the Creative Commons Attribution 4.0 License.
the Creative Commons Attribution 4.0 License.
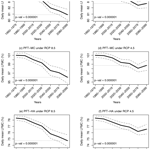
Assessing climate change impacts on live fuel moisture and wildfire risk using a hydrodynamic vegetation model
Wu Ma
Lu Zhai
Alexandria Pivovaroff
Jacquelyn Shuman
Polly Buotte
Junyan Ding
Bradley Christoffersen
Ryan Knox
Max Moritz
Rosie A. Fisher
Charles D. Koven
Lara Kueppers
Chonggang Xu
Live fuel moisture content (LFMC) plays a critical role in wildfire dynamics, but little is known about responses of LFMC to multivariate climate change, e.g., warming temperature, CO2 fertilization, and altered precipitation patterns, leading to a limited prediction ability of future wildfire risks. Here, we use a hydrodynamic demographic vegetation model to estimate LFMC dynamics of chaparral shrubs, a dominant vegetation type in fire-prone southern California. We parameterize the model based on observed shrub allometry and hydraulic traits and evaluate the model's accuracy through comparisons between observed and simulated LFMC of three plant functional types (PFTs) under current climate conditions. Moreover, we estimate the number of days per year of LFMC below 79 % (which is a critical threshold for wildfire danger rating of southern California chaparral shrubs) from 1960 to 2099 for each PFT and compare the number of days below the threshold for medium and high greenhouse gas emission scenarios (RCP4.5 and 8.5). We find that climate change could lead to more days per year (5.2 %–14.8 % increase) with LFMC below 79 % between the historical (1960–1999) and future (2080–2099) periods, implying an increase in wildfire danger for chaparral shrubs in southern California. Under the high greenhouse gas emission scenario during the dry season, we find that the future LFMC reductions mainly result from a warming temperature, which leads to 9.1 %–18.6 % reduction in LFMC. Lower precipitation in the spring leads to a 6.3 %–8.1 % reduction in LFMC. The combined impacts of warming and precipitation change on fire season length are equal to the additive impacts of warming and precipitation change individually. Our results show that the CO2 fertilization will mitigate fire risk by causing a 3.5 %–4.8 % increase in LFMC. Our results suggest that multivariate climate change could cause a significant net reduction in LFMC and thus exacerbate future wildfire danger in chaparral shrub systems.
- Article
(1968 KB) - Full-text XML
-
Supplement
(1242 KB) - BibTeX
- EndNote
Historical warming and changes in precipitation have already impacted wildfires at a global scale (e.g., Stocks et al., 1998; Gillett et al., 2004; Westerling et al., 2003, 2006) and it is expected that accelerating future warming will continue to substantially affect global wildfires (e.g., Flannigan et al., 2009; Liu et al., 2010; Moritz et al., 2012). So far, prior studies have mainly focused on the impacts of dead fuel moisture, fuel loads, and weather conditions on wildfires. Limited studies have applied proxies of live fuel moisture in global fire models. For example, dead fuel moisture is found to be related to fire ignition and fire spread potential (or potential area burned) (Aguado et al., 2007), specific weather conditions such as increased vapor pressure deficit (Williams et al., 2019) can lead to a vast increase in fire activity (Goss et al., 2020), and wildfire fuel loads are projected to increase under climate change (Matthews et al., 2012; Clarke et al., 2016). In global fire models, studies have used proxies of live fuel moisture (Bistinas et al., 2014; Kelley et al., 2019) as well as explicit representation of live fuels (Hantson et al., 2016; Rabin et al., 2017). While previous studies provide great insights into fire risks with changes in climate, dead fuel moisture, fuel loads, and representation of live fuel moisture, there is still limited understanding of how climate change influences live fuel moisture content (LFMC) and the consequent wildfire risks. This is particularly true for the combined impacts of warming temperature, altered precipitation, and increasing CO2 fertilization (Chuvieco et al., 2004; Pellizzaro et al., 2007; Caccamo et al., 2012a, b; Williams et al., 2019; Goss et al., 2020).
