson, M. S.: Taking climate model evaluation to the next level, Nat. Clim. Change, 9, 102–110, https://doi.org/10.1038/s41558-018-0355-y, 2019. a
Eyring, V., Bock, L., Lauer, A., Righi, M., Schlund, M., Andela, B., Arnone, E., Bellprat, O., Brötz, B., Caron, L. P., Carvalhais, N., Cionni, I., Cortesi, N., Crezee, B., Davin, E. L., Davini, P., Debeire, K., de Mora, L., Deser, C., Docquier, D., Earnshaw, P., Ehbrecht, C., Gier, B. K., Gonzalez-Reviriego, N., Goodman, P., Hagemann, S., Hardiman, S., Hassler, B., Hunter, A., Kadow, C., Kindermann, S., Koirala, S., Koldunov, N., Lejeune, Q., Lembo, V., Lovato, T., Lucarini, V., Massonnet, F., Müller, B., Pandde, A., Pérez-Zanón, N., Phillips, A., Predoi, V., Russell, J., Sellar, A., Serva, F., Stacke, T., Swaminathan, R., Torralba, V., Vegas-Regidor, J., von Hardenberg, J., Weigel, K., and Zimmermann, K.: Earth System Model Evaluation Tool (ESMValTool) v2.0 – an extended set of large-scale diagnostics for quasi-operational and comprehensive evaluation of Earth system models in CMIP, Geosci. Model Dev., 13, 3383–3438, https://doi.org/10.5194/gmd-13-3383-2020, 2020. a, b, c
Eyring, V., Gillett, N., Achuta Rao, K., Barimalala, R., Barreiro Parrillo, M., Bellouin, N., Cassou, C., Durack, P., Kosaka, Y., McGregor, S., Min, S., Morgenstern, O., and Sun, Y.: Human Influence on the Climate System, pp. 423–552, Cambridge University Press, Cambridge, United Kingdom and New York, NY, USA, https://doi.org/10.1017/9781009157896.005, 2021. a, b, c, d
Flato, G., Marotzke, J., Abiodun, B., Braconnot, P., Chou, S., Collins, W., Cox, P., Driouech, F., Emori, S., Eyring, V., Forest, C., Gleckler, P., Guilyardi, E., Jakob, C., Kattsov, V., Reason, C., and Rummukainen, M.: Evaluation of Climate Models, in: Climate Change 2013: The Physical Science Basis. Contribution of Working Group I to the Fifth Assessment Report of the Intergovernmental Panel on Climate Change, edited by: Stocker, T., Qin, D., Plattner, G.-K., Tignor, M., Allen, S., Boschung, J., Nauels, A., Xia, Y., Bex, V., and Midgley, P., book section 9, 741–866, Cambridge University Press, Cambridge, United Kingdom and New York, NY, USA, https://doi.org/10.1017/CBO9781107415324.020, 2013. a
Friedlingstein, P., Meinshausen, M., Arora, V. K., Jones, C. D., Anav, A., Liddicoat, S. K., and Knutti, R.: Uncertainties in CMIP5 Climate Projections due to Carbon Cycle Feedbacks, J. Clim., 27, 511–526, https://doi.org/10.1175/Jcli-D-12-00579.1, 2014. a, b
Friedlingstein, P., Jones, M. W., O'Sullivan, M., Andrew, R. M., Bakker, D. C. E., Hauck, J., Le Quéré, C., Peters, G. P., Peters, W., Pongratz, J., Sitch, S., Canadell, J. G., Ciais, P., Jackson, R. B., Alin, S. R., Anthoni, P., Bates, N. R., Becker, M., Bellouin, N., Bopp, L., Chau, T. T. T., Chevallier, F., Chini, L. P., Cronin, M., Currie, K. I., Decharme, B., Djeutchouang, L. M., Dou, X., Evans, W., Feely, R. A., Feng, L., Gasser, T., Gilfillan, D., Gkritzalis, T., Grassi, G., Gregor, L., Gruber, N., Gürses, O., Harris, I., Houghton, R. A., Hurtt, G. C., Iida, Y., Ilyina, T., Luijkx, I. T., Jain, A., Jones, S. D., Kato, E., Kennedy, D., Klein Goldewijk, K., Knauer, J., Korsbakken, J. I., Körtzinger, A., Landschützer, P., Lauvset, S. K., Lefèvre, N., Lienert, S., Liu, J., Marland, G., McGuire, P. C., Melton, J. R., Munro, D. R., Nabel, J. E. M. S., Nakaoka, S.-I., Niwa, Y., Ono, T., Pierrot, D., Poulter, B., Rehder, G., Resplandy, L., Robertson, E., Rödenbeck, C., Rosan, T. M., Schwinger, J., Schwingshackl, C., Séférian, R., Sutton, A. J., Sweeney, C., Tanhua, T., Tans, P. P., Tian, H., Tilbrook, B., Tubiello, F., van der Werf, G. R., Vuichard, N., Wada, C., Wanninkhof, R., Watson, A. J., Willis, D., Wiltshire, A. J., Yuan, W., Yue, C., Yue, X., Zaehle, S., and Zeng, J.: Global Carbon Budget 2021, Earth Syst. Sci. Data, 14, 1917–2005, https://doi.org/10.5194/essd-14-1917-2022, 2022. a, b
Friedlingstein, P., O'Sullivan, M., Jones, M. W., Andrew, R. M., Bakker, D. C. E., Hauck, J., Landschützer, P., Le Quéré, C., Luijkx, I. T., Peters, G. P., Peters, W., Pongratz, J., Schwingshackl, C., Sitch, S., Canadell, J. G., Ciais, P., Jackson, R. B., Alin, S. R., Anthoni, P., Barbero, L., Bates, N. R., Becker, M., Bellouin, N., Decharme, B., Bopp, L., Brasika, I. B. M., Cadule, P., Chamberlain, M. A., Chandra, N., Chau, T.-T.-T., Chevallier, F., Chini, L. P., Cronin, M., Dou, X., Enyo, K., Evans, W., Falk, S., Feely, R. A., Feng, L., Ford, D. J., Gasser, T., Ghattas, J., Gkritzalis, T., Grassi, G., Gregor, L., Gruber, N., Gürses, O., Harris, I., Hefner, M., Heinke, J., Houghton, R. A., Hurtt, G. C., Iida, Y., Ilyina, T., Jacobson, A. R., Jain, A., Jarníková, T., Jersild, A., Jiang, F., Jin, Z., Joos, F., Kato, E., Keeling, R. F., Kennedy, D., Klein Goldewijk, K., Knauer, J., Korsbakken, J. I., Körtzinger, A., Lan, X., Lefèvre, N., Li, H., Liu, J., Liu, Z., Ma, L., Marland, G., Mayot, N., McGuire, P. C., McKinley, G. A., Meyer, G., Morgan, E. J., Munro, D. R., Nakaoka, S.-I., Niwa, Y., O'Brien, K. M., Olsen, A., Omar, A. M., Ono, T., Paulsen, M., Pierrot, D., Pocock, K., Poulter, B., Powis, C. M., Rehder, G., Resplandy, L., Robertson, E., Rödenbeck, C., Rosan, T. M., Schwinger, J., Séférian, R., Smallman, T. L., Smith, S. M., Sospedra-Alfonso, R., Sun, Q., Sutton, A. J., Sweeney, C., Takao, S., Tans, P. P., Tian, H., Tilbrook, B., Tsujino, H., Tubiello, F., van der Werf, G. R., van Ooijen, E., Wanninkhof, R., Watanabe, M., Wimart-Rousseau, C., Yang, D., Yang, X., Yuan, W., Yue, X., Zaehle, S., Zeng, J., and Zheng, B.: Global Carbon Budget 2023, Earth Syst. Sci. Data, 15, 5301–5369, https://doi.org/10.5194/essd-15-5301-2023, 2023. a
Gatti, L. V., Basso, L. S., Miller, J. B., Gloor, M., Gatti Domingues, L., Cassol, H. L. G., Tejada, G., Aragão, L. E. O. C., Nobre, C., Peters, W., Marani, L., Arai, E., Sanches, A. H., Corrêa, S. M., Anderson, L., Von Randow, C., Correia, C. S. C., Crispim, S. P., and Neves, R. A. L.: Amazonia as a carbon source linked to deforestation and climate change, Nature, 595, 388–393, https://doi.org/10.1038/s41586-021-03629-6, 2021. a
Gibbs, H. K.: Olson's major world ecosystem complexes ranked by carbon in live vegetation: An updated database using the GLC2000 land cover product, ORNL Environmental Sciences Division [data set], https://doi.org/10.3334/CDIAC/LUE.NDP017.2006, 2006. a, b
