the Creative Commons Attribution 4.0 License.
the Creative Commons Attribution 4.0 License.
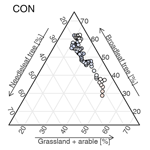
Fire hazard modulation by long-term dynamics in land cover and dominant forest type in eastern and central Europe
Angelica Feurdean
Boris Vannière
Walter Finsinger
Dan Warren
Simon C. Connor
Matthew Forrest
Johan Liakka
Andrei Panait
Christian Werner
Maja Andrič
Premysl Bobek
Vachel A. Carter
Basil Davis
Andrei-Cosmin Diaconu
Elisabeth Dietze
Ingo Feeser
Gabriela Florescu
Mariusz Gałka
Thomas Giesecke
Susanne Jahns
Eva Jamrichová
Katarzyna Kajukało
Jed Kaplan
Monika Karpińska-Kołaczek
Piotr Kołaczek
Petr Kuneš
Dimitry Kupriyanov
Mariusz Lamentowicz
Carsten Lemmen
Enikö K. Magyari
Katarzyna Marcisz
Elena Marinova
Aidin Niamir
Elena Novenko
Milena Obremska
Anna Pędziszewska
Mirjam Pfeiffer
Anneli Poska
Manfred Rösch
Michal Słowiński
Miglė Stančikaitė
Marta Szal
Joanna Święta-Musznicka
Ioan Tanţău
Martin Theuerkauf
Spassimir Tonkov
Orsolya Valkó
Jüri Vassiljev
Siim Veski
Ildiko Vincze
Agnieszka Wacnik
Julian Wiethold
Thomas Hickler
Wildfire occurrence is influenced by climate, vegetation and human activities. A key challenge for understanding the risk of fires is quantifying the mediating effect of vegetation on fire regimes. Here, we explore the relative importance of Holocene land cover, land use, dominant functional forest type, and climate dynamics on biomass burning in temperate and boreo-nemoral regions of central and eastern Europe over the past 12 kyr. We used an extensive data set of Holocene pollen and sedimentary charcoal records, in combination with climate simulations and statistical modelling. Biomass burning was highest during the early Holocene and lowest during the mid-Holocene in all three ecoregions (Atlantic, continental and boreo-nemoral) but was more spatially variable over the past 3–4 kyr. Although climate explained a significant variance in biomass burning during the early Holocene, tree cover was consistently the highest predictor of past biomass burning over the past 8 kyr. In temperate forests, biomass burning was high at ∼45 % tree cover and decreased to a minimum at between 60 % and 70 % tree cover. In needleleaf-dominated forests, biomass burning was highest at ∼ 60 %–65 % tree cover and steeply declined at >65 % tree cover. Biomass burning also increased when arable lands and grasslands reached ∼ 15 %–20 %, although this relationship was variable depending on land use practice via ignition sources, fuel type and quantities. Higher tree cover reduced the amount of solar radiation reaching the forest floor and could provide moister, more wind-protected microclimates underneath canopies, thereby decreasing fuel flammability. Tree cover at which biomass burning increased appears to be driven by warmer and drier summer conditions during the early Holocene and by increasing human influence on land cover during the late Holocene. We suggest that long-term fire hazard may be effectively reduced through land cover management, given that land cover has controlled fire regimes under the dynamic climates of the Holocene.
- Article
(3375 KB) - Full-text XML
-
Supplement
(2762 KB) - BibTeX
- EndNote
Wildfires can have dramatic environmental, economic and social impacts, as demonstrated by recent catastrophic fire events (Leverkus et al., 2019). However, fire is an integral part of many ecosystems and controls a range of evolutionary and ecological processes (Bond and Keeley, 2005; Bowman et al., 2009; Archibald et al., 2018). Fire regimes (i.e. fire frequency, size, intensity, severity, seasonality) are influenced by climate and vegetation properties (fuel moisture, availability, composition and structure) and vary both spatially and temporally (Bond and Keeley, 2005; van der Werf et al., 2010; Pausas and Paula, 2012; Archibald et al., 2018). A key challenge for understanding fire–climate interactions is quantifying the effect that vegetation properties have on mediating biomass burning. Overall, it has been hypothesized that, along a fuel load gradient, climate-induced fire hazard (ignition and spread) is lowest in both productive moist regions (with high fuel load provided by dense tree cover) and unproductive arid systems (with low fuel load and dominant grass and shrub cover) and is highest in intermediate systems that have a mixed fuel load provided by tree, shrub and grass cover (Pausas and Ribeiro, 2013). Grasses and shrubs are implicated in positive fire–fuel feedbacks, whereas an increase in tree cover beyond a specific threshold can reduce fire hazard, thereby fostering a negative feedback on fire (Beckage et al., 2009; Fréjaville et al., 2016). It has also been shown that plant functional traits (growth rate and architecture, leaf chemical composition and moisture content, litter decomposition) that determine flammability can mitigate climate-driven fire occurrence, leading to fire regimes other than those expected based solely on climate conditions (Girardin et al., 2013; Pausas and Ribeiro, 2013; Kloster et al., 2015; Rogers et al., 2015; Blarquez et al., 2015; Feurdean et al., 2017). For example, needleleaf trees with volatile compounds and resins, retention of dead biomass in the crown, ladder fuels, and slow litter decomposition rates promote fire hazard. Temperate broadleaf deciduous trees (with the exception of drought-adapted oaks) with high leaf moisture content and faster litter turnover usually have a low ignition probability and less flammable fuel, although under very dry conditions fire may spread with high intensity once fuel has been ignited (Sturtevant et al., 2009; Rogers et al., 2015).
Human activities, however, can also influence fuel load, composition and ignition patterns, thereby adding another level of complexity to fire regime variability and probability of occurrence. In this context, human activities are particularly relevant in Europe, where (i) a long history of human-driven decline in tree cover, often including the use of fire, is documented (Roberts et al., 2018) and (ii) forest extent has increased over the past few decades due to rural land abandonment, fire suppression and carbon abatement programmes (Jepsen et al., 2015). While high tree cover may reduce the likelihood of fire spread, widespread plantations of highly flammable trees (e.g. Pinus) produced by modern forestry may increase the probability and impact of catastrophic fires for human health, economy and ecosystems (Frejaville and Curt, 2017; Słowiński et al., 2019). In addition fire-promoting climatic conditions are also projected to increase in areas where natural fires were historically infrequent, e.g. in central and eastern Europe (Khabarov et al., 2016). However, the levels of forest cover and prevailing dominant tree types that will reduce or augment climate-driven fire hazard in the European context have not yet been quantified.
A fundamental limitation to understanding fire–climate–vegetation interactions based on observations or modelling approaches is that they are rooted in the modern environment. Yet, present-day ecosystems and fire regimes carry the legacies of past anthropogenic impact and climates (Marlon et al., 2016; Vannière et al., 2016). Palaeoecological studies based on pollen, plant macrofossils and charcoal sedimentary records provide centennial-to-millennial data sets to decipher past vegetation and fire dynamics and associated drivers. This is particularly relevant in forested ecosystems, which are dominated by species that have long generation times and fire return intervals that exceed observational records (Whitlock et al., 2017). Establishing how land cover changes influence fire regimes will be critical to inferring the probability of future fire occurrence and its impacts (Pausas and Paula, 2012; Whitlock et al., 2017).
Here we explore interactions between fire, land cover, land use and climate during the Holocene in major central and eastern European temperate and boreo-nemoral vegetation types. We use independent evidence of changes in fire, land cover composition and climate with a statistical modelling approach (generalized additive models, GAM) to quantify percentages in land cover and tree density associated with fire hazard.
2.1 Geographical location and charcoal site selection
To determine past biomass burned, we compiled a data set comprising 117 sedimentary charcoal records from sites located in central and eastern Europe (Fig. 1; File S1 in the Supplement). Of these, 70 records are from peatlands (bogs and fens), whereas 47 records are from lakes. For each record we compiled metadata including geographical coordinates, elevation, depositional environment and data source (File S1). Sedimentary charcoal is the most common proxy for determining relative changes in biomass burning (Whitlock and Larsen, 2001). While progress has been made in determining charcoal source areas, the quantification of absolute burned area from charcoal records is still challenging (Adolf et al., 2018). We therefore interpret the charcoal signal as indicative of relative trends in biomass burning (see Marlon et al., 2016). Regarding the depositional environment, bogs provide a more local representation of past fire occurrence than lakes, because they are characterized by limited charcoal transport and post-fire transport or erosion (Conedera et al., 2009; Rius et al., 2011). However, peatlands are susceptible to burning, which may introduce hiatuses in the depositional environment. The overall climate of the study area is temperate with considerable variability across regions due to marine or continental influences: the northern and eastern parts have long, cold winters and short, warm summers, whereas the central and southern parts have relatively warm, wet winters and dry, hot summers. Climate conditions also vary along an elevation gradient in this region.
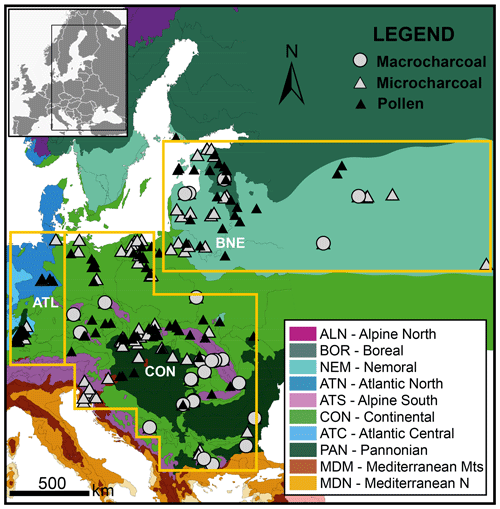
Figure 1Map showing the distribution of main environmental zones in central and eastern Europe (Metzger et al., 2005). Filled triangles and circles show the location of charcoal and pollen records. Orange rectangles denote the ecoregions analysed in this study: Atlantic (ATL), continental (CON) and boreo-nemoral (BNE).
