the Creative Commons Attribution 4.0 License.
the Creative Commons Attribution 4.0 License.
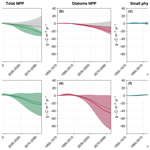
Toward more robust net primary production projections in the North Atlantic Ocean
Stéphane Doléac
Marina Lévy
Roy El Hourany
Laurent Bopp
Phytoplankton plays a crucial role in both climate regulation and marine biodiversity, yet it faces escalating threats due to climate change. Understanding future changes in phytoplankton biomass and productivity under climate change requires the utilization of Earth system models capable of resolving marine biogeochemistry. These models often differ significantly from one another, and most studies typically use the average response across an ensemble of models as the most reliable projection. However, in the North Atlantic, this straightforward method falls short of providing robust projections of phytoplankton net primary production (NPP) over the 21st century. A new inter-comparison approach was therefore developed and applied to eight models from the sixth phase of the Coupled Model Intercomparison Project (CMIP6) exhibiting substantial divergence in their NPP projections in the North Atlantic. The basin was first divided into three bioregions tailored to the characteristics of each model using a novel method based on a clustering procedure. The mechanisms controlling NPP projections were then identified in each model and in each bioregion, revealing two mechanisms responsible for a large part of model divergence: diazotrophy in the subtropical region and the presence of an ammonium pool in the subpolar region. This allowed for an informed selection of models in each region based on the way they represent these two mechanisms, resulting in reduced projection uncertainty, enhanced total NPP decrease in the subtropical region, and a strengthened increase in small phytoplankton NPP in the subpolar North Atlantic. These model selections enhanced the decreases in carbon export and phytoplankton biomass but had no impact on zooplankton biomass. This innovative approach has strong synergies with other widely used inter-comparison techniques, such as emergent constraints, and their combination would provide valuable insights into the future trajectory of the Earth's climate system.
- Article
(5712 KB) - Full-text XML
- BibTeX
- EndNote
The world's oceans are facing increasing pressures due to climate change, leading to significant alterations in their physical and biogeochemical conditions. Sea surface temperature (SST) has risen by an average of 0.88 °C between 1850–1900 and 2011–2020 (Fox-Kemper et al., 2021), pH has declined by 0.016 to 0.020 units per decade in the subtropical regions since the 1980s (Canadell et al., 2021), and upper-ocean stratification has increased by 5.5 ± 1.0 % between 1960 and 2018 (Li et al., 2020). These changes pose a threat to the oceans' ability to provide essential environmental services that many people rely on, such as fisheries or carbon sequestration. Phytoplankton is key to many of these services, and understanding how climate change will impact it is of primary importance but still remains an open question.
The global distribution of phytoplankton is highly heterogeneous and is strongly influenced by ocean dynamics. The North Atlantic Ocean displays sharp contrasts, featuring a true “sea desert” in its subtropical section and one of the most productive regions on Earth in its subpolar part (Behrenfeld and Falkowski, 1997; Westberry et al., 2008). This high productivity is notably facilitated by a nutrient stream associated with the Atlantic Meridional Overturning Circulation (AMOC), which transports nutrients from equatorial and southern regions to the North Atlantic (Williams et al., 2006, 2011; Palter and Lozier, 2008), and by deep convection areas that bring large quantities of nutrients to the surface (Abot et al., 2023). This elevated productivity supports rich ecosystems and, combined with ocean dynamics, enables the North Atlantic Ocean to sequester large amounts of organic carbon through the biological carbon pump (Nowicki et al., 2022).
Climate change has both direct and indirect effects on phytoplankton net primary production (NPP). Directly, NPP increases with rising temperatures (Eppley, 1972; Sauterey et al., 2023). Indirectly, climate change impacts nutrient supply and light availability. Nutrient fluxes are influenced locally and regionally by increased vertical stratification, which reduces vertical supplies (Bopp et al., 2013), and globally by the projected decrease in the AMOC intensity (Whitt, 2019). Sea ice retreat reduces light limitation in the polar region (Vancoppenolle et al., 2013), while zooplankton grazing is also affected by global warming (Laufkötter et al., 2015; Rohr et al., 2023). The impacts of these mechanisms vary regionally and affect the different phytoplankton groups differently, potentially causing shifts in community structures and total abundance (Kléparski et al., 2023; Benedetti et al., 2021).
Given this intricate complexity, projecting the precise impact of climate change on phytoplankton NPP poses significant challenges. Earth system models (ESMs) from the Coupled Model Intercomparison Project (CMIP) include most of the above processes and thus offer a comprehensive approach to evaluating climate change impact on NPP. CMIP provides standardized experimental protocols (Eyring et al., 2016) based on shared socioeconomic pathways (O'Neill et al., 2014, 2016), enabling comparison of projections across models. Traditionally, all available models from the same CMIP phase are studied together and are used to assess a projection uncertainty around the multi-model mean (Bopp et al., 2013; Fu et al., 2016; Kwiatkowski et al., 2020; Tagliabue et al., 2021). Each model is given the same weight, and the multi-model mean is often considered the best available projection, as it is assumed to be less impacted by the particular sets of parameterizations used in individual models. However, Kwiatkowski et al. (2020) have shown that in the case of NPP projections with models from the sixth phase of CMIP (CMIP6), there was a strong divergence in NPP projections between models at the global scale. This was confirmed in particular regions such as the Indian and Atlantic oceans by Tagliabue et al. (2021). Thus, in terms of NPP, the multi-model mean contains little information and may not be considered a reliable estimate of its future evolution. An approach different from the multi-model mean is therefore needed to assess how NPP will respond to climate change under a given projection scenario.
Several approaches have already been proposed and shown to effectively reduce projection uncertainty. Some studies advocate for model weighting based on their ability to replicate past observations (Sanderson et al., 2017; Knutti et al., 2017), while others highlight the use of emergent constraints as a promising method for reducing projection uncertainty (Eyring et al., 2019; Kwiatkowski et al., 2017). Additionally, in-depth analyses have been conducted to explore the physical drivers of NPP, attributing model discrepancies to variations in ocean physics and their interactions with biogeochemical variables (Whitt, 2019; Xiu et al., 2018; Mousing et al., 2023). However, many of these studies tend to overlook the significant differences in the biogeochemical components of CMIP6 models (Kearney et al., 2021; Séférian et al., 2020). Yet, it is well-established that certain processes, depending on their inclusion and parameterization, can substantially influence NPP projections (Bopp et al., 2022; Rohr et al., 2023).
Building on these insights, the present study seeks to go a step further by linking part of the divergence in NPP projections to specific differences in the biogeochemical processes and pools represented across CMIP6 models. To achieve this, we identify the processes driving long-term NPP evolution under the SSP5-8.5 scenario in each model and use these insights to assess the reliability of their projections. This process-based evaluation will then inform a model selection aimed at reducing projection uncertainties.
The North Atlantic Ocean, a highly productive region with significant future divergence between model projections (Tagliabue et al., 2021), will serve as a case study. Given that the processes controlling NPP vary across different regions, we divide the North Atlantic Basin into three bioregions (polar, subpolar, and subtropical) using a clustering method and evaluate each subregion individually. Finally, we explore how our revised estimates of NPP projections influence carbon export and plankton biomass – key indicators of the biological carbon pump and marine ecosystem health – and how our approach integrates with the other methods mentioned above.
2.1 CMIP6 models
All the CMIP6 models assessed in this study include an ocean biogeochemistry component. For both the historical period and the SSP5-8.5 scenario (O'Neill et al., 2016), a prerequisite for each model was to have a minimum of three ensemble members with available outputs for primary production and for the five clustering variables employed to construct bioregions (chlorophyll a surface concentrations, sea surface temperature (SST), mixed-layer depth (MLD), sea ice concentration, and nitrate surface concentrations). Out of the 16 models with primary production projections under the SSP5-8.5 scenario, only 8 fulfill these criteria. Among the eight excluded models, six have only one member, and the remaining two (CNRM-ESM2-1 and MIROC-ES2L) lack one clustering variable for several or all members. Details about the characteristics of the remaining eight models can be found in Table 1.
Ziehn et al. (2020); Oke et al. (2013)Moore et al. (2001)Moore et al. (2001)Swart et al. (2019); Christian et al. (2022); Zahariev et al. (2008)Swart et al. (2019); Christian et al. (2022)Boucher et al. (2020); Aumont et al. (2015)Mauritsen et al. (2019); Paulsen et al. (2017)Sellar et al. (2019); Yool et al. (2013)Table 1Characteristics of the eight CMIP6 models studied. The phytoplankton group diazotrophs (param.) refers to models with an implicit representation of N2 fixation.
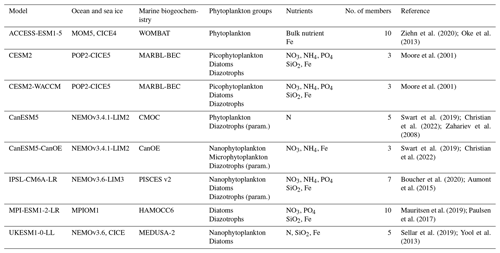
Ensemble means for each model were computed to eliminate a maximum amount of natural variability, allowing us to focus on the impact of anthropogenic climate change. Trends were derived by averaging ensemble mean outputs over 20-year periods every 5 years from 1950–1970 to 2080–2100. These trends were calculated over the region between 10–80° N and 270–360° E, referred to as the North Atlantic Ocean in the subsequent analysis. The period 1950–1970 was taken as the reference, and all projections were expressed as the evolution between this reference period and 2080–2100.
The complexity of ESM marine biogeochemistry components varies significantly from one model to another. Among the eight models selected (Table 1), the simplest model (CMOC) only includes one nutrient and one phytoplankton class (Zahariev et al., 2008), whereas the most complex model (MARBL-BEC) features five nutrients and three phytoplankton classes (Moore et al., 2001; see Kearney et al. (2021) for a summary of the biogeochemistry components of CMIP6 models). Due to these differences, each model is studied individually to comprehensively grasp the mechanisms controlling NPP projections.
Three phytoplankton groups were considered in this study: diatoms, diazotrophs, and small phytoplankton. The last category encompasses classes labeled nanophytoplankton, picophytoplankton, and bulk phytoplankton when sensitivity to silicate is absent. In MPI-ESM1-2-LR, the bulk phytoplankton class is grouped with diatoms due to its reliance on SiO2 (Ilyina et al., 2013), along with the large phytoplankton class in CanESM5-CanOE, despite the absence of SiO2 in the model (Christian et al., 2022). Furthermore, CMIP6 models can be categorized into three groups based on their representation of diazotrophy:
-
Models without any diazotrophy. These include ACCESS-ESM1-5 and UKESM1-0-LL.
-
Models with an explicit group of diazotrophs. These include CESM2, CESM2-WACCM, and MPI-ESM1-2-LR. In these models, diazotroph growth rate is not constrained by nitrogen; they can utilize atmospheric N2 but depend on PO4 and Fe availability (Moore et al. (2001) for CESM2 and CESM2-WACCM; Paulsen et al. (2017) for MPI-ESM1-2-LR).
-
Models with an implicit representation of diazotrophs. These include IPSL-CM6A-LR, CanESM5, and CanESM5-CanOE. N2 fixation is parameterized, and its interaction with other nutrients varies based on this parameterization. In IPSL-CM6A-LR, the initial parameterization from PISCES v2 was used (Aumont et al., 2015; Bopp et al., 2022).
CMIP6 models can also be distinguished based on how they represent nitrogen and phosphorus (Table 1). Among the eight models studied, three (ACCESS-ESM1-5, CanESM5, and UKESM1-0-LL) use a unique bulk nutrient meant to represent both nitrogen and phosphorus. In contrast, three others (IPSL-CM6A-LR, CESM2, and CESM2-WACCM) include a PO4 pool along with two nitrogen pools, NO3 and NH4. The CanESM5-CanOE model includes two nitrogen pools (NO3 and NH4) but lacks a PO4 pool. Lastly, MPI-ESM1-2-LR represents both a PO4 and an NO3 pool but does not include NH4. In these CMIP6 models, over our study region, there is an almost-perfect correlation between projected surface NO3 concentrations and those integrated over the first 100 m (not shown). This makes surface nutrient concentrations an excellent proxy for changes occurring throughout the euphotic layer. Therefore, we chose to use surface concentrations for simplicity in the following analysis.