A measure of water content within living plant tissue in relation to their dry weight, LFMC has been found to be one of the most critical factors influencing combustion, fire spread, and fire consumption (e.g., Agee et al., 2002; Zarco-Tejada et al., 2003; Bilgili and Saglam, 2003; Yebra et al., 2008; Dennison et al., 2008; Anderson and Anderson, 2010; Keeley et al., 2011). This is because a low LFMC leads to increased flammability and a higher likelihood of ignition (Dimitrakopoulos and Papaioannou, 2001). For instance, LFMC was found to be a significant factor contributing to the occurrence of wildfires in Australia (Plucinski, 2003; Nolan et al., 2016; Yebra et al., 2018; Rossa and Fernandes, 2018; Pimont et al., 2019), Spain (Chuvieco et al., 2009), and California (Santa Monica Mountains; Dennison et al., 2008; Dennison and Moritz, 2009; Pivovaroff et al., 2019). Dennison and Moritz (2009) found strong evidence of a LFMC threshold (79 %) for southern California chaparral shrubs, which may determine when large fires can occur in this region.
Vegetation moisture content is dependent on both ecophysiological characteristics of the species and environmental conditions, including both climatic variables and soil water availability (Rothermel, 1972; Castro et al., 2003; Pellizzaro et al., 2007; Pivovaroff et al., 2019; Nolan et al., 2020). So far, little is known about the relative importance of different climate variables to future LFMC dynamics. On one hand, warming could contribute to a higher atmospheric demand and higher evapotranspiration (Rind et al., 1990) and thus lead to a lower LFMC. On the other hand, higher CO2 concentration will decrease stomatal conductance (Wullschleger et al., 2002) and plant water loss and thus lead to a higher LFMC. The impacts of CO2 and warming could be complicated by local changes in precipitation patterns and humidity (Mikkelsen et al., 2008).
The sensitivity of LFMC to climate change is likely to be affected by plant hydraulic traits (the plant properties that regulate water transport and storage within plant tissues), which affect plant water regulation (Wu et al., 2020). Variations in hydraulic traits reflect contrasting plant drought adaptation strategies when responding to dry conditions. Two contrasting overall strategies are: (1) water stress avoiders and (2) water stress tolerators (Tobin et al., 1999; Wei et al., 2019). The “avoiders” are generally characterized by a more conservative hydraulic strategy under water stress by closing their stomata early, dropping leaves, or accessing deep water to avoid more negative water potentials and therefore xylem cavitation. Meanwhile, the “tolerators” typically build xylem and leaves that are more resistant to cavitation so that they can tolerate more negative water potential and continue to conduct photosynthesis under water stress. Therefore, compared with the tolerators, the avoiders normally have a lower sapwood density and higher plant water storage capacity in their tissues to avoid cavitation (Meinzer et al., 2003, 2009; Pineda-Garcia et al., 2013). Because the avoiders rely on water storage capacity as one way to avoid cavitation, thereby maintaining a relatively high LFMC, and because water loss from storage should increase with warming, LFMC could be more sensitive to climate change in avoiders relative to tolerators.
While over half of terrestrial landscapes on Earth are considered fire-prone (Krawchuk et al., 2009), Mediterranean-type climate regions are routinely impacted by fire, often on an annual basis. This is partly because Mediterranean climate regions are characterized by winter rains followed by an annual dry season, when little to no rainfall occurs for several months. Multiday periods of extreme high temperatures as well as katabatic hot, dry, and intense winds often punctuate the annual drought, leading to some of the worst fire weather in the world (Schroeder et al., 1964). This can result in wildfires that are large, high intensity, and stand replacing (Keeley, 1995; Keeley and Zedler, 2009; Balch et al., 2017). Globally, Mediterranean climate regions are characterized by evergreen sclerophyllous-leaved shrublands. The Mediterranean climate region in California is dominated by chaparral, which is adapted to the periodic fire regime in California (Venturas et al., 2016). Previous studies have proposed a variety of relationships between chaparral LFMC and fire danger in southern California (Dennison et al., 2008; Dennison and Moritz, 2009), but less is known about how climate changes could alter LFMC and fire danger. In chaparral, LFMC is usually high during the winter and spring (wet season) and then gradually declines during the dry season (summer and fall), which leads to a typical fire season approximately six months long in southern California (Pivovaroff et al., 2019). One key risk is that severe drought conditions are becoming exacerbated under climate change, which might lead to the occurrence of larger and higher-intensity fires in chaparral (Dennison et al., 2008; Dennison and Moritz, 2009).