In terms of fire activity, natural ignition sources such as lightning strikes occur at low frequencies, i.e. < 5 flashes km2 yr−1, and most fires are intentionally or accidentally ignited by humans (Christian et al., 2003). The average fire size is ∼10 ha in eastern Europe, between 5 and 10 ha in southern Europe and <5 ha in northern and central Europe (European Forest Fire Information System, https://effis.jrc.ec.europa.eu, last access: 24 February 2020). The number of fires per year is higher in northern, eastern and southern Europe (>50 fires yr−1) than in central Europe (1–50 fires yr−1).
2.2 Charcoal records and regional composite of biomass burning
Spatio-temporal patterns in fire–land cover interactions were investigated using a geographical delineation of central and eastern Europe (CEE) based on modern environmental stratification (Metzger et al., 2005). We defined three ecoregions within the central and eastern European region: continental (CON), boreo-nemoral (BNE), and Atlantic (ATL). The boreo-nemoral ecoregion includes 26 sites from the boreal and nemoral zones; the continental ecoregion includes 72 sites from the continental, alpine (conifer belt) and Pannonian zones, and the Atlantic region includes 19 sites from the Atlantic zone (Fig. 1).
All age measurements of the charcoal records were converted to calibrated years before present by using either the depth–age models provided by the original publications or new depth–age models established for this study (File S1). Charcoal concentrations were transformed into charcoal accumulation rates or influx (CHAR) by multiplying concentrations (charcoal counts (pieces cm−3) or charcoal areas (mm2 cm−3)) by sediment accumulation rates (cm yr−1) to account for variations in sedimentation among sequences. To allow comparison between and within charcoal records obtained from various depositional environments with different analytical methods and sampling resolution, we have applied the standardization technique established by Power et al. (2008) and modified by Daniau et al. (2012) and Blarquez et al. (2014). The standardization procedure included a min–max rescaling of CHAR values, followed by a Box–Cox transformation to homogenize within-record variance and a subsequent Z-score transformation using a base period from 12 to 0.15 ka. This base period includes the entire data set but excludes the effect of post-industrial human impact on fire activity. For compositing charcoal records by ecoregion, transformed charcoal records from each ecoregion were pre-binned in 100-year bins to reduce the influence of high-resolution records on the composite charcoal record. Pre-binned charcoal time series were smoothed with a LOWESS smoother with a 500-year window half width. Confidence interval values (95 %) were calculated by bootstrap resampling the binned charcoal series and calculating the mean of each bin 1000 times (default settings). For numerical processing of the CHAR series we used the R paleofire package version 4.0 (Blarquez et al., 2014). CHAR composite anomalies (100-year time interval) relative to the Holocene average of the entire CEE region and the three ecoregions represent regional trends in biomass burning, where zero Z-score values correspond to the mean charcoal influx over the base period, and positive/negative Z-score values represent greater-than-mean/lower-than-mean charcoal influx over the base period (Fig. 2).
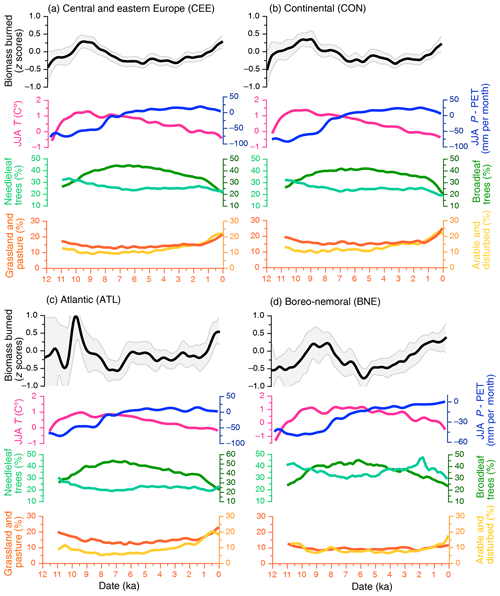
Figure 2Holocene trends in biomass burning, climate and land cover changes over all of central and eastern Europe (a) and in each of the following ecoregions: continental (b), Atlantic (c) and boreo-nemoral (d). Biomass burning is inferred based on charcoal influx (Z-score values). Climate conditions (anomalies) represent average simulated seasonal summer (June, July, August (JJA)) temperatures (T) and precipitation minus potential evapotranspiration (P−PET), from a global transient climate simulation. Relative abundance of needleleaf forests, broadleaf deciduous forests, grasslands and arable land is represented by their pollen-based percentages in relation to the total land cover (Fyfe et al., 2015). We fitted a 500-year LOESS smoother for JJA P−PET, temperature and land cover classes for each ecoregion.
2.3 Pollen-based regional composite of land cover classes
We used pollen-based land cover estimates at 200-year time intervals for the period 10.9 ka to present, based on the pseudobiomization method (Fyfe et al., 2015) to quantify changes in land cover type. The pseudobiomization approach groups pollen types into land cover classes that are directly comparable to other land cover classifications (Fyfe et al., 2015). These land cover estimates are available from the PANGAEA database for the entire study area, as opposed to other pollen-based quantitative vegetation reconstructions restricted mainly to northwestern Europe (Fyfe et al., 2015). We extracted six land cover classes: total forest, closed needleleaf forest, closed broadleaf deciduous forest, heath and scrubland, natural grasslands and open pastures, and arable and disturbed land cover other than heathland. Needleleaf forests are represented by the sum of pollen taxa dominated by Pinus, Picea, Abies and Larix, whereas broadleaf forests are represented by the sum of pollen of Quercus, Fagus, Betula, Carpinus, Ulmus, Tilia, Acer, Corylus and Alnus as the most common taxa. Heath and scrubland primarily includes Calluna, Empetrum, Ephedra, Erica, Hippophae and Juniperus (Fyfe et al., 2015). The natural grasslands and open pastures land cover class is a sum of a mixture of herb taxa, including pasture-specific taxa, whereas arable and disturbed land is defined by the sum of herb taxa typically adapted to cultivated and high-disturbance environments. For a full list of pollen taxa assigned to each land cover class see Fyfe et al. (2015). Throughout the text, we use the term “grassland cover” to denote both natural and human-modified grasslands (pastures) and “arable land cover” to denote arable and disturbed land, because it is not always possible to distinguish between natural and managed grasslands or between arable and other forms of disturbed open land cover based on pollen analysis (Fyfe et al., 2015). For example, the large proportion of open-land-cover classes (pasture and disturbed taxa) during the early Holocene (pre-Neolithic) is likely to represent natural landscape openness. We distinguished between the increase in arable–pasture cover and natural open land cover from the Neolithic onwards based on the change in abundance rather than on the absolute values (Fyfe et al., 2015). We assigned to each charcoal site the relative proportion of the six land cover classes from the nearest pollen site. We then generated composite estimates of land cover classes grouped by present-day ecoregions by spatially aggregating the averages of pollen records within the corresponding ecoregion.
2.4 Simulation of past climate conditions
Holocene climate conditions were derived from TraCE-21ka (Transient Climate Evolution over the last 21 000 years; Liu et al., 2009; He, 2011). This is a transient simulation of the last deglaciation phase (22 to 0 ka) using the fully coupled NCAR Community Climate System Model version 3 (CCSM3, Liu et al., 2009; He, 2011). Atmospheric and land model simulations were performed at the T31 (∼ 3.75∘ × 3.75∘) horizontal resolution and at approximately 3∘ in the ocean and sea-ice models. The simulation output data (near-surface temperature and precipitation) were downloaded at monthly temporal resolution from https://earthsystemgrid.org (last access: 24 February 2020). To remove systematic model biases, the climate simulation data were first bias-corrected using monthly climatologies between 1950 and 1980 from the Climatic Research Unit (CRU TS 3.1) observational data set (Harris et al., 2014). The bias correction was calculated with respect to the last 30 years of the TraCE-21ka simulation (representing pre-industrial conditions) as anomalies of the surface temperature and ratios of precipitation. The temperature anomalies were subsequently added, and precipitation ratios were multiplied by the CRU data in order to obtain the bias-corrected climate.
We used the boreal summer (June, July, August, hereafter JJA) surface temperature (JJA T) and precipitation minus potential evapotranspiration (JJA P−PET) as a proxy of peak summer dryness, which is a main driver of fire hazard. P−PET is a drought indicator that, opposed to P−AET (actual evapotranspiration), purely depends on atmospheric moisture demand independent of soil and vegetation and reflects the atmospherically driven intensity of drought conditions (Pidwirny, 2006). PET was calculated using the Thornthwaite model (Thornthwaite, 1948), which requires the surface temperature and average day length of each month as input variables. Surface temperature was taken directly from the bias-corrected TraCE-21ka data, and the average day length for each month going back to 12 ka was calculated using the Earth's orbital parameter scheme in CCSM3. The resulting bias-corrected climate fields were subsequently interpolated to the same locations as the charcoal records using a bilinear interpolation. We generated composite climate estimates by spatially aggregating individual climate records at 100-year time intervals within the corresponding ecoregions.