2.2 Construction of bioregions
The processes governing changes in NPP vary across different regions, necessitating a regional approach. Bioregions are the most suitable framework for conducting such a study because each bioregion corresponds to a specific production regime. However, their boundaries align with physical phenomena such as oceanic currents and sea ice, the precise localization of which varies among models. Consequently, fixed bioregions, like those established by Longhurst (2007) and used in Tagliabue et al. (2021), have limitations for inter-comparison studies.
To address this limitation, a novel approach was developed to establish bioregions tailored to the characteristics of each model. They were constructed using the self-organizing map (SOM) + hierarchical agglomerative clustering (HAC) procedure (defined in the following section), a robust method for extracting temporal and spatial patterns from large datasets. This procedure was first applied to the observed mean seasonal cycles over the recent historical period of five clustering variables, chosen for their relevance in explaining long-term NPP evolution and their availability in CMIP6 model outputs. The bioregions obtained were then replicated in each model. By employing this method, we ensured that each bioregion corresponded to a similar production regime across all models.
2.2.1 The SOM + HAC procedure
The SOM algorithm (Kohonen, 2013) is widely used for pattern identification and clustering analysis for large multi-variate datasets. In environmental sciences, it has been used to study precipitation patterns (Derouiche et al., 2022; Dilmi et al., 2017), monsoon weather regimes (Guèye et al., 2011)), phytoplankton biomes (El Hourany et al., 2021; Hofmann Elizondo et al., 2021), the oceanic circulation (Jouini et al., 2016), and aerosol concentrations (Yahi et al., 2013) or to identify biophysical regions in the Beaufort Sea (Hilborn and Devred, 2022). Similar to classical vector quantization procedures, such as k-means clustering, the SOM algorithm condensates the information contained in a multi-variate dataset into an optimal number of “neurons”, each associated with a vector characterizing a subset of the initial dataset. However, unlike k-means clustering, the neurons in the SOM algorithm are not independent. They are distributed over a 2D map with a topological constraint such that close neurons on the map are similar. Practically, this constraint means that, during the learning phase, neurons are not updated alone but as batches of close neurons, thus allowing the propagation of local updates to the global neuron map. This topological constraint allows for the preservation of the topological properties of the initial dataset. An advantage of the SOM algorithm over k-means clustering procedures is that it is robust to missing data (Vatanen et al., 2015), making it particularly interesting in the regions seasonally covered by sea ice.
When handling environmental data, the specific quantity of classes or neurons is not predetermined. In cases where the SOM is employed for vector quantization, a large number of neurons are utilized, each assigned to a geographic subregion. To determine major patterns among these neurons, an HAC is employed on them while preserving the topological constraints. This procedure is denoted SOM + HAC hereafter. The HAC produces a dendrogram offering various suggestions for estimating the number of classes. A balance is struck between the number of classes that can be logically explained and the number required to encompass the data's embedded information. This approach has demonstrated success in numerous studies (Farikou et al., 2015; Jouini et al., 2016; El Hourany et al., 2021; Baaklini et al., 2022).
2.2.2 The datasets used to build bioregions
To ensure that the bioregions obtained in each model were similar, they were first constructed from the observations before being reproduced in the models (see the following subsection). Here, we describe the datasets of observations used to build these bioregions.
Surface chlorophyll a concentrations were extracted from the GlobColour merged product distributed by the Copernicus Marine Service (MULTIOBS_GLO_PHY_TSUV_3D_MYNRT_015_012; Guinehut et al., 2012; Mulet et al., 2012). It provides daily outputs of surface chlorophyll concentrations over the global ocean from September 1997 to the present. Data from the period 2000–2020 were used.
Sea surface temperature and mixed-layer depth data were extracted from the Multi Observation Global Ocean 3D product from the Copernicus Marine Service (MULTIOBS_GLO_PHY_TSUV_3D_MYNRT_015_012). It provides weekly and monthly values of six variables, including SST and MLD, over the global ocean between 1993 and 2023, built from both in situ and satellite observations. Again, data from the period 2000–2020 were used.
NO3 concentrations were extracted from the World Ocean Atlas 2018 database (Garcia et al., 2018), while sea ice concentration data came from the EUMETSAT OSI SAF SSMIS product v2.0, distributed by the Copernicus Climate Change Service, and covered the period 1998–2015.
2.2.3 Application of the clustering procedure
The SOM + HAC was first applied to the historical mean seasonal cycles of five clustering variables (surface chlorophyll concentrations, surface nitrate concentrations, mixed-layer depth, sea ice concentration, and sea surface temperature) computed from the datasets previously described. They were regridded to 1° × 1° regular grids, concatenated, and the coastal areas were excluded by removing values from grid cells adjacent to the continents. The North Atlantic Ocean was thus represented by 3335 grid points, each associated with a vector of dimension 60, corresponding to the five successive climatological seasonal cycles. To ensure equal weighting of all variables in the clustering process, the dataset was normalized beforehand. Several normalization methods, such as those outlined in Derouiche et al. (2022), Dilmi et al. (2017), and Hilborn and Devred (2022), can be employed. It was decided to normalize each seasonal cycle by dividing it by the absolute maximum value reached over the entire period and region. This normalization method has the advantage of preserving the dataset structure while ensuring all values fall within the range of −1 and 1. The selection of clustering variables resulted from a compromise between their relevance in explaining primary production variability in the North Atlantic Ocean and their availability in CMIP6 model outputs. For instance, silicate concentration was excluded, despite its acknowledged impact on primary production in the subpolar gyre (Hátún et al., 2017; Sieracki et al., 1993), as only five out of eight models provided the output.
The neurons obtained from the observations were then used to replicate similar bioregions in the CMIP6 models. In each model, a matrix akin to that of the observations was reproduced with the average seasonal cycles over the period 2000–2020, using the same normalization method and constants. Each grid point in each model was subsequently assigned to its nearest neuron based on Euclidean distance and to the cluster associated with that neuron, thereby reproducing the bioregions in each model. This assignment was carried out regardless of the distance between the grid point and the neuron. Consequently, the regions obtained were the closest to those built from the observations but could be rather different from them.
3.1 Global projections
Primary production projections over the whole North Atlantic Ocean with CMIP6 models exhibit a large spread (Fig. 1a). Among the eight models, five (UKESM1-0-LL, CESM2, CESM2-WACCM, MPI-ESM1-2-LR, and CanESM5) project a strong decrease in net primary production (from −33.6 to −11.4 in 2080–2100 with respect to 1950–1970), while the remaining three (CanESM5-CanOE, IPSL-CM6A-LR, ACCESS-ESM1-5) project an increase (from +8.0 to +17.0 ). On average, CMIP6 models project an NPP decrease of −10.4 . This range is similar to the one obtained with the first member of the 16 models used in Tagliabue et al. (2021). Therefore, the selected subset of eight models is considered representative of the broader ensemble of CMIP6 models.
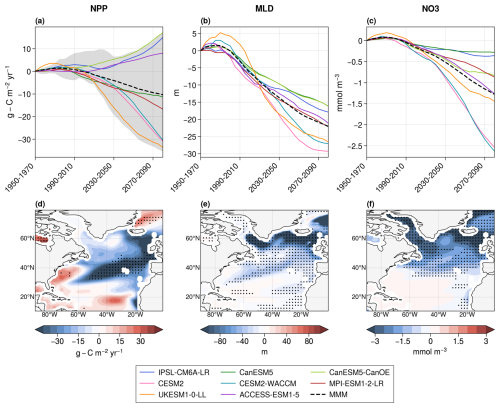
Figure 1Projections of NPP (a), mixed-layer depth (b), and surface NO3 concentrations (c) in the eight CMIP6 models over the whole North Atlantic Ocean. The dotted black lines are the multi-model means (MMMs), and the shaded area in the NPP panel corresponds to the range of projections with the first member of the 16 models used in Tagliabue et al. (2021). Multi-model means of the projected changes in NPP (d), mixed-layer depth (e), and surface NO3 concentrations (f) between 1950–1970 and 2080–2100. Stippling indicates areas of robust projections, defined by an 80 % agreement in the sign of change across models.
There is confidence in the sign of NPP projections in the northeastern section of the subtropical gyre and along Greenland's eastern coast only (Fig. 1d). Confidence is defined here, following Kwiatkowski et al. (2020), as at least 80 % of the projections having the same sign. Models disagree on the sign of the future evolution of NPP in most of the subpolar gyre and in the southern subtropical gyre. This lack of confidence happens despite agreement between models on the sign of the long-term evolution of MLD and surface nitrate concentrations, particularly in the subpolar North Atlantic Ocean (Fig. 1e and f). On average over the region, all models project a decrease in MLD and NO3 concentrations, with a multi-model mean decrease of −22.1 m and −1.3 mmol m−3, respectively.
3.2 Construction of the bioregions
The SOM + HAC procedure identified an optimal number of four bioregions in the observations: one subtropical region, one polar region, and two subpolar regions (Fig. 2). The subtropical bioregion, the largest at 20.8 million km2, exhibits an oligotrophic regime characterized by very low nitrate and chlorophyll a concentrations (maximum annual values of 0.87 mmol m−3 and 0.16 mg m−3, respectively). It is situated over the subtropical gyre and the Caribbean Sea, bordered by the North Atlantic Current to the north. The polar region, the smallest at 1.0 million km2, stands out due to the extensive presence of sea ice during winter and is further divided into two subregions: the Baffin Sea and the eastern Greenland Sea. The two subpolar regions, with surfaces of 3.3 and 4.8 million km2, respectively, correspond to the intergyre region with the northern part of the Labrador Sea and the northern subpolar gyre with the Norwegian Sea. The latter region exhibits a deeper winter mixed layer, higher nitrate surface concentrations, and a delayed bloom compared to the former. However, the two regions do not significantly differ in terms of annual maximum chlorophyll a concentrations (0.76 mg m−3 in August for the former and 0.80 mg m−3 in September for the latter).
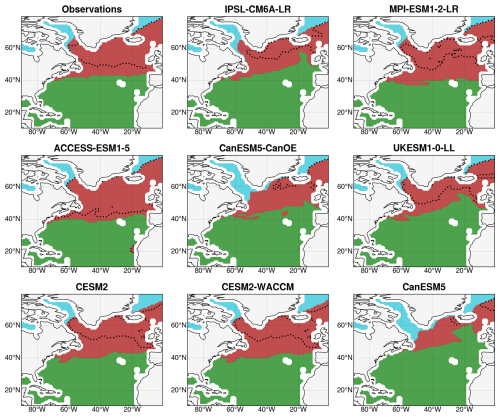
Figure 2Bioregions built with the SOM + HAC procedure from the historical seasonal cycles of chlorophyll a concentrations, nitrate concentrations, SST, MLD, and sea ice concentration and their reproduction in CMIP6 models for the period 2000–2020. The subtropical region is in green, the subpolar region is in red, and the polar region is in cyan. The dotted black lines indicate the boundary between the two original subpolar bioregions.
Qualitatively, the models reproduce the historical bioregions obtained from observations fairly well, in terms of both localization and physical boundaries, despite variations in surfaces (Fig. 2). The subtropical region's surface ranges from 19.1 million km2 in MPI-ESM1-2-LR to 24.1 million km2 in CanESM5, while the polar region's surface varies from 0.9 million km2 in MPI-ESM1-2-LR to 2.0 million km2 in CanESM5. The overall subpolar region is relatively consistent across different models, but the relative importance of the subregions varies significantly from one model to another. It was decided to merge the subpolar subregions into one large subpolar region, supported by strong inter-model correlations between the projections of NPP in the two regions (r = 0.91; p = 0.002), indicating a similar dominant mechanism. The three resulting bioregions are presented for the observations and each model in Fig. 2. Each of these bioregions will now be studied successively to determine the mechanisms controlling NPP projections.
3.3 Regional analysis
3.3.1 Subtropical region
NPP projections strongly diverge in the subtropical region, with three models projecting an increase (up to +19.5 in 2080–2100 with respect to the 1950–1970 mean), while the remaining five project a decrease (down to −33.4 ) (Fig. 3a). These divergent groups align with those observed for the entire North Atlantic Ocean (Fig. 1a), suggesting that the subtropical region predominantly influences the basin-scale divergence of projections.