Gibelin, A.-L., Calvet, J.-C., and Viovy, N.: Modelling energy and CO2 fluxes with an interactive vegetation land surface model-Evaluation at high and middle latitudes, Agr. Forest Meteorol., 148, 1611–1628, https://doi.org/10.1016/j.agrformet.2008.05.013, 2008. a
Gier, B. K., Buchwitz, M., Reuter, M., Cox, P. M., Friedlingstein, P., and Eyring, V.: Spatially resolved evaluation of Earth system models with satellite column-averaged CO2, Biogeosciences, 17, 6115–6144, https://doi.org/10.5194/bg-17-6115-2020, 2020. a, b
Giorgetta, M. A., Jungclaus, J., Reick, C. H., Legutke, S., Bader, J., Bottinger, M., Brovkin, V., Crueger, T., Esch, M., Fieg, K., Glushak, K., Gayler, V., Haak, H., Hollweg, H. D., Ilyina, T., Kinne, S., Kornblueh, L., Matei, D., Mauritsen, T., Mikolajewicz, U., Mueller, W., Notz, D., Pithan, F., Raddatz, T., Rast, S., Redler, R., Roeckner, E., Schmidt, H., Schnur, R., Segschneider, J., Six, K. D., Stockhause, M., Timmreck, C., Wegner, J., Widmann, H., Wieners, K. H., Claussen, M., Marotzke, J., and Stevens, B.: Climate and carbon cycle changes from 1850 to 2100 in MPI-ESM simulations for the Coupled Model Intercomparison Project phase 5, J. Adv. Model. Earth Sy., 5, 572–597, https://doi.org/10.1002/jame.20038, 2013. a, b
Gleckler, P. J., Taylor, K. E., and Doutriaux, C.: Performance metrics for climate models, J. Geophys. Res.-Atmos., 113, D06104, https://doi.org/10.1029/2007jd008972, 2008. a
Global Carbon Project: Supplemental data of Global Carbon Project 2021, Global Carbon Project [data set], https://doi.org/10.18160/GCP-2021, 2021. a
Goll, D. S., Winkler, A. J., Raddatz, T., Dong, N., Prentice, I. C., Ciais, P., and Brovkin, V.: Carbon–nitrogen interactions in idealized simulations with JSBACH (version 3.10), Geosci. Model Dev., 10, 2009–2030, https://doi.org/10.5194/gmd-10-2009-2017, 2017. a
Hajima, T., Abe, M., Arakawa, O., Suzuki, T., Komuro, Y., Ogura, T., Ogochi, K.,Watanabe, M., Yamamoto, A., Tatebe, H., Noguchi, M. A., Ohgaito, R., Ito, A., Yamazaki, D., Ito, A., Takata, K.,Watanabe, S., Kawamiya, M., and Tachiiri, K.: MIROC MIROC-ES2L model output prepared for CMIP6 CMIP historical, Earth System Grid Federation [data set], https://doi.org/10.22033/ESGF/CMIP6.5602, 2019. a, b
Hajima, T., Abe, M., Arakawa, O., Suzuki, T., Komuro, Y., Ogochi, K., Watanabe, M., Yamamoto, A., Tatebe, H., Noguchi, M. A., Ohgaito, R., Ito, A., Yamazaki, D., Ito, A., Takata, K.,Watanabe, S., Kawamiya, M., and Tachiiri, K.: MIROC MIROC-ES2L model output prepared for CMIP6 CMIP esm-hist, Earth System Grid Federation [data set], https://doi.org/10.22033/ESGF/CMIP6.5496, 2020a. a
Hajima, T., Watanabe, M., Yamamoto, A., Tatebe, H., Noguchi, M. A., Abe, M., Ohgaito, R., Ito, A., Yamazaki, D., Okajima, H., Ito, A., Takata, K., Ogochi, K., Watanabe, S., and Kawamiya, M.: Development of the MIROC-ES2L Earth system model and the evaluation of biogeochemical processes and feedbacks, Geosci. Model Dev., 13, 2197–2244, https://doi.org/10.5194/gmd-13-2197-2020, 2020b. a, b
Hosaka, M.: A new MRI land surface model HAL, in: AGU Fall Meeting Abstracts, vol. 2011, GC43B-0901, American Geophysical Union, 2011. a
Hugelius, G., Tarnocai, C., Broll, G., Canadell, J. G., Kuhry, P., and Swanson, D. K.: The Northern Circumpolar Soil Carbon Database: spatially distributed datasets of soil coverage and soil carbon storage in the northern permafrost regions, Earth Syst. Sci. Data, 5, 3–13, https://doi.org/10.5194/essd-5-3-2013, 2013. a, b, c
Hurrell, J. W., Holland, M. M., Gent, P. R., Ghan, S., Kay, J. E., Kushner, P. J., Lamarque, J.-F., Large, W. G., Lawrence, D., Lindsay, K., Lipscomb, W. H., Long, M. C., Mahowald, N., Marsh, D. R., Neale, R. B., Rasch, P., Vavrus, S., Vertenstein, M., Bader, D., Collins, W. D., Hack, J. J., Kiehl, J., and Marshall, S.: The Community Earth System Model: A Framework for Collaborative Research, Bull. Am. Meteorol. Soc., 94, 1339–1360, https://doi.org/10.1175/bams-d-12-00121.1, 2013. a
IPCC: Climate Change 2021: The Physical Science Basis, Contribution of Working Group I to the Sixth Assessment Report of the Intergovernmental Panel on Climate Change, vol. In Press, Cambridge University Press, Cambridge, United Kingdom and New York, NY, USA, https://doi.org/10.1017/9781009157896, 2021. a, b
Ito, A. and Inatomi, M.: Water-Use Efficiency of the Terrestrial Biosphere: A Model Analysis Focusing on Interactions between the Global Carbon and Water Cycles, J. Hydrometeorol., 13, 681–694, https://doi.org/10.1175/JHM-D-10-05034.1, 2012. a
Ji, D., Wang, L., Feng, J., Wu, Q., Cheng, H., Zhang, Q., Yang, J., Dong, W., Dai, Y., Gong, D., Zhang, R. H., Wang, X., Liu, J., Moore, J. C., Chen, D., and Zhou, M.: Description and basic evaluation of Beijing Normal University Earth System Model (BNU-ESM) version 1, Geosci. Model Dev., 7, 2039–2064, https://doi.org/10.5194/gmd-7-2039-2014, 2014. a, b, c
Jones, C. D.: So What Is in an Earth System Model?, J. Adv. Model. Earth Sy., 12, e2019MS001967, https://doi.org/10.1029/2019ms001967, 2020. a
Jones, C. D., Ziehn, T., Anand, J., Bastos, A., Burke, E., Canadell, J. G., Cardoso, M., Ernst, Y., Jain, A. K., Jeong, S., Keller, E. D., Kondo, M., Lauerwald, R., Lin, T.-S., Murray-Tortarolo, G., Nabuurs, G.-J., O’Sullivan, M., Poulter, B., Qin, X., von Randow, C., Sanches, M., Schepaschenko, D., Shvidenko, A., Smallman, T. L., Tian, H., Villalobos, Y., Wang, X., and Yun, J.: RECCAP2 Future Component: Consistency and Potential for Regional Assessment to Constrain Global Projections, AGU Adv., 4, e2023AV001024, https://doi.org/10.1029/2023AV001024, 2023. a
Jung, M., Reichstein, M., Margolis, H. A., Cescatti, A., Richardson, A. D., Arain, M. A., Arneth, A., Bernhofer, C., Bonal, D., Chen, J., Gianelle, D., Gobron, N., Kiely, G., Kutsch, W., Lasslop, G., Law, B. E., Lindroth, A., Merbold, L., Montagnani, L., Moors, E. J., Papale, D., Sottocornola, M., Vaccari, F., and Williams, C.: Global patterns of land-atmosphere fluxes of carbon dioxide, latent heat, and sensible heat derived from eddy covariance, satellite, and meteorological observations, J. Geophys. Res.-Biogeo., 116, G00J07, https://doi.org/10.1029/2010jg001566, 2011. a, b