There is a long history of wildfire modeling, with three types of models: (1) fine-scale fire behavior models (e.g., FIRETEC by Linn et al., 2002), (2) landscape-scale fire disturbance models (e.g., LANDIS-II by Sturtevant et al., 2009), and (3) global-scale fire dynamics models (e.g., Hantson et al., 2016; Rabin et al., 2017; SPITFIRE by Thonicke et al., 2010). While these models focus on simulation at different scales, fire measures of the simulation are mainly calculated from climate and dead fuel moisture and currently lack prediction of LFMC dynamics. One key limitation is that most previous models have not yet considered plant hydrodynamics (Holm et al., 2012; Xu et al., 2013; Seiler et al., 2014), which is integral to LFMC prediction. Recently, there have been important improvements to global dynamic and demographic vegetation models by incorporating plant hydrodynamics (McDowell et al., 2013; Xu et al., 2016; Fisher et al., 2018; Mencuccini et al., 2019). These models have been used to study the interaction between elevated CO2 and drought (Duursma and Medlyn, 2012), the impact of hydraulic traits on plant drought response (Christofferson et al., 2016), the role of hydraulic diversity in vegetation response to drought (Xu et al., 2016) and hydroclimate change (Powell et al., 2018), and vegetation water stress and root water uptake (Kennedy et al., 2019). While the main purpose of the new hydraulic components is to improve the vegetation response to drought, the fact that hydrodynamic models consider tissue water content as a prognostic variable provides an opportunity to assess the climate impacts on LFMC.
The objective of this study is to quantify LFMC dynamics and associated changes in fire season duration for a chaparral ecosystem in southern California under climate change using a vegetation demographic model (that resolves the size and age-since-disturbance structure of plant populations) (Xu et al., 2016; Fisher et al., 2018) that incorporates plant hydraulics. We test one overarching hypothesis: future climate change will decrease LFMC and consequently result in a longer fire season as determined by a critical threshold of LFMC (H0). Specifically, we test the following four sub-hypotheses: (1) warming has a stronger impact on LFMC than CO2 fertilization (H1), (2) the reductions in spring and autumn precipitation lead to a longer fire season as determined by LFMC (H2), (3) the combined impacts of warming and precipitation on fire season length are equal to the additive impacts of warming and precipitation change individually (H3), and (4) LFMC for plants with more conservative hydraulic strategies (“avoiders”) will be more vulnerable to warming (H4).
To understand climate change impacts on LFMC for the chaparral ecosystem, we applied the Functionally Assembled Terrestrial Simulator (FATES; Fisher et al., 2015; Massoud et al., 2019; Koven et al., 2020) coupled with a hydrodynamic vegetation module (FATES-HYDRO; Christoffersen et al., 2016) in the Santa Monica Mountains in California. We validated the model using the observed LFMC for three chaparral shrub plant functional types (PFTs). Then, we applied FATES-HYDRO to estimate long-term dynamics of leaf water content (LWC) during 1960–2099 for each PFT using downscaled Earth system model (ESM) climate scenarios. We converted simulated LWC to LFMC within leaves and shoots. Based on the simulated LFMC, we evaluated wildfire danger based on the number of days per year of LFMC below the critical value of 79 % from 1960 to 2099 for each PFT under RCP4.5 and 8.5. Finally, we assessed the relative importance of changes in individual and combined climate variables including CO2, temperature, and precipitation and tested the corresponding hypotheses.
2.1 Study site
The study site is located at the Stunt Ranch Santa Monica Mountains Reserve in the Santa Monica Mountains in California, USA (34∘05′ N, 118∘39′ W). Stunt Ranch is dominated by chaparral vegetation with an elevation of approximately 350 m, a west-facing slope, and a Mediterranean-type climate. The study site harbors an abundance of fauna, particularly birds and reptiles. The mean annual temperature is 18.1 ∘C. The mean annual precipitation is 478 mm, occurring mostly during the wet season (i.e., November–March) with almost no rainfall during the dry season (i.e., April–October). Stunt Ranch last burned in 1993. We focused on PFTs representing 11 study species (Fig. 1), including chamise (Adenostoma fasciculatum – Af), red shank (Adenostoma sparsifolium – As), big berry manzanita (Arctostaphylos glauca – Ag), buck brush (Ceanothus cuneatus – Cc), greenbark ceanothus (Ceanothus spinosus – Cs), mountain mahogany (Cercocarpus betuloides – Cb), toyon (Heteromeles arbutifolia – Ha), laurel sumac (Malosma laurina – Ml), scrub oak (Quercus berberidifolia – Qb), hollyleaf redberry (Rhamnus ilicifolia – Ri), and sugar bush (Rhus ovata – Ro). Detailed information about the study site and species characterizations found at Stunt Ranch can be found in Venturas et al. (2016) and Pivovaroff et al. (2019).