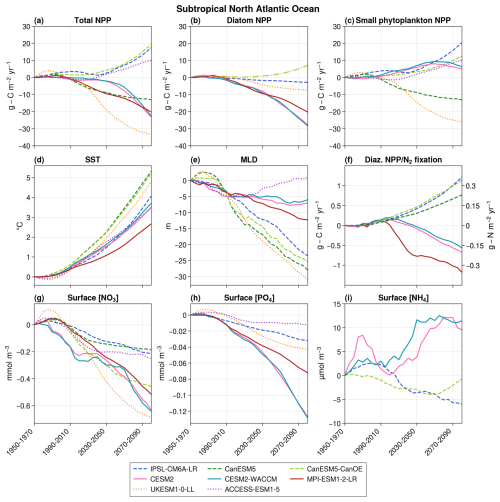
Figure 3Projections over the subtropical bioregion of total NPP (a), diatom NPP (b), small phytoplankton NPP (c), SST (d), MLD (e), diazotrophs NPP/N2 fixation (f), surface nitrate concentrations (g), surface phosphate concentrations (h), and surface ammonium concentrations (i). The average value over 1950–1970 is taken as the reference. The dotted lines represent models without diazotrophy, dashed lines correspond to models with parameterized N2 fixation, and solid lines indicate models with an explicit diazotroph group. In panel (f), diazotroph NPP is shown on the left axis for models with an explicit diazotroph group, while N2 fixation is plotted on the right axis for models with parameterized N2 fixation. In panel (b), CanESM5-CanOE is represented by a dashed–dotted line to distinguish it from the other models. This distinction is made because it is the only model that includes a diatom group without SiO2 and in which both diatoms and small phytoplankton are equally efficient at utilizing NH4.
This substantial discrepancy occurs despite coherent evolutions across models for other crucial physical and biogeochemical variables (Fig. 3). Notably, sea surface temperature (SST) increases in all models (ranging from +2.7 °C in MPI-ESM1-2-LR to 5.3 °C in CanESM5), while surface NO3 concentrations and surface PO4 concentrations decrease (NO3 concentrations from −0.19 to −0.69 mmol m−3; PO4 concentrations from −0.01 to −0.13 mmol m−3). Mixed-layer depth and carbon export at 100 m depth both decrease in all models but one (ACCESS-ESM1-5 and IPSL-CM6A-LR, respectively), in which they increase after a period of decrease.
Small phytoplankton's NPP exhibits a strong discrepancy. The only variable able to cause this divergence is N2 fixation, for which a clear separation appears between models with an implicit representation of diazotrophs, which project a strong increase in N2 fixation, and those with an explicit representation, which project a decrease in diazotrophs' NPP (Fig. 3). As detailed in the Methodology section, CMIP6 models can be categorized into three groups according to their representation of N2 fixation. We now examine each of these groups individually.
The two models with no diazotrophy project different primary production evolutions (ACCESS-ESM1-5 projects an increase of 10.0 , while UKESM1-0-LL projects a decrease of −33.4 ). In UKESM1-0-LL, the production of both small phytoplankton and diatoms decreases (Fig. 3). This discrepancy is mainly attributed to differences in annual maximum surface nitrate concentrations (1.0 and 0.3 mmol m−3 on average, respectively, in 2000–2020, whereas, according to the World Ocean Atlas 2018, the real value is 0.4 mmol m−3). In UKESM1-0-LL, nitrates are strongly limiting, and their declining trend (see Fig. 3g) leads to a decreased primary production for all phytoplankton groups. In ACCESS-ESM1-5, nitrates are not a strong limitation on average across the entire subtropical region, and the mechanisms causing NPP increase are more complex. Between 1950 and 2020, the opposing effects of rising SST and decreasing surface nutrient concentrations – partly due to mixed-layer shallowing – largely balance each other out, resulting in stable NPP. However, from 2020 to 2100, a recovery in MLD slows the decline in nutrient concentrations, allowing the SST effect to dominate, which leads to an increase in NPP.
The three models with an explicit representation of diazotrophs all project a decrease in both total primary production and N2 fixation. This decrease affects both diazotrophs and diatoms in these models, while small phytoplankton primary production in CESM2 and CESM2-WACCM initially increases before decreasing (Fig. 3b, c, and f). This coherence across phytoplankton groups suggests that the mechanism influencing primary production evolution could affect all of them, ruling out nitrates as a potential candidate, as they do not impact diazotrophs. The only variable capable of explaining these evolutions is PO4 concentrations, influencing all phytoplankton groups, consistently decreasing in all three models and known to limit N2 fixation in the region in CESM models (Wang et al., 2019). Additionally, in CESM2 and CESM2-WACCM, small phytoplankton has a lower PO4 half-saturation constant than diazotrophs and diatoms (0.00025, 0.0005, and 0.00125 mmol m−3, respectively; Moore et al., 2001), enabling them to thrive in environments with low PO4 concentrations. This explains the initial increase in their primary production before declining when PO4 concentrations become too low. Consequently, in models with an explicit representation of diazotrophs, decreasing PO4 concentrations lead to a reduction in overall primary production, including diazotrophs, subsequently causing a decrease in N2 fixation.
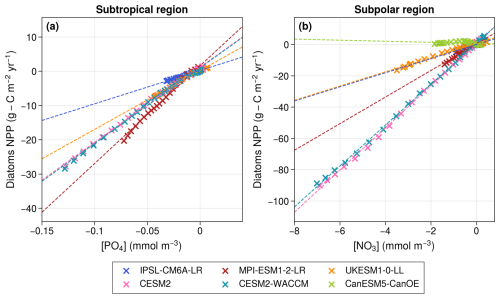
Figure 4Scatter plots representing the change in diatom NPP relative to 1950–1970 versus the change in surface PO4 concentrations in the subtropical region (a) and NO3 concentrations in the subpolar region (b). CanESM5-CanOE appears only in the subpolar panel due to the absence of PO4 in the model. The dashed lines represent linear regressions, all of which are significant at the p < 0.05 level, and show correlations greater than 0.96, except for CanESM5-CanOE, where the correlation is not significant (p = 0.11).
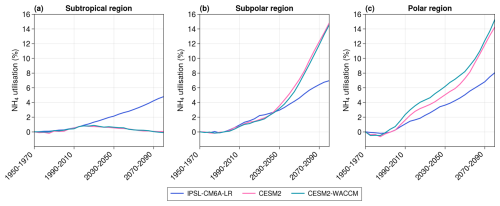
Figure 5Projections of the proportion of total NPP using NH4 rather than NO3 as a nitrogen source in the subtropical (a), subpolar (b), and polar (c) regions. The average value over 1950–1970 is taken as the reference. CanESM5-CanOE is not included in this figure, despite its representation of NH4, because the variable “intppnitrate”, used to construct this figure, is not available for that model.
The three models with parameterized diazotrophy exhibit contrasting behaviors in their total NPP projections. While IPSL-CM6A-LR and CanESM5-CanOE project an increase in total NPP, CanESM5 projects a decrease (Fig. 3a). In IPSL-CM6A-LR, the absence of effective control of PO4 concentrations over N2 fixation was identified as being responsible for the substantial increase in diazotrophy and primary production in subtropical oceans (Bopp et al., 2022). The increase in N2 fixation replenishes the NH4 pool (Aumont et al., 2015), leading to a greater proportion of total NPP being supported by NH4 rather than NO3 as a nitrogen source (Fig. 5a). This mechanism primarily benefits small phytoplankton, which are more efficient at consuming nutrients at low concentrations, such as NH4 (Aumont et al., 2015). As a result, small phytoplankton NPP increases in IPSL-CM6A-LR despite the decline in NO3 concentrations. The slight decrease in surface NH4 concentrations can be explained by the increased consumption by small phytoplankton. However, this process does not benefit diatoms, whose long-term growth is regulated by PO4 (Fig. 4a), leading to a decline in their NPP. In CanESM5-CanOE, N2 fixation similarly replenishes the ammonium pool, and the same trends as in IPSL-CM6A-LR are observed for small phytoplankton NPP and surface NH4 concentrations. Although we cannot compute the proportion of total NPP based on NH4 versus NO3 for CanESM5-CanOE due to the lack of data (Fig. 5), the similar behaviors between the models suggest that the mechanism is the same: the additional NH4 from N2 fixation supports the increase in small phytoplankton NPP. However, unlike IPSL-CM6A-LR, CanESM5-CanOE also shows an increase in diatom NPP. This can be attributed to the absence of PO4 in CanESM5-CanOE, which cannot control diatom NPP, and the fact that, unlike in IPSL-CM6A-LR, the half-saturation constants for ammonium are the same for diatoms and small phytoplankton (Christian et al., 2022). As a result, diatoms are as efficient as small phytoplankton in consuming NH4, allowing them to benefit from N2 fixation and leading to a rise in their NPP. Finally, in CanESM5, a single nitrogen pool combines both ammonium and nitrate. Despite the strong increase in N2 fixation, nitrogen concentrations in the subtropical region decrease overall (Fig. 3g), resulting in a decline in total NPP (Fig. 3a).
Diatom NPP decreases and is almost perfectly correlated with the evolution of surface PO4 concentrations (r≥ 0.99, p≪ 0.01) in all models except CanESM5-CanOE (Fig. 4a). As explained in the previous paragraph, the different behavior of the diatom group in CanESM5-CanOE is explained by the absence of PO4 in the model and by its high sensitivity to NH4. In the remaining models, the inter-model spread in diatom NPP projections is primarily driven by differences in the sensitivity of diatoms to declining PO4 concentrations.
The interactions between N2 fixation, phosphates, and small phytoplankton therefore appear to be a strong diverging factor between models, with the potential to partially decouple total NPP from NO3 concentrations. The models with the most realistic representations of diazotrophy (CESM2, CESM2-WACCM, and MPI-ESM1-2-LR) all project a decrease in NPP because of the declining PO4 concentrations. The strong increases observed in some models are caused by either nitrate concentrations that are too high when no diazotrophy is represented or their unchecked influence by PO4 concentrations, making such scenarios unlikely.
3.3.2 Subpolar region
NPP projections also significantly diverge in the subpolar region (Fig. 6a). Three models (CESM2, CESM2-WACCM, and UKESM1-0-LL) project a substantial decrease (−54.3, −53.7, and −44.7 , respectively, in 2080–2100 with respect to 1950–1970), while three others (IPSL-CM6A-LR, CanESM5-CanOE, and ACCESS-ESM1-5) project a modest increase (+5.4, +6.6, and +3.8 , respectively). The remaining two models project an intermediate decline (−12.7 in MPI-ESM1-2-LR and −10.0 in CanESM5). This disparity persists despite consistent trends across models for other physical and biogeochemical variables, such as SST, MLD, AMOC intensity, and NO3 and PO4 surface concentrations (Fig. 6). However, the breakdown of NPP projections into the different phytoplankton groups highlights that it is predominantly small phytoplankton that explains the model disagreement on the sign of total NPP future evolution (Fig. 6).
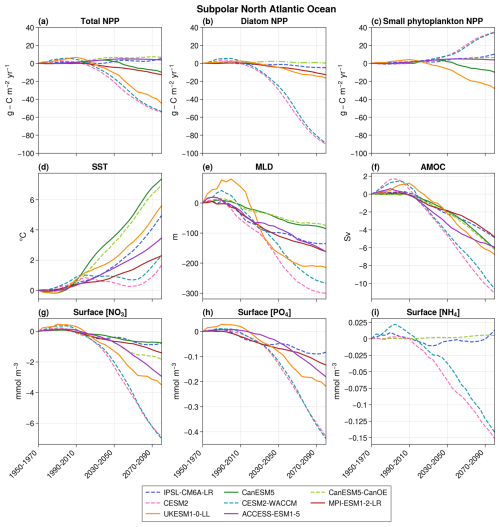
Figure 6Projections over the subpolar bioregion of total NPP (a), diatom NPP (b), small phytoplankton NPP (c), SST (d), MLD (e), AMOC (f), surface nitrate concentrations (g), surface phosphate concentrations (h), and surface ammonium concentrations (i). The average value over 1950–1970 is taken as the reference. The dashed lines represent models with an ammonium pool, and solid lines indicate models without NH4. In panel (b), CanESM5-CanOE is represented by a dashed–dotted line to distinguish it from the other models. This distinction is made because it is the only model that includes a diatom group without SiO2 and in which both diatoms and small phytoplankton are equally efficient at utilizing NH4.