2.5 Generalized additive models
We developed generalized additive models (GAMs) to explore the response of biomass burning to changes in percent land cover, dominant functional forest type and JJA climate. GAMs have been show to provide robust statistical analyses of trends in palaeoenvironmental time series (Simpson, 2018). The predictor is the sum of smoothed functions of land cover and climate (Hastie and Tibshirani, 1990). We used the mgcv package to fit models with thin-plate-spline predictors and a Gaussian-family error distribution to automatically determine the optimal level of smoothing for each term in the model and automatic term selection (Hastie and Tibshirani, 1990). We calculated Akaike information criterion (AIC) weights to identify the models that were best able to predict the observed changes in biomass burning (Wagenmakers and Farrell, 2004). AIC weights are a normalized indicator of support for each model given the goodness of fit while penalizing more complex models (Hastie and Tibshirani, 1990). We obtained AIC values using the AIC function in R and calculated AIC weights relative to the model with the lowest AIC score using the qpcR package (Wood, 2017). AIC values can only be compared across a common data set; we therefore fitted the GAMs and calculated AIC weights separately for each ecoregion (including land cover classes, JJA T and JJA P−PET). Visual inspection of plots produced by the gam.precheck R function showed that all selected models were well-fit (File S2). We first explored the effect of JJA climate alone for the full period (12–0 ka) then constructed GAMs using JJA climate for 12–8 and 8–0 ka separately to investigate the relationship between climate and fire without significant human impact (see Sect. 2.3). We also restricted the GAMs including all predictors to the last 8 kyr as the proportion of open land cover classes (arable and grassland cover) during this period should predominantly reflect the influence of human impact (see Sect. 2.3).
3.1 Biomass burning, land cover and climate dynamics
The amount of biomass burning was highest during the early Holocene (between ∼10.5 and 8 ka) over all of central and eastern Europe and within the three ecoregions, although the onset of this biomass burning increase was earlier (11 ka) in the CON ecoregion (Fig. 2a–d). The climate-model simulation indicates warmer-than-present summer temperatures (JJA T) and lower-than-present moisture availability (JJA P−PET) in the early Holocene across all three ecoregions (Fig. 2b–d). Biomass burning became lower than at present between ∼8 and 4 ka in all ecoregions (Fig. 2b–d). The reduction in biomass burning accompanied a decrease in JJA temperature and an increase in summer moisture availability (around 8 ka) in all ecoregions (Fig. 2b–d). We found differences in trends in biomass burning among ecoregions over the past 3 kyr. Biomass burning increased markedly at 3 ka in the BNE ecoregion, but this increase is less evident in the CON ecoregion and occurs only around 1.5 ka in the ATL ecoregion (Fig. 2b–d). Climate simulations display generally cool, moist climate conditions in all ecoregions over the past 4 kyr (Fig. 2a–d).
Pollen-based land cover reconstructions indicate that tree cover ranged between ∼ 40 % and 65 % in CON, ∼ 45 % and 73 % in ATL, and ∼ 55 % and 80 % in BNE (Fig. 2b–d). Tree cover reached the maximum extent between ∼ 9 and 5 ka in all ecoregions and was dominated by mixed broadleaf deciduous trees, while the abundance of needleleaf trees was highest between ∼ 11 and 9 ka for all ecoregions and between 4 and 1 ka for the BNE ecoregion (Fig. 2b–d). Arable land cover ranged between ∼ 5 % and 17 % in BNE, ∼ 5 % and 22 % in ATL, and ∼ 10 % and 25 % in CON, whereas grassland cover ranged between ∼ 5 % and 12 % in BNE, ∼ 10 % and 22 % in ATL, and ∼ 15 % and 25 % in CON. Grassland cover reached the maximum extent over the past 1.5 kyr in all ecoregions, whereas arable land cover reached the maximum extent over the past 4 kyr in the ATL and CON ecoregions and 1.5 kyr in the BNE ecoregion (Fig. 2b–d). Three-dimensional scatter plots of biomass burning and percentages of land cover classes show that locations with greater biomass burning tend to be consistently characterized by low broadleaf and high needleleaf tree cover in all three ecoregions (Fig. 3). Biomass burning also increases with arable and pasture cover, although percentages of the two land cover classes at which biomass burning increases vary between ecoregions (Fig. 3).
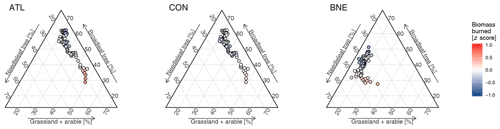
Figure 3Relationship between biomass burning, broadleaf tree cover, needleleaf tree cover, arable cover and grassland cover over the past 8 kyr in the three ecoregions in central and eastern Europe. Biomass burning and land cover are determined as above. Locations with greater biomass burning tend to be consistently characterized by low broadleaf tree cover in the CON and ATL ecoregions and by high needleleaf forest cover in the BNE ecoregion. In terms of land use, biomass burning increases with arable and pasture cover, but the patterns and thresholds vary between ecoregions, reflecting complex fire–human interactions. The coloured circles represent individual sampling points (n=80).
3.2 Generalized additive models
When considering GAMs fitted with only climate predictor variables over the full time series (12–0 ka), the proportion of the deviance of biomass burning in the three ecoregions averages 48 % (Table 1; File S2). We therefore investigated the drivers of biomass burning on the pre- and post-8 ka time periods separately. Climate alone explains a large proportion of the deviance of biomass burning for the 12–8 ka period (average 71.7 %), when biomass burning increased with increasing temperature and P−PET in all ecoregions (Table 1; Appendix A; File S2). On the contrary climate alone explains a considerably smaller proportion of the deviance (average 48 %) for the 8–0 ka period (Table 1; File S2). The full model selections procedure (climate and land cover) for the 8–0 ka period illustrated that the inclusion of land cover fractions in the GAMs increased the deviance explained to 76.6 % (Table 1; File S2). Furthermore, AIC values and weights for the full model selection procedure for the 8–0 ka period showed that models including land cover perform better in comparison to the models including climate alone in all ecoregions (Table 2). Evaluation of AIC values and weights demonstrates that most of the explanatory power (including >0.99 of the total cumulative AIC weight) comes from models that include broadleaf cover alone for the ATL and BNE ecoregions and the joint effects of total tree, broadleaf, heathland and arable cover for the CON ecoregion (Table 2; File S2). When examining the fire–tree cover relationships, we found that, in the ATL and CON ecoregions, biomass burning was high at 45 % tree cover, declining strongly towards 60 % tree cover (Fig. 4a). In the BNE ecoregion, biomass burning abruptly decreased as tree cover increased from its maximum of ∼ 65 % to 80 % (Fig. 4a). When examining fire–human relationships, biomass burning increased when arable and grassland cover reached ∼ 15 %–17 % in the ATL and CON ecoregions, and at ∼ 6 %–10 % in the BNE ecoregion (Figs. 3 and 4d, e). Biomass burning also increased for heathland cover greater than 12 % in the ATL and CON ecoregions (Fig. 4f; File S2).
Table 1Goodness of fit (deviance) and R2 adjusted for each ecoregion, including climate conditions only for the following periods: 12–0 ka (n=119), 12–8 ka (n=39), 8–0 ka (n=80).
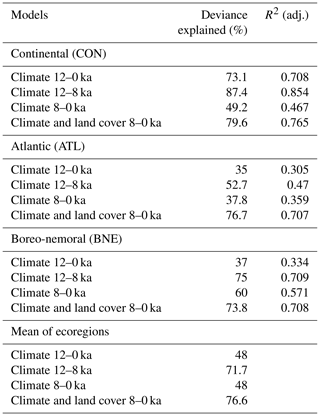
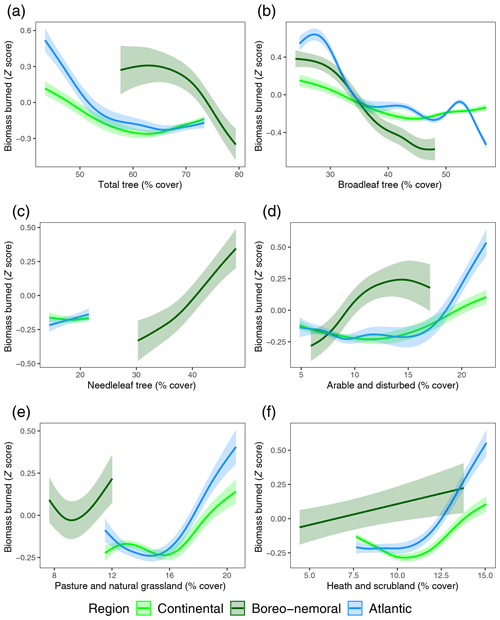
Figure 4Fire fuel type and load relationships in central and eastern Europe. The relationship between biomass burning, determined from Z-score composite charcoal values, and the main land cover types, derived from percentages of pollen-based land cover classes. These relationships were developed from generalized additive models (GAMs) for each ecoregion. Total tree cover (a), broadleaf tree cover (b), needleleaf tree cover (c), arable and disturbed cover (d), pastures and natural grasslands cover (e), and heath and scrubland cover (f).
Understanding fire regime variability is typically based on recent estimates of vegetation and burned area obtained from remote-sensing data as well as fire and vegetation models (Bistinas et al., 2014; Forkel et al., 2017). This may hinder our ability to recognize links and feedbacks between fire, vegetation and land use shifts, especially in ecosystems with species that have long generation times. Our study uses high-density millennial records of ecosystem history (vegetation, fire, climate, humans) and proposes a framework for testing the relationships between biomass burning, land cover, land use and climate conditions in three distinct ecoregions from central and eastern Europe.