Jung, M., Koirala, S., Weber, U., Ichii, K., Gans, F., Camps-Valls, G., Papale, D., Schwalm, C., Tramontana, G., and Reichstein, M.: The FLUXCOM ensemble of global land-atmosphere energy fluxes, Sci. Data, 6, 74, https://doi.org/10.1038/s41597-019-0076-8, 2019. a, b
Keenan, T. and Williams, C.: The Terrestrial Carbon Sink, Annu. Rev. Environ. Res., 43, 219–243, https://doi.org/10.1146/annurev-environ-102017-030204, 2018. a
Kou-Giesbrecht, S. and Arora, V. K.: Compensatory Effects Between CO2, Nitrogen Deposition, and Nitrogen Fertilization in Terrestrial Biosphere Models Without Nitrogen Compromise Projections of the Future Terrestrial Carbon Sink, Geophys. Res. Lett., 50, e2022GL102618, https://doi.org/10.1029/2022GL102618, 2023. a
Kou-Giesbrecht, S., Arora, V. K., Seiler, C., Arneth, A., Falk, S., Jain, A. K., Joos, F., Kennedy, D., Knauer, J., Sitch, S., O'Sullivan, M., Pan, N., Sun, Q., Tian, H., Vuichard, N., and Zaehle, S.: Evaluating nitrogen cycling in terrestrial biosphere models: a disconnect between the carbon and nitrogen cycles, Earth Syst. Dynam., 14, 767–795, https://doi.org/10.5194/esd-14-767-2023, 2023. a
Koven, C. D., Riley, W. J., Subin, Z. M., Tang, J. Y., Torn, M. S., Collins, W. D., Bonan, G. B., Lawrence, D. M., and Swenson, S. C.: The effect of vertically resolved soil biogeochemistry and alternate soil C and N models on C dynamics of CLM4, Biogeosciences, 10, 7109–7131, https://doi.org/10.5194/bg-10-7109-2013, 2013. a
Kowalczyk, E., Stevens, L., Law, R., Dix, M., Wang, Y., Harman, I., Haynes, K., Srbinovsky, J., Pak, B., and Ziehn, T.: The land surface model component of ACCESS: Description and impact on the simulated surface climatology, Austr. Meteorol. Oceanogr. J., 63, 65–82, 2013. a
Krasting, J. P., John, J. G., Blanton, C., McHugh, C., Nikonov, S., Radhakrishnan, A., Rand, K., Zadeh, N. T., Balaji, V., Durachta, J., Dupuis, C., Menzel, R., Robinson, T., Underwood, S., Vahlenkamp, H., Dunne, K. A., Gauthier, P. P., Ginoux, P., Griffies, S. M., Hallberg, R., Harrison, M., Hurlin, W., Malyshev, S., Naik, V., Paulot, F., Paynter, D. J., Ploshay, J., Reichl, B. G., Schwarzkopf, D. M., Seman, C. J., Silvers, L., Wyman, B., Zeng, Y., Adcroft, A., Dunne, J. P., Dussin, R., Guo, H., He, J., Held, I. M., Horowitz, L. W., Lin, P., Milly, P., Shevliakova, E., Stock, C., Winton, M., Wittenberg, A. T., Xie, Y., and Zhao, M.: NOAA-GFDL GFDL-ESM4 model output prepared for CMIP6 CMIP historical, Earth System Grid Federation [data set], https://doi.org/10.22033/ESGF/CMIP6.8597, 2018a. a, b
Krasting, J. P., John, J. G., Blanton, C., McHugh, C., Nikonov, S., Radhakrishnan, A., Rand, K., Zadeh, N. T., Balaji, V., Durachta, J., Dupuis, C., Menzel, R., Robinson, T., Underwood, S., Vahlenkamp, H., Dunne, K. A., Gauthier, P. P., Ginoux, P., Griffies, S. M., Hallberg, R., Harrison, M., Hurlin,W., Malyshev, S., Naik, V., Paulot, F., Paynter, D. J., Ploshay, J., Reichl, B. G., Schwarzkopf, D. M., Seman, C. J., Silvers, L., Wyman, B., Zeng, Y., Adcroft, A., Dunne, J. P., Dussin, R., Guo, H., He, J., Held, I. M., Horowitz, L. W., Lin, P., Milly, P., Shevliakova, E., Stock, C., Winton, M., Wittenberg, A. T., Xie, Y., and Zhao, M.: NOAA-GFDL GFDL-ESM4 model output prepared for CMIP6 CMIP esm-hist, Earth System Grid Federation [data set], https://doi.org/10.22033/ESGF/CMIP6.8522, 2018b. a
Krinner, G., Viovy, N., de Noblet-Ducoudré, N., Ogée, J., Polcher, J., Friedlingstein, P., Ciais, P., Sitch, S., and Prentice, I. C.: A dynamic global vegetation model for studies of the coupled atmosphere-biosphere system, Global Biogeochem. Cy., 19, GB1015, https://doi.org/10.1029/2003GB002199, 2005. a
Lauer, A., Eyring, V., Bellprat, O., Bock, L., Gier, B. K., Hunter, A., Lorenz, R., Pérez-Zanón, N., Righi, M., Schlund, M., Senftleben, D., Weigel, K., and Zechlau, S.: Earth System Model Evaluation Tool (ESMValTool) v2.0 – diagnostics for emergent constraints and future projections from Earth system models in CMIP, Geosci. Model Dev., 13, 4205–4228, https://doi.org/10.5194/gmd-13-4205-2020, 2020. a, b
Law, R. M., Ziehn, T., Matear, R. J., Lenton, A., Chamberlain, M. A., Stevens, L. E., Wang, Y. P., Srbinovsky, J., Bi, D., Yan, H., and Vohralik, P. F.: The carbon cycle in the Australian Community Climate and Earth System Simulator (ACCESS-ESM1) – Part 1: Model description and pre-industrial simulation, Geosci. Model Dev., 10, 2567–2590, https://doi.org/10.5194/gmd-10-2567-2017, 2017. a
Lawrence, D. M., Oleson, K. W., Flanner, M. G., Thornton, P. E., Swenson, S. C., Lawrence, P. J., Zeng, X., Yang, Z.-L., Levis, S., Sakaguchi, K., Bonan, G. B., and Slater, A. G.: Parameterization improvements and functional and structural advances in Version 4 of the Community Land Model, J. Adv. Model. Earth Sy., 3, M03001, https://doi.org/10.1029/2011ms000045, 2011. a
Lawrence, D. M., Fisher, R. A., Koven, C. D., Oleson, K. W., Swenson, S. C., Bonan, G., Collier, N., Ghimire, B., van Kampenhout, L., Kennedy, D., Kluzek, E., Lawrence, P. J., Li, F., Li, H., Lombardozzi, D., Riley, W. J., Sacks, W. J., Shi, M., Vertenstein, M., Wieder, W. R., Xu, C., Ali, A. A., Badger, A. M., Bisht, G., van den Broeke, M., Brunke, M. A., Burns, S. P., Buzan, J., Clark, M., Craig, A., Dahlin, K., Drewniak, B., Fisher, J. B., Flanner, M., Fox, A. M., Gentine, P., Hoffman, F., Keppel-Aleks, G., Knox, R., Kumar, S., Lenaerts, J., Leung, L. R., Lipscomb, W. H., Lu, Y., Pandey, A., Pelletier, J. D., Perket, J., Randerson, J. T., Ricciuto, D. M., Sanderson, B. M., Slater, A., Subin, Z. M., Tang, J., Thomas, R. Q., Val Martin, M., and Zeng, X.: The Community Land Model Version 5: Description of New Features, Benchmarking, and Impact of Forcing Uncertainty, J. Adv. Model. Earth Sy., 11, 4245–4287, https://doi.org/10.1029/2018ms001583, 2019. a, b
Lee, W.-L. and Liang, H.-C.: AS-RCEC TaiESM1.0 model output prepared for CMIP6 CMIP historical, Earth System Grid Federation [data set], https://doi.org/10.22033/ESGF/CMIP6.9755, 2020. a
Lee, E., Felzer, B. S., and Kothavala, Z.: Effects of nitrogen limitation on hydrological processes in CLM4-CN, J. Adv. Model. Earth Sy., 5, 741–754, https://doi.org/10.1002/jame.20046, 2013. a