Five out of seven models project an increase in small phytoplankton NPP, despite a consistent decrease in NO3 and PO4 concentrations across models (Fig. 6). Of these five, four (IPSL-CM6A-LR, CanESM5-CanOE, CESM2, and CESM2-WACCM) include an ammonium pool. In IPSL-CM6A-LR, CESM2, and CESM2-WACCM, the proportion of total NPP derived from NH4 rather than NO3 significantly increases (Fig. 5b). As observed in the subtropical region, this rise offsets the impact of decreasing NO3 concentrations, leading to an increase in small phytoplankton NPP, along with a decrease in surface NH4 concentrations in CESM2 and CESM2-WACCM. However, in these models, diatoms are strongly dependent on NO3 (Fig. 4), and their NPP declines accordingly. For CanESM5-CanOE, it is not possible to compute the proportion of total NPP supported by NH4 versus NO3, but it can be hypothesized that NH4 similarly sustains NPP, which would explain the slight increase in small phytoplankton NPP and the stagnation in diatom NPP. Notably, only in IPSL-CM6A-LR does the rise in small phytoplankton NPP fully compensate for the decline in diatom NPP, explaining the overall positive trend in total NPP.
The increased NPP of small phytoplankton in ACCESS-ESM1-5, where there is no NH4 pool, was attributed to the absence of nitrate limitation (minimum annual concentration of 1.5 mmol m−3 over 2080–2100 despite a consistent decrease throughout the 21st century). The elevation in SST and the reduction in light limitation due to the shallowing of MLD can consequently enhance NPP. Conversely, in UKESM1-0-LL and CanESM5, nitrates exert strong limitations (minimum annual concentration of 0.04 and 0.003 mmol m−3, respectively, over 2080–2100), and their depletion leads to a reduction in NPP.
Diatom NPP consistently decreases across all models except CanESM5-CanOE. In all models but CanESM5-CanOE, projections of diatom NPP are strongly and significantly correlated with maximum annual surface NO3 concentrations (r > 0.96, p≪ 0.01; Fig. 4b). Therefore, as in the subtropical region, the inter-model spread in diatom NPP projections is primarily driven by differences in the sensitivity of diatoms to declining NO3 concentrations. In contrast, the increase in diatom NPP in CanESM5-CanOE, along with its lack of correlation with surface NO3 concentrations, is likely influenced by NH4. Unlike IPSL-CM6A-LR, CESM2, and CESM2-WACCM, CanESM5-CanOE uses identical ammonium uptake half-saturation constants for both small phytoplankton and diatoms (Christian et al., 2022). As a result, in this model, diatoms can utilize ammonium as efficiently as small phytoplankton, leading to similar behavior between the two groups. This likely explains why CanESM5-CanOE is the only model where the long-term evolution of diatoms is not controlled by macro-nutrient concentrations.
The strong increase in small phytoplankton's NPP observed in some CMIP6 models is therefore made possible by the presence of an ammonium pool, which is an alternative source of nitrogen and sustains NPP. NH4 is only present in small concentrations but is quickly regenerated and is therefore preferentially used by small phytoplankton rather than diatoms, except in CanESM5-CanOE. This explains why diatoms cannot benefit from it and why they decrease with macro-nutrient concentrations.
3.3.3 Polar region
Primary production increases in all models in the polar region, ranging from +6.2 to +25.5 in 2080–2100 with respect to 1950–1970 (Fig. 7). These increases are mainly caused by sea ice retreat (on average, 36.4 % of the initial polar regions are still covered in sea ice in the winters of 2080–2100 against 95.5 % in 1950–1970), which leaves space for phytoplankton's growth and thus leads to an increase in NPP. Surface concentrations of NO3 and PO4 decrease across all models (Fig. 7g and h), driven by both changes in ocean physics and increased consumption by phytoplankton. The proportion of NPP relying on NH4 rather than NO3 increases in IPSL-CM6A-LR, CESM2, and CESM2-WACCM (Fig. 5c), thus leading to a decrease in surface NH4 concentrations in the three models.
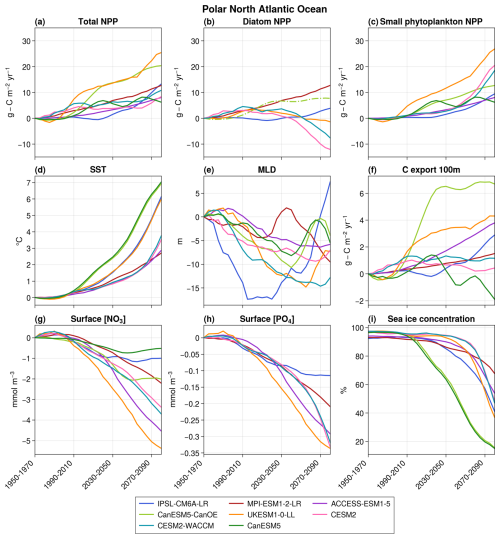
Figure 7Projections over the polar bioregion of total NPP (a), diatom NPP (b), small phytoplankton NPP (c), SST (d), MLD (e), carbon export at 100 m (f), surface nitrate concentration (g), surface phosphate concentration (h), and sea ice concentration (i). The average value over 1950–1970 is taken as the reference for all variables except sea ice concentration. In panel (b), CanESM5-CanOE is represented by a dashed–dotted line to distinguish it from the other models. This distinction is made because it is the only model that includes a diatom group without SiO2 and in which both diatoms and small phytoplankton are equally efficient at utilizing NH4.
This increase in total NPP is observed in all models for small phytoplankton but not for diatoms, for which three models (CESM2, CESM2-WACCM, and UKESM1-0-LL) project a decrease in their primary production after an initial increase (Fig. 7). These three models are the most sensitive to a decrease in NO3 concentrations, as they have the highest NO3 half-saturation constants for diatoms of the six models (2.5 mmol m−3 for CESM2 and CESM2-WACCM, Moore et al., 2001; 0.75 mmol m−3 for UKESM1-0-LL, Yool et al., 2013). Moreover, they all reach very low annual minimum NO3 concentrations (0.02, 0.02, and 0.19 mmol m−3, respectively, in 2080–2100 (not shown)), indicating a termination of the bloom by nitrates. Therefore, in these three models, after a first period in which the retreat of sea ice leads to an increase in primary production through the reduction in light limitation, nitrates become the main controlling factor of primary production, which leads to its decrease. In IPSL-CM6A-LR, nitrate concentrations are even lower than in the previous models (annual minimum of 0.01 mmol m−3 in 2080–2100), but diatoms are less sensitive to low nitrate concentrations (half-saturation constant of 0.39 mmol m−3, Aumont et al., 2015), and their primary production can keep increasing under the reduction in light limitation. In MPI-ESM1-2-LR and CanESM5-CanOE, nitrate concentrations are high throughout the 21st century (annual minimum concentration of 1.18 and 0.47 mmol m−3, respectively, in 2080–2100 despite a consistent decrease throughout the century) and never become limiting. Diatom primary production can therefore freely increase with sea ice retreat.
To summarize, climate change leads to an increase in small phytoplankton NPP in all CMIP6 models because of sea ice retreat. The fate of diatoms is the result of a compromise between their sensitivities to light and nitrates and the evolutions of NO3 concentrations and sea ice, as was already the case in CMIP5 (Vancoppenolle et al., 2013). However, only a small portion of the Arctic was included in this study, and the results found cannot be expanded to the total Arctic Ocean.
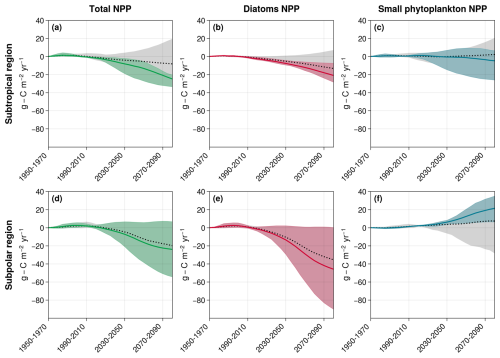
Figure 8New estimates of total NPP (a, d), diatom NPP (b, e), and small phytoplankton's NPP (c, f) projections with the selected CMIP6 models in the subtropical (a–c) and subpolar (d–f) regions. The gray-shaded areas and the dotted black lines correspond to the projection inter-model spreads with all models and the associated multi-model means, respectively. The colored shaded areas and the plain lines correspond to the new inter-model spreads and multi-model means with the selected models. In the subtropical region, the selected models include MPI-ESM1-2-LR, CESM2, CESM2-WACCM, and UKESM1-0-LL. For the subpolar region, the selected models are IPSL-CM6A-LR, CanESM5-CanOE, CESM2, and CESM2-WACCM.
3.4 Toward more robust NPP projections
The model representation of small phytoplankton emerged as the main cause of divergence in NPP projections between models in the subtropical and subpolar North Atlantic Ocean. In the subtropical basin, differing representations of diazotrophy explain model disparities. When not adequately regulated by nutrients like PO4, it has the potential to significantly boost small phytoplankton's NPP, while other nutrient concentrations decline. Only the three models explicitly representing diazotrophs represent these controls and project a decrease in NPP. The three models with an implicit representation of diazotrophs and no effective control from PO4 are therefore not reliable for studying the evolution of small phytoplankton's NPP in the region. Among the models without diazotrophy, ACCESS-ESM1-5 emerged as overly rich in nutrients on average across the subtropical region, making it unreliable for projecting average NPP evolution. Thus, only four out of the eight models appeared dependable for projecting small phytoplankton's NPP evolution in the region (MPI-ESM1-2-LR, CESM2, CESM2-WACCM, and UKESM1-0-LL). They project an average decrease of −4.8 (from −26.0 to +6.4 ) against +2.2 (from −26.0 to +20.4 ) with all models (Fig. 8c). This selection also leads to a strengthening of diatom projected NPP decrease (Fig. 8b), thus resulting in a total NPP decrease of −24.9 (−33.4 to −20.3) against −8.2 (−33.4 to +19.5) (Fig. 8a; Table 2).
Table 2Comparison of the mean projections of total NPP, carbon export at 100 m, and phytoplankton and zooplankton biomasses between 1950–1970 and 2080–2100 in the subtropical and subpolar regions for all models and for the subsets determined in each region (see Fig. 8). The values within the brackets correspond to inter-model spread.
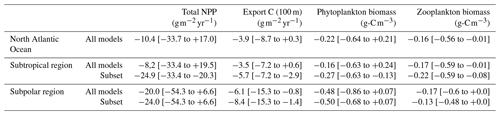
In the subpolar region, NH4 appeared to be able to sustain small phytoplankton's NPP in the models where it is present (IPSL-CM6A-LR, CanESM5-CanOE, CESM2, and CESM2-WACCM). If we hypothesize that ammonium will indeed play such a role in reality, models without an ammonium pool then tend to overestimate the impact of decreasing NO3 concentrations on small phytoplankton. We can therefore set them aside and obtain narrowed estimates of small phytoplankton's NPP projections: +21.4 (from +6.4 to +35.0 ) instead of 7.3 (from −28.2 to +35.0 ) with all models (Fig. 8f). However, such a hypothesis would have no impact on total NPP because it would not affect diatoms (Fig. 8e): −24.0 (from −54.3 to +6.6 ) instead of −20.0 (from −54.3 to +6.6 ) with all models (Fig. 8d and Table 2).
The selection of models based on the processes governing small phytoplankton NPP thus leads to narrowed projections for diatoms and total NPP in the subtropical region but not in the subpolar one. In the polar region, no discriminating mechanism was identified, and it was not possible to narrow projections using the same method.
3.5 Impact on carbon export and plankton biomass
NPP is closely linked with carbon export and plankton biomass, which are crucial variables for comprehending the future trajectory of the biological carbon pump and the effects of climate change on marine ecosystems. Consequently, the process-based selection of NPP models made previously can be used to estimate new projections of these other two variables.