4.1 The effect of tree cover and dominant functional forest type on fire
At the millennial scale considered here, we detected non-linear relations between biomass burning and percentages of tree cover (Fig. 4a). While we set up the GAMs with biomass burning as the response variable, we acknowledge that the relationship can be bidirectional: increased biomass burning can be a response to decreasing forest cover, but more frequent fires can also lead to a decrease in forest cover. Our findings are consistent with emerging evidence on fire–fuel relationships that suggests a strong link between tree cover and fire hazard in modern environments (Hirota et al., 2011; Pausas and Paula, 2012; Scheffer et al., 2012; Frejaville et al., 2016; van Nes et al., 2018). The shape of the fire–tree cover relationship could be explained by feedback mechanisms linking climate and fuel composition and structure. Low biomass burning at high tree cover may have been driven by dense tree stands with reduced understorey, which created a cool and moist microclimate that lowers ignition potential and fuel flammability (Kloster et al., 2015). Radiative properties of the land surface at higher tree cover can decrease evaporation and/or enhance cloud formation, which in turn contributes to a moister local climate (Teuling et al., 2017). A reduction in tree cover allows the development of more understorey vegetation that provides a favourable fuel mix composed of fine herbs, shrubs and coarse woody debris that, in turn, facilitates ignition and surface fire spread, as well as the transition from surface to crown fire (Pausas and Paula, 2012; Frejaville et al., 2016). Further, in open forests, radiation can penetrate more deeply into the canopy and the wind speed close to the ground is higher, which dries the understorey vegetation and litter, ultimately increasing flammability (Ryan, 2002).
The shape of the relationship between tree cover and biomass burning differs among the ecoregions, associated with the dominant functional forest type (Figs. 3 and 4a). In ecoregions dominated by temperate forests (CON and ATL), biomass burning is high at 45 % tree cover and declines towards ∼60 % tree cover (Fig. 4a). In the BNE ecoregion, where needleleaf trees dominate, the relationship is distinctly different: biomass burning decreases sharply as tree cover increases from 65 % to its maximum of 80 % (Fig. 4a). The pronounced shift in biomass burning in the BNE ecoregion resembles a system crossing a critical ecological threshold and transitioning to a new vegetation and/or fire regime state (Scheffer et al., 2012). The GAMs run separately on broadleaf and needleleaf forest type further demonstrate regional differences in the relationship between biomass burning and percentages of tree cover associated with the dominant functional forest type (Figs. 3, 4). Broadleaf cover had the most pronounced negative effect on biomass burning in the BNE and ATL ecoregions, and the second-most negative effect after total tree cover in the CON ecoregion (Fig. 4b; Table 1; File S2). Contrastingly, biomass burning shows an increase rather than a decrease with increasing needleleaf cover, which is evident in the BNE ecoregion with a considerable proportion of needleleaf forests (Fig. 4c; Table 1). This finding is in line with the ecological inference that deciduous broadleaf trees have low ignition probability and rates of fire spread (Sturtevant et al., 2009). Fire occurrence in boreal forest systems is often augmented at higher tree cover (up to 75 %) due to the high flammability of needleleaf biomass, in particular when it is exposed to dry, windy conditions (Scheffer et al., 2012; Rogers et al., 2015). Life history and morphological traits of the dominant species (relatively short life cycle, high relative growth rates, shallow roots, accumulation of dead biomass and slow litter decomposition) create a substantial amount of readily available dead fuel in boreal forests (Scheffer et al., 2012). Pollen records from central and eastern Europe additionally indicate shifts in the species composition within the needleleaf forest functional group (Giesecke et al., 2017) and consequently a change in fire-related vegetation traits. Abundant Pinus diploxylon-type pollen during the early Holocene indicates that Pinus sylvestris was the dominant needleleaf tree in all ecoregions at the time of high biomass burning. As a fire resister, Pinus sylvestris could have withstood fire as a result of its fire-resistant morphological traits, i.e. thick insulating bark, deep roots and a well-developed capacity for post-fire recovery (Groot et al., 2013; Rogers et al., 2015; Adámek et al., 2016; Feurdean et al., 2017; Carter et al., 2018; Bobek et al., 2019). Picea abies became the dominant needleleaf species in the CON ecoregion during the mid-Holocene and in the BNE ecoregion during the late Holocene (Giesecke et al., 2017), at times when biomass burning varied among ecoregions, but was lower compared to the early Holocene maxima. As a fire avoider, Picea abies does not have functional traits required for fire survival but boosts crown fires because its low branches create a ladder canopy structure and dead branches are retained in the crown.
4.2 The impact of climate on fire via conditions conducive to fire and fuel moisture
We found that climate, specifically warmer-than-present summer temperatures but still lower-than-present moisture content, between 12 and 8 ka exerted a positive control on biomass burning in all ecoregions (Table 1; Appendix A; File S2). Enhanced biomass burning with increasing temperature and moisture in the early Holocene in CEE is expected, as fuel builds up progressively following the cold and dry conditions with limited biomass that prevailed during the Late Glacial Interstadial (Feurdean et al., 2014). These climatic conditions, coupled with the fact that biomass was primarily composed of needleleaf trees, likely created a positive feedback on biomass burning. The finding of low biomass burning at low fuel amount under climate conditions conducive to fire (high temperatures, low moisture) supports the notion that climate-induced fire hazard is lower in less productive systems (Pausas and Ribeiro, 2013).
In contrast, GAMs indicate that the importance of tree cover on biomass burning became greater and the influence of climate less pronounced during the 8–0 ka period in all ecoregions (Tables 1, 2). For most of this period, biomass burning declined when temperature and moisture increased. Climate conditions also determined forest expansion, in particular broadleaf tree cover, with positive effects on fuel moisture and a dampening effect on biomass burning (see Sect. 4.1). Higher levels of burning over large areas in Europe during the early Holocene were generally associated with warmer summers and/or drier conditions, whereas a stronger effect of land cover for the middle to late Holocene was detected particularly at low to mid-latitudes (Vannière et al., 2016; Dietze et al., 2018; Molinari et al., 2018).
Table 2Model selection results for generalized additive models of the effects of land cover and climate on biomass burning for the period 8–0 ka. Model selection metrics were obtained using the summary functions in the mgcv and qpcR packages in R. Lower values of Akaike information criterion (AIC) and higher values of AIC weights identify the models that were best able to predict the observed changes in biomass burning. Delta AIC values measure the relative performance of each model compared to the best model for that region; a delta AIC of >2 between two models is typically considered to indicate a significant difference in explanatory power.
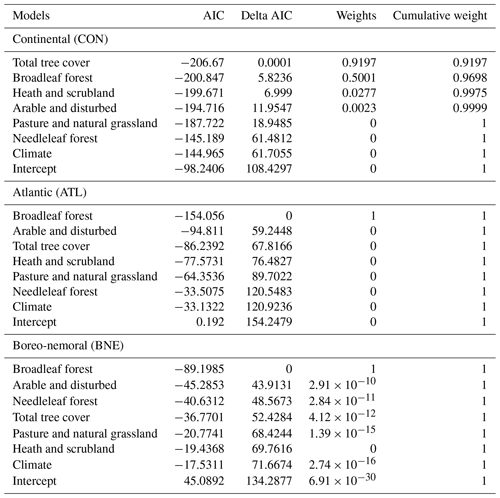
Proxy data do not provide all climate variables (T, P−PET) and the monthly resolution available from climate models. Proxy-based climate data sets were used to check whether the model simulations depict general trends in past climate conditions. TraCE-21ka simulation and proxy-based climate reconstructions are generally in good agreement, with both indicating warm and dry climate conditions for the early Holocene and increased moisture availability during the mid-Holocene in all ecoregions and cooler summer temperatures in the CON ecoregion (Davis and Brewer, 2009; Heiri et al., 2015; Veski et al., 2015; Tóth et al., 2015; Hájková et al., 2016; Diaconu et al., 2017; Marcisz et al., 2017). Although the climate simulation and most proxy-based climate reconstructions show cool and moist climate conditions throughout the late Holocene, proxy-based reconstructions indicate greater spatial and temporal climate variability (Davis and Brewer, 2009; Heiri et al., 2015; Tóth et al., 2015; Diaconu et al., 2017; Marcisz et al., 2017). On the one hand, this disagreement could be explained by the coarse resolution of the climate model. Further, the model does not account for changes in the length of individual months or seasons due to variations in Earth's orbit (e.g. Bartlein and Shafer, 2019). Therefore future work might analyse the monthly time series instead of seasonal averages. On the other hand, increasing human impact on the proxies used for climate reconstructions, such as the effect of water acidification and eutrophication on chironomid assemblages and deforestation on testate amoebae composition, could also be responsible for disagreement between proxy- and simulation-based inference of past climate conditions (Heiri et al., 2015).