Lee, W. L., Wang, Y. C., Shiu, C. J., Tsai, I., Tu, C. Y., Lan, Y. Y., Chen, J. P., Pan, H. L., and Hsu, H. H.: Taiwan Earth System Model Version 1: description and evaluation of mean state, Geosci. Model Dev., 13, 3887–3904, https://doi.org/10.5194/gmd-13-3887-2020, 2020. a, b
Li, X., Melaas, E., Carrillo, C. M., Ault, T., Richardson, A. D., Lawrence, P., Friedl, M. A., Seyednasrollah, B., Lawrence, D. M., and Young, A. M.: A Comparison of Land Surface Phenology in the Northern Hemisphere Derived from Satellite Remote Sensing and the Community Land Model, J. Hydrometeorol., 23, 859–873, https://doi.org/10.1175/JHM-D-21-0169.1, 2022. a
Liang, S., Cheng, J., Jia, K., Jiang, B., Liu, Q., Xiao, Z., Yao, Y., Yuan, W., Zhang, X., Zhao, X., and Zhou, J.: The Global Land Surface Satellite (GLASS) Product Suite, Bull. Am. Meteorol. Soc., 102, E323–E337, https://doi.org/10.1175/BAMS-D-18-0341.1, 2021. a, b
Lindeskog, M., Arneth, A., Bondeau, A., Waha, K., Seaquist, J., Olin, S., and Smith, B.: Implications of accounting for land use in simulations of ecosystem carbon cycling in Africa, Earth Syst. Dynam., 4, 385–407, https://doi.org/10.5194/esd-4-385-2013, 2013. a
Lovato, T. and Peano, D.: CMCC CMCC-CM2-SR5 model output prepared for CMIP6 CMIP historical, Earth System Grid Federation [data set], https://doi.org/10.22033/ESGF/CMIP6.3825, 2020. a, b
Lovato, T., Peano, D., and Butenschön, M.: CMCC CMCC-ESM2 model output prepared for CMIP6 CMIP historical, Earth System Grid Federation [data set], https://doi.org/10.22033/ESGF/CMIP6.13195, 2021. a
Lovato, T., Peano, D., Butenschon, M., Materia, S., Iovino, D., Scoccimarro, E., Fogli, P. G., Cherchi, A., Bellucci, A., Gualdi, S., Masina, S., and Navarra, A.: CMIP6 Simulations With the CMCC Earth System Model (CMCC-ESM2), J. Adv. Model. Earth Sy., 14, e2021MS002814, https://doi.org/10.1029/2021MS002814, 2022. a
Mauritsen, T., Bader, J., Becker, T., Behrens, J., Bittner, M., Brokopf, R., Brovkin, V., Claussen, M., Crueger, T., Esch, M., Fast, I., Fiedler, S., Fläschner, D., Gayler, V., Giorgetta, M., Goll, D. S., Haak, H., Hagemann, S., Hedemann, C., Hohenegger, C., Ilyina, T., Jahns, T., Jimenéz-de-la Cuesta, D., Jungclaus, J., Kleinen, T., Kloster, S., Kracher, D., Kinne, S., Kleberg, D., Lasslop, G., Kornblueh, L., Marotzke, J., Matei, D., Meraner, K., Mikolajewicz, U., Modali, K., Möbis, B., Müller, W. A., Nabel, J. E. M. S., Nam, C. C. W., Notz, D., Nyawira, S.-S., Paulsen, H., Peters, K., Pincus, R., Pohlmann, H., Pongratz, J., Popp, M., Raddatz, T. J., Rast, S., Redler, R., Reick, C. H., Rohrschneider, T., Schemann, V., Schmidt, H., Schnur, R., Schulzweida, U., Six, K. D., Stein, L., Stemmler, I., Stevens, B., von Storch, J.-S., Tian, F., Voigt, A., Vrese, P., Wieners, K.-H., Wilkenskjeld, S., Winkler, A., and Roeckner, E.: Developments in the MPI-M Earth System Model version 1.2 (MPI-ESM1.2) and Its Response to Increasing CO2, J. Adv. Model. Earth Sy., 11, 998–1038, https://doi.org/10.1029/2018ms001400, 2019. a, b, c
McInerney, F. A. and Wing, S. L.: The Paleocene-Eocene Thermal Maximum: A Perturbation of Carbon Cycle, Climate, and Biosphere with Implications for the Future, Annu. Rev. Earth Pl. Sc., 39, 489–516, https://doi.org/10.1146/annurev-earth-040610-133431, 2011. a
Meehl, G. A., Boer, G. J., Covey, C., Latif, M., and Stouffer, R. J.: The Coupled Model Intercomparison Project (CMIP), Bull. Am. Meteorol. Soc., 81, 313–318, 2000. a
Neubauer, D., Ferrachat, S., Siegenthaler-Le Drian, C., Stier, P., Partridge, D. G., Tegen, I., Bey, I., Stanelle, T., Kokkola, H., and Lohmann, U.: The global aerosol–climate model ECHAM6.3–HAM2.3 – Part 2: Cloud evaluation, aerosol radiative forcing, and climate sensitivity, Geosci. Model Dev., 12, 3609–3639, https://doi.org/10.5194/gmd-12-3609-2019, 2019a. a, b
Neubauer, D., Ferrachat, S., Siegenthaler-Le Drian, C., Stoll, J., Folini, D. S., Tegen, I., Wieners, K.-H., Mauritsen, T., Stemmler, I., Barthel, S., Bey, I., Daskalakis, N., Heinold, B., Kokkola, H., Partridge, D., Rast, S., Schmidt, H., Schutgens, N., Stanelle, T., Stier, P., Watson-Parris, D., and Lohmann, U.: HAMMOZ-Consortium MPI-ESM1.2-HAM model output prepared for CMIP6 CMIP historical, Earth System Grid Federation [data set], https://doi.org/10.22033/ESGF/CMIP6.5016, 2019b. a
Oleson, K. W., Niu, G.-Y., Yang, Z.-L., Lawrence, D. M., Thornton, P. E., Lawrence, P. J., Stöckli, R., Dickinson, R. E., Bonan, G. B., Levis, S., Dai, A., and Qian, T.: Improvements to the Community Land Model and their impact on the hydrological cycle, J. Geophys. Res.-Biogeo., 113, G01021, https://doi.org/10.1029/2007jg000563, 2008. a
Olson, J. S., Watts, J. A., and Allison, L. J.: Major world ecosystem complexes ranked by carbon in live vegetation: a database, https://www.osti.gov/biblio/6944260 (last access: 18 November 2024), 1985. a
Park, H. and Jeong, S.: Leaf area index in Earth system models: how the key variable of vegetation seasonality works in climate projections, Environ. Res. Lett., 16, 034027, https://doi.org/10.1088/1748-9326/abe2cf, 2021. a
Park, S. and Shin, J.: SNU SAM0-UNICON model output prepared for CMIP6 CMIP historical, Earth System Grid Federation [data set], https://doi.org/10.22033/ESGF/CMIP6.7789, 2019. a
Park, S., Shin, J., Kim, S., Oh, E., and Kim, Y.: Global Climate Simulated by the Seoul National University Atmosphere Model Version 0 with a Unified Convection Scheme (SAM0-UNICON), J. Clim., 32, 2917–2949, https://doi.org/10.1175/jcli-d-18-0796.1, 2019. a
Qiao, F. L., Song, Z. Y., Bao, Y., Song, Y. J., Shu, Q., Huang, C. J., and Zhao, W.: Development and evaluation of an Earth System Model with surface gravity waves, J. Geophys. Res.-Ocean., 118, 4514–4524, https://doi.org/10.1002/jgrc.20327, 2013. a
Rabin, S. S., Ward, D. S., Malyshev, S. L., Magi, B. I., Shevliakova, E., and Pacala, S. W.: A fire model with distinct crop, pasture, and non-agricultural burning: use of new data and a model-fitting algorithm for FINAL.1, Geosci. Model Dev., 11, 815–842, https://doi.org/10.5194/gmd-11-815-2018, 2018. a
Reuter, M., Buchwitz, M., Hilker, M., Heymann, J., Schneising, O., Pillai, D., Bovensmann, H., Burrows, J. P., Bösch, H., Parker, R., Butz, A., Hasekamp, O., O'Dell, C. W., Yoshida, Y., Gerbig, C., Nehrkorn, T., Deutscher, N. M., Warneke, T., Notholt, J., Hase, F., Kivi, R., Sussmann, R., Machida, T., Matsueda, H., and Sawa, Y.: Satellite-inferred European carbon sink larger than expected, Atmos. Chem. Phys., 14, 13739–13753, https://doi.org/10.5194/acp-14-13739-2014, 2014. a