Projections for carbon export across the global North Atlantic Ocean reveal significant disparities, ranging from −8.7 to +0.3 , with an average decrease of −3.9 . However, there is less uncertainty regarding the direction of its future changes compared to NPP projections (Table 2). While some models anticipate a slight increase in the subtropical region, all models project a decline in carbon export in the subpolar region. The model selection allows for a reduction in projection uncertainties in the subtropical region, resulting in a larger average decrease (−5.7 on average compared to −3.5 with all models). In the subpolar region, it does not reduce projection uncertainties but strengthens the average decrease (Table 2). The slight global increase in carbon export projected by some models is therefore explained by the strong increase in NPP they project in the subtropical region and is therefore unlikely to happen. The NPP process-based selection of models thus strengthens carbon export decrease at the end of the 21st century in the North Atlantic Ocean.
Phytoplankton biomass projections also exhibit a strong discrepancy, similar to that of NPP for the global North Atlantic Ocean and for the subtropical region (Table 2). However, the future evolution of phytoplankton biomass in the subpolar region is less uncertain compared to NPP, despite considerable model divergence (Table 2). The selection of models leads to a significant reduction in projection uncertainties in the subtropical region, where a new inter-model agreement on a future decrease in phytoplankton biomass emerges. The strong increases projected by some models are therefore caused by their inadequate representation of diazotrophy in the subtropical region, making such projections improbable.
All models agree on a decrease in zooplankton biomass across the global North Atlantic Ocean and in the two individual regions (Table 2). Both selections only slightly narrow projections in the two regions. This limited impact is due to the varying representations of zooplankton in CMIP6 models (Rohr et al., 2023), leading to a relative inter-model decoupling of phytoplankton and zooplankton. An analysis similar to the one conducted here should therefore be done to fully understand zooplankton projections in CMIP6 models.
4.1 Model democracy and emergent constraints
NPP projections in the North Atlantic Ocean emerged as a case where the traditional approach of model inter-comparison studies through “model democracy” may not be adapted. This approach assumes that all ESMs are equally relevant and reliable for projecting future climate evolution (Knutti, 2010), whereas we showed here that some models, due to their representation or lack thereof of certain processes, are not dependable for projecting NPP in the region. A democratic multi-model mean computed with equal weights attributed to all models, as is done in Kwiatkowski et al. (2020) and Tagliabue et al. (2021), would therefore not be the optimal estimate of the future evolution of NPP.
However, we did not entirely set aside the model democracy approach because it was applied to model subsets in both regions. This application is questionable because it assumes independence among selected models, which is often not the case (Eyring et al., 2019). Some CMIP6 models are essentially variations of a similar base model (e.g., CESM2 and CESM2-WACCM) and share components and parameterizations (Masson and Knutti, 2011; Bishop and Abramowitz, 2013; Alexander and Easterbrook, 2015). This lack of independence results in systematic biases within the multi-model mean when model democracy is adopted. To address this issue, various studies have attempted to assign different weights to models based on their independence and ability to reproduce historical observations (Sanderson et al., 2015, 2017). While such efforts have sometimes improved agreement between multi-model means and observations (Räisänen et al., 2010; Knutti et al., 2017), the question of the validity of weighting methods for future projections remains unresolved. For example, in the context of NPP projections in the subtropical North Atlantic Ocean, the divergence of diazotrophy between models with effective PO4 control and those without only arises around the year 2020 (Fig. 3). Consequently, weighting based solely on observations would likely overlook this divergence. Thus, process-based model selections, such as the one conducted here, and weighting methods are complementary techniques that should ideally be used together to combine their respective advantages.
Moreover, our approach failed at improving total NPP projections in the subpolar North Atlantic Ocean because we were not able to constrain diatoms. The discrepancy of diatom NPP projections arises from differences in sensitivity to a similar mechanism (Fig. 4), which prevents a process-based approach. However, it might set a good framework for an emergent constraint approach, which consists of exploiting an inter-model correlation between an observable variable and the response of another variable to climate change to constrain projections (Eyring et al., 2019; Sanderson et al., 2021). Emergent constrains have successfully been used to constrain projections of climate sensitivity (Cox et al., 2018), sea ice extent (Qu and Hall, 2014), extreme-precipitation events (O'Gorman, 2012), and NPP in the tropical ocean (Kwiatkowski et al., 2017). But, the reliability of emergent constraint outcomes is often hindered by the presence of systematic biases in large ensembles, stemming from model interdependence (Sanderson et al., 2021). Nevertheless, it is sometimes possible to enhance this reliability with the identification of a robust mechanism explaining the correlation used. Emergent constraints and process-based approaches are therefore complementary, particularly when competing mechanisms coexist. The case of NPP projections in the subpolar North Atlantic Ocean thus seems to be an ideal situation for combining a process-based method applied to small phytoplankton, carried out in this study, with an emergent constraint approach applied to the differing sensitivities of diatom NPP to nitrate concentrations. This will be the objective of a future study.
4.2 The influence of oceanic physics on NPP projection divergence
Divergences in NPP projections might in principle result from differences in both biogeochemical model components and the representation of physical processes, which may either amplify or mitigate the effects of biogeochemistry. In this study, we focused on the former, largely setting aside the latter. Our focus was motivated by the observation that, unlike NPP, the evolution with climate change of key oceanic parameters known to influence NPP, such as temperature, MLD, nutrient concentrations, and sea ice cover, had the same sign across all models. Specifically, all models indicated an increase in temperature, a decrease in nutrient concentrations, a reduction in MLD, and a decline in sea ice cover, while some projected an increase in NPP and others a decrease. However, we should point out that agreement on the sign of the evolution of these variables does not necessarily rule out their contribution to diverging NPP projections. The response of NPP to changes in MLD, for instance, is not always monotonic (Llort et al., 2019), and non-monotonicity may also result from complex interactions between physical and biogeochemical processes on seasonal timescales (Mousing et al., 2023). Future efforts are therefore needed to fully assess how differing physical mechanisms control NPP, further refining projection reliability and reducing uncertainty.
4.3 Estimation of climate change impacts on high trophic levels
CMIP model output serves as data inputs for the Inter-Sectoral Impact Model Intercomparison Project (ISIMIP), an exercise aimed at examining the effects of climate change on human societies and ecosystems (Frieler et al., 2024). In the context of studying fisheries and marine ecosystems, CMIP models are used as inputs for marine ecosystem models. The connecting variable between plankton and higher trophic levels could be either NPP or the biomass of phytoplankton and zooplankton, depending on the marine ecosystem model employed (Tittensor et al., 2021). Additionally, some models rely on total NPP or biomass (Carozza et al., 2016), while others require inputs for each phytoplankton and zooplankton group, providing information on species composition (Cheung et al., 2016). Only a limited selection of CMIP models is incorporated into these studies. For the third phase of ISIMIP, specifically, only four CMIP6 models were chosen for marine ecosystem studies: GFDL-ESM4, UKESM1-0-LL, MPI-ESM1-2-HR, and IPSL-CM6A-LR. The biases exhibited by these models in projecting NPP and plankton biomass consequently influence the marine ecosystem models that employ them to investigate climate change impacts.
We demonstrated that IPSL-CM6A-LR lacks reliability in projecting NPP in the subtropical region due to its failure to represent PO4 control over N2 fixation. Conversely, UKESM1-0-LL lacks an NH4 pool, likely resulting in an overestimation of NPP decrease in the subpolar region. These model deficiencies in projecting NPP directly affect phytoplankton biomass, and similar issues may arise for zooplankton biomass due to their disparate representations across CMIP6 models (Rohr et al., 2023). Such biases could significantly impact ISIMIP outcomes, which are subsequently used by policymakers to develop adaptation measures to climate change. Hence, it is imperative to identify these biases, assess the reliability of CMIP models in projecting variables used in ISIMIP studies, and estimate the repercussions of CMIP biases for ISIMIP results.
4.4 Perspectives on the future evolution of the biological carbon pump
Carbon export caused by the sinking of particulate organic matter is a key feature of the biological carbon pump, which helps to store carbon in the deep ocean and reduces atmospheric CO2 concentrations. Depending on the remineralization depth of organic matter and the residency time of inorganic carbon in the deep ocean (Wilson et al., 2022), a decrease in carbon export caused by climate change might reduce the amount of carbon stored in the deep ocean and increase atmospheric CO2 concentrations, thus forming a positive feedback mechanism. However, the sequestration time of the exported organic carbon strongly varies spatially (Nowicki et al., 2022). In the subtropical North Atlantic Ocean, it is only stored for a few decades, whereas it remains sequestered for several hundreds of years in the subpolar North Atlantic Ocean.
The strengthened decrease in carbon export obtained in both regions in this study therefore means that the North Atlantic biological carbon pump might be more affected by climate change than previously anticipated and on particularly long timescales for the subpolar region. However, because we were not able to reduce diatom NPP projection uncertainties in the region, the spread in carbon export projections remains very large.
Nevertheless, there is no quantitative relationship between carbon export and the efficiency of the biological carbon pump (DeVries et al., 2012). A reduction in the former does not necessarily mean a decrease in the latter. Further work is therefore needed to understand how the decrease in carbon export in the North Atlantic Ocean would affect the biological carbon pump, in both the short and the long term.
NPP is a key biogeochemical variable for which large uncertainties exist regarding its future evolution under climate change, particularly in the North Atlantic Ocean. The traditional approach to this problem, based on a multi-model mean computed through the model democracy approach, fails to provide informative projections for NPP in the region. We therefore developed a new methodology to exploit CMIP6 model diversity in order to gain new insights into the way NPP will be affected by climate change in the region. Thanks to an innovative regionalization of the North Atlantic Ocean, we were able to identify the processes responsible for the divergence of model projections and assess their likelihood of occurrence. This analysis allowed us to proceed to an informed selection of models in each bioregion, which gave us new estimates of the future evolution of NPP, along with carbon export and plankton biomasses, in the subtropical and subpolar bioregions.
CMIP6 model diversity was essential for conducting such an approach because it allowed us to identify the mechanisms responsible for projection divergence. The models set aside in the different bioregions should therefore not be discarded from the CMIP6 ensemble. Nevertheless, it is necessary to recognize that all models are not equally relevant depending on the region, the variable considered, and the question asked. A direct model democracy approach is therefore limited and should only be regarded as a first step in an inter-comparison study. It should be completed by more advanced methods, such as informed model selection, observation-based weighting, or emergent constraints, and by their combination. Moreover, when CMIP6 model outputs are used to assess the impacts climate change will have on ecosystems or human societies, the reliability of the models used to conduct these studies should be assessed in order to avoid strong biases stemming from CMIP6 models affecting the results.
Publicly available datasets were analyzed in this study. This data can be found at https://esgf.llnl.gov/ (Earth System Grid Federation, 2024). The SOM + HAC algorithm used to build the bioregions can be found at https://github.com/ilarinieminen/SOM-Toolbox (ilarinieminen, 2023). This study has been conducted using EU Copernicus Marine Service information: https://doi.org/10.48670/moi-00281 (global ocean colour; E.U. Copernicus Marine Service Information, 2023a) and https://doi.org/10.48670/moi-00052 (Multi Observation Global Ocean 3D Temperature Salinity Height Geostrophic Current and MLD; E.U. Copernicus Marine Service Information, 2023b; Guinehut et al., 2012; Mulet et al., 2012). The sea ice concentration data used in this study was downloaded from the Copernicus Climate Change Service website (https://doi.org/10.24381/cds.3cd8b812, Copernicus Climate Change Service, 2010).
ML, LB, and SD conceived the study. SD did the analysis and wrote the paper. REH helped with the construction of the bioregions.
The contact author has declared that none of the authors has any competing interests.
Publisher's note: Copernicus Publications remains neutral with regard to jurisdictional claims made in the text, published maps, institutional affiliations, or any other geographical representation in this paper. While Copernicus Publications makes every effort to include appropriate place names, the final responsibility lies with the authors.
We are grateful to the World Climate Research Programme's Working Group on Coupled Modelling, which is responsible for the CMIP exercises. For CMIP, the US Department of Energy’s Program for Climate Model Diagnosis and Intercomparison provided coordinating support and led the development of software infrastructure in partnership with the Global Organization for Earth System Science Portals. This study benefitted from the ESPRI (Ensemble de Services Pour la Recherche à l’IPSL) computing and data centre (https://mesocentre.ipsl.fr/, last access: 13 February 2025), which is supported by CNRS, Sorbonne Université, École Polytechnique, and CNES and through national and international grants. We thank the two anonymous reviewers whose comments have helped improve our manuscript. We also thank Olivier Torres, who helped with the data management, and Lester Kwiatkowski, whose comments and insights helped improve our study.
