the Creative Commons Attribution 4.0 License.
the Creative Commons Attribution 4.0 License.
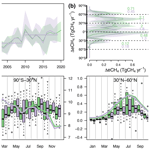
Ensemble estimates of global wetland methane emissions over 2000–2020
Benjamin Poulter
Joe R. Melton
William J. Riley
George H. Allen
David J. Beerling
Philippe Bousquet
Josep G. Canadell
Etienne Fluet-Chouinard
Philippe Ciais
Nicola Gedney
Peter O. Hopcroft
Akihiko Ito
Robert B. Jackson
Atul K. Jain
Katherine Jensen
Fortunat Joos
Thomas Kleinen
Sara H. Knox
Tingting Li
Xin Li
Xiangyu Liu
Kyle McDonald
Gavin McNicol
Paul A. Miller
Jurek Müller
Prabir K. Patra
Changhui Peng
Shushi Peng
Zhangcai Qin
Ryan M. Riggs
Marielle Saunois
Hanqin Tian
Xiaoming Xu
Yuanzhi Yao
Wenxin Zhang
Qing Zhu
Qiuan Zhu
Qianlai Zhuang
Due to ongoing climate change, methane (CH4) emissions from vegetated wetlands are projected to increase during the 21st century, challenging climate mitigation efforts aimed at limiting global warming. However, despite reports of rising emission trends, a comprehensive evaluation and attribution of recent changes remains limited. Here we assessed global wetland CH4 emissions from 2000–2020 based on an ensemble of 16 process-based wetland models. Our results estimated global average wetland CH4 emissions at 158 ± 24 (mean ± 1σ) Tg CH4 yr−1 over a total annual average wetland area of 8.0 ± 2.0×106 km2 for the period 2010–2020, with an average increase of 6–7 Tg CH4 yr−1 in 2010–2019 compared to the average for 2000–2009. The increases in the four latitudinal bands of 90–30° S, 30° S–30° N, 30–60° N, and 60–90° N were 0.1–0.2, 3.6–3.7, 1.8–2.4, and 0.6–0.8 Tg CH4 yr−1, respectively, over the 2 decades. The modeled CH4 sensitivities to temperature show reasonable consistency with eddy-covariance-based measurements from 34 sites. Rising temperature was the primary driver of the increase, while precipitation and rising atmospheric CO2 concentrations played secondary roles with high levels of uncertainty. These modeled results suggest that climate change is driving increased wetland CH4 emissions and that direct and sustained measurements are needed to monitor developments.
- Article
(2206 KB) - Full-text XML
-
Supplement
(3441 KB) - BibTeX
- EndNote
Wetlands are the largest single source in the global methane (CH4) budget, representing ∼ 25–35 % of the total combined natural and anthropogenic sources (Kirschke et al., 2013; Saunois et al., 2016, 2020), with an uncertainty range of 100–230 Tg CH4 yr−1 (Cao et al., 1996; Gedney et al., 2004; Bousquet et al., 2006; Petrescu et al., 2010; Spahni et al., 2011; Melton et al., 2013; Bridgham et al., 2013; Bloom et al., 2017; Poulter et al., 2017). Covering 8 %–10 % of the global land surface (Zhang et al., 2021a), wetland area is sensitive to climate variations (Zhang et al., 2018; Zhu et al., 2017). Over the last deglaciation, wetlands played an important role in driving the rise of atmospheric CH4 concentrations (Hopcroft et al., 2017; Nisbet et al., 2023; Kleinen et al., 2023). In recent decades, wetlands have experienced unprecedented and ongoing changes, including continuous thawing of permafrost (Natali et al., 2019; Treat et al., 2018), land-use change (Fluet-Chouinard et al., 2023), a lengthening of the growing season in the Arctic (Arndt et al., 2019), and expansion in tropical areas due to enhanced precipitation (Fleischmann, 2023). Recent evidence from in situ measurements (Rößger et al., 2022), data-driven estimates (Yuan et al., 2024; Ying et al., 2024), and satellite observations (Feng et al., 2022) suggests that these ongoing changes could enhance wetland CH4 emissions and thus affect the trajectory of the atmospheric CH4 concentration. Furthermore, atmospheric δ13C-CH4 records also show a trend toward increased depletion since the late 2000s (Lan et al., 2021; Nisbet et al., 2019), indicating that isotopically light biogenic sources, such as wetlands (Basu et al., 2022; Feng et al., 2022), agriculture, and waste (Schaefer et al., 2016; Zhang et al., 2021b), have become dominant contributors to the rise in atmospheric CH4. Current estimates of wetland CH4 emissions (hereafter denoted as eCH4) in response to climate change are projected to increase by up to 15 %–30 % by 2050 (Koffi et al., 2020; Zhang et al., 2017), accounting for 25 %–40 % of the pledged reduction in anthropogenic emissions (Shindell et al., 2019). These trends and projections suggest that the emerging wetland CH4 climate feedback that influences atmospheric CH4 concentration requires a better understanding of long-term changes in eCH4.
Directly diagnosing the variations and trends of eCH4 at large scales is challenging. Site-level measurements, such as those from chamber and eddy covariance techniques, are useful for identifying underlying mechanisms and monitoring CH4 fluxes at the landscape scale but are difficult to upscale due to large uncertainties in extrapolation and the high spatial heterogeneity of wetland CH4 fluxes (Chu et al., 2021; Kuhn et al., 2021). Interpreting eCH4 using satellite observations and inversions of atmospheric concentration data is also subject to uncertainties in anthropogenic sources, other natural sources, atmospheric chemistry, and model errors associated with atmospheric transport (Gatti et al., 2021; Gloor et al., 2021; Palmer et al., 2022; Patra et al., 2011; Zhang et al., 2021c). Global wetland models, integrated within land biosphere models, can serve to bridge our understanding of wetland CH4 processes and diagnosing wetland CH4 dynamics at large scales (Melton et al., 2013; Wania et al., 2013). These models provide mechanistic explanations for the causes of changes in eCH4 dynamics. Furthermore, recent advances in wetland models (Arora et al., 2018; Kaiser et al., 2017; Shu et al., 2020; Grant, 2017; Chang et al., 2020) show significant potential for improving our understanding of eCH4 through the incorporation of complex biogeochemical processes.
Current studies have reached various conclusions on the change in eCH4 over the last decades. Studies based on single biogeochemical models (Zhang et al., 2018; Zhu et al., 2017) suggest a significant increase in eCH4 from 2000–2006 to 2007–2017, while atmospheric inversions (Zhang et al., 2021c; Yin et al., 2021; Basu et al., 2022; Feng et al., 2022) suggested even higher rate increases from 2 to 3 Tg CH4 yr−1 yr−1 during the post-2010 period. Poulter et al. (2017) reported no significant change between the 2000–2006 and 2007–2012 periods based on an ensemble of wetland models, while Saunois et al. (2020) show a slight increase (∼ 2 Tg CH4 yr−1) on average for 2007–2017 compared to the 2000–2006 level using a large set of wetland CH4 models. However, these models demonstrate considerable differences in estimated eCH4, both spatially and temporally (Ma et al., 2021; Parker et al., 2020; Chang et al., 2023), primarily due to the sensitivity of their estimations to the wetland areal extent, the implemented biogeochemical structures, and parameterizations. The multi-model ensemble approach is applied to increase the skill, reliability, and consistency of model forecasts, potentially offsetting individual model errors (Schaefer et al., 2012). However, a recent study (Chang et al., 2023) found that downsampling atmospheric inversion and wetland model CH4 predictions based on a comparison to eddy covariance data did not reduce uncertainty in global eCH4 estimates. Therefore, it has become necessary to thoroughly evaluate the performance of these models using the most recent generation of wetland models against an increasingly dense network of observations (Delwiche et al., 2021; Knox et al., 2019) from eddy covariance sites.
Here we conducted ensemble simulations of 16 wetland biogeochemical models following a common modeling protocol to provide monthly integrated global eCH4 for the period of 2000–2020, as part of the Global Carbon Project's methane budget activity. The inundation dynamics of each model were simulated using a model-specific prognostic hydrological modeling approach as well as a set of diagnostic satellite-driven simulations. A set of factorial simulations were carried out to isolate the effects of temperature, precipitation, and rising atmospheric CO2 concentration. The modeled temperature sensitivity was evaluated against the global eddy covariance database FLUXNET-CH4 (Delwiche et al., 2021; Knox et al., 2019) and a data-driven global wetland CH4 upscaling dataset UpCH4 (McNicol et al., 2023) based on FLUXNET-CH4. In addition, we examined the changes in eCH4 for the year 2020, which was characterized as an extremely warm and wet year with the highest growth rate of atmospheric CH4 observed over the study period.
2.1 Wetland model ensemble
Sixteen wetland models participated in the ensemble simulations (Table S1). Wetland CH4 models can be generally described as functions describing the biogeochemical processes that control CH4 production and oxidation through methanogenesis and methanotrophy, as well as the biophysical processes that regulate CH4 transport from the soil to the atmosphere (Table S1). Methanogenesis in the models is linked to different proxies (e.g., carbon substrate, heterotrophic respiration, net primary production) with a wide range of model complexity – more sophisticated models include wetland plant functional types (PFTs) and explicitly simulate the processes of CH4 production, consumption, and transport, while the simplified models use generalized empirical equations to simulate net fluxes without explicitly calculating individual components of the CH4 flux.
Wetlands were defined as naturally vegetated forested and non-forested ecosystems with saturated/inundated areas, excluding coastal wetlands; cultivated wetlands such as rice paddies; and open water systems such as rivers, lakes, ponds, and reservoirs. A prognostic wetland inundation scheme and a diagnostic wetland dataset (Wetland Area and Dynamics for Methane Modeling (WAD2M v2); Zhang et al., 2021a) were applied to identify the wetland areal dynamics. The prognostic wetland areal dynamics were independently determined by each model's hydrological modules, which use water table depth or soil moisture, combined with subgrid topographic conditions, to determine saturated areas within a land surface grid cell (Zhang et al., 2016; Xi et al., 2022). Among the participating models, there was a large variation in complexity and in the level of comprehensiveness with which wetland extents were characterized. The modules for simulating inundation ranged from simplified TOPMODEL approaches to more sophisticated representations of water table variation, with the estimated magnitude being influenced by the hydrologic schemes utilized and the sensitivities to precipitation. The prognostic modeled wetland extent showed large variability in estimated magnitude but was consistent with satellite-based inundation products in predicting different phases of inundation (Xi et al., 2022; Zhang, et al., 2021a). The ensemble mean of the modeled wetland extent is close to 7.5×106 km2 as estimated by WAD2M but higher than the 4.6×106 km2 by the satellite-based product Global Surface Water Extent and Dynamics version 2 (GIEMS-2; Prigent et al., 2020). The modeled temporal variations in wetland areas show high correlations with satellite-based products for temperate regions and high latitudes (Fig. S1), except in the tropics. The limited agreement in the tropics may be due to the influence of aerosols and clouds on satellite-based measurements, as well as the process-based model's performance limitations in representing wetland areas. The diagnostic runs are exclusively used for temperature dependence calculations due to a discontinuity issue in WAD2Mv2 over a few tropical hotspots, which affect a subset of wetland models that are particularly sensitive to inundation in the hotspots.
2.2 Modeling protocol and simulation setups
The modeling protocol aimed to provide wetland CH4 fluxes and quantify the associated uncertainties arising from model differences, meteorological forcing, and wetland extent dynamics. To quantify meteorological forcing uncertainty, we used two climate inputs: a ground-based monthly climate dataset from the Climatic Research Unit (CRU) (Harris et al., 2014) up to 2020 and a harmonized daily dataset from the Global Soil Wetness Project Phase 3 (GSWP3-W5E5) through the year 2019, which is a multiple-source-based daily dataset (Cucchi et al., 2020; Dirmeyer et al., 2006) used in the Inter-Sectoral Impact Model Intercomparison Project 3a (ISIMIP3a). For models that require 6-hourly meteorological forcings, a temporal-interpolation dataset (CRU-JRA) was applied based on the Japanese Reanalysis Agency (JRA55) aligned with CRU. The atmospheric CO2 concentration values for 1861–2020 were obtained from the CMIP6 experimental protocol (Meinshausen et al., 2017). Ancillary data, such as soil texture and CH4-related parameter sets, used model-specific inputs. All the models were run in “natural vegetation” mode without the transient effects of land-use or land-cover change. Methane oxidation in wetland soils was implicitly included in the estimate, but the upland oxidative sink was not included as it was not part of the net wetland emission calculations. Models included the spin-up period to pre-industrial conditions, assuming net ecosystem exchange equilibrium before 1860, by recycling fixed CO2 concentrations (1860 level of 286.42 ppm) and meteorology (1901–1920).