Righi, M., Andela, B., Eyring, V., Lauer, A., Predoi, V., Schlund, M., Vegas-Regidor, J., Bock, L., Brötz, B., de Mora, L., Diblen, F., Dreyer, L., Drost, N., Earnshaw, P., Hassler, B., Koldunov, N., Little, B., Loosveldt Tomas, S., and Zimmermann, K.: Earth System Model Evaluation Tool (ESMValTool) v2.0 – technical overview, Geosci. Model Dev., 13, 1179–1199, https://doi.org/10.5194/gmd-13-1179-2020, 2020. a, b
Rödenbeck, C.: Estimating CO2 sources and sinks from atmospheric mixing ratio measurements using a global inversion of atmospheric transport Technical Report 6, Max Planck Institute for Biogeochemistry, Jena, http://www.bgc-jena.mpg.de/CarboScope/s/tech_report6.pdf (last access: 18 November 2024), 2005. a, b
Sanderson, B. M., Booth, B. B. B., Dunne, J., Eyring, V., Fisher, R. A., Friedlingstein, P., Gidden, M. J., Hajima, T., Jones, C. D., Jones, C. G., King, A., Koven, C. D., Lawrence, D. M., Lowe, J., Mengis, N., Peters, G. P., Rogelj, J., Smith, C., Snyder, A. C., Simpson, I. R., Swann, A. L. S., Tebaldi, C., Ilyina, T., Schleussner, C.-F., Séférian, R., Samset, B. H., van Vuuren, D., and Zaehle, S.: The need for carbon-emissions-driven climate projections in CMIP7, Geosci. Model Dev., 17, 8141–8172, https://doi.org/10.5194/gmd-17-8141-2024, 2024. a, b
Sato, H., Itoh, A., and Kohyama, T.: SEIB–DGVM: A new Dynamic Global Vegetation Model using a spatially explicit individual-based approach, Ecol. Model., 200, 279–307, https://doi.org/10.1016/j.ecolmodel.2006.09.006, 2007. a
Schlund, M., Hassler, B., Lauer, A., Andela, B., Jöckel, P., Kazeroni, R., Loosveldt Tomas, S., Medeiros, B., Predoi, V., Sénési, S., Servonnat, J., Stacke, T., Vegas-Regidor, J., Zimmermann, K., and Eyring, V.: Evaluation of native Earth system model output with ESMValTool v2.6.0, Geosci. Model Dev., 16, 315–333, https://doi.org/10.5194/gmd-16-315-2023, 2023. a
Seiler, C., Melton, J. R., Arora, V. K., Sitch, S., Friedlingstein, P., Anthoni, P., Goll, D., Jain, A. K., Joetzjer, E., Lienert, S., Lombardozzi, D., Luyssaert, S., Nabel, J. E. M. S., Tian, H., Vuichard, N., Walker, A. P., Yuan, W., and Zaehle, S.: Are Terrestrial Biosphere Models Fit for Simulating the Global Land Carbon Sink?, J. Adv. Model. Earth Sy., 14, e2021MS002946, https://doi.org/10.1029/2021MS002946, 2022. a, b
Seland, Ø., Bentsen, M., Oliviè, D. J. L., Toniazzo, T., Gjermundsen, A., Graff, L. S., Debernard, J. B., Gupta, A. K., He, Y., Kirkevåg, A., Schwinger, J., Tjiputra, J., Aas, K. S., Bethke, I., Fan, Y., Griesfeller, J., Grini, A., Guo, C., Ilicak, M., Karset, I. H. H., Landgren, O. A., Liakka, J., Moseid, K. O., Nummelin, A., Spensberger, C., Tang, H., Zhang, Z., Heinze, C., Iversen, T., and Schulz, M.: NCC NorESM2-LM model output prepared for CMIP6 CMIP esm-hist, Earth System Grid Federation [data set], https://doi.org/10.22033/ESGF/CMIP6.7924, 2019a. a
Seland, Ø., Bentsen, M., Oliviè, D. J. L., Toniazzo, T., Gjermundsen, A., Graff, L. S., Debernard, J. B., Gupta, A. K., He, Y., Kirkevåg, A., Schwinger, J., Tjiputra, J., Aas, K. S., Bethke, I., Fan, Y., Griesfeller, J., Grini, A., Guo, C., Ilicak, M., Karset, I. H. H., Landgren, O. A., Liakka, J., Moseid, K. O., Nummelin, A., Spensberger, C., Tang, H., Zhang, Z., Heinze, C., Iversen, T., and Schulz, M.: NCC NorESM2-LM model output prepared for CMIP6 CMIP historical, Earth System Grid Federation [data set], https://doi.org/10.22033/ESGF/CMIP6.8036, 2019b. a
Seland, Ø., Bentsen, M., Olivié, D., Toniazzo, T., Gjermundsen, A., Graff, L. S., Debernard, J. B., Gupta, A. K., He, Y.-C., Kirkevåg, A., Schwinger, J., Tjiputra, J., Aas, K. S., Bethke, I., Fan, Y., Griesfeller, J., Grini, A., Guo, C., Ilicak, M., Karset, I. H. H., Landgren, O., Liakka, J., Moseid, K. O., Nummelin, A., Spensberger, C., Tang, H., Zhang, Z., Heinze, C., Iversen, T., and Schulz, M.: Overview of the Norwegian Earth System Model (NorESM2) and key climate response of CMIP6 DECK, historical, and scenario simulations, Geosci. Model Dev., 13, 6165–6200, https://doi.org/10.5194/gmd-13-6165-2020, 2020. a, b
Sellar, A. A., Jones, C. G., Mulcahy, J. P., Tang, Y., Yool, A., Wiltshire, A., O'Connor, F. M., Stringer, M., Hill, R., Palmieri, J., Woodward, S., de Mora, L., Kuhlbrodt, T., Rumbold, S. T., Kelley, D. I., Ellis, R., Johnson, C. E., Walton, J., Abraham, N. L., Andrews, M. B., Andrews, T., Archibald, A. T., Berthou, S., Burke, E., Blockley, E., Carslaw, K., Dalvi, M., Edwards, J., Folberth, G. A., Gedney, N., Griffiths, P. T., Harper, A. B., Hendry, M. A., Hewitt, A. J., Johnson, B., Jones, A., Jones, C. D., Keeble, J., Liddicoat, S., Morgenstern, O., Parker, R. J., Predoi, V., Robertson, E., Siahaan, A., Smith, R. S., Swaminathan, R., Woodhouse, M. T., Zeng, G., and Zerroukat, M.: UKESM1: Description and Evaluation of the UK Earth System Model, J. Adv. Model. Earth Sy., 11, 4513–4558, https://doi.org/10.1029/2019MS001739, 2019. a, b
Shi, M., Fisher, J. B., Brzostek, E. R., and Phillips, R. P.: Carbon cost of plant nitrogen acquisition: global carbon cycle impact from an improved plant nitrogen cycle in the Community Land Model, Glob. Change Biol., 22, 1299–1314, https://doi.org/10.1111/gcb.13131, 2016. a
Smith, B., Wårlind, D., Arneth, A., Hickler, T., Leadley, P., Siltberg, J., and Zaehle, S.: Implications of incorporating N cycling and N limitations on primary production in an individual-based dynamic vegetation model, Biogeosciences, 11, 2027–2054, https://doi.org/10.5194/bg-11-2027-2014, 2014. a
Sulman, B. N., Phillips, R. P., Oishi, A. C., Shevliakova, E., and Pacala, S. W.: Microbe-driven turnover offsets mineral-mediated storage of soil carbon under elevated CO2, Nat. Clim. Change, 4, 1099–1102, https://doi.org/10.1038/nclimate2436, 2014. a
Sulman, B. N., Shevliakova, E., Brzostek, E. R., Kivlin, S. N., Malyshev, S., Menge, D. N., and Zhang, X.: Diverse Mycorrhizal Associations Enhance Terrestrial C Storage in a Global Model, Global Biogeochem. Cy., 33, 501–523, https://doi.org/10.1029/2018GB005973, 2019. a