4.3 The human impact on fire
Humans have likely altered the temporal and spatial structure of fuel availability and timing and frequency of ignitions since the early Holocene (Pfeiffer et al., 2013; Marlon et al., 2016; Vannière et al., 2016; Andela et al., 2017; Dietze et al., 2018) and potentially even earlier (Kaplan et al., 2016). While ignitions tend to increase until the population reaches intermediate density, human-caused change in land cover from forest to arable land and associated fuel limitation and landscape fragmentation may result in a decline in biomass burning (Pfeiffer et al., 2013; Andela et al., 2017). A contrasting position suggests that increasing population density exclusively reduces fire frequency and burned area through the impact of land conversion and landscape fragmentation on fuel availability and continuity (Knorr et al., 2014). Our results reveal that biomass burning mostly shows a positive response to increases in arable and grassland cover in all ecoregions. However, this relationship is variable and may illustrate a complex fire–human interaction (Figs. 3, 4). In the CON ecoregion, the most evident increase in biomass burning occurred after 3 ka (Fig. 2b) and is consistent with percentages in arable and grassland cover at which biomass burning shows positive responses in the GAMs (Fig. 4d, e). Historically, the onset of this rise in biomass burning corresponds to the Late Bronze Age to the Iron Age periods characterized by the establishment of urban centres, farms, early industries and mining activities (Rösch, et al., 2014; Chapman, 2017). The sharp increase in biomass burning over the last millennium coincides with a marked population growth and renewed deforestation (Jamrichova et al., 2017; Marquer et al., 2017). It is therefore apparent that land use intensification in the CON ecoregion involved deforestation-related burning that accompanied creation of arable land, starting with the Bronze or Iron Age and reaching a maximum during the early modern period. In the ATL ecoregion, arable and grassland cover rose steadily from ∼4 to 1.5 ka, but biomass burning remained constant during the same time (Fig. 2), which is consistent with percentages in arable and grassland cover at which biomass burning shows no responses in the GAMs (Fig. 4c, d). This may suggest that the local intensification in land use between 4 and 1.5 ka did not involve major use of fire for deforestation (Fig. 2). If fire was primarily restricted to burning of agricultural waste, e.g. straw and chaff, to improve soil fertility and clean the land, this should have provided less biomass to burn than wood (Pfeiffer et al., 2013). However, the further increase in arable and grassland cover in the ATL ecoregion from ∼1 ka onwards coincident with a rise in biomass burning may be explained by renewed deforestation activity at marginal sites during medieval times (Marquer et al., 2017). In the BNE ecoregion, we detected increases in biomass burning over the past 4 kyr, while the rise in abundance of arable and grassland cover to values at which biomass burning shows the strongest positive responses only occurred over the past 2 kyr (Fig. 2d). It therefore appears that the rise in biomass burning at 4 ka could be primarily related to the naturally or human-driven increase in the needleleaf component and only after 2 ka to a perpetual use of fire for deforestation and agricultural activities (Fig. 2d). The relatively late occurrence of land conversion in the BNE ecoregion is probably due to the fact that broadleaf forests were edaphically more suitable for conversion to arable fields and pastures than needleleaf forests (Roberts et al., 2018). It should be noted that inferences of fire regime from sedimentary charcoal have their limitations, in particular with respect to the cause of fires. Therefore, if humans have significantly increased fire frequency since prehistory (Vannière et al., 2016) or earlier (Kaplan et al., 2016), the impact of post-deforestation mosaic landscape heritage and the land use on fuel amounts may have reduced the probability of large fires and consequently the amount of charcoal preserved in the depositional environments. Nevertheless, our fuel–fire relationship using pooled charcoal and pollen records reflects a regional pattern, and individual sites may more effectively depict small-scale alterations in land cover and burning pattern (Gavin et al., 2006).
4.4 Potential implications for fire–vegetation modelling
Global fire–vegetation models are useful tools for projecting future changes in fire regimes and assessing fire–vegetation interactions. Improving such models is an area of active development (Rabin et al., 2017) and typically utilizes recent estimates of burned area and a few land cover types obtained from remote-sensing data and other vegetation-related products to evaluate the models (Bistinas et al., 2014; Forkel et al., 2017). However, this reliance on short-term data does not offer the full picture of fire–vegetation interactions, particularly as fire regimes and present-day ecosystems carry legacies of past anthropogenic activities (Vannière et al., 2016). The analysis presented here provides complementary evidence to evaluate fire–vegetation model development in several ways. Firstly, the fire–land cover relationships emerging from fire–vegetation models can be evaluated against the charcoal- and pollen-derived fire–land cover relationships attained here. Secondly, synthesized charcoal records provide data for fire model evaluation in regions where fire return intervals are significantly longer than the short time for which satellite data are available. Finally, the coherent time series presented here provide an independent test case, i.e. under different climates and with different, limited or no human influence, for fire models outside of the time period in which they were calibrated. Once we incorporate these aspects into fire–vegetation models, we can more accurately model changes through time, i.e. the past range of fire regimes (Pfeiffer et al., 2013; Forkel et al., 2017), and examine critical ecological transitions hypothesized to be mediated by fire–vegetation interactions (Scheiter et al., 2012).
Our results provide compelling evidence that land cover, especially percent of tree cover, was strongly related to biomass burning for most of the Holocene. Regional differences, however, were observed among major vegetation types. Specifically, in ecoregions dominated by temperate forests (CON and ATL), biomass burning was high at 45 % tree cover and declined towards ∼60 % tree cover. In the BNE ecoregion where needleleaf trees dominate, biomass burning was highest at ∼ 60 %–65 % tree cover and abruptly declined at >65 % tree cover. The non-linear biomass burning shift in the BNE ecoregion resembles a system crossing a critical ecological threshold and transitioning to a new state. Biomass burning showed a positive response when arable and grassland cover reached ∼ 15 %–20 %, but this relationship is regionally distinct and highlights the complex fire–human interactions that depend on land use intensity in the different ecoregions. A higher tree cover reduces radiative energy influx to the forest floor and provides moister, more wind-protected microclimates, which decreases fuel flammability. Our evaluation of past fire–fuel interactions indicate that tree cover is a first-order predictor of the probability of fire occurrence whatever the driver of vegetation dynamic. Our observations cover the full range of Holocene climate variability and therefore provide a long-term test of vegetation–climate–fire interactions. An important implication of this test is that effective mitigation of future fire hazard relies on regional-scale land cover management. Information derived from such long-term fire–vegetation relationships can be used to improve fire mitigation strategies and fire–vegetation models. Future work should also include an examination of post-fire vegetation responses to shifts in fire frequency and intensity, i.e. a higher forest cover may lead to less frequent fires, but more frequent fires can also lead to a decrease in forest cover, and possible ways of fire hazard mitigation.
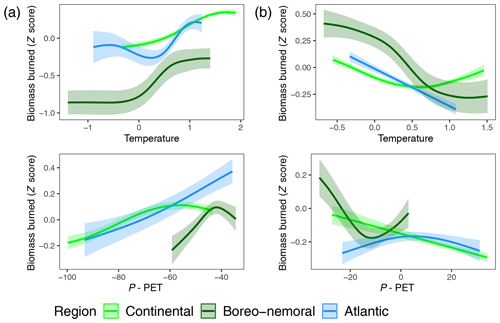
Figure A1Fire–climate relationship in the three ecoregions of central and eastern Europe for 12–8 ka (a) and 8–0 ka (b), respectively. The relationship between biomass burning, determined from Z-score composite charcoal values, and simulated seasonal summer (June, July, August (JJA)) temperatures and precipitation minus potential evapotranspiration (P−PET) was developed from generalized additive models (GAMs).
R code, plots and descriptive information demonstrating the development of generalized additive models (GAMs) are presented in File S2 in the Supplement and at: https://www.paleofire.org/index.php?p=exportceed (Central East European Database, 2020).
All essential charcoal input and output data are open access and available online at: https://www.paleofire.org/index.php?p=exportceed (Central East European Database, 2020).
File S1 contains metadata. File S2 contains R code; plots; and descriptive information demonstrating the development of generalized additive models (GAMs) to explore the relationship between fire, land cover classes and climate for each region. The supplement related to this article is available online at: https://doi.org/10.5194/bg-17-1213-2020-supplement.
AF, BV and WF designed the study with contributions from TH, MF and DW. AF compiled site-based data with the help of GF. AF performed the analyses. DW, MF, AP, JL and CW developed the model codes and/or performed the modelling. All other authors provided data or carried out a minor component of data compilation or analysis. AF prepared the paper with contributions from BV, WF, SCC and MP and input from all the authors.
The authors declare that they have no conflict of interest.
TraCE-21ka was made possible by the DOE INCITE computing program and supported by NCAR, the NSF P2C2 program, and the DOE Abrupt Change and EaSM programs. We thank Marc Metzger for providing the environmental stratification of Europe and Olivier Blarquez for guidance with the paleofire package.
This study is based on the PAGES Global Charcoal Database (https://www.paleofire.org, last access: 22 February 2020) initiative developed by the Global Paleofire Working Group phase 2 (https://www.paleofire.org/index.php, last access: 24 February 2020), which in turn received support from the US National Science Foundation and the Swiss Academy of Sciences. This database is hosted and funded by the Chrono-environment laboratory at the University of Burgundy Franche-Comté (France). Data consolidation was undertaken during a PAGES-funded workshop in 2016 in Frankfurt, Germany.
This research has primarily been supported by the German Research
Foundation (grant nos. FE-1096/4-1 and FE-1096/6-1). Additional financial support also came from the Slovenian Research Agency (grant nos. P6-0064 and J7-6857); the UEFISCDI in Romania (grant no. PN-III-P4-ID-PCE-2016-0711); the Research Council of Lithuania (grant no. S-MIP-17-133); the Swiss Government Excellence Postdoctoral Scholarship (grant no. FIRECO 2016.0310); the National Science Centre in Poland (grant nos. 2015/17/B/ST10/01656 and 2015/17/B/ST10/03430); the Czech Science Foundation (grant nos. 16-06915S, 19-14271Y and GA14-22658S); the Estonian Research Council (grant no. PRG323); the Czech Academy of Sciences (grant no. RVO 67985939); the ANR OBRESOC project (grant no. ANR 09-CEP-004-01); and the Hungarian National Research, Development and Innovation Office (grant nos. NKFIH 101362 and GINOP-2.3.2-15-2016-00019).
The publication of this article was funded by the
Open Access Fund of the Leibniz Association.
This paper was edited by Kirsten Thonicke and reviewed by Graciela Gil Romera, Patrick Bartlein, and Christoph Schwörer.
Adámek, M., Hadincová, V., and Wild, J.: Long-term effect of wildfires on temperate Pinus sylvestris forests: Vegetation dynamics and ecosystem resilience, Forest Ecol. Manage., 380, 285–295, https://doi.org/10.1016/j.foreco.2016.08.051, 2016.
Adolf, C., Wunderle, S., Colombaroli, D., Weber, H., Gobet, E., Heiri, O., van Leeuwen, J. F. N. C., Bigler, C., Connor, S. E., Galka, M., La Mantia, T., Makhortykh, S., Svitavska-Svobodova, H., Vanniere, B., and Tinner, W.: The sedimentary and remote-sensing reflection of biomass burning in Europe. Global Ecol. Biogeogr., 27, 199–212, https://doi.org/10.1111/geb.12682, 2018.