2.3 FLUXNET-CH4 and the machine learning-based upscaling product UpCH4
FLUXNET-CH4 is the first global dataset of CH4 eddy covariance measurements that includes ∼ 80 sites globally, including different wetland types such as peatlands (e.g., bog, fen), mineral wetlands (e.g., marsh, swamp), and rice paddies. For this study, a subset of natural freshwater wetland sites was selected for the analysis. All the eddy covariance measurements used in this study were gap-filled daily and total fluxes were filled using an artificial neural network (ANN) approach (Knox et al., 2019). In addition, a data-driven gridded dataset (UpCH4; McNicol et al., 2023) for 2001–2018, which is based on 119 site-years of CH4 fluxes from the FLUXNET-CH4 dataset, was applied in the comparison. This dataset used a random forest model to upscale ground-based eddy covariance CH4 flux data and was then forced with globally gridded predictor data and two wetland extent products to predict wetland CH4 emissions. The predictors included data sources from climate, biometeorological, and soil properties.
2.4 Time series decomposition and statistical analyses
To attribute the time series of global wetland CH4 emissions to what we consider the dominant drivers of change (i.e., temperature, precipitation, and CO2 concentration), we applied a multiple regression approach (Piao et al., 2013) to estimate the parameters of global wetland CH4 sensitivity to climate drivers using the following equation:
where y is the global annual total wetland CH4 emission of each model from the transient run or from the observation-based upscaling dataset UpCH4, and Tmp, Pre, and CO2 are the mean annual temperature, total annual precipitation, and mean atmospheric CO2 concentration for that year, respectively. γ, δ, β, and c are regression coefficients, and ε is the residual error term. The regression coefficients were calculated using a maximum likelihood estimate. Changes in other meteorological forcings may also influence the estimation of y. These confounding drivers, such as solar radiation and wind speed, although they are considered to have minor impacts on the variations of eCH4, were implicitly accounted for in the regression coefficients.
2.5 Model factorial experiment
To further separate the contribution of different controls on the change in methane emissions (ΔeCH4) by climate variations and rising CO2, we used a subset of four models that conducted factorial experimental simulations by holding each factor constant during part of the transient runs. This subset of the wetland models (i.e., four wetland models: ELM-ECA, LPJ-wsl, SDGVM, and VISIT) performed a set of factorial simulations to specifically attribute the effect of temperature, precipitation, and rising CO2 concentration on wetland CH4 fluxes with the climatology of 2000–2006 for 2007–2020. The simulations were performed by running the model while keeping one factor constant at a time to estimate the contribution of each component to the total range of variations (Table S2). For these factorial simulations, we evaluated the annual amplitude of wetland eCH4 as a relative percentage change to minimize the impacts of different modeling implementation choices, such as different input variables among models. The effect of the total changes on the relative change in amplitude was represented by the difference between the transient (one factor is time-varying) and baseline (static at 2000–2006 levels) runs. For simplicity, the relative contribution of a single driver to eCH4 variations was quantified as the transient run minus the specific control run. To calculate the contribution of each driver using the subset of the models, we calculated weighting factors per year across the models, with lower bias resulting in higher weight relative to the full ensemble mean using an inverse function.
2.6 Temperature dependence calculation
To further evaluate the response of eCH4 to rising temperatures, we calculated the modeled seasonal eCH4 temperature dependence, referred to as the apparent Q10 metric at the locations of 34 FLUXNET-CH4 sites. This seasonal Q10 differs from the intrinsic Q10 prescribed in the parameterization of respiratory processes in each model. Here it represents the overall response of eCH4 along geographic temperature gradients. The apparent Q10 is defined as eCH4 sensitivity to temperature change. We calculated apparent Q10 based on CH4 emitting strength over a standard wetland area, which was calculated as the CH4 fluxes divided by inundated area on a per-pixel basis to exclude the effect of inundation dynamics. To derive the temperature dependence of eCH4 at the soil or ecosystem level, we applied the following equation:
where R(i) is the net wetland flux at the location of site i, Rb(i) is the basal net CH4 flux at the reference temperature Tref, and T(i) is ambient temperature. The parameters Q10, Γ=10°, and Tref=15° are all time-independent constants. The Q10 acting on specific timescales can be obtained from eCH4 at corresponding specific timescales (i.e., seasonal total and annual total) by fitting an exponential regression with modeled eCH4 and air temperature from CRU or GSWP3-W5E5. To quantify the uncertainty in observed apparent Q10, we employed 1000 sets of resampled FLUXNET-CH4 observations generated based on a Gaussian distribution. The uncertainty range in measured seasonal mean CH4 fluxes was determined by aggregating the uncertainty of daily total fluxes obtained through ANN gap filling.
3.1 Changes in eCH4 during the period 2000–2020
The multi-model ensemble based on the prognostic inundation schemes shows that the average annual global eCH4 over the period 2000–2020 was 156 ± 24 Tg CH4 yr−1 (mean ± 1σ). The average annual eCH4 increased from 153 ± 23 Tg CH4 yr−1 during 2000–2009 to 158 ± 24 Tg CH4 yr−1 during 2010–2020. And 15 out of 22 model simulations show significant positive linear trends (p<0.01), with an ensemble mean increase rate of 0.6 ± 0.3 Tg CH4 yr−1 yr−1 over 2000–2020 (Fig. 1a; Table 1; Fig. S2). Differences in total annual emissions between the two sets of simulations driven by two different climate datasets (CRU and GSWP3-W5E5) agree well in the magnitude of the annual anomalies. Notable eCH4 variations to climate events were observed, such as the rise during the 2010 La Niña (+5.2 Tg CH4 yr−1) and the decline during the 2015 El Niño (−4.6 Tg CH4 yr−1) after removing the positive linear trends. The multi-model ensemble wetland eCH4 response to climate events is consistent with those reported by earlier studies (Zhang et al., 2018; Zhu et al., 2017) using single wetland models, indicating a modulation of the phase of eCH4 anomaly (ΔeCH4) by the El Niño–Southern Oscillation. The model ensemble demonstrates a consistent increase in interannual variability (IAV) in ΔeCH4 from 3.6 ± 1.6 Tg CH4 yr−1 during 2000–2009 to 4.7 ± 1.5 Tg CH4 yr−1 during 2010–2020, suggesting a potential increase in eCH4 variability under climate change.
The models consistently show that 2020 is the strongest positive anomaly year during 2000–2020, with a net increase of 2 [−2, 7] Tg CH4 yr−1 (mean [min, max]) in 2020 compared to 2019. This positive anomaly in 2020 (Table 1) is broadly consistent with a recent study (Peng et al., 2022) that reported 6 ± 2.3 Tg CH4 yr−1 based on simulations of two bottom-up models with different climate datasets. The discrepancy in estimated magnitude between the Peng et al. (2022) and our results is partly due to the parameterizations of the CH4 module that causes lower annual magnitude in this study (∼ 162 ± 23 Tg CH4 yr−1 in 2020) compared to the Peng et al. (2022) study (177 ± 31 Tg CH4 yr−1 in 2020). Additionally, the precipitation inputs in the climate forcing used in this study show a lower positive anomaly (∼ of 20 mm yr−1 in CRU over global wetland) in precipitation in 2020 compared to the reanalysis-based estimates (∼ 40–117 mm yr−1) over global wetlands used in the study by Peng et al. (2022), which leads to lower estimates of wetland area and consequently lower emissions in this study. Moreover, our model ensemble does not indicate a strong increase (−0.2 [−1.5–0.7] Tg CH4 yr−1) in eCH4 in Africa in 2020. This contrasts with recent atmospheric inversions (Feng et al., 2023; Qu et al., 2022), which suggest a large increase of 11–17 Tg CH4 yr−1 above 2019 levels in African CH4 emissions for 2020. The estimated increase from these inversions is equivalent to 55 %–85 % of total eCH4 in Africa during 2010–2019 in our study (Fig. 2). These discrepancies highlight the need for further studies to investigate the differences between these two approaches, including uncertainty in climate inputs in process-based bottom-up models and partitioning different sources in atmospheric inversions.
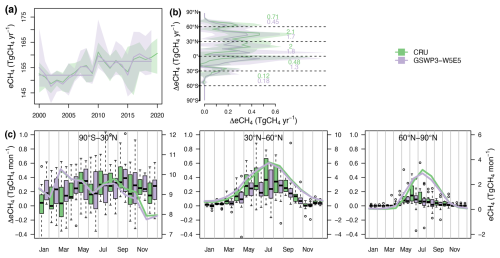
Figure 1Simulated global wetland CH4 emissions from the model ensemble for 2000–2020. (a) Time series of annual total emissions during 2000–2020, with the shaded area representing the range between minimum and maximum modeled emissions. The horizontal lines represent the ensemble means of 2000–2009 (152 Tg CH4 yr−1) and 2010–2019 (158 Tg CH4 yr−1), respectively. (b) Latitudinal gradient of eCH4 difference (ΔeCH4), with the mean annual total ΔeCH4 for each of the 30° latitude bins from the two sets of simulations shown. The change is calculated relative to the mean of the 2000–2009 level from the two sets of simulations with prognostic wetland emission models grouped by different climate datasets, CRU and GSWP3-W5E5. (c) Boxplots of mean seasonal ΔeCH4 for the three regions. The central mark and the bottom and top edges of the box indicate the median and the 25th and 75th percentiles of the ensemble, respectively. The colored lines represent the average seasonal cycle of 2000–2009 from the simulations grouped by two climate datasets, CRU and GSWP3-W5E5.
There were widespread net increases in eCH4 across all latitudinal bands during 2010–2020, compared to the average of 2000–2009, with the largest magnitudes occurring in the 90° S–30° N bands (there are relatively few wetlands in the southern extratropics of 90–30° S, contributing 0.1–0.2 Tg CH4 yr−1) and temperate regions (30–60° N) (Fig. 1b). The annual magnitude of eCH4 increased by 3.7–3.8, 1.8–2.4, and 0.6–0.8 Tg CH4 yr−1 in the tropical, temperate, and Arctic wetlands, respectively. The tropics have experienced the largest increases in annual total emissions with an increase of 3 % relative to 2000–2009 (Table 1). This finding is aligned with the results of several recent atmospheric inversions (Basu et al., 2022; Feng et al., 2022; Lan et al., 2021) using satellite observations and/or isotopic measurements that suggest a large increase in microbial emissions for the post-2007 period in the tropics. While the increase in annual total emissions from temperate wetlands is lower than that from the tropics, they nevertheless show a larger relative increase of 5 %–8 % compared to 2000–2009. Arctic wetlands also show an increased rate of 5 %–7 % relative to the same period.
The increase in eCH4 occurs in parallel with differing patterns of enhanced seasonal cycles between tropical and extratropical wetlands (30–90° N) (Fig. 1c). In temperate and Arctic wetlands, the majority of the increase in emissions (60 %–92 %) occurred primarily during the growing season (May–October). Specifically, increases in Arctic wetlands occurred during the early growing season (May–July), aligning with findings from a data-driven estimate (Yuan et al., 2024) and a long-term eddy-covariance-based study (Rößger et al., 2022) that observed early-growing-season increases in eCH4 due to continuous warming in a Siberian wetland. In contrast, the increase in emissions within the 90° S–30° N band exhibited relatively minor seasonal variations throughout the year, with the May–October period accounting for a 24 % greater increase in ΔeCH4 compared to the November–April period (Fig. S3).
3.2 Spatial distribution of eCH4
A few key regions contribute significantly to global emissions (Fig. 2a, c). These regions are mainly floodplains located along major river basins such as the Amazon, Ganges, Mississippi, and Yangtze; tropical peatlands in the Congo and Southeastern Asia; and high-latitude peatlands in the Hudson Bay Lowland (HBL) and West Siberian Lowland (WSL). However, inter-model variabilities in eCH4 reveal varying levels of spatial agreement between models, with the largest discrepancies coming from South America and Africa. South America is one of the largest contributors to the global total eCH4. Still, the net change in that region shows only a moderate increase, with diverging trends within the Amazon Basin during the 2010s (Fig. 2b, d). The uncertain temporal trends are consistent with a long-term, large-scale atmospheric inversion based on airborne campaigns (Basso et al., 2021). South Asia and Africa are among the regions with the largest increases in the tropics, next to North America, but have high uncertainty with a lower level of agreement among the models (Fig. S4). The model ensemble shows that northwestern South Asia has a significant percentage increase in eCH4 during 2010–2019 relative to its average levels from 2000–2009, suggesting a possible high sensitivity of eCH4 to climate change in this region.