This paper was edited by Ciavatta Stefano and reviewed by two anonymous referees.
Abot, L., Provost, C., and Poli, L.: Recent Convection Decline in the Greenland Sea: Insights From the Mercator Ocean System Over 2008–2020, J. Geophys. Res.-Oceans, 128, e2022JC019320, https://doi.org/10.1029/2022JC019320, 2023. a
Alexander, K. and Easterbrook, S. M.: The software architecture of climate models: a graphical comparison of CMIP5 and EMICAR5 configurations, Geosci. Model Dev., 8, 1221–1232, https://doi.org/10.5194/gmd-8-1221-2015, 2015. a
Aumont, O., Ethé, C., Tagliabue, A., Bopp, L., and Gehlen, M.: PISCES-v2: an ocean biogeochemical model for carbon and ecosystem studies, Geosci. Model Dev., 8, 2465–2513, https://doi.org/10.5194/gmd-8-2465-2015, 2015. a, b, c, d, e
Baaklini, G., El Hourany, R., Fakhri, M., Brajard, J., Issa, L., Fifani, G., and Mortier, L.: Surface circulation properties in the eastern Mediterranean emphasized using machine learning methods, Ocean Sci., 18, 1491–1505, https://doi.org/10.5194/os-18-1491-2022, 2022. a
Behrenfeld, M. J. and Falkowski, P. G.: Photosynthetic rates derived from satellite-based chlorophyll concentration, Limnol. Oceanogr., 42, 1–20, https://doi.org/10.4319/lo.1997.42.1.0001, 1997. a
Benedetti, F., Vogt, M., Elizondo, U. H., Righetti, D., Zimmermann, N. E., and Gruber, N.: Major restructuring of marine plankton assemblages under global warming, Nat. Commun., 12, 5226, https://doi.org/10.1038/s41467-021-25385-x, 2021. a
Bishop, C. H. and Abramowitz, G.: Climate model dependence and the replicate Earth paradigm, Clim. Dynam., 41, 885–900, https://doi.org/10.1007/s00382-012-1610-y, 2013. a
Bopp, L., Resplandy, L., Orr, J. C., Doney, S. C., Dunne, J. P., Gehlen, M., Halloran, P., Heinze, C., Ilyina, T., Séférian, R., Tjiputra, J., and Vichi, M.: Multiple stressors of ocean ecosystems in the 21st century: projections with CMIP5 models, Biogeosciences, 10, 6225–6245, https://doi.org/10.5194/bg-10-6225-2013, 2013. a, b
Bopp, L., Aumont, O., Kwiatkowski, L., Clerc, C., Dupont, L., Ethé, C., Gorgues, T., Séférian, R., and Tagliabue, A.: Diazotrophy as a key driver of the response of marine net primary productivity to climate change, Biogeosciences, 19, 4267–4285, https://doi.org/10.5194/bg-19-4267-2022, 2022. a, b, c
Boucher, O., Servonnat, J., Albright, A. L., Aumont, O., Balkanski, Y., Bastrikov, V., Bekki, S., Bonnet, R., Bony, S., Bopp, L., Braconnot, P., Brockmann, P., Cadule, P., Caubel, A., Cheruy, F., Codron, F., Cozic, A., Cugnet, D., D'Andrea, F., Davini, P., de Lavergne, C., Denvil, S., Deshayes, J., Devilliers, M., Ducharne, A., Dufresne, J.-L., Dupont, E., Éthé, C., Fairhead, L., Falletti, L., Flavoni, S., Foujols, M.-A., Gardoll, S., Gastineau, G., Ghattas, J., Grandpeix, J.-Y., Guenet, B., Guez, Lionel, E., Guilyardi, E., Guimberteau, M., Hauglustaine, D., Hourdin, F., Idelkadi, A., Joussaume, S., Kageyama, M., Khodri, M., Krinner, G., Lebas, N., Levavasseur, G., Lévy, C., Li, L., Lott, F., Lurton, T., Luyssaert, S., Madec, G., Madeleine, J.-B., Maignan, F., Marchand, M., Marti, O., Mellul, L., Meurdesoif, Y., Mignot, J., Musat, I., Ottlé, C., Peylin, P., Planton, Y., Polcher, J., Rio, C., Rochetin, N., Rousset, C., Sepulchre, P., Sima, A., Swingedouw, D., Thiéblemont, R., Traore, A. K., Vancoppenolle, M., Vial, J., Vialard, J., Viovy, N., and Vuichard, N.: Presentation and Evaluation of the IPSL-CM6A-LR Climate Model, J. Adv. Model. Earth Sy., 12, e2019MS002010, https://doi.org/10.1029/2019MS002010, 2020. a
Canadell, J. G., Scheel Monteiro, P., Costa, M. H., Cotrim da Cunha, L., Cox, P. M., Eliseev, A. V., Henson, S., Ishii, M., Jaccard, S., Koven, C., Lohila, A., Patra, P. K., Piao, S., Rogelj, J., Syampungani, S., Zaehle, S., and Zickfeld, K.: Global carbon and other biogeochemical cycles and feedbacks, in: Climate Change 2021: The Physical Science Basis. Contribution of Working Group I to the Sixth Assessment Report of the Intergovernmental Panel on Climate Change, edited by: Masson-Delmotte, V., Zhai, P., Pirani, A., Connors, S. L., Péan, C., Berger, S., Caud, N., Chen, Y., Goldfarb, L., Gomis, M. I., Huang, M., Leitzell, K., Lonnoy, E., Matthews, J. B. R., Maycock, T. K., Waterfield, T., Yelekçi, R., Yu, R., and Zhou, B., Cambridge University Press, Cambridge, United Kingdom and New York, NY, USA, 673–816, https://doi.org/10.1017/9781009157896.001, 2021. a
Carozza, D. A., Bianchi, D., and Galbraith, E. D.: The ecological module of BOATS-1.0: a bioenergetically constrained model of marine upper trophic levels suitable for studies of fisheries and ocean biogeochemistry, Geosci. Model Dev., 9, 1545–1565, https://doi.org/10.5194/gmd-9-1545-2016, 2016. a
Cheung, W. W. L., Frölicher, T. L., Asch, R. G., Jones, M. C., Pinsky, M. L., Reygondeau, G., Rodgers, K. B., Rykaczewski, R. R., Sarmiento, J. L., Stock, C., and Watson, J. R.: Building confidence in projections of the responses of living marine resources to climate change, ICES J. Mar. Sci., 73, 1283–1296, https://doi.org/10.1093/icesjms/fsv250, 2016. a
Christian, J. R., Denman, K. L., Hayashida, H., Holdsworth, A. M., Lee, W. G., Riche, O. G. J., Shao, A. E., Steiner, N., and Swart, N. C.: Ocean biogeochemistry in the Canadian Earth System Model version 5.0.3: CanESM5 and CanESM5-CanOE, Geosci. Model Dev., 15, 4393–4424, https://doi.org/10.5194/gmd-15-4393-2022, 2022. a, b, c, d, e
Copernicus Climate Change Service (C3S): Sea ice concentration daily gridded data from 1978 to present derived from satellite observations, Copernicus Climate Change Service (C3S) Climate Data Store (CDS) [data set], https://doi.org/10.24381/cds.3cd8b812, 2020. a
Cox, P. M., Huntingford, C., and Williamson, M. S.: Emergent constraint on equilibrium climate sensitivity from global temperature variability, Nature, 553, 319–322, https://doi.org/10.1038/nature25450, 2018. a
Derouiche, S., Mallet, C., Hannachi, A., and Bargaoui, Z.: Characterisation of rainfall events in northern Tunisia using self-organising maps, J. Hydrol., 42, 101159, https://doi.org/10.1016/j.ejrh.2022.101159, 2022. a, b
DeVries, T., Primeau, F., and Deutsch, C.: The sequestration efficiency of the biological pump, Geophys. Res. Lett., 39, L13601, https://doi.org/10.1029/2012GL051963, 2012. a
Dilmi, M. D., Mallet, C., Barthes, L., and Chazottes, A.: Data-driven clustering of rain events: microphysics information derived from macro-scale observations, Atmos. Meas. Tech., 10, 1557–1574, https://doi.org/10.5194/amt-10-1557-2017, 2017. a, b
Earth System Grid Federation (ESGF): https://esgf.llnl.gov/, last access: January 2024. a
El Hourany, R., Mejia, C., Faour, G., Crépon, M., and Thiria, S.: Evidencing the Impact of Climate Change on the Phytoplankton Community of the Mediterranean Sea Through a Bioregionalization Approach, J. Geophys. Res.-Oceans, 126, e2020JC016808, https://doi.org/10.1029/2020JC016808, 2021. a, b
Eppley, R. W.: Temperature and phytoplankton growth in the sea, Fishery Bulletin, 70, 1972. a
E.U. Copernicus Marine Service Information (CMEMS): Global Ocean Colour (Copernicus-GlobColour), Bio-Geo-Chemical, L4 (monthly and interpolated) from Satellite Observations (1997–ongoing), Marine Data Store (MDS) [data set], https://doi.org/10.48670/moi-00281, 2023a. a
E.U. Copernicus Marine Service Information (CMEMS): Multi Observation Global Ocean 3D Temperature Salinity Height Geostrophic Current and MLD, Marine Data Store (MDS) [data set], https://doi.org/10.48670/moi-00052, 2023b. a
Eyring, V., Bony, S., Meehl, G. A., Senior, C. A., Stevens, B., Stouffer, R. J., and Taylor, K. E.: Overview of the Coupled Model Intercomparison Project Phase 6 (CMIP6) experimental design and organization, Geosci. Model Dev., 9, 1937–1958, https://doi.org/10.5194/gmd-9-1937-2016, 2016. a
Eyring, V., Cox, P. M., Flato, G. M., Gleckler, P. J., Abramowitz, G., Caldwell, P., Collins, W. D., Gier, B. K., Hall, A. D., Hoffman, F. M., Hurtt, G. C., Jahn, A., Jones, C. D., Klein, S. A., Krasting, J. P., Kwiatkowski, L., Lorenz, R., Maloney, E., Meehl, G. A., Pendergrass, A. G., Pincus, R., Ruane, A. C., Russell, J. L., Sanderson, B. M., Santer, B. D., Sherwood, S. C., Simpson, I. R., Stouffer, R. J., and Williamson, M. S.: Taking climate model evaluation to the next level, Nat. Clim. Change, 9, 102–110, https://doi.org/10.1038/s41558-018-0355-y, 2019. a, b, c
Farikou, O., Sawadogo, S., Niang, A., Diouf, D., Brajard, J., Mejia, C., Dandonneau, Y., Gasc, G., Crepon, M., and Thiria, S.: Inferring the seasonal evolution of phytoplankton groups in the Senegalo-Mauritanian upwelling region from satellite ocean-color spectral measurements, J. Geophys. Res.-Oceans, 120, 6581–6601, https://doi.org/10.1002/2015JC010738, 2015. a
Fox-Kemper, B., Hewitt, H. T., Xiao, C., Aðalgeirsdóttir, G., Drijfhout, S. S., Edwards, T. L., Golledge, N. R., Hemer, M., Kopp, R. E., Krinner, G., Mix, A., Notz, D., Nowicki, S., Nurhati, I. S., Ruiz, L., Sallée, J.-B., Slangen, A. B. A., and Yu, Y.: Ocean, cryosphere, and sea level change, in: Climate Change 2021: The Physical Science Basis. Contribution of Working Group I to the Sixth Assessment Report of the Intergovernmental Panel on Climate Change, edited by: Masson-Delmotte, V., Zhai, P., Pirani, A., Connors, S. L., Péan, C., Berger, S., Caud, N., Chen, Y., Goldfarb, L., Gomis, M. I., Huang, M., Leitzell, K., Lonnoy, E., Matthews, J. B. R., Maycock, T. K., Waterfield, T., Yelekçi, R., Yu, R., and Zhou, B., Cambridge University Press, Cambridge, United Kingdom and New York, NY, USA, 1211–1362, https://doi.org/10.1017/9781009157896.001, 2021. a