Andela, N., Morton, D. C., Giglio, L., Chen, Y., van der Werf, G. R., Kasibhatla, P. S., DeFries, R. S., Collatz, G. J., Hantson, S., Kloster, S., Bachelet, D., Forrest, M., Lasslop, G., Li, F., Mangeon, S., Melton, J. R., Yue, C., and Randerson, J. T.: A human-driven decline in 10 global burned area, Science, 356, 1356–1362, https://doi.org/10.1126/science.aal4108, 2017.
Archibald, S., Lehmann, C. E. R., Belcher, C. M., Bond, W. J., Bradstock, R. A., Daniau, A. L., Dexter, K. G., Forrestel, E. J., Greve, M., He, T., Higgins, S. I., Hoffmann, W. A., Lamont, B. B., McGlinn, D. J., Moncrieff, G. R., Osborne, C. P., Pausas, J. G., Price, O., Ripley, B. S., Rogers, B. M., Schwilk, D. W., Simon, M. F., Turetsky, M. R., Van Der Werf, G. R., and Zanne, A.: Biological and geophysical feedbacks with fire in the Earth system. Environ. Res. Lett., 13, 033003, https://doi.org/10.1088/1748-9326/aa9ead, 2018.
Bartlein, P. J. and Shafer, S. L.: Paleo calendar-effect adjustments in time-slice and transient climate-model simulations (PaleoCalAdjust v1.0): impact and strategies for data analysis, Geosci. Model Dev., 12, 3889–3913, https://doi.org/10.5194/gmd-12-3889-2019, 2019.
Beckage, B., Platt, W. J., and Gross, L. J.: Vegetation, fire, and feedbacks: A disturbance mediated model of savannas, Am. Nat., 174, 805–818, https://doi.org/10.1086/648458, 2019.
Bistinas, I., Harrison, S. P., Prentice, I. C., and Pereira, J. M. C.: Causal relationships versus emergent patterns in the global controls of fire frequency, Biogeosciences, 11, 5087–5101, https://doi.org/10.5194/bg-11-5087-2014, 2014.
Blarquez, O., Vannière, B., Marlon, J. R., Daniau, A.-L., Power, M. J., Brewer, S., and Bartlein, P. J.: Paleofire An R package to analyse sedimentary charcoal records from the Global Charcoal Database to reconstruct past biomass burning, Comput. Geosci., 72, 255–261, https://doi.org/10.1016/j.cageo.2014.07.020, 2014.
Blarquez, O., Ali, A. A., Girardin, M. P., Grondin, P., Fréchette, B., Bergeron, Y., and Hély, C.: Regional paleofire regimes affected by non-uniform climate, vegetation and human drivers, Sci. Rep.-UK, 5, 13356, https://doi.org/10.1038/srep13356, 2015.
Bobek, P., Svitavská, H., Pokorný, P., Šamonil, P., Kuneš, P., Kozáková, R., Abraham, V., Klinerová, T., Švarcová, M. G., Jamrichov,á, E., Krauseová, E., and Wild, J.: Divergent fire history trajectories in Central European temperate forests revealed a pronounced influence of broadleaved trees on fire dynamics, Quaternary Sci. Rev., 222, 105865, https://doi.org/10.1016/j.quascirev.2019.105865, 2019.
Bond, W. J. and Keeley, J. E.: Fire as a global herbivore: the ecology and evolution of flammable ecosystems, Trends Ecol. Evol., 20, 387–394, https://doi.org/10.1016/j.tree.2005.04.025, 2005.
Bowman, D. M. J. S., Balch, J. K., Artaxo, P., Bond, W. J., Carlson, J. M., Cochrane, M. A., D'Antonio, C. M., DeFries, R. S., Doyle, J. C., Harrison, S. P., Johnston, F. H., Keeley, J. E., Krawchuk, M. A., Kull, C. A., Marston, J. B., Moritz, M. A., Prentice, I. C., Roos, C. I., Scott, A. C., Swetnam, T. W., van der Werf, G. R., and Pyne, S. J.: Fire in the Earth System, Science, 324, 481–484, https://doi.org/10.1126/science.1163886, 2009.
Carter, V. A., Moravcová, A., Chiverrell, R. C., Clear, J. L., Finsinger, W., Dreslerová, D., Halsall, K., and Kuneš, P.: Holocene-scale fire dynamics of central European temperate spruce-beech forests, Quaternary Sci. Rev., 191, 15–30, https://doi.org/10.1016/j.quascirev.2018.05.001, 2018.
Central East European Database: CEE-GCD-2020_Feurdean_et_al_2020, available at: https://www.paleofire.org/index.php?p=exportceed, last access: 24 February 2020.
Chapman, J.: Climatic and human impact on the environment? A question of scale, Quaternary Int., 496, 3–13, https://doi.org/10.1016/j.quaint.2017.08.010, 2017.
Christian, H. J., Blakeslee, R. J., Boccippio, D. J., Boeck, W. L., Buechler, D. E., Driscoll, K. T., Goodman, S. J., Hall, J. M., Koshak, W. J., Mach, D. M., and Stewart, M. F.: Global frequency and distribution of lightning as observed from space by the Optical Transient Detector, J. Geophys. Res.-Atmos., 108, ACL 4-1–ACL 4-15, https://doi.org/10.1029/2002JD002347, 2003.
Conedera, M., Tinner, W., Neff, C., Meurer, M., Dickens, A. F., and Krebs, P.: Reconstructing past fire regimes: methods, applications, and relevance to fire management and conservation, Quaternary Sci. Rev., 28, 555–576, https://doi.org/10.1016/j.quascirev.2008.11.005, 2009.
Daniau, A. L., Bartlein, P. J., Harrison, S. P., Prentice, I. C., Brewer, S., Friedlingstein, P., Harrison-Prentice, T. I., Inoue, J., Izumi, K., Marlon, J. R., Mooney, S., Power, M. J., Stevenson, J., Tinner, W., Andrič, M., Atanassova, J., Behling, H., Black, M., Blarquez, O., Brown, K. J., Carcaillet, C., Colhoun, E. A., Colombaroli, D., Davis, B. A. S., D'Costa, D., Dodson, J., Dupont, L., Eshetu, Z., Gavin, D. G., Genries, A., Haberle, S., Hallett, D. J., Hope, G., Horn, S. P., Kassa, T. G., Katamura, F., Kennedy, L. M., Kershaw, P., Krivonogov, S., Long, C., Magri, D., Marinova, E., McKenzie, G. M., Moreno, P. I., Moss, P., Neumann, F. H., Norström, E., Paitre, C., Rius, D., Roberts, N., Robinson, G. S., Sasaki, N., Scott, L., Takahara, H., Terwilliger, V., Thevenon, F., Turner, R., Valsecchi, V. G., Vannière, B., Walsh, M., Williams, N., and Zhang, Y.: Predictability of biomass burning in response to climate changes, Global Biogeochem. Cy., 26, GB4007, https://doi.org/10.1029/2011GB004249, 2012.
Davis, B. A. S. and Brewer, S.: Orbital forcing and role of the latitudinal insolation/ temperature gradient, Clim. Dynam., 32, 143–165, https://doi.org/10.1007/s00382-008-0480-9, 2009.
Diaconu, A. C., Tóth, M., Lamentowicz, M., Heiri, O., Kuske, E., Tanţău, I., Panait, A., Braun, M., and Feurdean, A.: How warm? How wet? Hydroclimate reconstruction of the past 7500 years in northern Carpathians, Romania, Palaeogeogr. Palaeocl., 482, 1–12, https://doi.org/10.1016/j.palaeo.2017.05.007, 2017.
Dietze, E., Theuerkauf, M., Bloom, K., Brauer, A., Dörfler, W., Feeser, I., Feurdean, A., Gedminienė, L., Giesecke, T., Jahns, S., Karpińska-Kołaczek, M., Kołaczek, P., Lamentowicz, M., Latałowa, M., Marcisz, K., Obremska, M., Pędziszewska, A., Poska, A., Rehfeld, K., Stančikaitė, M., Stivrins, N., Święta-Musznicka, J., Szal, M., Vassiljev, J., Veski, S., Wacnik, A., Weisbrodt, D., Wiethold, J., Vannière, B., and Słowiński, M.: Holocene fire activity during low-natural flammability periods reveals scale-dependent cultural human-fire relationships in Europe, Quaternary Sci. Rev., 201, 44–56, https://doi.org/10.1016/j.quascirev.2018.10.005, 2018.
Feurdean, A., Perşoiu, A., Tanţău, I., Stevens, T., Magyari, E. K., Onac, B. P., Marković, S., Andrič, M., Connor, S., Fărcaş, S., Gałka, M., Gaudeny, T., Hoek, W., Kolaczek, P., Kuneš, P., Lamentowicz, M., Marinova, E., Michczyńska, D. J., Perşoiu, I., Płociennik, M., Słowiński, M., Stancikaite, M., Sumegi, P., Svensson, A., Tămaş, T., Timar, A., Tonkov, S., Toth, M., Veski, S., Willis, K. J., and Zernitskaya, V.: Climate variabilityand associated vegetation response throughout Central and Eastern Climate variability and associated vegetation response throughout Central and Eastern Europe (CEE) between 60 and 8 ka, Quaternary Sci. Rev., 106, 206–224, https://doi.org/10.1016/j.quascirev.2014.06.003, 2014.
Feurdean, A., Veski, S., Florescu, G., Vannière, B., Pfeiffer, M., O'Hara, R. B., Stivrins, N., Amon, L., Heinsalu, A., Vassiljev, J., and Hickler, T.: Broadleaf deciduous forest counterbalanced the direct effect of climate on Holocene fire regime in hemiboreal/boreal region (NE Europe), Quaternary Sci. Rev., 169, 378–390, https://doi.org/10.1016/j.quascirev.2017.05.024, 2017.