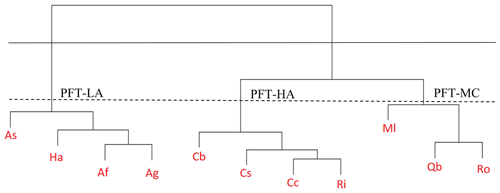
Figure 1Hierarchical cluster analysis of allometry and hydraulic traits for 11 chaparral shrub species used to define three plant functional types at Stunt Ranch. The plant functional types with a low productivity and an aggressive drought tolerance hydraulic strategy (PFT-LA) were defined based on traits of red shank (Adenostoma sparsifolium – As), toyon (Heteromeles arbutifolia – Ha), chamise (Adenostoma fasciculatum – Af), and big berry manzanita (Arctostaphylos glauca – Ag). The plant functional types with a high productivity and an aggressive drought tolerance hydraulic strategy (PFT-HA) were defined based on traits of mountain mahogany (Cercocarpus betuloides – Cb), greenbark ceanothus (Ceanothus spinosus – Cs), buck brush (Ceanothus cuneatus – Cc), and hollyleaf redberry (Rhamnus ilicifolia – Ri). The plant functional types with a medium productivity and an conservative drought tolerance hydraulic strategy (PFT-MC) were defined based on traits of laurel sumac (Malosma laurina – Ml), scrub oak (Quercus berberidifolia – Qb), and sugar bush (Rhus ovata – Ro).
2.2 FATES-HYDRO model
FATES is a vegetation demographic model (Fisher et al., 2015) that uses a size-structured group of plants (cohorts) and successional trajectory-based patches based on the ecosystem demography approach (Moorcroft et al., 2001). FATES simulates the demographic process including seed production, seed emergence, growth, and mortality (Koven et al., 2020). Because the main purpose is to assess LFMC, we controlled for variation in plant size structure that could arise from plant traits or climate differences between model runs by using a reduced-complexity configuration of the model where growth and mortality are turned off and ecosystem structure is held constant. FATES has to be hosted by a land surface model to simulate the soil hydrology, canopy temperature, and transpiration. These host land models include the Exascale Energy Earth System Model (E3SM, Caldwell et al., 2019) land model (ELM) as well as the Community Earth System Model (Fisher et al., 2015) and the Norwegian Earth System Model (NorESM, Tjiputra et al., 2013). In this study, we used the DOE-sponsored ELM as our host land model. The time step of FATES to calculate carbon and water fluxes is 30 min and it can downscale the data from 6-hourly climate drivers.
A key component of FATES, the plant hydrodynamic model (HYDRO, based on Christoffersen et al., 2016), simulates the water flow from soil through the roots, stem, and leaves to the atmosphere. In this model, water flow is calculated based on water pressure gradients across different plant compartments (leaf, stem, transporting roots, absorbing roots, and rhizosphere). Specifically, flow between compartment i and i+1 (Qi) is given by
where Ki is the total conductance (kg MPa−1 s−1) at the boundary of compartments i and i+1 and Δhi is the total water potential difference between the compartments:
where zi is compartment distance above (+) or below (−) the soil surface (m), ρw is the density of water (103 kg m−3), g is acceleration due to gravity (9.8 m s−2), and ψi is tissue or soil matric water potential (MPa). Ki is treated here as the product of a maximum boundary conductance between compartments i and i+1 () and the fractional maximum hydraulic conductance of the adjacent compartments (FMCi or FMCi+1), which is a function of the tissue water content. A key parameter that controls FMC is the critical water potential (P50) that leads to 50 % loss of hydraulic conductivity. The tissue water potential is calculated based on pressure–volume (PV) theory (Tyree and Hammel, 1972; Tyree and Yang, 1990; Bartlett et al., 2012). For leaves, it is described by three phases: (1) capillary water phase with full turgor, (2) elastic drainage phase before reaching turgor loss point, and (3) post-turgor loss phase. For other tissues, it only has phases 2 and 3. Compared to a non-hydrodynamic model, this formulation allows the simulation of plant water transport limitation on transpiration. For the non-hydrodynamic version of FATES, the water limitation factor for transpiration (Btran) is calculated based on the soil moisture potential (Fisher et al., 2015). For the hydrodynamic version, Btran is calculated based on the leaf water potential (ψl) (Christoffersen et al., 2016) as follows:
where P50_gsis the leaf water potential that leads to 50 % loss of stomatal conductance and al is the shape parameter. Please refer to Christoffersen, et al. (2016) for details of formulations of FMC for different plant tissues.