The comparison with previous estimates from bottom-up approaches and top-down atmospheric inversions (Table S3) suggests that the model ensemble mean generally captures well the spatial distribution of annual eCH4, with a potential underestimation for a few methane hotspots (Fig. S5). The model ensemble means for the Amazon Basin, HBL, and WSL show good agreement with atmospheric inversions (Bergamaschi et al., 2013; Pickett-Heaps et al., 2011; Ringeval et al., 2014; Tunnicliffe et al., 2020; Wilson et al., 2016, 2021) and bottom-up modeling estimates (Bansal et al., 2023; Bloom et al., 2017; Bohn et al., 2015), with relatively low uncertainty. The model ensemble highlights WSL and HBL as CH4 hotspots in the high latitudes, with good agreements of annual magnitudes with atmospheric inversions and in situ observations (Bohn et al., 2015; Glagolev et al., 2011; Pickett-Heaps et al., 2011), while the models have lower estimates for Alaska compared to the inversions (Chang et al., 2014; Miller et al., 2016). However, for the two hotspots of the Pantanal and Sudd wetlands, the models tended to underestimate the annual eCH4 compared to a few recent satellite-based estimates (Gerlein-Safdi et al., 2021; Gloor et al., 2021; Lunt et al., 2021; Pandey et al., 2021), with a large uncertainty range of up to 2 orders of magnitude across the model ensemble (Fig. S5). In addition to the regions where eCH4 are being underestimated, recent studies (France et al., 2022; Shaw et al., 2022) based on aircraft measurements suggest that the bottom-up models likely underestimate high eCH4 fluxes in some little-studied wetlands, such as those in Zambia and Bolivia. The underestimations by process-based wetland models can be attributed to (1) the challenge in accurately capturing the areal dynamics of wetlands under varying hydrological conditions, such as in flat terrains that receive lateral transport of water from upper streams (Li et al., 2024; Lunt et al., 2021; Gerlein-Safdi et al., 2021); (2) existing knowledge gaps in mapping wetlands in remote areas, which affect the parameterization of inundation modeling; and (3) the limited representation of water table regulation (Chen et al., 2021) and wetland PFTs (Bastviken et al., 2023) on eCH4 in biogeochemical models.
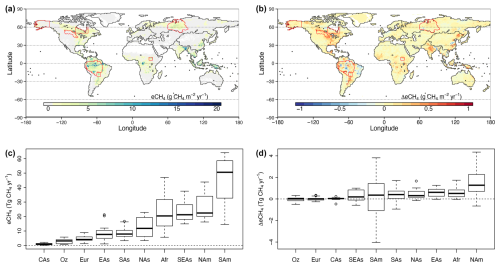
Figure 2Spatial distribution of eCH4 and the average change between the 2010s and 2000s. (a) Map of mean eCH4 (unit: g CH4 m−2 yr−1 per 0.5° grid cell) for 2000–2020. The regions defined in panels (c) and (d) and regional CH4 hotspots in Table S3 are outlined in black and red, respectively. (b) Map of change in mean annual wetland emissions (ΔeCH4) between the 2010s and 2000s. (c) Boxplot of mean annual eCH4 and (d) ΔeCH4 by regions for 2000–2020 in ascending order for median estimates. Afr: Africa; CAs: Central Asia; EAs: East Asia; Eur: Europe; NAm: North America; NAs: North Asia; Oz: Oceania; SAm: South America; SAs: South Asia; SEAs: Southeast Asia.
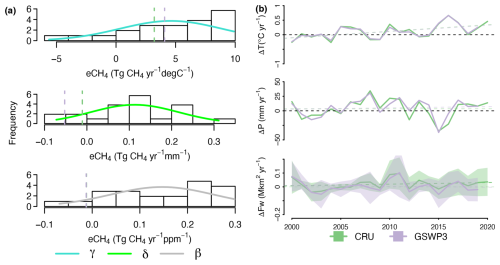
Figure 3Attributions of ΔeCH4 during 2000–2020. (a) Histogram showing the sensitivity coefficients derived from a multiple regression approach (see the Methods section) for temperature (γ), precipitation (δ), and atmospheric CO2 concentration (β). The curves represent probability distributions of sensitivity coefficients across the models, assuming a Gaussian distribution. Vertical lines represent estimates from the machine learning-based dataset UpCH4, with different colors corresponding to different climate datasets. (b) Time series of anomalies for annual mean temperature (ΔT), annual total precipitation (ΔP), and annual mean wetland extent (ΔFw) for 2000–2020 for CRU and 2000–2019 for GSWP3. The shaded areas in ΔFw represent the minimum and maximum ranges from the prognostic model simulations. Dashed lines are linear fitted trends for the corresponding variables.
3.3 Attribution of wetland CH4 changes
To evaluate the relative contribution of different factors on global eCH4, we calculated the sensitivity of eCH4 to mean annual temperature (denoted as γ), annual total precipitation (denoted as δ), and CO2 concentration (denoted as β) using a multiple regression approach for each model run over the period of 2000–2020. The same approach was applied to the upscaled gridded machine learning dataset UpCH4, which uses eddy covariance measurements from FLUXNET-CH4 as training inputs. The model ensemble suggests that temperature is the primary driver of the increase in eCH4 (Fig. 3a). The regression coefficient for γ is 4.6 Tg CH4 yr−1 °C−1, with a range of −0.4 and 9.0 Tg CH4 yr−1 °C−1 between the 10th and 90th percentiles among all models. This mean temperature sensitivity is slightly higher than the γ coefficient of 3.2–4.1 Tg CH4 yr−1 °C−1 estimated for UpCH4. In contrast, precipitation contributed little to the increase from the prognostic simulations, with a coefficient δ of 0–0.3 Tg CH4 yr−1 mm−1. The coefficient δ was lower at −0.05–0 Tg CH4 yr−1 mm−1 for UpCH4, as precipitation was not chosen as a model training predictor through its feature selection, based on site-level eddy covariance measurements (McNicol et al., 2023). However, precipitation is a more dominant factor at large scales, especially for tropical floodplains, which contribute the largest proportion of emissions but are poorly represented by eddy covariance measurements. The model-ensemble-estimated β remains small, ranging from 0 to 0.3 Tg CH4 yr−1 ppm−1, while UpCH4 suggests a β at −0.01 Tg CH4 yr−1 ppm−1. However, other confounding drivers might influence eCH4 as well, such as solar radiation, wind speed, and nitrogen deposition. Thus, the inferred sensitivities are implicitly accounted for in the regression coefficients despite their relatively small impacts compared to the major drivers.
Generally, the factorial simulations of the four-model subset indicated a consistently positive contribution (three out of four) from rising temperature to ΔeCH4, with a large variability (SD = 4.3 Tg CH4 yr−1) of contributions from precipitation (Fig. S6). The strength of the CO2 fertilization effect varied among models and was moderate but positive in all models. Two models (ELM-ECA and SDGVM) were among the models with higher sensitivity to climate variations, while LPJ-wsl and VISIT were close to the full ensemble mean. ELM-ECA produced a negative temperature effect on eCH4, likely due to its modeled nutrient constraints and higher temperature sensitivity for methanotrophic compared to methanogenic processes. Considering the deviation of each model from the full ensemble mean, the weighted mean (Fig. S7) contributions for temperature, precipitation, and CO2 concentration from the subset models were 3.2, 1.8, and 1.4 Tg CH4 yr−1, respectively. The results from the subset of the models consistently demonstrate that temperature is the primary factor influencing eCH4.
Overall, the interannual variations of modeled eCH4 were primarily associated with rising temperature, altered precipitation patterns, and rising atmospheric CO2 concentrations that stimulated ecosystem productivity through the CO2 fertilization effect (Yvon-Durocher et al., 2014). We note that a recent study found strong hysteresis in the seasonal temperature dependence of observed eCH4 using the FLUXNET-CH4 dataset (Chang et al., 2021). Those hysteretic features likely result in uncertainty in annual temperature sensitivity estimates but would not bias the conclusion of temperature as a dominant controller of eCH4 at the decadal timescale. The links between rising temperature and enhanced net CH4 fluxes are evident (as described below), as the annual global average temperature over wetland areas has significantly (p<0.01) increased by 0.5–0.7 °C from 2000–2020 (Fig. 3b). The modeled interannual variations of wetland extent dynamics reproduced the response to strong climate events (e.g., positive anomaly during the La Niña phase in 2010/2011 (Boening et al., 2012) and 2020). Both climate-forcing datasets suggest no significant trend in the anomaly of annual mean wetland area globally over the same period based on the prognostic hydrological simulations (Fig. 3b). Similarly, no significant regional trends in wetland area were found for most of the subregions, with the exception of South America, which shows a decrease, and East Asia, which shows a slight increase (Fig. S8). Considering that the extent of modeled wetland areas is primarily driven by precipitation, we do not detect a substantial contribution of changes in wetland extent to the long-term increase in eCH4 over 2000–2020 based on the climate datasets. However, considerable differences in annual and seasonal precipitation estimates between the climate datasets used in this study and those derived from reanalysis or satellite-based products (Zhang et al., 2023a) result in large uncertainties in the estimated trends in wetland extent.
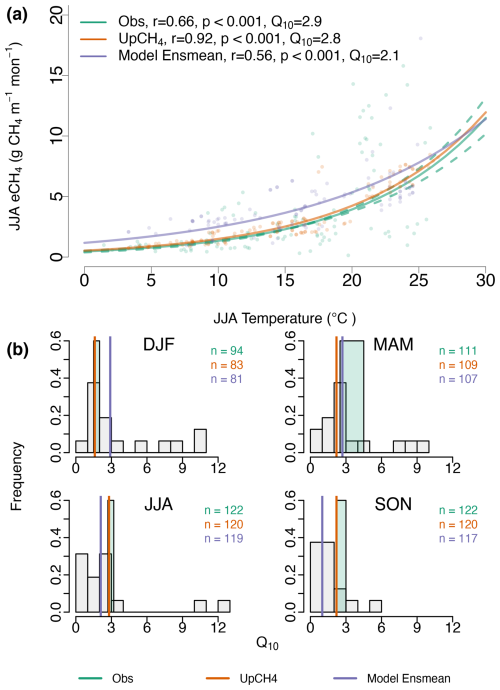
Figure 4Temperature dependence of simulated seasonal eCH4 across locations of FLUXNET-CH4 sites. (a) Model ensemble mean (“Model Ensmean”) of simulated eCH4 against seasonal mean temperature for the JJA season along the temperature gradient at the locations of FLUXNET-CH4 sites in comparison to the estimates from eddy covariance measurements (“Obs”; Fig. S10; Table S4) and UpCH4. Each dot represents the value at one site for an individual year when observations are available. The unit of the simulated CH4 emissions is gCH4 m−1 month−1 per standard wetland area to exclude the effect of inundation on eCH4. The exponential fitted curves are shown. (b) Histogram of the seasonal Q10 for the 16 individual models for the months of DJF, MAM, JJA, and SON. Sample sizes are shown in the plot. The Q10 values derived from FLUXNET-CH4, UpCH4, and the model ensemble mean are shown as vertical solid lines, with a width of the bar for “Obs” indicating the uncertainty range of Q10 based on measurement uncertainty.
3.4 Temperature dependence of wetland CH4 models
The modeled CH4 emissions show an exponential relationship between eCH4 and air temperature, with higher temperatures corresponding to higher mean eCH4 during the peak growing season (JJA, June–July–August) in the Northern Hemisphere (Fig. 4a). The model ensemble mean of eCH4 response to temperature shows good agreement within the range of the spread when compared to the site-level measurements from FLUXNET-CH4 and the gridded product UpCH4. The model ensemble mean has a higher CH4 emitting strength (i.e., CH4 emission per standard wetland area) for the high latitudes, leading to lower apparent Q10. This implies that the model-ensemble-estimated temperature dependence for the high latitudes could be potentially overestimated during the JJA season. The apparent Q10 values for individual models show a large spread (Fig. S9), with 11 out of the 16 models having statistically significant (p<0.01) exponential relationships. The good agreement between the ensemble mean and observations suggests that the ensemble approach provides a better constraint compared to single models alone. Furthermore, it is important to acknowledge that the sparse spatial coverage of FLUXNET-CH4 over low latitudes, especially for underrepresented areas such as Africa, Southeast Asia, and South America, limits our ability to evaluate temperature dependencies over high-temperature regions (Fig. S10).