Forkel, M., Dorigo, W., Lasslop, G., Teubner, I., Chuvieco, E., and Thonicke, K.: A data-driven approach to identify controls on global fire activity from satellite and climate observations (SOFIA V1), Geosci. Model Dev., 10, 4443–4476, https://doi.org/10.5194/gmd-10-4443-2017, 2017.
Frejaville, T. and Curt, T.: Seasonal changes in the human alteration of fire regimes beyond the climate forcing, Environ. Res. Lett., 12, 035006, https://doi.org/10.1088/1748-9326/aa5d23, 2017.
Fréjaville, T., Curt, T., and Carcaillet, C.: Tree cover and seasonal precipitation drive understorey flammability in alpine mountain forests, J. Biogeogr., 43, 1869–1880, https://doi.org/10.1111/jbi.12745, 2016.
Fyfe, R. M., Woodbridge, J., and Roberts N.: From forest to farmland: pollen inferred land cover change across Europe using the pseudobiomization approach, Glob. Change Biol., 21, 1197–1212, https://doi.org/10.1111/gcb.12776, 2015.
Gavin, D. G., Hu, F. S., Lertzman, K., and Corbett, P.: Weak climatic control of standscale fire history during the late Holocene, Ecology, 87, 1722–1732, https://doi.org/10.1890/0012-9658(2006)87[1722:WCCOSF]2.0.CO;2, 2006.
Giesecke, T., Brewer, S., Finsinger, W., Leydet, M., and Bradshaw, R. H.: Patterns and dynamics of European vegetation change over the last 15,000 years, J. Biogeogr., 44, 1441–1456, https://doi.org/10.1111/jbi.12974, 2017.
Girardin, M. P., Ali, A. A., Carcaillet, C., Blarquez, O., Hély, C., Terrier, A., Genries, A., and Bergeron, Y.: Vegetation limits the impact of a warm climate on boreal wildfires, New Phytol., 199, 1001–1011, https://doi.org/10.1111/nph.12322, 2013.
Grooth, W. J., Cantin, A. S., Flannigan, M. D., Soja, A. J., Gowman, L. M., and Newbery, A.: A comparison of Canadian and Russian boreal forest fie regimes, Forest Ecol. Manage., 294, 23–34, https://doi.org/10.1016/j.foreco.2012.07.033, 2013.
Hájková, P., Pařil, P., Petr, L., Chattová, B., Grygar, T. M., and Heiri, O.: A first chironomid-based summer temperature reconstruction (13–5 ka BP) around 49∘ N in inland Europe compared with local lake development, Quaternary Sci. Rev., 141, 94–111, https://doi.org/10.1016/j.quascirev.2016.04.001, 2016.
Harris, I., Jones, P. D., Osborn, T. J., and Lister, D. H.: Updated high-resolution grids of monthly climatic observations – the CRU TS3.10 Dataset, Int. J. Climatol., 34, 623–642, https://doi.org/10.1002/joc.3711, 2014.
Hastie, T. J. and Tibshirani, R. J.: Generalized additive models, Vol. 43 of Monographs on Statistics and Applied Probability, Taylor and Francis Group, Chapman & Hall/CRC, 1990.
He, F.: Simulating transient climate evolution of the last deglaciation with CCSM3, PhD thesis, University of Wisconsin-Madison, available at: https://www.researchgate.net/publication/263618839_Simulating_transient_climate_evolution_of_the_last_deglaciation_with_CCSM3 (last access: 24 February 2020), 2011.
Heiri, O., Ilyashuk, B., Millet, L., Samartin, S., and Lotter, A. F.: Stacking of discontinuous regional paleoclimate records: chironomid-based summer temperaturesfrom the Alpine region, Holocene, 25, 137–149, https://doi.org/10.1177/0959683614556382, 2015.
Hirota, M., Holmgren, M., and Van Nes, E. H., and Scheffer, M.: Global resilience of tropical forest and savanna to critical transitions, Science, 334, 232–235, https://doi.org/10.1126/science.1210657, 2011.
Jamrichová, E., Petr, L., Jiménez‐Alfaro, B., Jankovská, V., Dudová, L., Pokorný, P., Kołaczek, P., Zernitskaya, V., Čierniková, M., Břízová, E., and Syrovátka, V.: Pollen-inferred millennial changes in landscape patterns at a major biogeographical interface within Europe, J. Biogeogr., 44, 2386–2397, https://doi.org/10.1111/jbi.13038, 2017.
Jepsen, M. R., Kuemmerle, T., Müller, D., Erb, K., Verburg, P. H., Haberl, H., Vesterager, J. P., Andrič, M., Antrop, M., Austrheim, G., Björn, I., Bondeau, A., Bürgi, M., Bryson, J., Caspar, G., Cassar, L. F., Conrad, E., Chromý, P., Daugirdas, V., Van Eetvelde, V., Elena-Rosselló, R., Gimmi, U., Izakovicova, Z., Jančák, V., Jansson, U., Kladnik, D., Kozak, J., Konkoly-Gyuró, E., Krausmann, F., Mander, Ü., McDonagh, J., Pärn, J., Niedertscheider, M., Nikodemus, O., Ostapowicz, K., Pérez-Sobaa, M., Pinto-Correia, T., Ribokas, G., Rounsevell, M., Schistou, D., Schmit, C., Terkenli, T. S., Tretvik, A. M., Trzepacz, P., Vadineanu A., Walz, A., Zhllima, E., and Reenberg, A.: Transitions in European land-management regimes between 1800 and 2010, Land Use Policy, 49, 53–64, https://doi.org/10.1016/j.landusepol.2015.07.003, 2015.
Kaplan, J. O., Pfeiffer, M., Kolen, J. C. A., and Davis, B. A. S.: Large Scale Anthropogenic Reduction of Forest Cover in Last Glacial Maximum Europe, PLOS ONE, 11, e0166726, https://doi.org/10.1371/journal.pone.0166726, 2016.
Khabarov, N., Krasovskii, A., and Obersteiner, M.: Forest fires and adaptation options in Europe, Reg. Environ. Change, 16, 21–30, https://doi.org/10.1007/s10113-014-0621-0, 2016.
Kloster, S., Brücher, T., Brovkin, V., and Wilkenskjeld, S.: Controls on fire activity over the Holocene, Clim. Past, 11, 781–788, https://doi.org/10.5194/cp-11-781-2015, 2015.
Knorr, W., Kaminski, T., Arneth, A., and Weber, U.: Impact of human population density on fire frequency at the global scale, Biogeosciences, 11, 1085–1102, https://doi.org/10.5194/bg-11-1085-2014, 2014.
Leverkus, A. B., Murillo, P. G., Dona, V. J., and Pausas, J. G.: Wildfire: opportunity for restoration?, Science, 363, 134–135, https://doi.org/10.1126/science.aaw2134, 2019.
Liu, Z., Otto-Bliesner, B. L., He, F., Brady, E. C., Tomas, R., Clark, P. U., Carlson, A. E., Lynch-Stieglitz, J., Curry, W., Brook, E., Erickson, D., Jacob, R., Kutzbach, J., and Cheng, J.: Transient Simulation of Last Deglaciation with a New Mechanism for Bølling-Allerød Warming, Science, 325, 310–314, https://doi.org/10.1126/science.1171041, 2009.
Marcisz, K., Gałka, M., Pietrala, P., Miotk-Szpiganowicz, G., Obremska, M., Tobolski, K., and Lamentowicz, M.: Fire activity and hydrological dynamics in the past 5700 years reconstructed from Sphagnum peatlands along the oceanic–continental climatic gradient in northern Poland, Quaternary Sci. Rev., 177, 145–157, https://doi.org/10.1016/j.quascirev.2017.10.018, 2017.
Marlon, J. R., Kelly, R., Daniau, A.-L., Vannière, B., Power, M. J., Bartlein, P., Higuera, P., Blarquez, O., Brewer, S., Brücher, T., Feurdean, A., Romera, G. G., Iglesias, V., Maezumi, S. Y., Magi, B., Courtney Mustaphi, C. J., and Zhihai, T.: Reconstructions of biomass burning from sediment-charcoal records to improve data–model comparisons, Biogeosciences, 13, 3225–3244, https://doi.org/10.5194/bg-13-3225-2016, 2016.
Marquer, L., Gaillard, M. J., Sugita, S., Poska, A., Trondman, A. K., Mazier, F., Nielsen, A. B., Fyfe, R. M., Jönsson, A. M., Smith, B., Kaplan, J. O., Alenius, T., Birks, H. J. B., Bjune, A. E., Christiansen, J., Dodson, J., Edwards, K. J., Giesecke, T., Herzschuh, U., Kangur, M., Koff, T., Latałowa, M., Lechterbeck, J., Olofsson, J., and Seppä, H.: Quantifying the effects of land use and climate on Holocene vegetation in Europe, Quaternary Sci. Rev., 171, 20–37, https://doi.org/10.1016/j.quascirev.2017.07.001, 2017.
Metzger, M. J., Bunce, R. G. H., Jongman, R. H. G., Mücher, C. A., and Watkins, J. W.: A climatic stratification of the environment of Europe, Global Ecol. Biogeogr., 14, 549–563, https://doi.org/10.1111/j.1466-822X.2005.00190.x, 2005.
Molinari, C., Lehsten, V., Blarquez, O., Carcaillet, C., Davis, B. A., Kaplan, J. O., Clear, J., and Bradshaw, R. H.: The climate, the fuel and the land use: Long-term regional variability of biomass burning in boreal forests, Glob. Change Biol., 24, 4929–4945, https://doi.org/10.1111/gcb.14380, 2018.
Pausas, J. G. and Paula, S.: Fuel shapes the fire–climate relationship: evidence from Mediterranean ecosystems, Global Ecol. Biogeogr., 21, 1074–82, https://doi.org/10.1111/j.1466-8238.2012.00769.x, 2012.