Swart, N. C., Cole, J. N. S., Kharin, V. V., Lazare, M., Scinocca, J. F., Gillett, N. P., Anstey, J., Arora, V., Christian, J. R., Hanna, S., Jiao, Y., Lee, W. G., Majaess, F., Saenko, O. A., Seiler, C., Seinen, C., Shao, A., Sigmond, M., Solheim, L., von Salzen, K., Yang, D., and Winter, B.: The Canadian Earth System Model version 5 (CanESM5.0.3), Geosci. Model Dev., 12, 4823–4873, https://doi.org/10.5194/gmd-12-4823-2019, 2019a. a, b, c
Swart, N. C., Cole, J. N., Kharin, V. V., Lazare, M., Scinocca, J. F., Gillett, N. P., Anstey, J., Arora, V., Christian, J. R., Jiao, Y., Lee, W. G., Majaess, F., Saenko, O. A., Seiler, C., Seinen, C., Shao, A., Solheim, L., von Salzen, K., Yang, D., Winter, B., and Sigmond, M.: CCCma CanESM5 model output prepared for CMIP6 CMIP esm-hist, Earth System Grid Federation [data set], https://doi.org/10.22033/ESGF/CMIP6.3583, 2019b. a
Swart, N. C., Cole, J. N., Kharin, V. V., Lazare, M., Scinocca, J. F., Gillett, N. P., Anstey, J., Arora, V., Christian, J. R., Jiao, Y., Lee, W. G., Majaess, F., Saenko, O. A., Seiler, C., Seinen, C., Shao, A., Solheim, L., von Salzen, K., Yang, D., Winter, B., and Sigmond, M.: CCCma CanESM5-CanOE model output prepared for CMIP6 CMIP esm-hist, Earth System Grid Federation [data set], https://doi.org/10.22033/ESGF/CMIP6.10232, 2019c. a
Swart, N. C., Cole, J. N., Kharin, V. V., Lazare, M., Scinocca, J. F., Gillett, N. P., Anstey, J., Arora, V., Christian, J. R., Jiao, Y., Lee, W. G., Majaess, F., Saenko, O. A., Seiler, C., Seinen, C., Shao, A., Solheim, L., von Salzen, K., Yang, D., Winter, B., and Sigmond, M.: CCCma CanESM5-CanOE model output prepared for CMIP6 CMIP historical, Earth System Grid Federation [data set], https://doi.org/10.22033/ESGF/CMIP6.10260, 2019d. a
Swart, N. C., Cole, J. N., Kharin, V. V., Lazare, M., Scinocca, J. F., Gillett, N. P., Anstey, J., Arora, V., Christian, J. R., Jiao, Y., Lee, W. G., Majaess, F., Saenko, O. A., Seiler, C., Seinen, C., Shao, A., Solheim, L., von Salzen, K., Yang, D., Winter, B., and Sigmond, M.: CCCma CanESM5 model output prepared for CMIP6 CMIP historical, Earth System Grid Federation [data set], https://doi.org/10.22033/ESGF/CMIP6.3610, 2019e. a
Séférian, R.: CNRM-CERFACS CNRM-ESM2-1 model output prepared for CMIP6 CMIP historical, Earth System Grid Federation [data set], https://doi.org/10.22033/ESGF/CMIP6.4068, 2018. a
Séférian, R.: CNRM-CERFACS CNRM-ESM2-1 model output prepared for CMIP6 CMIP esm-hist, Earth System Grid Federation [data set], https://doi.org/10.22033/ESGF/CMIP6.4003, 2019. a
Séférian, R., Nabat, P., Michou, M., Saint-Martin, D., Voldoire, A., Colin, J., Decharme, B., Delire, C., Berthet, S., Chevallier, M., Sénési, S., Franchisteguy, L., Vial, J., Mallet, M., Joetzjer, E., Geoffroy, O., Guérémy, J.-F., Moine, M.-P., Msadek, R., Ribes, A., Rocher, M., Roehrig, R., Salas-y Mélia, D., Sanchez, E., Terray, L., Valcke, S., Waldman, R., Aumont, O., Bopp, L., Deshayes, J., Éthé, C., and Madec, G.: Evaluation of CNRM Earth System Model, CNRM-ESM2-1: Role of Earth System Processes in Present-Day and Future Climate, J. Adv. Model. Earth Sy., 11, 4182–4227, https://doi.org/10.1029/2019MS001791, 2019. a, b
Tang, Y., Rumbold, S., Ellis, R., Kelley, D., Mulcahy, J., Sellar, A., Walton, J., and Jones, C.: MOHC UKESM1.0-LL model output prepared for CMIP6 CMIP esm-hist, Earth System Grid Federation [data set], https://doi.org/10.22033/ESGF/CMIP6.5929, 2019a. a
Tang, Y., Rumbold, S., Ellis, R., Kelley, D., Mulcahy, J., Sellar, A., Walton, J., and Jones, C.: MOHC UKESM1.0-LL model output prepared for CMIP6 CMIP historical, Earth System Grid Federation [data set], https://doi.org/10.22033/ESGF/CMIP6.6113, 2019b. a
Takata, K., Emori, S., and Watanabe, T.: Development of the minimal advanced treatments of surface interaction and runoff, Glob. Planet. Change, 38, 209–222, https://doi.org/10.1016/S0921-8181(03)00030-4, 2003. a
Taylor, K. E., Stouffer, R. J., and Meehl, G. A.: An Overview of CMIP5 and the Experiment Design, Bull. Am. Meteorol. Soc., 93, 485–498, https://doi.org/10.1175/bams-d-11-00094.1, 2012. a, b
Team, T. H. D., Martin, G., Bellouin, N., Collins, W. J., Culverwell, I. D., Halloran, P. R., Hardiman, S. C., Hinton, T. J., Jones, C. D., McDonald, R. E., McLaren, A. J., O'Connor, F. M., Roberts, M. J., Rodriguez, J. M., Woodward, S., Best, M. J., Brooks, M. E., Brown, A. R., Butchart, N., Dearden, C., Derbyshire, S. H., Dharssi, I., Doutriaux-Boucher, M., Edwards, J. M., Falloon, P. D., Gedney, N., Gray, L. J., Hewitt, H. T., Hobson, M., Huddleston, M. R., Hughes, J., Ineson, S., Ingram, W. J., James, P. M., Johns, T. C., Johnson, C. E., Jones, A., Jones, C. P., Joshi, M. M., Keen, A. B., Liddicoat, S., Lock, A. P., Maidens, A. V., Manners, J. C., Milton, S. F., Rae, J. G. L., Ridley, J. K., Sellar, A., Senior, C. A., Totterdell, I. J., Verhoef, A., Vidale, P. L., and Wiltshire, A.: The HadGEM2 family of Met Office Unified Model climate configurations, Geosci. Model Dev., 4, 723–757, https://doi.org/10.5194/gmd-4-723-2011, 2011. a, b
Tegen, I., Neubauer, D., Ferrachat, S., Siegenthaler-Le Drian, C., Bey, I., Schutgens, N., Stier, P., Watson-Parris, D., Stanelle, T., Schmidt, H., Rast, S., Kokkola, H., Schultz, M., Schroeder, S., Daskalakis, N., Barthel, S., Heinold, B., and Lohmann, U.: The global aerosol–climate model ECHAM6.3–HAM2.3 – Part 1: Aerosol evaluation, Geosci. Model Dev., 12, 1643–1677, https://doi.org/10.5194/gmd-12-1643-2019, 2019. a, b
Tjiputra, J. F., Roelandt, C., Bentsen, M., Lawrence, D. M., Lorentzen, T., Schwinger, J., Seland, O., and Heinze, C.: Evaluation of the carbon cycle components in the Norwegian Earth System Model (NorESM), Geosci. Model Dev., 6, 301–325, https://doi.org/10.5194/gmd-6-301-2013, 2013. a
Todd-Brown, K. E. O., Randerson, J. T., Hopkins, F., Arora, V., Hajima, T., Jones, C., Shevliakova, E., Tjiputra, J., Volodin, E., Wu, T., Zhang, Q., and Allison, S. D.: Changes in soil organic carbon storage predicted by Earth system models during the 21st century, Biogeosciences, 11, 2341–2356, https://doi.org/10.5194/bg-11-2341-2014, 2014. a
Tuomi, M., Laiho, R., Repo, A., and Liski, J.: Wood decomposition model for boreal forests, Ecol. Model., 222, 709–718, https://doi.org/10.1016/j.ecolmodel.2010.10.025, 2011. a
UCAR: CLM technical note, https://escomp.github.io/ctsm-docs/versions/release-clm5.0/html/tech_note/ (last access: 18 November 2024), 2020. a