2.3 Allometry and trait data for model parameterization
FATES-HYDRO has a large number of parameters (> 80; see Massoud et al., 2019 for a complete list except for hydraulic parameters). Based on a previous sensitivity analysis study (Massoud et al., 2019), we focused our parameter estimation efforts on the most influential parameters for allometry, leaf and wood traits, and hydraulic traits from observations of 11 chaparral shrub species (see Table S2 in the Supplement) collected from Jacobsen et al. (2008) and Venturas et al. (2016). For this study, we assumed that the allometry of a shrub is analogous to that of a small tree. However, we did make several important modifications to accommodate the allometry of a shrub as their height and crown area relationships to diameter could be different from trees. First, instead of using the diameter at breast height as the basis for allometry to calculate the height, crown area and leaf biomass, we used the basal diameter as the basis for shrubs. Second, in the allometry of trees, the diameter for maximum height (d1: Fates_allom_dbh_maxheight; Table S1) is the same as the diameter for maximum crown area (d2: Fates_allom_d2ca_max; Table S1). As our data showed that d1 and d2 are different for shrubs, we have modified the codes so that d1 and d2 can be set for different values. It is possible that different branching and path length patterns for stems of chaparral species could impact the hydraulics compared to trees; however, FATES-HYDRO treats all the aboveground xylem as a single pool and thus it should not affect our model simulation results.
Based on a hierarchical cluster analysis (Bridges, 1966) of allometry and trait data, there is a clear separation among the shrub species. First, the dendrogram is built and every data point finally merges into a single cluster with the height shown on the y axis. Then we cut the dendrogram in order to create the desired number of clusters determined by a pragmatic choice based on hydraulic traits of 11 chaparral shrub species (Fig. 1). R's rect.hclust function (https://www.rdocumentation.org/packages/stats/versions/3.6.2/topics/rect.hclust, last access: 25 April 2021) was used to see the clusters on the dendrogram. All parameters of allometry, leaf and wood traits, and hydraulic traits were collected from observations shown in Tables S2 and S3. According to the principle of model parsimony, we do not want to classify the species into more than 3 PFTs. Meanwhile, we also want to differentiate the fundamental plant growth and water use strategies that will determine plant transpiration rate and the corresponding LFMC. If we choose to classify the species into two PFTs (based on the solid horizontal line in Fig. 1), then we will not be able to differentiate species with aggressive and conservative hydraulic strategies in the second group and not be able to test H4. Therefore, the chaparral shrub species were classified into three PFTs (based on the dotted horizontal line in Fig. 1 and Table S3) that are able to differentiate plant growth and hydraulic strategy. The three PFTs include a low productivity, aggressive drought tolerance hydraulic strategy PFT (PFT-LA) with a relatively low Vc,max25 (the maximum carboxylation rate at 25 ∘C) and a very negative P50 (the leaf water potential leading to 50 % loss of hydraulic conductivity); a medium productivity, conservative drought tolerance hydraulic strategy PFT (PFT-MC) represented by a medium Vc,max25 and a less negative P50, turgor loss point and water potential at full turgor; and a high productivity, aggressive drought tolerance hydraulic strategy PFT (PFT-HA) with a relatively high Vc,max25 and a very negative P50. The mean of the species-level trait data weighted by species abundance at the site were used to parameterize FATES-HYDRO.
2.4 Model initialization
Our model simulation is transient in terms of soil water content, leaf water content, and carbon and water fluxes. The forest structure (plant sizes and number density) is fixed and is parameterized based on a vegetation inventory from Venturas et al. (2016). The soil texture and depth information are parameterized based on a national soil survey database (https://websoilsurvey.sc.egov.usda.gov/App/WebSoilSurvey.aspx, last access: 28 March 2021; Table S1). The soil moisture is initialized with 50 % of the saturation and the tissue plant water content is initialized so that it is in equilibrium with the soil water potential. We run the model for 10 years based on 1950–1960 climate so that the simulated soil moisture, leaf water content, and carbon and water fluxes are not dependent on their initial conditions.