Pausas, J. G. and Ribeiro, E.: The global fire–productivity relationship. Global Ecol. Biogeogr., 22, 728–736, https://doi.org/10.1111/geb.12043, 2013.
Pfeiffer, M., Spessa, A., and Kaplan, J. O.: A model for global biomass burning in preindustrial time: LPJ-LMfire (v1.0), Geosci. Model Dev., 6, 643–685, https://doi.org/10.5194/gmd-6-643-2013, 2013.
Pidwirny, M.: Actual and Potential Evapotranspiration, Fundamentals of Physical Geography, 2nd Edn., University of British Columbia, Okanagan, Canada, 2006.
Power, M. J., Marlon, J., Ortiz, N., Bartlein, P. J., Harrison, S. P., Mayle, F. E., Ballouche, A., Bradshaw, R. H. W., Carcaillet C., Cordova, C., Mooney, S., Moreno, P. I., Prentice, I. C., Thonicke, K., Tinner, W., Whitlock, C., Zhang, Y., Zhao, Y., Ali, A. A., Anderson, R. S., Beer, R., Behling, H., Briles, C., Brown, K. J., Brunelle, A., Bush, M., Camill, P., Chu, G. Q., Clark, J., Colombaroli, D., Connor, S., Daniau, A. L., Daniels, M., Dodson, J., Doughty, E., Edwards, M. E., Finsinger, W., Foster, D., Frechette, J., Gaillard, M. J., Gavin, D. G., Gobet, E., Haberle, S., Hallett, D. J., Higuera, P., Hope, G., Horn, S., Inoue, J., Kaltenrieder, P., Kennedy, L., Kong, Z. C., Larsen, C., Long, C. J., Lynch, J., Lynch, E. A., McGlone, M., Meeks, S., Mensing, S., Meyer, G., Minckley, T., Mohr, J., Nelson, D.M., New, J., Newnham, R., Noti, R., Oswald, W., Pierce, J., Richard, P. J. H., Rowe, C., Sanchez Goñi, M. F., Shuman, B. N., Takahara, H., Toney, J., Turney, C., Urrego-Sanchez, D. H., Umbanhowar, C., Vandergoes, M., Vanniere, B., Vescovi, E., Walsh, M., Wang, X., Williams, N., Wilmshurst, J., and Zhang, J. H.: Changes in fire regimes since the Last Glacial Maximum: an assessment based on a global synthesis and analysis of charcoal data, Clim. Dynam., 30, 887–907, https://doi.org/10.1007/s00382-007-0334-x, 2008.
Rabin, S. S., Melton, J. R., Lasslop, G., Bachelet, D., Forrest, M., Hantson, S., Kaplan, J. O., Li, F., Mangeon, S., Ward, D. S., Yue, C., Arora, V. K., Hickler, T., Kloster, S., Knorr, W., Nieradzik, L., Spessa, A., Folberth, G. A., Sheehan, T., Voulgarakis, A., Kelley, D. I., Prentice, I. C., Sitch, S., Harrison, S., and Arneth, A.: The Fire Modeling Intercomparison Project (FireMIP), phase 1: experimental and analytical protocols with detailed model descriptions, Geosci. Model Dev., 10, 1175–1197, https://doi.org/10.5194/gmd-10-1175-2017, 2017.
Rius, D., Vannière, B., Galop, D., and Richard, H.: Holocene fire regime changes from multiple-site sedimentary charcoal analyses in the Lourdes basin (Pyrenees, France), Quaternary Sci. Rev., 30, 1696–709, https://doi.org/10.1016/j.quascirev.2011.03.014, 2011.
Roberts, N., Fyfe, R. M., Woodbridge, J., Gaillard, M. J., Davis, B. A., Kaplan, J. O., Marquer, L., Mazier, F., Nielsen, A. B., Sugita, S., and Trondman, A. K.: Europe's lost forests: a pollen-based synthesis for the last 11,000 years, Sci. Rep.-UK, 158, 716, https://doi.org/10.1038/s41598-017-18646-7, 2018.
Rogers, B. M., Soja, A. J., Goulden, M. L., and Randerson, J. T.: Influence of tree species on continental differences in boreal fires and climate feedbacks, Nat. Geosci., 8, 228–234, https://doi.org/10.1038/ngeo2352, 2015.
Rösch, M., Kleinmann, A., Lechterbeck, J., and Wick, L.: Botanical off-site and on-site data as indicators of different land use systems: a discussion with examples from Southwest Germany, Veg. Hist. Archaeobot., 23, 121–133, https://doi.org/10.1007/s00334-014-0437-3, 2014.
Ryan, K. C.: Dynamic interactions between forest structure and fire behavior in boreal ecosystems, Silva Fenn., 36, 13–39, https://doi.org/10.14214/sf.548, 2002.
Scheffer, M., Hirota, M., Holmgren, M., Van Nes, E. H., and Chapin III, F. S.: Thresholds for Boreal Biome Transitions, P. Natl. Acad. Sci. USA, 109, 21384–21389, https://doi.org/10.1073/pnas.1219844110, 2012
Scheiter, S., Higgins, S. I., Osborne, C. P., Bradshaw, C., Lunt, D., Ripley, B. S., Taylor, L. L., and Beerling, D. J.: Fire and fire-adapted vegetation promoted C4 expansion in the late Miocene, New Phytol., 195, 653–666, https://doi.org/10.1111/j.1469-8137.2012.04202.x, 2012.
Simpson, G. L.: Modelling palaeoecological time series using generalized additive models, Frontiers in Ecology and Evolution, 6, 149, https://doi.org/10.3389/fevo.2018.00149, 2018.
Słowiński, M., Lamentowicz, M., Łuców, D., Barabach, J., Brykała, D., Tyszkowski, S., Pieńczewska, A., Śnieszko, Z., Dietze, E., Jażdżewski, K., Obremska, M., Ott, F., Brauer, A., and Marcisz, K.: Paleoecological and historical data as an important tool in ecosystem management, J. Environ. Manage., 236, 755–768, https://doi.org/10.1016/j.jenvman.2019.02.002, 2019.
Sturtevant, B. R., Miranda, B. R., Yang, J., He, H. S., Gustafson, E. J., and Scheller, R. M.: Studying Fire Mitigation Strategies in Multi-Ownership Landscapes: Balancing the Management of Fire-Dependent Ecosystems and Fire Risk, Ecosystems, 12, 445, https://doi.org/10.1007/s10021-009-9234-8, 2009.
Teuling, A. J., Taylor, C. M., Meirink, J. F., Melsen, L. A., Miralles, D. G., Van Heerwaarden, C. C., Vautard, R., Stegehuis, A. I., Nabuurs, G. J., and de Arellano, J. V. G.: Observational evidence for cloud cover enhancement over western European forests, Nat. Commun., 8, 14065, https://doi.org/10.1038/ncomms14065, 2017.
Thornthwaite, C. W.: An approach toward a rational classification of climate, Geogr. Rev., 38, 55–94, 1948.
Tóth, M., Magyari, E. K., Buczkó, K., Braun, M., Panagiotopoulos, K., and Heiri, O.: Chironomid-inferred Holocene temperature changes in the South Carpathians (Romania), Holocene, 25, 569–582, https://doi.org/10.1177/0959683614565953, 2015.
van der Werf, G. R., Randerson, J. T., Giglio, L., Collatz, G. J., Mu, M., Kasibhatla, P. S., Morton, D. C., DeFries, R. S., Jin, Y., and van Leeuwen, T. T.: Global fire emissions and the contribution of deforestation, savanna, forest, agricultural, and peat fires (1997–2009), Atmos. Chem. Phys., 10, 11707–11735, https://doi.org/10.5194/acp-10-11707-2010, 2010.
van Nes, E. H., Staal, A., Hantson, S., Holmgren, M., Pueyo, S., and Bernardi, R. E.: Fire forbids fifty-fifty forest, PLoS ONE, 13, e0191027, https://doi.org/10.1371/journal.pone.0191027, 2018.
Vannière, B., Blarquez, O., Rius, D., Doyen, E., Brücher, T., Colombaroli, D., Connor, S., Feurdean, A., Hickler, T., Kaltenrieder, P., Lemmen, C., Leys, B., Massa, C., and Olofsson, J.: 7000-year human legacy of elevation-dependent European fire regimes, Quaternary Sci. Rev., 132, 206–212, https://doi.org/10.1016/j.quascirev.2015.11.012, 2016.
Veski, S, Seppä, H., Stančikaitė, M., Zernitskaya, V., Reitalu, T., Gryguc, G., Heinsalu, A., Stivrins, N., Amon, L., Vassiljev, J., and Heiri, O.: Quantitative summer and winter temperature reconstructions from pollen and chironomid data between 15 and 8 ka BP in the Baltic-Belarus area, Quaternary Int., 388, 4–11, https://doi.org/10.1016/j.quaint.2014.10.059, 2015.
Wagenmakers, E. J. and Farrell, S.: AIC model selection using Akaike weights, Psychon. B. Rev., 11, 192–196, 2004.
Whitlock, C. and Larsen, C.: Charcoal as a fire proxy, in: Tracking environmental change using lake sediments, edited by: Smol, J. P., Birks, H. J. B., and Last, W. M., Vol. 3: terrestrial, algal, and siliceous indicators, Kluwer Academic Publishers, 75–97, https://doi.org/10.1007/0-306-47668-1, 2001.
Whitlock, C., Colombaroli, D., Conedera, M., and Tinner, W.: Land-use history as a guide for forest conservation and management, Conserv. Biol., 32, 84–97, https://doi.org/10.1111/cobi.12960, 2017.
Wood, S. N.: Generalized Additive Models: An Introduction with R, 2nd Edn., Taylor and Francis Group, Chapman and Hall/CRC, https://doi.org/10.1201/9781315370279, 2017.