The modeled apparent Q10 exhibits an average temperature dependence similar to that of ecosystem respiration, as reported by previous studies (Bloom et al., 2017; Mahecha et al., 2010; Yvon-Durocher et al., 2014), indicating that the underlying factors controlling the response of eCH4 and ecosystem respiration to temperature covary. The modeled temperature dependences are more constrained with less spread for JJA and SON (September–October–November) than DJF (December–January–February) and MAM (March–April–May) when most site-level measurements have limited availability. The seasonal variations of modeled apparent Q10 differ from site-level observations or UpCH4, reflecting discrepancies in the involved processes between eddy covariance and land surface models. Given that underrepresented processes such as substrate supply tend to have higher sensitivity of ecosystem metabolic processes to temperature, it is likely that the models do not entirely capture the fine-scale processes that affect the overall temperature response (Chang et al., 2021). In addition, the absence or underrepresentation of certain biophysical processes could lead to lower modeled apparent Q10. For instance, the ensemble mean of modeled apparent Q10 for SON months is underestimated, likely linked to the limited representation of processes during the freeze–thaw cycle (e.g., zero-curtain period), as suggested by previous observational studies (Mastepanov et al., 2008; Zona et al., 2016).
Our results estimated global average wetland CH4 emissions at 158 ± 24 (mean ± 1σ) Tg CH4 yr−1 for the period 2010–2020, with an average decadal increase of 6–7 Tg CH4 yr−1 compared to the decade of 2000–2009. The increases in the four latitudinal bands of 90–30° S, 30° S–30° N, 30–60° N, and 60–90° N were 0.1–0.2, 3.6–3.7, 1.8–2.4, and 0.6–0.8 Tg CH4 yr−1, respectively, during the 2 decades. Our analysis reveals how global wetlands respond to variations in the primary climatic controls of temperature, precipitation, and rising CO2 concentrations. The model average shows good agreement with eddy covariance measurements on temperature dependence, confirming the primary role of temperature in the rising trajectory of eCH4 at decadal timescales. Furthermore, the modeled ensembles of prognostic wetland extents offer a complementary approach to satellite-based estimates (Prigent et al., 2020; Zhang, et al., 2021a) and enable further investigation into the uncertainties in wetland area estimation. These differences can motivate improvements to inundation schemes through an improved water table position (Chen et al., 2021) and lateral flow representation. Note that a large portion of tropical wetlands comprise inundated floodplains connecting rivers, where the leaching of methane production from wetlands to river networks is not accounted for in the wetland models. The prognostic models estimate an annual mean maximum wetland area of 8.0 ± 2.0×106 km2, with a seasonal cycle (annual maximum minus annual minimum) of 4.7 ± 2.0×106 km2. Resolving the large uncertainty in wetland areas and seasonal variation remains a high priority to refine bottom-up estimates of eCH4. Lastly, our results highlight the important but highly uncertain CO2 fertilization effect on eCH4. The mean sensitivity coefficient β and results from the factorial experiment suggest a net increase in eCH4 of 0.1 %–2.3 % relative to the annual total under an average ∼ 20 ppm increase in atmospheric CO2 concentration. In comparison, a synthesis study based on field experiments (van Groenigen et al., 2011) shows a narrower range of 0.3 %–0.6 % average increase for every 20 ppm increase, assuming a linear fertilization effect between CO2 concentration and eCH4.
Our results show that an ensemble of process-based wetland methane models provides quantification for uncertainty in eCH4, as well as better constraints than a single model on the predicted trend and magnitude of eCH4. However, nominally distinct models might have similar biases because of similarities in the way they represent a subset of processes (see Table S1 for the model summary). Future evaluation of modeled processes, such as oxidation, production, and transport pathways, along with model errors across different timescales using statistical tools, could help identify similarities in model behaviors to guide model development (Zhang, 2023b). Furthermore, the eCH4 estimates are subject to forcing uncertainty, given that the two climate datasets applied in the simulation protocol do not cover the full magnitude and variability of climatic variables. Specifically, precipitation has a significant impact on wetland extent and anaerobic soil conditions but has large uncertainty in spatiotemporal patterns (Sun et al., 2018). Thus, we recommend that future ensemble simulations consider the uncertainty in climate variables among different datasets. In addition, the sensitivity parameters derived from the multiple regression are not independent of climate datasets. Thus, they are affected by the choice of meteorological drivers. Overall, quantitatively accounting for model performance and dependence and thoroughly evaluating the effectiveness (Chang et al., 2023) could improve the wetland model ensemble estimation in future studies.
The code for the wetland models is available upon request from the respective model groups. The wetland ensemble results are publicly available in the Zenodo repository at https://doi.org/10.5281/zenodo.11309188 (Zhang, 2024). The wetland estimates from individual models are available upon request from the respective model groups. The FLUXNET-CH4 dataset is publicly available at the following link: https://fluxnet.org/data/fluxnet-ch4-community-product/ (last access: 28 December 2024, FLUXNET-CH4 Community Product, 2024). The UpCH4 dataset can be found at the link in McNicol et al. (2023).
The supplement related to this article is available online at: https://doi.org/10.5194/bg-22-305-2025-supplement.
BP and ZZ designed the simulation experiment with contributions from JM and WR. ZZ conducted data collection and data analysis. JM, WR, GB, PC, NG, PH, AI, AJ, FJ, TK, TL, XL, PM, JM, CP, SP, ZQ, QS, HT, XX, YY, XY, WZ, QinZ, QiuZ, QiaZ, and ZZ performed the simulations. ZZ prepared the manuscript with contributions from all co-authors.
At least one of the (co-)authors is a member of the editorial board of Biogeosciences. The peer-review process was guided by an independent editor, and the authors also have no other competing interests to declare.
Publisher's note: Copernicus Publications remains neutral with regard to jurisdictional claims made in the text, published maps, institutional affiliations, or any other geographical representation in this paper. While Copernicus Publications makes every effort to include appropriate place names, the final responsibility lies with the authors.
This paper is the result of a collaborative international effort under the umbrella of the Global Carbon Project, a project of Future Earth, and a research partner of the World Climate Research Programme. Joe R. Melton thanks Jade Skye for her assistance in running and processing the CLASSIC model simulations.
Zhen Zhang acknowledges support from the National Natural Science Foundation of China Basic Science Center for Tibetan Plateau Earth System project. Yi Xi and Shushi Peng were funded by NSFC (41830643, 41722101). Thomas Kleinen acknowledges support from the German Federal Ministry of Education and Research ((BMBF) grant no. 01LP1921A). Akihiko Ito and Prabir Patra were partly supported by the Arctic Challenge for Sustainability II (ArCS-II) project (grant no. JPMXD1420318865), funded by Japan's Ministry of Education, Culture, Sports, Science and Technology (MEXT). Xiangyu Liu and Qianlai Zhuang are supported by a NASA project (NNX17AK20G). Qiuan Zhu and Changhui Peng are supported by the Second Tibetan Plateau Scientific Expedition (2019QZKK0304). Jurek Müller, Qing Sun, and Fortunat Joos were supported by the Swiss National Science Foundation (#200020_200511). Qing Zhu and William Riley were supported by the Reducing Uncertainties in Biogeochemical Interactions through Synthesis and Computation (RUBISCO) Scientific Focus Area and Energy Exascale Earth System Modeling Project, which are sponsored by the Earth and Environmental Systems Modeling (EESM) program under the Office of Biological and Environmental Research of the U.S. Department of Energy Office of Science. Yuanzhi Yao and Hanqin Tian are funded in part by an NSF program (award number: #1903722) and the NASA CMS Program (award number: NX14AO73G). Tingting Li was supported by the National Key Scientific and Technological Infrastructure project “Earth System Science Numerical Simulator Facility” (EarthLab) and the Open Research Program of the International Research Center of Big Data for Sustainable Development Goals (grant no. CBAS2023ORP02). Peter Hopcroft was supported by a Birmingham Fellowship and the University of Birmingham's BlueBEAR high-performance computing (HPC) service. Wenxin Zhang acknowledges support from the LUNARC computation project LU 2021/2-114 and the Swedish Research Council (Vetenskapsrådet), starting grant 2020-05338. Wenxin Zhang and Paul A. Miller acknowledge this study as a contribution to the strategic research areas Modeling the Regional and Global Earth System (MERGE) and Biodiversity and Ecosystem Services in a Changing Climate (BECC) at Lund University. Robert B. Jackson acknowledges support from the United Nations Environment Programme (UNEP) to Stanford University DTIE21-EN3143. Nicola Gedney was supported by the Newton Fund through the Met Office Climate Science for Service Partnership Brazil (CSSP Brazil). Atul K. Jain and Xiaoming Xu were supported by the US National Science Foundation (NSF-831361857) and would like to acknowledge the high-performance computing support from Cheyenne (https://www.cisl.ucar.edu/ncar-supercomputing-history/cheyenne, last access: 15 January 2025, Cheyenne HPE, 2016), provided by NCAR’s Computational and Information Systems Laboratory, sponsored by the National Science Foundation. Philippe Ciais acknowledges support from the space Agency Climate Change Initiative (ESA CCI) RECCAP2 project (grant no. ESRIN/4000123002/18/I-NB). Josep G. Canadell acknowledges the support of the Australian National Environmental Climate Science Program – Climate Systems hub. Gavin McNicol acknowledges support from the NASA CMS program (award number: NNH20ZDA001N).
This paper was edited by Tyler Cyronak and reviewed by two anonymous referees.
Arndt, K. A., Oechel, W. C., Goodrich, J. P., Bailey, B. A., Kalhori, A., Hashemi, J., Sweeney, C., and Zona, D.: Sensitivity of Methane Emissions to Later Soil Freezing in Arctic Tundra Ecosystems, J. Geophys. Res.-Biogeo., 124, 2595–2609, https://doi.org/10.1029/2019JG005242, 2019.
Arora, V. K., Melton, J. R., and Plummer, D.: An assessment of natural methane fluxes simulated by the CLASS-CTEM model, Biogeosciences, 15, 4683–4709, https://doi.org/10.5194/bg-15-4683-2018, 2018.
Bansal, S., Post van der Burg, M., Fern, R. R., Jones, J. W., Lo, R., McKenna, O. P., Tangen, B. A., Zhang, Z., and Gleason, R. A.: Large increases in methane emissions expected from North America's largest wetland complex, Sci. Adv., 9, eade1112, https://doi.org/10.1126/sciadv.ade1112, 2023.
Basso, L. S., Marani, L., Gatti, L. V., Miller, J. B., Gloor, M., Melack, J., Cassol, H. L. G., Tejada, G., Domingues, L. G., Arai, E., Sanchez, A. H., Corrêa, S. M., Anderson, L., Aragão, L. E. O. C., Correia, C. S. C., Crispim, S. P., and Neves, R. A. L.: Amazon methane budget derived from multi-year airborne observations highlights regional variations in emissions, Commun. Earth Environ., 2, 1–13, https://doi.org/10.1038/s43247-021-00314-4, 2021.
Bastviken, D., Treat, C. C., Pangala, S. R., Gauci, V., Enrich-Prast, A., Karlson, M., Gålfalk, M., Romano, M. B., and Sawakuchi, H. O.: The importance of plants for methane emission at the ecosystem scale, Aquat. Bot., 184, 103596, https://doi.org/10.1016/j.aquabot.2022.103596, 2023.
Basu, S., Lan, X., Dlugokencky, E., Michel, S., Schwietzke, S., Miller, J. B., Bruhwiler, L., Oh, Y., Tans, P. P., Apadula, F., Gatti, L. V., Jordan, A., Necki, J., Sasakawa, M., Morimoto, S., Di Iorio, T., Lee, H., Arduini, J., and Manca, G.: Estimating emissions of methane consistent with atmospheric measurements of methane and δ13C of methane, Atmos. Chem. Phys., 22, 15351–15377, https://doi.org/10.5194/acp-22-15351-2022, 2022.
Bergamaschi, P., Houweling, S., Segers, A., Krol, M., Frankenberg, C., Scheepmaker, R. A., Dlugokencky, E., Wofsy, S. C., Kort, E. A., Sweeney, C., Schuck, T., Brenninkmeijer, C., Chen, H., Beck, V., and Gerbig, C.: Atmospheric CH4 in the first decade of the 21st century: Inverse modeling analysis using SCIAMACHY satellite retrievals and NOAA surface measurements, J. Geophys. Res.-Atmos., 118, 7350–7369, https://doi.org/10.1002/jgrd.50480, 2013.