Frieler, K., Volkholz, J., Lange, S., Schewe, J., Mengel, M., del Rocío Rivas López, M., Otto, C., Reyer, C. P. O., Karger, D. N., Malle, J. T., Treu, S., Menz, C., Blanchard, J. L., Harrison, C. S., Petrik, C. M., Eddy, T. D., Ortega-Cisneros, K., Novaglio, C., Rousseau, Y., Watson, R. A., Stock, C., Liu, X., Heneghan, R., Tittensor, D., Maury, O., Büchner, M., Vogt, T., Wang, T., Sun, F., Sauer, I. J., Koch, J., Vanderkelen, I., Jägermeyr, J., Müller, C., Rabin, S., Klar, J., Vega del Valle, I. D., Lasslop, G., Chadburn, S., Burke, E., Gallego-Sala, A., Smith, N., Chang, J., Hantson, S., Burton, C., Gädeke, A., Li, F., Gosling, S. N., Müller Schmied, H., Hattermann, F., Wang, J., Yao, F., Hickler, T., Marcé, R., Pierson, D., Thiery, W., Mercado-Bettín, D., Ladwig, R., Ayala-Zamora, A. I., Forrest, M., and Bechtold, M.: Scenario setup and forcing data for impact model evaluation and impact attribution within the third round of the Inter-Sectoral Impact Model Intercomparison Project (ISIMIP3a), Geosci. Model Dev., 17, 1–51, https://doi.org/10.5194/gmd-17-1-2024, 2024. a
Fu, W., Randerson, J. T., and Moore, J. K.: Climate change impacts on net primary production (NPP) and export production (EP) regulated by increasing stratification and phytoplankton community structure in the CMIP5 models, Biogeosciences, 13, 5151–5170, https://doi.org/10.5194/bg-13-5151-2016, 2016. a
Garcia, H. E., Weathers, K. W., Paver, C. R., Smolyar, I., Boyer, T. P., Locarnini, R. A., Zweng, M. M., Mishonov, A. V., Baranova, O. K., Seidov, D., and Reagan, J. R.: World Ocean Atlas 2018, Volume 4: Dissolved Inorganic Nutrients (phosphate, nitrate and nitrate + nitrite, silicate), edited by: Mishonov, A. (Technical Ed.), NOAA Atlas NESDIS, 84, 35 pp., https://www.ncei.noaa.gov/sites/default/files/2020-04/woa18_vol4.pdf (last access: October 2023), 2018. a
Guèye, A. K., Janicot, S., Niang, A., Sawadogo, S., Sultan, B., Diongue-Niang, A., and Thiria, S.: Weather regimes over Senegal during the summer monsoon season using self-organizing maps and hierarchical ascendant classification. Part I: synoptic time scale, Clim. Dynam., 36, 1–18, https://doi.org/10.1007/s00382-010-0782-6, 2011. a
Guinehut, S., Dhomps, A.-L., Larnicol, G., and Le Traon, P.-Y.: High resolution 3-D temperature and salinity fields derived from in situ and satellite observations, Ocean Sci., 8, 845–857, https://doi.org/10.5194/os-8-845-2012, 2012. a, b
Hátún, H., Azetsu-Scott, K., Somavilla, R., Rey, F., Johnson, C., Mathis, M., Mikolajewicz, U., Coupel, P., Tremblay, J.-R., Hartman, S., Pacariz, S. V., Salter, I., and Ólafsson, J.: The subpolar gyre regulates silicate concentrations in the North Atlantic, Sci. Rep., 7, 14576, https://doi.org/10.1038/s41598-017-14837-4, 2017. a
Hilborn, A. and Devred, E.: Delineation of Eastern Beaufort Sea Sub-regions Using Self-Organizing Maps Applied to 17 Years of MODIS-Aqua Data, Frontiers in Marine Science, 9, 912865, https://www.frontiersin.org/articles/10.3389/fmars.2022.912865 (last access: 3 November 2023), 2022. a, b
Hofmann Elizondo, U., Righetti, D., Benedetti, F., and Vogt, M.: Biome partitioning of the global ocean based on phytoplankton biogeography, Prog. Oceanogr., 194, 102530, https://doi.org/10.1016/j.pocean.2021.102530, 2021. a
ilarinieminen: SOM-Toolbox, GitHub [code], https://github.com/ilarinieminen/SOM-Toolbox, last access: October 2023. a
Ilyina, T., Six, K. D., Segschneider, J., Maier-Reimer, E., Li, H., and Núñez-Riboni, I.: Global ocean biogeochemistry model HAMOCC: Model architecture and performance as component of the MPI-Earth system model in different CMIP5 experimental realizations, J. Adv. Model. Earth Sy., 5, 287–315, https://doi.org/10.1029/2012MS000178, 2013. a
Jouini, M., Béranger, K., Arsouze, T., Beuvier, J., Thiria, S., Crépon, M., and Taupier-Letage, I.: The Sicily Channel surface circulation revisited using a neural clustering analysis of a high-resolution simulation, J. Geophys. Res.-Oceans, 121, 4545–4567, https://doi.org/10.1002/2015JC011472, 2016. a, b
Kearney, K. A., Bograd, S. J., Drenkard, E., Gomez, F. A., Haltuch, M., Hermann, A. J., Jacox, M. G., Kaplan, I. C., Koenigstein, S., Luo, J. Y., Masi, M., Muhling, B., Pozo Buil, M., and Woodworth-Jefcoats, P. A.: Using Global-Scale Earth System Models for Regional Fisheries Applications, Front. Mar. Sci., 8, 622206, https://doi.org/10.3389/fmars.2021.622206, 2021. a, b
Kléparski, L., Beaugrand, G., Edwards, M., and Ostle, C.: Phytoplankton life strategies, phenological shifts and climate change in the North Atlantic Ocean from 1850 to 2100, Glob. Change Biol., 29, 3833–3849, https://doi.org/10.1111/gcb.16709, 2023. a
Knutti, R.: The end of model democracy?, Climatic Change, 102, 395–404, https://doi.org/10.1007/s10584-010-9800-2, 2010. a
Knutti, R., Sedláček, J., Sanderson, B. M., Lorenz, R., Fischer, E. M., and Eyring, V.: A climate model projection weighting scheme accounting for performance and interdependence, Geophys. Res. Lett., 44, 1909–1918, https://doi.org/10.1002/2016GL072012, 2017. a, b
Kohonen, T.: Essentials of the self-organizing map, Neural Networks, 37, 52–65, https://doi.org/10.1016/j.neunet.2012.09.018, 2013. a
Kwiatkowski, L., Bopp, L., Aumont, O., Ciais, P., Cox, P. M., Laufkötter, C., Li, Y., and Séférian, R.: Emergent constraints on projections of declining primary production in the tropical oceans, Nat. Clim. Change, 7, 355–358, https://doi.org/10.1038/nclimate3265, 2017. a, b
Kwiatkowski, L., Torres, O., Bopp, L., Aumont, O., Chamberlain, M., Christian, J. R., Dunne, J. P., Gehlen, M., Ilyina, T., John, J. G., Lenton, A., Li, H., Lovenduski, N. S., Orr, J. C., Palmieri, J., Santana-Falcón, Y., Schwinger, J., Séférian, R., Stock, C. A., Tagliabue, A., Takano, Y., Tjiputra, J., Toyama, K., Tsujino, H., Watanabe, M., Yamamoto, A., Yool, A., and Ziehn, T.: Twenty-first century ocean warming, acidification, deoxygenation, and upper-ocean nutrient and primary production decline from CMIP6 model projections, Biogeosciences, 17, 3439–3470, https://doi.org/10.5194/bg-17-3439-2020, 2020. a, b, c, d
Laufkötter, C., Vogt, M., Gruber, N., Aita-Noguchi, M., Aumont, O., Bopp, L., Buitenhuis, E., Doney, S. C., Dunne, J., Hashioka, T., Hauck, J., Hirata, T., John, J., Le Quéré, C., Lima, I. D., Nakano, H., Seferian, R., Totterdell, I., Vichi, M., and Völker, C.: Drivers and uncertainties of future global marine primary production in marine ecosystem models, Biogeosciences, 12, 6955–6984, https://doi.org/10.5194/bg-12-6955-2015, 2015. a
Li, G., Cheng, L., Zhu, J., Trenberth, K. E., Mann, M. E., and Abraham, J. P.: Increasing ocean stratification over the past half-century, Nat. Clim. Change, 10, 1116–1123, https://doi.org/10.1038/s41558-020-00918-2, 2020. a
Llort, J., Lévy, M., Sallée, J. B., and Tagliabue, A.: Nonmonotonic Response of Primary Production and Export to Changes in Mixed-Layer Depth in the Southern Ocean, Geophys. Res. Lett., 46, 3368–3377, https://doi.org/10.1029/2018GL081788, 2019. a
Longhurst, A. R.: Chapter 1 – Toward an ecological geography of the sea, in: Ecological Geography of the Sea (Second Edition), edited by: Longhurst, A. R., Academic Press, Burlington, 1–17, https://doi.org/10.1016/B978-012455521-1/50002-4, 2007. a
Masson, D. and Knutti, R.: Climate model genealogy: CLIMATE MODEL GENEALOGY, Geophys. Res. Lett., 38, L08703, https://doi.org/10.1029/2011GL046864, 2011. a
Mauritsen, T., Bader, J., Becker, T., Behrens, J., Bittner, M., Brokopf, R., Brovkin, V., Claussen, M., Crueger, T., Esch, M., Fast, I., Fiedler, S., Fläschner, D., Gayler, V., Giorgetta, M., Goll, D. S., Haak, H., Hagemann, S., Hedemann, C., Hohenegger, C., Ilyina, T., Jahns, T., Jimenéz-de-la Cuesta, D., Jungclaus, J., Kleinen, T., Kloster, S., Kracher, D., Kinne, S., Kleberg, D., Lasslop, G., Kornblueh, L., Marotzke, J., Matei, D., Meraner, K., Mikolajewicz, U., Modali, K., Möbis, B., Müller, W. A., Nabel, J. E. M. S., Nam, C. C. W., Notz, D., Nyawira, S.-S., Paulsen, H., Peters, K., Pincus, R., Pohlmann, H., Pongratz, J., Popp, M., Raddatz, T. J., Rast, S., Redler, R., Reick, C. H., Rohrschneider, T., Schemann, V., Schmidt, H., Schnur, R., Schulzweida, U., Six, K. D., Stein, L., Stemmler, I., Stevens, B., von Storch, J.-S., Tian, F., Voigt, A., Vrese, P., Wieners, K.-H., Wilkenskjeld, S., Winkler, A., and Roeckner, E.: Developments in the MPI-M Earth System Model version 1.2 (MPI-ESM1.2) and Its Response to Increasing CO2, J. Adv. Model. Earth Sy., 11, 998–1038, https://doi.org/10.1029/2018MS001400, 2019. a
Moore, J. K., Doney, S. C., Kleypas, J. A., Glover, D. M., and Fung, I. Y.: An intermediate complexity marine ecosystem model for the global domain, Deep-Sea Res. Pt. II, 49, 403–462, https://doi.org/10.1016/S0967-0645(01)00108-4, 2001. a, b, c, d, e, f
Mousing, E. A., Ellingen, I., Hjøllo, S. S., Husson, B., Skogen, M. D., and Wallhead, P.: Why do regional biogeochemical models produce contrasting future projections of primary production in the Barents Sea?, J. Sea Res., 192, 102366, https://doi.org/10.1016/j.seares.2023.102366, 2023. a, b
Mulet, S., Rio, M. H., Mignot, A., Guinehut, S., and Morrow, R.: A new estimate of the global 3D geostrophic ocean circulation based on satellite data and in-situ measurements, Deep-Sea Res. Pt. II, 77–80, 70–81, https://doi.org/10.1016/j.dsr2.2012.04.012, 2012. a, b
Nowicki, M., DeVries, T., and Siegel, D. A.: Quantifying the Carbon Export and Sequestration Pathways of the Ocean's Biological Carbon Pump, Global Biogeochem. Cy., 36, e2021GB007083, https://doi.org/10.1029/2021GB007083, 2022. a, b
Oke, P. R., Griffin, D. A., Schiller, A., Matear, R. J., Fiedler, R., Mansbridge, J., Lenton, A., Cahill, M., Chamberlain, M. A., and Ridgway, K.: Evaluation of a near-global eddy-resolving ocean model, Geosci. Model Dev., 6, 591–615, https://doi.org/10.5194/gmd-6-591-2013, 2013. a