2.5 Live fuel moisture content for model validation
In this study, we used measured LFMC to validate simulated LFMC. FATES-HYDRO does not directly simulate the LFMC. Thus, we estimated the LFMC based on simulated LWC. The LWC in the model is calculated as follows:
where fw is the fresh weight and dw is the dry weight, which are both simulated within FATES-HYDRO. Then, we estimated the LFMC within leaves and shoots (< 6 mm diameter) using the empirical equation derived from shrub LFMC and LWC data including the three regenerative strategies (seeder (S), resprouter (R), and seeder–resprouter (SR)), in summer, autumn, and winter from Figs. 4 and 5 in the study by Saura-Mas and Lloret (2007) as follows (Fig. S4):
The climate in Saura-Mas and Lloret's study is Mediterranean (northeast Iberian Peninsula), which is consistent with the climate of our study area. LFMC was measured on our site approximately every three weeks, concurrently with plant water potentials in 2015 and 2016. LFMC measurement details can be found in Pivovaroff et al. (2019). For comparison with our model outputs, we calculated the mean LFMC within leaves and shoots for each PFT weighted by the species abundance (Venturas et al., 2016). Species abundance was calculated by dividing mean density of a specific species by the mean density of all species.
2.6 Climate drivers
We forced the FATES-HYDRO model with 6-hourly temperature, precipitation, downward solar radiation, and wind components. Historical climate data during 2012–2019, which were used for FATES-HYDRO calibration, were extracted from a local weather station (https://stuntranch.ucnrs.org/weather-date/, last access: 26 April 2021). Historical and future climate data during 1950–2099, which were used for simulations of LFMC by the FATES-HYDRO model, were downloaded from the Multivariate Adaptive Constructed Analogs (MACA) datasets (Abatzoglou and Brown, 2012; http://maca.northwestknowledge.net, last access: 25 April 2021). The MACA datasets (∘ or approximately 4 km; Abatzoglou and Brown, 2012) include 20 ESMs with historical forcings during 1950–2005 and future Representative Concentration Pathways (RCPs) RCP4.5 and RCP8.5 scenarios during 2006–2099 from the native resolution of the ESMs. The gridded surface meteorological dataset METDATA (Abatzoglou, 2013) were used with high spatial resolution (∘) and daily timescales for near-surface minimum and maximum temperature, precipitation, downward solar radiation, and wind components. Then we downscaled the MACA daily data to 6-hourly based on the temporal anomaly of the observed mean daily data to the hourly data for each day during 2012–2019. The model is driven by yearly CO2 data obtained from Meinshausen et al. (2011).
2.7 Hypothesis testing
To test H0 (future climate change will decrease LFMC and consequently result in a longer fire season as determined by a critical threshold of LFMC), we compared the simulated mean LFMC, derived from modeled leaf water content, under the climate projections from 20 ESMs under RCP4.5 and 8.5. We then tested if the LFMC during the April–October dry season in the historic 960–1999 period is significantly higher than that in the future 2080–2099 period. For the fire season duration, we estimated the number of days per year below a critical threshold of LFMC (79 %). Similarly, we tested if the number of days per year below the critical threshold of LFMC during the historical period are significantly different from that during the future period. We used a bootstrapped approach (Jackson, 1993) to test if the mean of LFMC or fire season duration are significantly different between these two periods. Specifically, we randomly draw 10 000 samples from the simulated residuals of LFMC or fire season durations estimated by 20 ESMs for these two periods under the null hypothesis that there is no difference in the mean. We then calculated p-values by comparing the simulated mean difference to the empirical distribution of difference estimated from these 10 000 samples (see supplementary Sect. 5.2 within Xu et al. (2019) for details).
To test H1 (warming has a stronger impact on LFMC than CO2 fertilization), we compared mean simulated LFMC and fire season length for three PFTs with and without CO2 changes (fixed CO2 at 367 ppm vs. dynamic CO2 concentrations from RCP4.5 or RCP8.5) and warming. To remove the future warming trend, future temperature was replaced with historical (1986–2005) temperature data for every 20 year period. Similarly, to test H2 (the reductions in spring and autumn precipitation lead to a longer fire season as determined by LFMC), we compared the model outputs of LFMC and fire season length for three PFTs with and without precipitation changes. To test H3 (the combined impacts of warming and precipitation on fire season length are equal to the additive impacts of warming and precipitation change individually), we compared model outputs of LFMC and fire season length for three PFTs under three scenarios: (1) without warming, (2) without precipitation changes, and (3) without warming and precipitation changes. Finally, to test H4 (LFMC for plants with more conservative hydraulic strategies will be more vulnerable to warming), we compared model outputs of LFMC and fire season length across the three different PFTs with different hydraulic strategies.