Bloom, A. A., Bowman, K. W., Lee, M., Turner, A. J., Schroeder, R., Worden, J. R., Weidner, R., McDonald, K. C., and Jacob, D. J.: A global wetland methane emissions and uncertainty dataset for atmospheric chemical transport models (WetCHARTs version 1.0), Geosci. Model Dev., 10, 2141–2156, https://doi.org/10.5194/gmd-10-2141-2017, 2017.
Boening, C., Willis, J. K., Landerer, F. W., Nerem, R. S., and Fasullo, J.: The 2011 La Niña: So strong, the oceans fell: La Niña 2011-SO strong, the oceans fell, Geophys. Res. Lett., 39, L19602, https://doi.org/10.1029/2012GL053055, 2012.
Bohn, T. J., Melton, J. R., Ito, A., Kleinen, T., Spahni, R., Stocker, B. D., Zhang, B., Zhu, X., Schroeder, R., Glagolev, M. V., Maksyutov, S., Brovkin, V., Chen, G., Denisov, S. N., Eliseev, A. V., Gallego-Sala, A., McDonald, K. C., Rawlins, M. A., Riley, W. J., Subin, Z. M., Tian, H., Zhuang, Q., and Kaplan, J. O.: WETCHIMP-WSL: intercomparison of wetland methane emissions models over West Siberia, Biogeosciences, 12, 3321–3349, https://doi.org/10.5194/bg-12-3321-2015, 2015.
Bousquet, P., Ciais, P., Miller, J. B., Dlugokencky, E. J., Hauglustaine, D. A., Prigent, C., Van der Werf, G. R., Peylin, P., Brunke, E.-G., Carouge, C., Langenfelds, R. L., Lathière, J., Papa, F., Ramonet, M., Schmidt, M., Steele, L. P., Tyler, S. C., and White, J.: Contribution of anthropogenic and natural sources to atmospheric methane variability, Nature, 443, 439–443, https://doi.org/10.1038/nature05132, 2006.
Bridgham, S. D., Cadillo-Quiroz, H., Keller, J. K., and Zhuang, Q.: Methane emissions from wetlands: biogeochemical, microbial, and modeling perspectives from local to global scales, Glob. Change Biol., 19, 1325–1346, https://doi.org/10.1111/gcb.12131, 2013.
Cao, M., Marshall, S., and Gregson, K.: Global carbon exchange and methane emissions from natural wetlands: Application of a process-based model, J. Geophys. Res.-Atmos., 101, 14399–14414, https://doi.org/10.1029/96JD00219, 1996.
Chang, K.-Y., Riley, W. J., Crill, P. M., Grant, R. F., and Saleska, S. R.: Hysteretic temperature sensitivity of wetland CH4 fluxes explained by substrate availability and microbial activity, Biogeosciences, 17, 5849–5860, https://doi.org/10.5194/bg-17-5849-2020, 2020.
Chang, K.-Y., Riley, W. J., Knox, S. H., Jackson, R. B., McNicol, G., Poulter, B., Aurela, M., Baldocchi, D., Bansal, S., Bohrer, G., Campbell, D. I., Cescatti, A., Chu, H., Delwiche, K. B., Desai, A. R., Euskirchen, E., Friborg, T., Goeckede, M., Helbig, M., Hemes, K. S., Hirano, T., Iwata, H., Kang, M., Keenan, T., Krauss, K. W., Lohila, A., Mammarella, I., Mitra, B., Miyata, A., Nilsson, M. B., Noormets, A., Oechel, W. C., Papale, D., Peichl, M., Reba, M. L., Rinne, J., Runkle, B. R. K., Ryu, Y., Sachs, T., Schäfer, K. V. R., Schmid, H. P., Shurpali, N., Sonnentag, O., Tang, A. C. I., Torn, M. S., Trotta, C., Tuittila, E.-S., Ueyama, M., Vargas, R., Vesala, T., Windham-Myers, L., Zhang, Z., and Zona, D.: Substantial hysteresis in emergent temperature sensitivity of global wetland CH4 emissions, Nat. Commun., 12, 2266, https://doi.org/10.1038/s41467-021-22452-1, 2021.
Chang, K.-Y., Riley, W. J., Collier, N., McNicol, G., Fluet-Chouinard, E., Knox, S. H., Delwiche, K. B., Jackson, R. B., Poulter, B., Saunois, M., Chandra, N., Gedney, N., Ishizawa, M., Ito, A., Joos, F., Kleinen, T., Maggi, F., McNorton, J., Melton, J. R., Miller, P., Niwa, Y., Pasut, C., Patra, P. K., Peng, C., Peng, S., Segers, A., Tian, H., Tsuruta, A., Yao, Y., Yin, Y., Zhang, W., Zhang, Z., Zhu, Q., Zhu, Q., and Zhuang, Q.: Observational constraints reduce model spread but not uncertainty in global wetland methane emission estimates, Glob. Change Biol., 29, 4298–4312, https://doi.org/10.1111/gcb.16755, 2023.
Chang, R. Y.-W., Miller, C. E., Dinardo, S. J., Karion, A., Sweeney, C., Daube, B. C., Henderson, J. M., Mountain, M. E., Eluszkiewicz, J., Miller, J. B., Bruhwiler, L. M. P., and Wofsy, S. C.: Methane emissions from Alaska in 2012 from CARVE airborne observations, P. Natl. Acad. Sci. USA, 111, 16694–16699, https://doi.org/10.1073/pnas.1412953111, 2014.
Chu, H., Luo, X., Ouyang, Z., Chan, W. S., Dengel, S., Biraud, S. C., Torn, M. S., Metzger, S., Kumar, J., Arain, M. A., Arkebauer, T. J., Baldocchi, D., Bernacchi, C., Billesbach, D., Black, T. A., Blanken, P. D., Bohrer, G., Bracho, R., Brown, S., Brunsell, N. A., Chen, J., Chen, X., Clark, K., Desai, A. R., Duman, T., Durden, D., Fares, S., Forbrich, I., Gamon, J. A., Gough, C. M., Griffis, T., Helbig, M., Hollinger, D., Humphreys, E., Ikawa, H., Iwata, H., Ju, Y., Knowles, J. F., Knox, S. H., Kobayashi, H., Kolb, T., Law, B., Lee, X., Litvak, M., Liu, H., Munger, J. W., Noormets, A., Novick, K., Oberbauer, S. F., Oechel, W., Oikawa, P., Papuga, S. A., Pendall, E., Prajapati, P., Prueger, J., Quinton, W. L., Richardson, A. D., Russell, E. S., Scott, R. L., Starr, G., Staebler, R., Stoy, P. C., Stuart-Haëntjens, E., Sonnentag, O., Sullivan, R. C., Suyker, A., Ueyama, M., Vargas, R., Wood, J. D., and Zona, D.: Representativeness of Eddy-Covariance flux footprints for areas surrounding AmeriFlux sites, Agr. Forest Meteorol., 301/302, 108350, https://doi.org/10.1016/j.agrformet.2021.108350, 2021.
Cucchi, M., Weedon, G. P., Amici, A., Bellouin, N., Lange, S., Müller Schmied, H., Hersbach, H., and Buontempo, C.: WFDE5: bias-adjusted ERA5 reanalysis data for impact studies, Earth Syst. Sci. Data, 12, 2097–2120, https://doi.org/10.5194/essd-12-2097-2020, 2020.
Delwiche, K. B., Knox, S. H., Malhotra, A., Fluet-Chouinard, E., McNicol, G., Feron, S., Ouyang, Z., Papale, D., Trotta, C., Canfora, E., Cheah, Y.-W., Christianson, D., Alberto, Ma. C. R., Alekseychik, P., Aurela, M., Baldocchi, D., Bansal, S., Billesbach, D. P., Bohrer, G., Bracho, R., Buchmann, N., Campbell, D. I., Celis, G., Chen, J., Chen, W., Chu, H., Dalmagro, H. J., Dengel, S., Desai, A. R., Detto, M., Dolman, H., Eichelmann, E., Euskirchen, E., Famulari, D., Fuchs, K., Goeckede, M., Gogo, S., Gondwe, M. J., Goodrich, J. P., Gottschalk, P., Graham, S. L., Heimann, M., Helbig, M., Helfter, C., Hemes, K. S., Hirano, T., Hollinger, D., Hörtnagl, L., Iwata, H., Jacotot, A., Jurasinski, G., Kang, M., Kasak, K., King, J., Klatt, J., Koebsch, F., Krauss, K. W., Lai, D. Y. F., Lohila, A., Mammarella, I., Belelli Marchesini, L., Manca, G., Matthes, J. H., Maximov, T., Merbold, L., Mitra, B., Morin, T. H., Nemitz, E., Nilsson, M. B., Niu, S., Oechel, W. C., Oikawa, P. Y., Ono, K., Peichl, M., Peltola, O., Reba, M. L., Richardson, A. D., Riley, W., Runkle, B. R. K., Ryu, Y., Sachs, T., Sakabe, A., Sanchez, C. R., Schuur, E. A., Schäfer, K. V. R., Sonnentag, O., Sparks, J. P., Stuart-Haëntjens, E., Sturtevant, C., Sullivan, R. C., Szutu, D. J., Thom, J. E., Torn, M. S., Tuittila, E.-S., Turner, J., Ueyama, M., Valach, A. C., Vargas, R., Varlagin, A., Vazquez-Lule, A., Verfaillie, J. G., Vesala, T., Vourlitis, G. L., Ward, E. J., Wille, C., Wohlfahrt, G., Wong, G. X., Zhang, Z., Zona, D., Windham-Myers, L., Poulter, B., and Jackson, R. B.: FLUXNET-CH4: a global, multi-ecosystem dataset and analysis of methane seasonality from freshwater wetlands , Earth Syst. Sci. Data, 13, 3607–3689, https://doi.org/10.5194/essd-13-3607-2021, 2021.
Dirmeyer, P. A., Gao, X., Zhao, M., Guo, Z., Oki, T., and Hanasaki, N.: GSWP-2: Multimodel Analysis and Implications for Our Perception of the Land Surface, Bull. Am. Meteorol. Soc., 87, 1381–1398, https://doi.org/10.1175/BAMS-87-10-1381, 2006.
Feng, L., Palmer, P. I., Zhu, S., Parker, R. J., and Liu, Y.: Tropical methane emissions explain large fraction of recent changes in global atmospheric methane growth rate, Nat. Commun., 13, 1378, https://doi.org/10.1038/s41467-022-28989-z, 2022.
Fleischmann, A. Papa, F., Hamilton, S. K., Fassoni-Andrade, A., Wongchuig, S., Espinoza, J., Paiva, R., Melack, J., Fluet-Chouinard, E., Castello, L., Almeida, R., Bonnet, M., Alves, L., Moreira, D., Yamazaki, D., Revel, M., and Collischonn, W.: Increased floodplain inundation in the Amazon since 1980, Environ. Res. Lett., 18, 034024, https://doi.org/10.1088/1748-9326/acb9a7, 2023.
Fluet-Chouinard, E., Stocker, B. D., Zhang, Z., Malhotra, A., Melton, J. R., Poulter, B., Kaplan, J. O., Goldewijk, K. K., Siebert, S., Minayeva, T., Hugelius, G., Joosten, H., Barthelmes, A., Prigent, C., Aires, F., Hoyt, A. M., Davidson, N., Finlayson, C. M., Lehner, B., Jackson, R. B., and McIntyre, P. B.: Extensive global wetland loss over the past three centuries, Nature, 614, 281–286, https://doi.org/10.1038/s41586-022-05572-6, 2023.
FLUXNET-CH4 Community Product: A global database of eddy covariance methane flux measurements, FLUXNET [data set], https://fluxnet.org/data/fluxnet-ch4-community-product/ (last access: 28 December 2024).
France, J. L., Lunt, M. F., Andrade, M., Moreno, I., Ganesan, A. L., Lachlan-Cope, T., Fisher, R. E., Lowry, D., Parker, R. J., Nisbet, E. G., and Jones, A. E.: Very large fluxes of methane measured above Bolivian seasonal wetlands, P. Natl. Acad. Sci. USA, 119, e2206345119, https://doi.org/10.1073/pnas.2206345119, 2022.
Gatti, L. V., Basso, L. S., Miller, J. B., Gloor, M., Gatti Domingues, L., Cassol, H. L. G., Tejada, G., Aragão, L. E. O. C., Nobre, C., Peters, W., Marani, L., Arai, E., Sanches, A. H., Corrêa, S. M., Anderson, L., Von Randow, C., Correia, C. S. C., Crispim, S. P., and Neves, R. A. L.: Amazonia as a carbon source linked to deforestation and climate change, Nature, 595, 388–393, https://doi.org/10.1038/s41586-021-03629-6, 2021.