O'Gorman, P. A.: Sensitivity of tropical precipitation extremes to climate change, Nat. Geosci., 5, 697–700, https://doi.org/10.1038/ngeo1568, 2012. a
O'Neill, B. C., Kriegler, E., Riahi, K., Ebi, K. L., Hallegatte, S., Carter, T. R., Mathur, R., and van Vuuren, D. P.: A new scenario framework for climate change research: the concept of shared socioeconomic pathways, Climatic Change, 122, 387–400, https://doi.org/10.1007/s10584-013-0905-2, 2014. a
O'Neill, B. C., Tebaldi, C., van Vuuren, D. P., Eyring, V., Friedlingstein, P., Hurtt, G., Knutti, R., Kriegler, E., Lamarque, J.-F., Lowe, J., Meehl, G. A., Moss, R., Riahi, K., and Sanderson, B. M.: The Scenario Model Intercomparison Project (ScenarioMIP) for CMIP6, Geosci. Model Dev., 9, 3461–3482, https://doi.org/10.5194/gmd-9-3461-2016, 2016. a, b
Palter, J. B. and Lozier, M. S.: On the source of Gulf Stream nutrients, J. Geophys. Res.-Oceans, 113, C06018, https://doi.org/10.1029/2007JC004611, 2008. a
Paulsen, H., Ilyina, T., Six, K. D., and Stemmler, I.: Incorporating a prognostic representation of marine nitrogen fixers into the global ocean biogeochemical model HAMOCC, J. Adv. Model. Earth Sy., 9, 438–464, https://doi.org/10.1002/2016MS000737, 2017. a, b
Qu, X. and Hall, A.: On the persistent spread in snow-albedo feedback, Clim. Dynam., 42, 69–81, https://doi.org/10.1007/s00382-013-1774-0, 2014. a
Räisänen, J., Ruokolainen, L., and Ylhäisi, J.: Weighting of model results for improving best estimates of climate change, Clim. Dynam., 35, 407–422, https://doi.org/10.1007/s00382-009-0659-8, 2010. a
Rohr, T., Richardson, A. J., Lenton, A., Chamberlain, M. A., and Shadwick, E. H.: Zooplankton grazing is the largest source of uncertainty for marine carbon cycling in CMIP6 models, Commun. Earth Environ., 4, 1–22, https://doi.org/10.1038/s43247-023-00871-w, 2023. a, b, c, d
Sanderson, B. M., Knutti, R., and Caldwell, P.: Addressing Interdependency in a Multimodel Ensemble by Interpolation of Model Properties, J. Climate, 28, 5150–5170, https://doi.org/10.1175/JCLI-D-14-00361.1, 2015. a
Sanderson, B. M., Wehner, M., and Knutti, R.: Skill and independence weighting for multi-model assessments, Geosci. Model Dev., 10, 2379–2395, https://doi.org/10.5194/gmd-10-2379-2017, 2017. a, b
Sanderson, B. M., Pendergrass, A. G., Koven, C. D., Brient, F., Booth, B. B. B., Fisher, R. A., and Knutti, R.: The potential for structural errors in emergent constraints, Earth Syst. Dynam., 12, 899–918, https://doi.org/10.5194/esd-12-899-2021, 2021. a, b
Sauterey, B., Gland, G. L., Cermeño, P., Aumont, O., Lévy, M., and Vallina, S. M.: Phytoplankton adaptive resilience to climate change collapses in case of extreme events – A modeling study, Ecol Model., 483, 110437, https://doi.org/10.1016/j.ecolmodel.2023.110437, 2023. a
Séférian, R., Berthet, S., Yool, A., Palmiéri, J., Bopp, L., Tagliabue, A., Kwiatkowski, L., Aumont, O., Christian, J., Dunne, J., Gehlen, M., Ilyina, T., John, J. G., Li, H., Long, M. C., Luo, J. Y., Nakano, H., Romanou, A., Schwinger, J., Stock, C., Santana-Falcón, Y., Takano, Y., Tjiputra, J., Tsujino, H., Watanabe, M., Wu, T., Wu, F., and Yamamoto, A.: Tracking Improvement in Simulated Marine Biogeochemistry Between CMIP5 and CMIP6, Current Climate Change Reports, 6, 95–119, https://doi.org/10.1007/s40641-020-00160-0, 2020. a
Sellar, A. A., Jones, C. G., Mulcahy, J. P., Tang, Y., Yool, A., Wiltshire, A., O'Connor, F. M., Stringer, M., Hill, R., Palmieri, J., Woodward, S., de Mora, L., Kuhlbrodt, T., Rumbold, S. T., Kelley, D. I., Ellis, R., Johnson, C. E., Walton, J., Abraham, N. L., Andrews, M. B., Andrews, T., Archibald, A. T., Berthou, S., Burke, E., Blockley, E., Carslaw, K., Dalvi, M., Edwards, J., Folberth, G. A., Gedney, N., Griffiths, P. T., Harper, A. B., Hendry, M. A., Hewitt, A. J., Johnson, B., Jones, A., Jones, C. D., Keeble, J., Liddicoat, S., Morgenstern, O., Parker, R. J., Predoi, V., Robertson, E., Siahaan, A., Smith, R. S., Swaminathan, R., Woodhouse, M. T., Zeng, G., and Zerroukat, M.: UKESM1: Description and Evaluation of the U.K. Earth System Model, J. Adv. Model. Earth Sy., 11, 4513–4558, https://doi.org/10.1029/2019MS001739, 2019. a
Sieracki, M. E., Verity, P. G., and Stoecker, D. K.: Plankton community response to sequential silicate and nitrate depletion during the 1989 North Atlantic spring bloom, Deep-Sea Res. Pt. II, 40, 213–225, https://doi.org/10.1016/0967-0645(93)90014-E, 1993. a
Swart, N. C., Cole, J. N. S., Kharin, V. V., Lazare, M., Scinocca, J. F., Gillett, N. P., Anstey, J., Arora, V., Christian, J. R., Hanna, S., Jiao, Y., Lee, W. G., Majaess, F., Saenko, O. A., Seiler, C., Seinen, C., Shao, A., Sigmond, M., Solheim, L., von Salzen, K., Yang, D., and Winter, B.: The Canadian Earth System Model version 5 (CanESM5.0.3), Geosci. Model Dev., 12, 4823–4873, https://doi.org/10.5194/gmd-12-4823-2019, 2019. a, b
Tagliabue, A., Kwiatkowski, L., Bopp, L., Butenschön, M., Cheung, W., Lengaigne, M., and Vialard, J.: Persistent Uncertainties in Ocean Net Primary Production Climate Change Projections at Regional Scales Raise Challenges for Assessing Impacts on Ecosystem Services, Frontiers in Climate, 3, 738224, https://doi.org/10.3389/fclim.2021.738224, 2021. a, b, c, d, e, f, g
Tittensor, D. P., Novaglio, C., Harrison, C. S., Heneghan, R. F., Barrier, N., Bianchi, D., Bopp, L., Bryndum-Buchholz, A., Britten, G. L., Büchner, M., Cheung, W. W. L., Christensen, V., Coll, M., Dunne, J. P., Eddy, T. D., Everett, J. D., Fernandes-Salvador, J. A., Fulton, E. A., Galbraith, E. D., Gascuel, D., Guiet, J., John, J. G., Link, J. S., Lotze, H. K., Maury, O., Ortega-Cisneros, K., Palacios-Abrantes, J., Petrik, C. M., du Pontavice, H., Rault, J., Richardson, A. J., Shannon, L., Shin, Y.-J., Steenbeek, J., Stock, C. A., and Blanchard, J. L.: Next-generation ensemble projections reveal higher climate risks for marine ecosystems, Nat. Clim. Change, 11, 973–981, https://doi.org/10.1038/s41558-021-01173-9, 2021. a
Vancoppenolle, M., Bopp, L., Madec, G., Dunne, J., Ilyina, T., Halloran, P. R., and Steiner, N.: Future Arctic Ocean primary productivity from CMIP5 simulations: Uncertain outcome, but consistent mechanisms: FUTURE ARCTIC OCEAN PRIMARY PRODUCTIVITY, Global Biogeochem. Cy., 27, 605–619, https://doi.org/10.1002/gbc.20055, 2013. a, b
Vatanen, T., Osmala, M., Raiko, T., Lagus, K., Sysi-Aho, M., Orešič, M., Honkela, T., and Lähdesmäki, H.: Self-organization and missing values in SOM and GTM, Neurocomputing, 147, 60–70, https://doi.org/10.1016/j.neucom.2014.02.061, 2015. a
Wang, W.-L., Moore, J. K., Martiny, A. C., and Primeau, F. W.: Convergent estimates of marine nitrogen fixation, Nature, 566, 205–211, https://doi.org/10.1038/s41586-019-0911-2, 2019. a
Westberry, T., Behrenfeld, M. J., Siegel, D. A., and Boss, E.: Carbon-based primary productivity modeling with vertically resolved photoacclimation, Global Biogeochem. Cy., 22, 2007GB003078, https://doi.org/10.1029/2007GB003078, 2008. a
Whitt, D. B.: On the Role of the Gulf Stream in the Changing Atlantic Nutrient Circulation During the 21st Century, in: Geophysical Monograph Series, 1 edn., edited by: Nagai, T., Saito, H., Suzuki, K., and Takahashi, M., Wiley, 51–82, https://doi.org/10.1002/9781119428428.ch4, 2019. a, b
Williams, R. G., Roussenov, V., and Follows, M. J.: Nutrient streams and their induction into the mixed layer: NUTRIENT STREAMS AND INDUCTION, Global Biogeochem. Cy., 20, GB1016, https://doi.org/10.1029/2005GB002586, 2006. a
Williams, R. G., McDonagh, E., Roussenov, V. M., Torres-Valdes, S., King, B., Sanders, R., and Hansell, D. A.: Nutrient streams in the North Atlantic: Advective pathways of inorganic and dissolved organic nutrients, Global Biogeochem. Cy., 25, GB4008, https://doi.org/10.1029/2010GB003853, 2011. a
Wilson, J. D., Andrews, O., Katavouta, A., de Melo Viríssimo, F., Death, R. M., Adloff, M., Baker, C. A., Blackledge, B., Goldsworth, F. W., Kennedy-Asser, A. T., Liu, Q., Sieradzan, K. R., Vosper, E., and Ying, R.: The biological carbon pump in CMIP6 models: 21st century trends and uncertainties, P. Natl. Acad. Sci. USA, 119, e2204369119, https://doi.org/10.1073/pnas.2204369119, 2022. a
Xiu, P., Chai, F., Curchitser, E. N., and Castruccio, F. S.: Future changes in coastal upwelling ecosystems with global warming: The case of the California Current System, Sci. Rep., 8, 2866, https://doi.org/10.1038/s41598-018-21247-7, 2018. a
Yahi, H., Marticorena, B., Thiria, S., Chatenet, B., Schmechtig, C., Rajot, J. L., and Crepon, M.: Statistical relationship between surface PM10 concentration and aerosol optical depth over the Sahel as a function of weather type, using neural network methodology, J. Geophys. Res.-Atmos., 118, 13265–13281, https://doi.org/10.1002/2013JD019465, 2013. a
Yool, A., Popova, E. E., and Anderson, T. R.: MEDUSA-2.0: an intermediate complexity biogeochemical model of the marine carbon cycle for climate change and ocean acidification studies, Geosci. Model Dev., 6, 1767–1811, https://doi.org/10.5194/gmd-6-1767-2013, 2013. a, b
Zahariev, K., Christian, J. R., and Denman, K. L.: Preindustrial, historical, and fertilization simulations using a global ocean carbon model with new parameterizations of iron limitation, calcification, and N2 fixation, Prog. Oceanogr., 77, 56–82, https://doi.org/10.1016/j.pocean.2008.01.007, 2008. a, b
Ziehn, T., Chamberlain, M. A., Law, R. M., Lenton, A., Bodman, R. W., Dix, M., Stevens, L., Wang, Y.-P., and Srbinovsky, J.: The Australian Earth System Model: ACCESS-ESM1.5, Journal of Southern Hemisphere Earth Systems Science, 70, 193–214, https://doi.org/10.1071/ES19035, 2020. a