Varney, R. M., Chadburn, S. E., Burke, E. J., and Cox, P. M.: Evaluation of soil carbon simulation in CMIP6 Earth system models, Biogeosciences, 19, 4671–4704, https://doi.org/10.5194/bg-19-4671-2022, 2022. a, b, c, d
Varney, R. M., Chadburn, S. E., Burke, E. J., Jones, S., Wiltshire, A. J., and Cox, P. M.: Simulated responses of soil carbon to climate change in CMIP6 Earth system models: the role of false priming, Biogeosciences, 20, 3767–3790, https://doi.org/10.5194/bg-20-3767-2023, 2023. a
Varney, R. M., Friedlingstein, P., Chadburn, S. E., Burke, E. J., and Cox, P. M.: Soil carbon-concentration and carbon-climate feedbacks in CMIP6 Earth system models, Biogeosciences, 21, 2759–2776, https://doi.org/10.5194/bg-21-2759-2024, 2024. a
Verseghy, D. L.: Class – A Canadian land surface scheme for GCMS, I. Soil model, Int. J. Climatol., 11, 111–133, https://doi.org/10.1002/joc.3370110202, 1991. a
Verseghy, D. L.: The Canadian land surface scheme (CLASS): Its history and future, Atmos.-Ocean, 38, 1–13, https://doi.org/10.1080/07055900.2000.9649637, 2000. a
Verseghy, D. L., McFarlane, N. A., and Lazare, M.: Class – A Canadian land surface scheme for GCMS, II. Vegetation model and coupled runs, Int. J. Climatol., 13, 347–370, https://doi.org/10.1002/joc.3370130402, 1993. a
Volodin, E. M.: Atmosphere-ocean general circulation model with the carbon cycle, Izvestiya, Atmos. Oceanic Phys., 43, 266–280, https://doi.org/10.1134/s0001433807030024, 2007. a
Volodin, E. M., Dianskii, N. A., and Gusev, A. V.: Simulating present-day climate with the INMCM4.0 coupled model of the atmospheric and oceanic general circulations, Izvestiya, Atmos. Oceanic Phys., 46, 414–431, https://doi.org/10.1134/S000143381004002X, 2010. a
Volodin, E. M., Mortikov, E. V., Kostrykin, S. V., Galin, V. Y., Lykosov, V. N., Gritsun, A. S., Diansky, N. A., Gusev, A. V., and Yakovlev, N. G.: Simulation of modern climate with the new version of the INM RAS climate model, Izvestiya, Atmos. Oceanic Phys., 53, 142–155, https://doi.org/10.1134/S0001433817020128, 2017a. a
Volodin, E. M., Mortikov, E. V., Kostrykin, S. V., Galin, V. Y., Lykossov, V. N., Gritsun, A. S., Diansky, N. A., Gusev, A. V., and Iakovlev, N. G.: Simulation of the present-day climate with the climate model INMCM5, Clim. Dynam., 49, 3715–3734, https://doi.org/10.1007/s00382-017-3539-7, 2017b. a, b
Volodin, E. M., Mortikov, E. V., Kostrykin, S. V., Galin, V. Y., Lykossov, V. N., Gritsun, A. S., Diansky, N. A., Gusev, A. V., Iakovlev, N. G., Shestakova, A. A., and Emelina, S. V.: Simulation of the modern climate using the INM-CM48 climate model, Russ. J. Numer. Anal. M., 33, 367–374, https://doi.org/10.1515/rnam-2018-0032, 2018. a
Volodin, E., Mortikov, E., Gritsun, A., Lykossov, V., Galin, V., Diansky, N., Gusev, A., Kostrykin, S., Iakovlev, N., Shestakova, A., and Emelina, S.: INM INM-CM4-8 model output prepared for CMIP6 CMIP historical, Earth System Grid Federation [data set], https://doi.org/10.22033/ESGF/CMIP6.5069, 2019a. a
Volodin, E., Mortikov, E., Gritsun, A., Lykossov, V., Galin, V., Diansky, N., Gusev, A., Kostrykin, S., Iakovlev, N., Shestakova, A., and Emelina, S.: INM INM-CM5-0 model output prepared for CMIP6 CMIP historical, Earth System Grid Federation [data set], https://doi.org/10.22033/ESGF/CMIP6.5070, 2019b. a
Wang, S., Luo, Y., and Niu, S.: Reparameterization Required After Model Structure Changes From Carbon Only to Carbon-Nitrogen Coupling, J. Adv. Model. Earth Sy., 14, e2021MS002798, https://doi.org/10.1029/2021MS002798, 2022. a, b
Wang, Y. P., Law, R. M., and Pak, B.: A global model of carbon, nitrogen and phosphorus cycles for the terrestrial biosphere, Biogeosciences, 7, 2261–2282, https://doi.org/10.5194/bg-7-2261-2010, 2010. a
Watanabe, S., Hajima, T., Sudo, K., Nagashima, T., Takemura, T., Okajima, H., Nozawa, T., Kawase, H., Abe, M., Yokohata, T., Ise, T., Sato, H., Kato, E., Takata, K., Emori, S., and Kawamiya, M.: MIROC-ESM 2010: model description and basic results of CMIP5-20c3m experiments, Geosci. Model Dev., 4, 845–872, https://doi.org/10.5194/gmd-4-845-2011, 2011. a, b, c, d
Wei, N., Xia, J., Zhou, J., Jiang, L., Cui, E., Ping, J., and Luo, Y.: Evolution of Uncertainty in Terrestrial Carbon Storage in Earth System Models from CMIP5 to CMIP6, J. Clim., 35, 5483–5499, https://doi.org/10.1175/JCLI-D-21-0763.1, 2022. a
Weigel, K., Bock, L., Gier, B. K., Lauer, A., Righi, M., Schlund, M., Adeniyi, K., Andela, B., Arnone, E., Berg, P., Caron, L.-P., Cionni, I., Corti, S., Drost, N., Hunter, A., Lledó, L., Mohr, C. W., Paçal, A., Pérez-Zanón, N., Predoi, V., Sandstad, M., Sillmann, J., Sterl, A., Vegas-Regidor, J., von Hardenberg, J., and Eyring, V.: Earth System Model Evaluation Tool (ESMValTool) v2.0 – diagnostics for extreme events, regional and impact evaluation, and analysis of Earth system models in CMIP, Geosci. Model Dev., 14, 3159–3184, https://doi.org/10.5194/gmd-14-3159-2021, 2021. a, b
Weng, E. S., Malyshev, S., Lichstein, J. W., Farrior, C. E., Dybzinski, R., Zhang, T., Shevliakova, E., and Pacala, S. W.: Scaling from individual trees to forests in an Earth system modeling framework using a mathematically tractable model of height-structured competition, Biogeosciences, 12, 2655–2694, https://doi.org/10.5194/bg-12-2655-2015, 2015. a
Wieder, W.: Regridded Harmonized World Soil Database v1.2, ORNL Distributed Active Archive Center [data set], https://doi.org/10.3334/ORNLDAAC/1247, 2014. a, b
Wieder, W. R., Lawrence, D. M., Fisher, R. A., Bonan, G. B., Cheng, S. J., Goodale, C. L., Grandy, A. S., Koven, C. D., Lombardozzi, D. L., Oleson, K. W., and Thomas, R. Q.: Beyond Static Benchmarking: Using Experimental Manipulations to Evaluate Land Model Assumptions, Global Biogeochem. Cy., 33, 1289–1309, https://doi.org/10.1029/2018gb006141, 2019. a