Gedney, N.: Climate feedback from wetland methane emissions, Geophys. Res. Lett., 31, L20503, https://doi.org/10.1029/2004GL020919, 2004.
Gerlein-Safdi, C., Bloom, A. A., Plant, G., Kort, E. A., and Ruf, C. S.: Improving Representation of Tropical Wetland Methane Emissions With CYGNSS Inundation Maps, Global Biogeochem. Cy., 35, e2020GB006890, https://doi.org/10.1029/2020GB006890, 2021.
Glagolev, M., Kleptsova, I., Filippov, I., Maksyutov, S., and Machida, T.: Regional methane emission from West Siberia mire landscapes, Environ. Res. Lett., 6, 045214, https://doi.org/10.1088/1748-9326/6/4/045214, 2011.
Gloor, M., Gatti, L. V., Wilson, C., Parker, R. J., Boesch, H., Popa, E., Chipperfield, M. P., Poulter, B., Zhang, Z., Basso, L., Miller, J., McNorton, J., Jimenez, C., and Prigent, C.: Large Methane Emissions From the Pantanal During Rising Water-Levels Revealed by Regularly Measured Lower Troposphere CH4 Profiles, Global Biogeochem. Cy., 35, e2021GB006964, https://doi.org/10.1029/2021GB006964, 2021.
Grant, R. F., Mekonnen, Z. A., Riley, W. J., Arora, B., and Torn, M. S.: Mathematical Modelling of Arctic Polygonal Tundra with Ecosys: 2. Microtopography Determines How CO2 and CH4 Exchange Responds to Changes in Temperature and Precipitation, J. Geophys. Res.-Biogeo., 122, 3174–3187, https://doi.org/10.1002/2017JG004037, 2017.
Harris, I., Jones, P. D., Osborn, T. J., and Lister, D. H.: Updated high-resolution grids of monthly climatic observations – the CRU TS3.10 Dataset: updated high-resolution grids of monthly climatic observations, Int. J. Climatol., 34, 623–642, https://doi.org/10.1002/joc.3711, 2014.
Hopcroft, P. O., Valdes, P. J., O'Connor, F. M., Kaplan, J. O., and Beerling, D. J.: Understanding the glacial methane cycle, Nat. Commun., 8, 14383, https://doi.org/10.1038/ncomms14383, 2017.
Kaiser, S., Göckede, M., Castro-Morales, K., Knoblauch, C., Ekici, A., Kleinen, T., Zubrzycki, S., Sachs, T., Wille, C., and Beer, C.: Process-based modelling of the methane balance in periglacial landscapes (JSBACH-methane), Geosci. Model Dev., 10, 333–358, https://doi.org/10.5194/gmd-10-333-2017, 2017.
Kirschke, S., Bousquet, P., Ciais, P., Saunois, M., Canadell, J. G., Dlugokencky, E. J., Bergamaschi, P., Bergmann, D., Blake, D. R., Bruhwiler, L., Cameron-Smith, P., Castaldi, S., Chevallier, F., Feng, L., Fraser, A., Heimann, M., Hodson, E. L., Houweling, S., Josse, B., Fraser, P. J., Krummel, P. B., Lamarque, J.-F., Langenfelds, R. L., Le Quéré, C., Naik, V., O'Doherty, S., Palmer, P. I., Pison, I., Plummer, D., Poulter, B., Prinn, R. G., Rigby, M., Ringeval, B., Santini, M., Schmidt, M., Shindell, D. T., Simpson, I. J., Spahni, R., Steele, L. P., Strode, S. A., Sudo, K., Szopa, S., van der Werf, G. R., Voulgarakis, A., van Weele, M., Weiss, R. F., Williams, J. E., and Zeng, G.: Three decades of global methane sources and sinks, Nat. Geosci., 6, 813–823, https://doi.org/10.1038/ngeo1955, 2013.
Kleinen, T., Gromov, S., Steil, B., and Brovkin, V.: Atmospheric methane since the last glacial maximum was driven by wetland sources, Clim. Past, 19, 1081–1099, https://doi.org/10.5194/cp-19-1081-2023, 2023.
Knox, S. H., Jackson, R. B., Poulter, B., McNicol, G., Fluet-Chouinard, E., Zhang, Z., Hugelius, G., Bousquet, P., Canadell, J. G., Saunois, M., Papale, D., Chu, H., Keenan, T. F., Baldocchi, D., Torn, M. S., Mammarella, I., Trotta, C., Aurela, M., Bohrer, G., Campbell, D. I., Cescatti, A., Chamberlain, S., Chen, J., Chen, W., Dengel, S., Desai, A. R., Euskirchen, E., Friborg, T., Gasbarra, D., Goded, I., Goeckede, M., Heimann, M., Helbig, M., Hirano, T., Hollinger, D. Y., Iwata, H., Kang, M., Klatt, J., Krauss, K. W., Kutzbach, L., Lohila, A., Mitra, B., Morin, T. H., Nilsson, M. B., Niu, S., Noormets, A., Oechel, W. C., Peichl, M., Peltola, O., Reba, M. L., Richardson, A. D., Runkle, B. R. K., Ryu, Y., Sachs, T., Schäfer, K. V. R., Schmid, H. P., Shurpali, N., Sonnentag, O., Tang, A. C. I., Ueyama, M., Vargas, R., Vesala, T., Ward, E. J., Windham-Myers, L., Wohlfahrt, G., and Zona, D.: FLUXNET-CH4 Synthesis Activity: Objectives, Observations, and Future Directions, Bull. Am. Meteorol. Soc., 100, 2607–2632, https://doi.org/10.1175/BAMS-D-18-0268.1, 2019.
Koffi, E. N., Bergamaschi, P., Alkama, R., and Cescatti, A.: An observation-constrained assessment of the climate sensitivity and future trajectories of wetland methane emissions, Sci. Adv., 6, eaay4444, https://doi.org/10.1126/sciadv.aay4444, 2020.
Kuhn, M. A., Varner, R. K., Bastviken, D., Crill, P., MacIntyre, S., Turetsky, M., Walter Anthony, K., McGuire, A. D., and Olefeldt, D.: BAWLD-CH4: a comprehensive dataset of methane fluxes from boreal and arctic ecosystems, Earth Syst. Sci. Data, 13, 5151–5189, https://doi.org/10.5194/essd-13-5151-2021, 2021.
Li, M., Kort, E. A., Bloom, A. A., Wu, D., Plant, G., Gerlein-Safdi, C., and Pu, T.: Underestimated Dry Season Methane Emissions from Wetlands in the Pantanal, Environ. Sci. Technol., 58, 3278–3287, https://doi.org/10.1021/acs.est.3c09250, 2024.
Lan, X., Basu, S., Schwietzke, S., Bruhwiler, L. M. P., Dlugokencky, E. J., Michel, S. E., Sherwood, O. A., Tans, P. P., Thoning, K., Etiope, G., Zhuang, Q., Liu, L., Oh, Y., Miller, J. B., Pétron, G., Vaughn, B. H., and Crippa, M.: Improved Constraints on Global Methane Emissions and Sinks Using δ13C-CH4, Global Biogeochem. Cy., 35, e2021GB007000, https://doi.org/10.1029/2021GB007000, 2021.
Lunt, M. F., Palmer, P. I., Lorente, A., Borsdorff, T., Landgraf, J., Parker, R. J., and Boesch, H.: Rain-fed pulses of methane from East Africa during 2018–2019 contributed to atmospheric growth rate, Environ. Res. Lett., 16, 024021, https://doi.org/10.1088/1748-9326/abd8fa, 2021.
Mahecha, M. D., Reichstein, M., Carvalhais, N., Lasslop, G., Lange, H., Seneviratne, S. I., Vargas, R., Ammann, C., Arain, M. A., Cescatti, A., Janssens, I. A., Migliavacca, M., Montagnani, L., and Richardson, A. D.: Global Convergence in the Temperature Sensitivity of Respiration at Ecosystem Level, Science, 329, 838–840, https://doi.org/10.1126/science.1189587, 2010.
Mastepanov, M., Sigsgaard, C., Dlugokencky, E. J., Houweling, S., Ström, L., Tamstorf, M. P., and Christensen, T. R.: Large tundra methane burst during onset of freezing, Nature, 456, 628–630, https://doi.org/10.1038/nature07464, 2008.
McNicol, G., Fluet-Chouinard, E., Ouyang, Z., Knox, S., Zhang, Z., Aalto, T., Bansal, S., Chang, K.-Y., Chen, M., Delwiche, K., Feron, S., Goeckede, M., Liu, J., Malhotra, A., Melton, J. R., Riley, W., Vargas, R., Yuan, K., Ying, Q., Zhu, Q., Alekseychik, P., Aurela, M., Billesbach, D. P., Campbell, D. I., Chen, J., Chu, H., Desai, A. R., Euskirchen, E., Goodrich, J., Griffis, T., Helbig, M., Hirano, T., Iwata, H., Jurasinski, G., King, J., Koebsch, F., Kolka, R., Krauss, K., Lohila, A., Mammarella, I., Nilson, M., Noormets, A., Oechel, W., Peichl, M., Sachs, T., Sakabe, A., Schulze, C., Sonnentag, O., Sullivan, R. C., Tuittila, E.-S., Ueyama, M., Vesala, T., Ward, E., Wille, C., Wong, G. X., Zona, D., Windham-Myers, L., Poulter, B., and Jackson, R. B.: Upscaling Wetland Methane Emissions From the FLUXNET-CH4 Eddy Covariance Network (UpCH4 v1.0): Model Development, Network Assessment, and Budget Comparison, AGU Advances, 4, e2023AV000956, https://doi.org/10.1029/2023AV000956, 2023.
Meinshausen, M., Vogel, E., Nauels, A., Lorbacher, K., Meinshausen, N., Etheridge, D. M., Fraser, P. J., Montzka, S. A., Rayner, P. J., Trudinger, C. M., Krummel, P. B., Beyerle, U., Canadell, J. G., Daniel, J. S., Enting, I. G., Law, R. M., Lunder, C. R., O'Doherty, S., Prinn, R. G., Reimann, S., Rubino, M., Velders, G. J. M., Vollmer, M. K., Wang, R. H. J., and Weiss, R.: Historical greenhouse gas concentrations for climate modelling (CMIP6), Geosci. Model Dev., 10, 2057–2116, https://doi.org/10.5194/gmd-10-2057-2017, 2017.
Melton, J. R., Wania, R., Hodson, E. L., Poulter, B., Ringeval, B., Spahni, R., Bohn, T., Avis, C. A., Beerling, D. J., Chen, G., Eliseev, A. V., Denisov, S. N., Hopcroft, P. O., Lettenmaier, D. P., Riley, W. J., Singarayer, J. S., Subin, Z. M., Tian, H., Zürcher, S., Brovkin, V., van Bodegom, P. M., Kleinen, T., Yu, Z. C., and Kaplan, J. O.: Present state of global wetland extent and wetland methane modelling: conclusions from a model inter-comparison project (WETCHIMP), Biogeosciences, 10, 753–788, https://doi.org/10.5194/bg-10-753-2013, 2013.
Miller, S. M., Miller, C. E., Commane, R., Chang, R. Y.-W., Dinardo, S. J., Henderson, J. M., Karion, A., Lindaas, J., Melton, J. R., Miller, J. B., Sweeney, C., Wofsy, S. C., and Michalak, A. M.: A multiyear estimate of methane fluxes in Alaska from CARVE atmospheric observations: METHANE FLUXES FROM ALASKA, Global Biogeochem. Cy., 30, 1441–1453, https://doi.org/10.1002/2016GB005419, 2016.
Natali, S. M., Watts, J. D., Rogers, B. M., Potter, S., Ludwig, S. M., Selbmann, A.-K., Sullivan, P. F., Abbott, B. W., Arndt, K. A., Birch, L., Björkman, M. P., Bloom, A. A., Celis, G., Christensen, T. R., Christiansen, C. T., Commane, R., Cooper, E. J., Crill, P., Czimczik, C., Davydov, S., Du, J., Egan, J. E., Elberling, B., Euskirchen, E. S., Friborg, T., Genet, H., Göckede, M., Goodrich, J. P., Grogan, P., Helbig, M., Jafarov, E. E., Jastrow, J. D., Kalhori, A. A. M., Kim, Y., Kimball, J. S., Kutzbach, L., Lara, M. J., Larsen, K. S., Lee, B.-Y., Liu, Z., Loranty, M. M., Lund, M., Lupascu, M., Madani, N., Malhotra, A., Matamala, R., McFarland, J., McGuire, A. D., Michelsen, A., Minions, C., Oechel, W. C., Olefeldt, D., Parmentier, F.-J. W., Pirk, N., Poulter, B., Quinton, W., Rezanezhad, F., Risk, D., Sachs, T., Schaefer, K., Schmidt, N. M., Schuur, E. A. G., Semenchuk, P. R., Shaver, G., Sonnentag, O., Starr, G., Treat, C. C., Waldrop, M. P., Wang, Y., Welker, J., Wille, C., Xu, X., Zhang, Z., Zhuang, Q., and Zona, D.: Large loss of CO2 in winter observed across the northern permafrost region, Nat. Clim. Change, 9, 852–857, https://doi.org/10.1038/s41558-019-0592-8, 2019.
Nisbet, E. G., Manning, M. R., Dlugokencky, E. J., Fisher, R. E., Lowry, D., Michel, S. E., Myhre, C. L., Platt, S. M., Allen, G., Bousquet, P., Brownlow, R., Cain, M., France, J. L., Hermansen, O., Hossaini, R., Jones, A. E., Levin, I., Manning, A. C., Myhre, G., Pyle, J. A., Vaughn, B. H., Warwick, N. J., and White, J. W. C.: Very Strong Atmospheric Methane Growth in the 4 Years 2014–2017: Implications for the Paris Agreement, Global Biogeochem. Cy., 33, 318–342, https://doi.org/10.1029/2018GB006009, 2019.
Nisbet, E. G., Manning, M. R., Dlugokencky, E. J., Michel, S. E., Lan, X., Röckmann, T., Denier van der Gon, H. A. C., Schmitt, J., Palmer, P. I., Dyonisius, M. N., Oh, Y., Fisher, R. E., Lowry, D., France, J. L., White, J. W. C., Brailsford, G., and Bromley, T.: Atmospheric Methane: Comparison Between Methane's Record in 2006–2022 and During Glacial Terminations, Global Biogeochem. Cy., 37, e2023GB007875, https://doi.org/10.1029/2023GB007875, 2023.
Palmer, P. I., Woodwark, A. J. P., Finch, D. P., Taylor, T. E., Butz, A., Tamminen, J., Bösch, H., Eldering, A., and Vincent-Bonnieu, S.: Role of space station instruments for improving tropical carbon flux estimates using atmospheric data, npj Microgravity, 8, 51, https://doi.org/10.1038/s41526-022-00231-6, 2022.
Pandey, S., Houweling, S., Lorente, A., Borsdorff, T., Tsivlidou, M., Bloom, A. A., Poulter, B., Zhang, Z., and Aben, I.: Using satellite data to identify the methane emission controls of South Sudan's wetlands, Biogeosciences, 18, 557–572, https://doi.org/10.5194/bg-18-557-2021, 2021.
Parker, R. J., Wilson, C., Bloom, A. A., Comyn-Platt, E., Hayman, G., McNorton, J., Boesch, H., and Chipperfield, M. P.: Exploring constraints on a wetland methane emission ensemble (WetCHARTs) using GOSAT observations, Biogeosciences, 17, 5669–5691, https://doi.org/10.5194/bg-17-5669-2020, 2020.
Patra, P. K., Houweling, S., Krol, M., Bousquet, P., Belikov, D., Bergmann, D., Bian, H., Cameron-Smith, P., Chipperfield, M. P., Corbin, K., Fortems-Cheiney, A., Fraser, A., Gloor, E., Hess, P., Ito, A., Kawa, S. R., Law, R. M., Loh, Z., Maksyutov, S., Meng, L., Palmer, P. I., Prinn, R. G., Rigby, M., Saito, R., and Wilson, C.: TransCom model simulations of CH4 and related species: linking transport, surface flux and chemical loss with CH4 variability in the troposphere and lower stratosphere, Atmos. Chem. Phys., 11, 12813–12837, https://doi.org/10.5194/acp-11-12813-2011, 2011.
Peng, S., Lin, X., Thompson, R. L., Xi, Y., Liu, G., Hauglustaine, D., Lan, X., Poulter, B., Ramonet, M., Saunois, M., Yin, Y., Zhang, Z., Zheng, B., and Ciais, P.: Wetland emission and atmospheric sink changes explain methane growth in 2020, Nature, 612, 477–482, https://doi.org/10.1038/s41586-022-05447-w, 2022.
Petrescu, A. M. R., van Beek, L. P. H., van Huissteden, J., Prigent, C., Sachs, T., Corradi, C. A. R., Parmentier, F. J. W., and Dolman, A. J.: Modeling regional to global CH 4 emissions of boreal and arctic wetlands: Modeling Global CH4 Emissions, Global Biogeochem. Cy., 24, GB4009, https://doi.org/10.1029/2009GB003610, 2010.
Piao, S., Sitch, S., Ciais, P., Friedlingstein, P., Peylin, P., Wang, X., Ahlström, A., Anav, A., Canadell, J. G., Cong, N., Huntingford, C., Jung, M., Levis, S., Levy, P. E., Li, J., Lin, X., Lomas, M. R., Lu, M., Luo, Y., Ma, Y., Myneni, R. B., Poulter, B., Sun, Z., Wang, T., Viovy, N., Zaehle, S., and Zeng, N.: Evaluation of terrestrial carbon cycle models for their response to climate variability and to CO2 trends, Glob. Change Biol., 19, 2117–2132, https://doi.org/10.1111/gcb.12187, 2013.
Pickett-Heaps, C. A., Jacob, D. J., Wecht, K. J., Kort, E. A., Wofsy, S. C., Diskin, G. S., Worthy, D. E. J., Kaplan, J. O., Bey, I., and Drevet, J.: Magnitude and seasonality of wetland methane emissions from the Hudson Bay Lowlands (Canada), Atmos. Chem. Phys., 11, 3773–3779, https://doi.org/10.5194/acp-11-3773-2011, 2011.
Poulter, B., Bousquet, P., Canadell, J. G., Ciais, P., Peregon, A., Saunois, M., Arora, V. K., Beerling, D. J., Brovkin, V., Jones, C. D., Joos, F., Gedney, N., Ito, A., Kleinen, T., Koven, C. D., McDonald, K., Melton, J. R., Peng, C., Peng, S., Prigent, C., Schroeder, R., Riley, W. J., Saito, M., Spahni, R., Tian, H., Taylor, L., Viovy, N., Wilton, D., Wiltshire, A., Xu, X., Zhang, B., Zhang, Z., and Zhu, Q.: Global wetland contribution to 2000–2012 atmospheric methane growth rate dynamics, Environ. Res. Lett., 12, 094013, https://doi.org/10.1088/1748-9326/aa8391, 2017.
Prigent, C., Jimenez, C., and Bousquet, P.: Satellite-Derived Global Surface Water Extent and Dynamics Over the Last 25 Years (GIEMS-2), J. Geophys. Res.-Atmos., 125, e2019JD030711, https://doi.org/10.1029/2019JD030711, 2020.
Qu, Z., Jacob, D. J., Zhang, Y., Shen, L., Varon, D. J., Lu, X., Scarpelli, T., Bloom, A., Worden, J., and Parker, R. J.: Attribution of the 2020 surge in atmospheric methane by inverse analysis of GOSAT observations, Environ. Res. Lett., 17, 094003, https://doi.org/10.1088/1748-9326/ac8754, 2022.
Ringeval, B., Houweling, S., van Bodegom, P. M., Spahni, R., van Beek, R., Joos, F., and Röckmann, T.: Methane emissions from floodplains in the Amazon Basin: challenges in developing a process-based model for global applications, Biogeosciences, 11, 1519–1558, https://doi.org/10.5194/bg-11-1519-2014, 2014.
Rößger, N., Sachs, T., Wille, C., Boike, J., and Kutzbach, L.: Seasonal increase of methane emissions linked to warming in Siberian tundra, Nat. Clim. Change, 12, 1031–1036, https://doi.org/10.1038/s41558-022-01512-4, 2022.
Saunois, M., Bousquet, P., Poulter, B., Peregon, A., Ciais, P., Canadell, J. G., Dlugokencky, E. J., Etiope, G., Bastviken, D., Houweling, S., Janssens-Maenhout, G., Tubiello, F. N., Castaldi, S., Jackson, R. B., Alexe, M., Arora, V. K., Beerling, D. J., Bergamaschi, P., Blake, D. R., Brailsford, G., Brovkin, V., Bruhwiler, L., Crevoisier, C., Crill, P., Covey, K., Curry, C., Frankenberg, C., Gedney, N., Höglund-Isaksson, L., Ishizawa, M., Ito, A., Joos, F., Kim, H.-S., Kleinen, T., Krummel, P., Lamarque, J.-F., Langenfelds, R., Locatelli, R., Machida, T., Maksyutov, S., McDonald, K. C., Marshall, J., Melton, J. R., Morino, I., Naik, V., O'Doherty, S., Parmentier, F.-J. W., Patra, P. K., Peng, C., Peng, S., Peters, G. P., Pison, I., Prigent, C., Prinn, R., Ramonet, M., Riley, W. J., Saito, M., Santini, M., Schroeder, R., Simpson, I. J., Spahni, R., Steele, P., Takizawa, A., Thornton, B. F., Tian, H., Tohjima, Y., Viovy, N., Voulgarakis, A., van Weele, M., van der Werf, G. R., Weiss, R., Wiedinmyer, C., Wilton, D. J., Wiltshire, A., Worthy, D., Wunch, D., Xu, X., Yoshida, Y., Zhang, B., Zhang, Z., and Zhu, Q.: The Global Methane Budget 2000–2012, Earth Syst. Sci. Data, 8, 697–751, https://doi.org/10.5194/essd-8-697-2016, 2016.
Saunois, M., Stavert, A. R., Poulter, B., Bousquet, P., Canadell, J. G., Jackson, R. B., Raymond, P. A., Dlugokencky, E. J., Houweling, S., Patra, P. K., Ciais, P., Arora, V. K., Bastviken, D., Bergamaschi, P., Blake, D. R., Brailsford, G., Bruhwiler, L., Carlson, K. M., Carrol, M., Castaldi, S., Chandra, N., Crevoisier, C., Crill, P. M., Covey, K., Curry, C. L., Etiope, G., Frankenberg, C., Gedney, N., Hegglin, M. I., Höglund-Isaksson, L., Hugelius, G., Ishizawa, M., Ito, A., Janssens-Maenhout, G., Jensen, K. M., Joos, F., Kleinen, T., Krummel, P. B., Langenfelds, R. L., Laruelle, G. G., Liu, L., Machida, T., Maksyutov, S., McDonald, K. C., McNorton, J., Miller, P. A., Melton, J. R., Morino, I., Müller, J., Murguia-Flores, F., Naik, V., Niwa, Y., Noce, S., O'Doherty, S., Parker, R. J., Peng, C., Peng, S., Peters, G. P., Prigent, C., Prinn, R., Ramonet, M., Regnier, P., Riley, W. J., Rosentreter, J. A., Segers, A., Simpson, I. J., Shi, H., Smith, S. J., Steele, L. P., Thornton, B. F., Tian, H., Tohjima, Y., Tubiello, F. N., Tsuruta, A., Viovy, N., Voulgarakis, A., Weber, T. S., van Weele, M., van der Werf, G. R., Weiss, R. F., Worthy, D., Wunch, D., Yin, Y., Yoshida, Y., Zhang, W., Zhang, Z., Zhao, Y., Zheng, B., Zhu, Q., Zhu, Q., and Zhuang, Q.: The Global Methane Budget 2000–2017, Earth Syst. Sci. Data, 12, 1561–1623, https://doi.org/10.5194/essd-12-1561-2020, 2020.
Schaefer, K., Schwalm, C. R., Williams, C., Arain, M. A., Barr, A., Chen, J. M., Davis, K. J., Dimitrov, D., Hilton, T. W., Hollinger, D. Y., Humphreys, E., Poulter, B., Raczka, B. M., Richardson, A. D., Sahoo, A., Thornton, P., Vargas, R., Verbeeck, H., Anderson, R., Baker, I., Black, T. A., Bolstad, P., Chen, J., Curtis, P. S., Desai, A. R., Dietze, M., Dragoni, D., Gough, C., Grant, R. F., Gu, L., Jain, A., Kucharik, C., Law, B., Liu, S., Lokipitiya, E., Margolis, H. A., Matamala, R., McCaughey, J. H., Monson, R., Munger, J. W., Oechel, W., Peng, C., Price, D. T., Ricciuto, D., Riley, W. J., Roulet, N., Tian, H., Tonitto, C., Torn, M., Weng, E., and Zhou, X.: A model-data comparison of gross primary productivity: Results from the North American Carbon Program site synthesis, J. Geophys. Res.-Biogeo., 117, G03010, https://doi.org/10.1029/2012JG001960, 2012.