Wieners, K.-H., Giorgetta, M., Jungclaus, J., Reick, C., Esch, M., Bittner, M., Legutke, S., Schupfner, M., Wachsmann, F., Gayler, V., Haak, H., de Vrese, P., Raddatz, T., Mauritsen, T., von Storch, J.-S., Behrens, J., Brovkin, V., Claussen, M., Crueger, T., Fast, I., Fiedler, S., Hagemann, S., Hohenegger, C., Jahns, T., Kloster, S., Kinne, S., Lasslop, G., Kornblueh, L., Marotzke, J., Matei, D., Meraner, K., Mikolajewicz, U., Modali, K., Müller, W., Nabel, J., Notz, D., Peters-von Gehlen, K., Pincus, R., Pohlmann, H., Pongratz, J., Rast, S., Schmidt, H., Schnur, R., Schulzweida, U., Six, K., Stevens, B., Voigt, A., and Roeckner, E.: MPI-M MPI-ESM1.2-LR model output prepared for CMIP6 CMIP historical, Earth System Grid Federation [data set], https://doi.org/10.22033/ESGF/CMIP6.6595, 2019a. a, b
Wieners, K.-H., Giorgetta, M., Jungclaus, J., Reick, C., Esch, M., Bittner, M., Legutke, S., Schupfner, M., Wachsmann, F., Gayler, V., Haak, H., de Vrese, P., Raddatz, T., Mauritsen, T., von Storch, J.-S., Behrens, J., Brovkin, V., Claussen, M., Crueger, T., Fast, I., Fiedler, S., Hagemann, S., Hohenegger, C., Jahns, T., Kloster, S., Kinne, S., Lasslop, G., Kornblueh, L., Marotzke, J., Matei, D., Meraner, K., Mikolajewicz, U., Modali, K., Müller, W., Nabel, J., Notz, D., Peters-von Gehlen, K., Pincus, R., Pohlmann, H., Pongratz, J., Rast, S., Schmidt, H., Schnur, R., Schulzweida, U., Six, K., Stevens, B., Voigt, A., and Roeckner, E.: MPI-M MPI-ESM1.2-LR model output prepared for CMIP6 CMIP esm-hist, Earth System Grid Federation [dataset], https://doi.org/10.22033/ESGF/CMIP6.6545, 2019b. a
Wiltshire, A. J., Burke, E. J., Chadburn, S. E., Jones, C. D., Cox, P. M., Davies-Barnard, T., Friedlingstein, P., Harper, A. B., Liddicoat, S., Sitch, S., and Zaehle, S.: JULES-CN: a coupled terrestrial carbon–nitrogen scheme (JULES vn5.1), Geosci. Model Dev., 14, 2161–2186, https://doi.org/10.5194/gmd-14-2161-2021, 2021. a
Xiao, Z., Liang, S., and Jiang, B.: Evaluation of four long time-series global leaf area index products, Agr. Forest Meteorol., 246, 218–230, https://doi.org/10.1016/j.agrformet.2017.06.016, 2017. a, b
Yang, X., Thornton, P., Ricciuto, D., Wang, Y., and Hoffman, F.: Global evaluation of terrestrial biogeochemistry in the Energy Exascale Earth System Model (E3SM) and the role of the phosphorus cycle in the historical terrestrial carbon balance, Biogeosciences, 20, 2813–2836, https://doi.org/10.5194/bg-20-2813-2023, 2023. a
Yuan, W., Liu, S., Zhou, G., Zhou, G., Tieszen, L. L., Baldocchi, D., Bernhofer, C., Gholz, H., Goldstein, A. H., Goulden, M. L., Hollinger, D. Y., Hu, Y., Law, B. E., Stoy, P. C., Vesala, T., and Wofsy, S. C.: Deriving a light use efficiency model from eddy covariance flux data for predicting daily gross primary production across biomes, Agr. Forest Meteorol., 143, 189–207, https://doi.org/10.1016/j.agrformet.2006.12.001, 2007. a
Yukimoto, S., Yoshimura, H., Hosaka, M., Sakami, T., Tsujino, H., Hirabara, M., Tanaka, T. Y., Deushi, M., Obata, A., Nakano, H., Adachi, Y., Shindo, E., Yabu, S., Ose, T., and Kitoh, A.: Meteorological Research Institute-Earth System Model Version 1 (MRI-ESM1), Tech. Rep., 64, 88, https://doi.org/10.11483/mritechrepo.64, 2011. a
Yukimoto, S., Kawai, H., Koshiro, T., Oshima, N., Yoshida, K., Urakawa, S., Tsujino, H., Deushi, M., Tanaka, T., Hosaka, M., Yabu, S., Yoshimura, H., Shindo, E., Mizuta, R., Obata, A., Adachi, Y., and Ishii, M.: The Meteorological Research Institute Earth System Model Version 2.0, MRI-ESM2.0: Description and Basic Evaluation of the Physical Component, J. Meteorol. Soc. Jpn. Ser. II, 97, 931–965, https://doi.org/10.2151/jmsj.2019-051, 2019a. a
Yukimoto, S., Koshiro, T., Kawai, H., Oshima, N., Yoshida, K., Urakawa, S., Tsujino, H., Deushi, M., Tanaka, T., Hosaka, M., Yoshimura, H., Shindo, E., Mizuta, R., Ishii, M., Obata, A., and Adachi, Y.: MRI MRI-ESM2.0 model output prepared for CMIP6 CMIP esm-hist, Earth System Grid Federation [data set], https://doi.org/10.22033/ESGF/CMIP6.6807, 2019b. a
Yukimoto, S., Koshiro, T., Kawai, H., Oshima, N., Yoshida, K., Urakawa, S., Tsujino, H., Deushi, M., Tanaka, T., Hosaka, M., Yoshimura, H., Shindo, E., Mizuta, R., Ishii, M., Obata, A., and Adachi, Y.: MRI MRI-ESM2.0 model output prepared for CMIP6 CMIP historical, Earth System Grid Federation [data set], https://doi.org/10.22033/ESGF/CMIP6.6842, 2019c. a, b
Zaehle, S., Jones, C. D., Houlton, B., Lamarque, J.-F., and Robertson, E.: Nitrogen Availability Reduces CMIP5 Projections of Twenty-First-Century Land Carbon Uptake, J. Clim., 28, 2494–2511, https://doi.org/10.1175/JCLI-D-13-00776.1, 2015. a
Zhang, Q., Pitman, A. J., Wang, Y. P., Dai, Y. J., and Lawrence, P. J.: The impact of nitrogen and phosphorous limitation on the estimated terrestrial carbon balance and warming of land use change over the last 156 yr, Earth Syst. Dynam., 4, 333–345, https://doi.org/10.5194/esd-4-333-2013, 2013. a
Zhu, Z., Bi, J., Pan, Y., Ganguly, S., Anav, A., Xu, L., Samanta, A., Piao, S., Nemani, R., and Myneni, R.: Global Data Sets of Vegetation Leaf Area Index (LAI)3g and Fraction of Photosynthetically Active Radiation (FPAR)3g Derived from Global Inventory Modeling and Mapping Studies (GIMMS) Normalized Difference Vegetation Index (NDVI3g) for the Period 1981 to 2011, Remote Sens., 5, 927–948, https://doi.org/10.3390/rs5020927, 2013. a, b
Ziehn, T., Lenton, A., Law, R. M., Matear, R. J., and Chamberlain, M. A.: The carbon cycle in the Australian Community Climate and Earth System Simulator (ACCESS-ESM1) – Part 2: Historical simulations, Geosci. Model Dev., 10, 2591–2614, https://doi.org/10.5194/gmd-10-2591-2017, 2017. a, b, c
Ziehn, T., Chamberlain, M., Lenton, A., Law, R., Bodman, R., Dix, M., Wang, Y., Dobrohotoff, P., Srbinovsky, J., Stevens, L., Vohralik, P., Mackallah, C., Sullivan, A., O'Farrell, S., and Druken, K.: CSIRO ACCESS-ESM1.5 model output prepared for CMIP6 CMIP esm-hist, Earth System Grid Federation [data set], https://doi.org/10.22033/ESGF/CMIP6.4242, 2019a. a
Ziehn, T., Chamberlain, M., Lenton, A., Law, R., Bodman, R., Dix, M., Wang, Y., Dobrohotoff, P., Srbinovsky, J., Stevens, L., Vohralik, P., Mackallah, C., Sullivan, A., O'Farrell, S., and Druken, K.: CSIRO ACCESS-ESM1.5 model output prepared for CMIP6 CMIP historical, Earth System Grid Federation [data set], https://doi.org/10.22033/ESGF/CMIP6.4272, 2019b. a
Ziehn, T., Chamberlain, M. A., Law, R. M., Lenton, A., Bodman, R. W., Dix, M., Stevens, L., Wang, Y.-P., and Srbinovsky, J.: The Australian Earth System Model: ACCESS-ESM1.5, J. Southern Hemisph. Earth Syst. Sci., 70, 193–214, https://doi.org/10.1071/es19035, 2020. a, b