Schaefer, H., Fletcher, S. E. M., Veidt, C., Lassey, K. R., Brailsford, G. W., Bromley, T. M., Dlugokencky, E. J., Michel, S. E., Miller, J. B., Levin, I., Lowe, D. C., Martin, R. J., Vaughn, B. H., and White, J. W. C.: A 21st-century shift from fossil-fuel to biogenic methane emissions indicated by 13CH4, Science, 352, 80–84, https://doi.org/10.1126/science.aad2705, 2016.
Shaw, J. T., Allen, G., Barker, P., Pitt, J. R., Pasternak, D., Bauguitte, S. J.-B., Lee, J., Bower, K. N., Daly, M. C., Lunt, M. F., Ganesan, A. L., Vaughan, A. R., Chibesakunda, F., Lambakasa, M., Fisher, R. E., France, J. L., Lowry, D., Palmer, P. I., Metzger, S., Parker, R. J., Gedney, N., Bateson, P., Cain, M., Lorente, A., Borsdorff, T., and Nisbet, E. G.: Large Methane Emission Fluxes Observed From Tropical Wetlands in Zambia, Global Biogeochem. Cy., 36, e2021GB007261, https://doi.org/10.1029/2021GB007261, 2022.
Shindell, D. and Smith, C. J.: Climate and air-quality benefits of a realistic phase-out of fossil fuels, Nature, 573, 408–411, https://doi.org/10.1038/s41586-019-1554-z, 2019.
Shu, S., Jain, A. K., and Kheshgi, H. S.: Investigating Wetland and Nonwetland Soil Methane Emissions and Sinks Across the Contiguous United States Using a Land Surface Model, Global Biogeochem. Cy., 34, e2019GB006251, https://doi.org/10.1029/2019GB006251, 2020.
Spahni, R., Wania, R., Neef, L., van Weele, M., Pison, I., Bousquet, P., Frankenberg, C., Foster, P. N., Joos, F., Prentice, I. C., and van Velthoven, P.: Constraining global methane emissions and uptake by ecosystems, Biogeosciences, 8, 1643–1665, https://doi.org/10.5194/bg-8-1643-2011, 2011.
Sun, Q., Miao, C., Duan, Q., Ashouri, H., Sorooshian, S., and Hsu, K.-L.: A Review of Global Precipitation Data Sets: Data Sources, Estimation, and Intercomparisons, Rev. Geophys., 56, 79–107, https://doi.org/10.1002/2017RG000574, 2018.
Treat, C. C., Bloom, A. A., and Marushchak, M. E.: Nongrowing season methane emissions-a significant component of annual emissions across northern ecosystems, Glob. Change Biol., 24, 3331–3343, https://doi.org/10.1111/gcb.14137, 2018.
Tunnicliffe, R. L., Ganesan, A. L., Parker, R. J., Boesch, H., Gedney, N., Poulter, B., Zhang, Z., Lavrič, J. V., Walter, D., Rigby, M., Henne, S., Young, D., and O'Doherty, S.: Quantifying sources of Brazil's CH4 emissions between 2010 and 2018 from satellite data, Atmos. Chem. Phys., 20, 13041–13067, https://doi.org/10.5194/acp-20-13041-2020, 2020.
van Groenigen, K. J., Osenberg, C. W., and Hungate, B. A.: Increased soil emissions of potent greenhouse gases under increased atmospheric CO2, Nature, 475, 214–216, https://doi.org/10.1038/nature10176, 2011.
Wania, R., Melton, J. R., Hodson, E. L., Poulter, B., Ringeval, B., Spahni, R., Bohn, T., Avis, C. A., Chen, G., Eliseev, A. V., Hopcroft, P. O., Riley, W. J., Subin, Z. M., Tian, H., van Bodegom, P. M., Kleinen, T., Yu, Z. C., Singarayer, J. S., Zürcher, S., Lettenmaier, D. P., Beerling, D. J., Denisov, S. N., Prigent, C., Papa, F., and Kaplan, J. O.: Present state of global wetland extent and wetland methane modelling: methodology of a model inter-comparison project (WETCHIMP), Geosci. Model Dev., 6, 617–641, https://doi.org/10.5194/gmd-6-617-2013, 2013.
Wilson, C., Gloor, M., Gatti, L. V., Miller, J. B., Monks, S. A., McNorton, J., Bloom, A. A., Basso, L. S., and Chipperfield, M. P.: Contribution of regional sources to atmospheric methane over the Amazon Basin in 2010 and 2011: REGIONAL CH 4 SOURCES IN THE AMAZON BASIN, Global Biogeochem. Cy., 30, 400–420, https://doi.org/10.1002/2015GB005300, 2016.
Wilson, C., Chipperfield, M. P., Gloor, M., Parker, R. J., Boesch, H., McNorton, J., Gatti, L. V., Miller, J. B., Basso, L. S., and Monks, S. A.: Large and increasing methane emissions from eastern Amazonia derived from satellite data, 2010–2018, Atmos. Chem. Phys., 21, 10643–10669, https://doi.org/10.5194/acp-21-10643-2021, 2021.
Xi, Y., Peng, S., Ducharne, A., Ciais, P., Gumbricht, T., Jimenez, C., Poulter, B., Prigent, C., Qiu, C., Saunois, M., and Zhang, Z.: Gridded maps of wetlands dynamics over mid-low latitudes for 1980–2020 based on TOPMODEL, Sci. Data, 9, 347, https://doi.org/10.1038/s41597-022-01460-w, 2022.
Yin, Y., Chevallier, F., Ciais, P., Bousquet, P., Saunois, M., Zheng, B., Worden, J., Bloom, A. A., Parker, R. J., Jacob, D. J., Dlugokencky, E. J., and Frankenberg, C.: Accelerating methane growth rate from 2010 to 2017: leading contributions from the tropics and East Asia, Atmos. Chem. Phys., 21, 12631–12647, https://doi.org/10.5194/acp-21-12631-2021, 2021.
Ying, Q., Poulter, B., Watts, J. D., Arndt, K. A., Virkkala, A.-M., Bruhwiler, L., Oh, Y., Rogers, B. M., Natali, S. M., Sullivan, H., Schiferl, L. D., Elder, C., Peltola, O., Bartsch, A., Armstrong, A., Desai, A. R., Euskirchen, E., Göckede, M., Lehner, B., Nilsson, M. B., Peichl, M., Sonnentag, O., Tuittila, E.-S., Sachs, T., Kalhori, A., Ueyama, M., and Zhang, Z.: WetCH4: A Machine Learning-based Upscaling of Methane Fluxes of Northern Wetlands during 2016–2022, Earth Syst. Sci. Data Discuss. [preprint], https://doi.org/10.5194/essd-2024-84, in review, 2024.
Yuan, K., Li, F., McNicol, G., Chen, M., Hoyt, A., Knox, S., Riley, W. J., Jackson, R., and Zhu, Q.: Boreal–Arctic wetland methane emissions modulated by warming and vegetation activity, Nat. Clim. Change, 14, 282–288, https://doi.org/10.1038/s41558-024-01933-3, 2024.
Yvon-Durocher, G., Allen, A. P., Bastviken, D., Conrad, R., Gudasz, C., St-Pierre, A., Thanh-Duc, N., and del Giorgio, P. A.: Methane fluxes show consistent temperature dependence across microbial to ecosystem scales, Nature, 507, 488–491, https://doi.org/10.1038/nature13164, 2014.
Zhang, Y., Jacob, D. J., Lu, X., Maasakkers, J. D., Scarpelli, T. R., Sheng, J.-X., Shen, L., Qu, Z., Sulprizio, M. P., Chang, J., Bloom, A. A., Ma, S., Worden, J., Parker, R. J., and Boesch, H.: Attribution of the accelerating increase in atmospheric methane during 2010–2018 by inverse analysis of GOSAT observations, Atmos. Chem. Phys., 21, 3643–3666, https://doi.org/10.5194/acp-21-3643-2021, 2021b.
Zhang, Z.: Ensemble estimate of global Wetland Methane (CH4) Emissions (2000–2020), Zenodo [data set], https://doi.org/10.5281/zenodo.11309188, 2024.
Zhang, Z., Zimmermann, N. E., Stenke, A., Li, X., Hodson, E. L., Zhu, G., Huang, C., and Poulter, B.: Emerging role of wetland methane emissions in driving 21st century climate change, P. Natl. Acad. Sci. USA, 114, 9647–9652, https://doi.org/10.1073/pnas.1618765114, 2017.
Zhang, Z., Zimmermann, N. E., Calle, L., Hurtt, G., Chatterjee, A., and Poulter, B.: Enhanced response of global wetland methane emissions to the 2015–2016 El Niño-Southern Oscillation event, Environ. Res. Lett., 13, 074009, https://doi.org/10.1088/1748-9326/aac939, 2018.
Zhang, Z., Fluet-Chouinard, E., Jensen, K., McDonald, K., Hugelius, G., Gumbricht, T., Carroll, M., Prigent, C., Bartsch, A., and Poulter, B.: Development of the global dataset of Wetland Area and Dynamics for Methane Modeling (WAD2M), Earth Syst. Sci. Data, 13, 2001–2023, https://doi.org/10.5194/essd-13-2001-2021, 2021a.
Zhang, Z., Poulter, B., Knox, S., Stavert, A., McNicol, G., Fluet-Chouinard, E., Feinberg, A., Zhao, Y., Bousquet, P., Canadell, J. G., Ganesan, A., Hugelius, G., Hurtt, G., Jackson, R. B., Patra, P. K., Saunois, M., Höglund-Isaksson, L., Huang, C., Chatterjee, A., and Li, X.: Anthropogenic emission is the main contributor to the rise of atmospheric methane during 1993–2017, Nat. Sci. Rev., 9, nwab200, https://doi.org/10.1093/nsr/nwab200, 2021c.
Zhang, Z., Poulter, B., Feldman, A. F., Ying, Q., Ciais, P., Peng, S., and Li, X.: Recent intensification of wetland methane feedback, Nat. Clim. Change, 13, 430–433, https://doi.org/10.1038/s41558-023-01629-0, 2023a.
Zhang, Z., Bansal, S., Chang, K.-Y., Fluet-Chouinard, E., Delwiche, K., Goeckede, M., Gustafson, A., Knox, S., Leppänen, A., Liu, L., Liu, J., Malhotra, A., Markkanen, T., McNicol, G., Melton, J. R., Miller, P. A., Peng, C., Raivonen, M., Riley, W. J., Sonnentag, O., Aalto, T., Vargas, R., Zhang, W., Zhu, Q., Zhu, Q., Zhuang, Q., Windham-Myers, L., Jackson, R. B., and Poulter, B.: Characterizing Performance of Freshwater Wetland Methane Models Across Time Scales at FLUXNET-CH4 Sites Using Wavelet Analyses, J. Geophys. Res.-Biogeo., 128, e2022JG007259, https://doi.org/10.1029/2022JG007259, 2023b.
Zhu, Q., Peng, C., Ciais, P., Jiang, H., Liu, J., Bousquet, P., Li, S., Chang, J., Fang, X., Zhou, X., Chen, H., Liu, S., Lin, G., Gong, P., Wang, M., Wang, H., Xiang, W., and Chen, J.: Interannual variation in methane emissions from tropical wetlands triggered by repeated El Niño Southern Oscillation, Glob. Change Biol., 23, 4706–4716, https://doi.org/10.1111/gcb.13726, 2017.
Zona, D., Gioli, B., Commane, R., Lindaas, J., Wofsy, S. C., Miller, C. E., Dinardo, S. J., Dengel, S., Sweeney, C., Karion, A., Chang, R. Y.-W., Henderson, J. M., Murphy, P. C., Goodrich, J. P., Moreaux, V., Liljedahl, A., Watts, J. D., Kimball, J. S., Lipson, D. A., and Oechel, W. C.: Cold season emissions dominate the Arctic tundra methane budget, P. Natl. Acad. Sci. USA, 113, 40–45, https://doi.org/10.1073/pnas.1516017113, 2016.