3.1 Comparison between simulated and measured LFMC
Our results showed that FATES-HYDRO was able to capture variation in the LFMC for different PFTs and soil water content at 5 cm depth (Figs. 2 and S3) as well as for chamise in 2018 (Fig. S5), although we had limited observed LFMC data. Specifically, the model was able to capture 96 %, 86 %, and 80 % of the variance in observed LFMC for the 2015–2016 period for three PFTs, respectively (Fig. 2b, d, f). The model was also able to capture the seasonal dynamics of soil water content, LFMC, and LFMC below the threshold of 79 % in comparison to observed data (Figs. 2a, c, e and S3). To validate that FATES-HYDRO is able to capture the interannual variability of LFMC, we compared the simulated LFMC for PFT-LA with the long-term observations of LFMC for the chamise species (Adenostoma fasciculatum; Fig. S5). Our results showed that the model is able to reasonably capture the seasonal and interannual variability for the 2006–2019 period (R2=0.7), although it underestimates peaks in LFMC in 4 of 14 years.
3.2 Changes in the LFMC and fire season length from historical to future periods
Using the validated model driven by climate projections from 20 ESMs under greenhouse gas emission scenarios RCP4.5 and RCP8.5, we found that the daily mean LFMC during the future 2080–2099 period was projected to become significantly lower than that during the historical 1960–1999 period for all three PFTs (Fig. 3; p < 0.000001). Our results also showed that the spread among models increases with time, suggesting a larger uncertainty in the future projection. Specifically, the histogram of daily mean LFMC during the April–October dry season showed that there was a higher probability of low LFMC under future climate conditions (Fig. S1). The daily mean LFMC decreased from 84.7 %, 101.3 %, and 78.4 % during the historical 1960–1999 period to 81.0 %–82.8 %, 96.3 %–98.8 %, and 74.8 %–76.6 % during the future 2080–2099 period under both climate scenarios for PFT-LA, PFT-MC, and PFT-HA, respectively (Fig. 3).
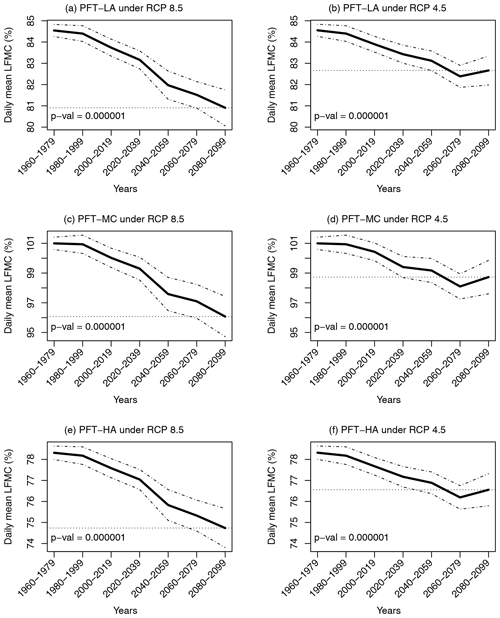
Figure 3Temporal changes in daily mean live fuel moisture content (black solid line) and 95 % confidence interval (black dash-dot line) from 1960 to 2099 for three PFTs (see Fig. 1 for an explanation of the PFTs) under climate scenarios RCP4.5 and 8.5 with 20 Earth system models considering all climatic variables changes. The p values were calculated using bootstrap sampling to test whether the daily mean live fuel moisture content across different models during the future period (2080–2099) was significantly lower than that during the historical period (1960–1999). The gray horizontal dotted line represents the ensemble mean for 2080–2099.
Based on the projected LFMC, there was a significant increase in the fire season length with the critical threshold of LFMC from the historical 1960–1999 period to the future 2080–2099 period for three PFTs. With the critical threshold of 79 % LFMC, the fire season length was projected to increase by 20, 22, and 19 d under RCP8.5 (Fig. 4 and Table S4) and to increase by 9, 11, and 8 d under RCP4.5 (Fig. 4 and Table S4). Our results also showed that the spread among models increases with time, suggesting a larger uncertainty in the future projection. The above results for mean LFMC and fire season length support hypothesis H0 that future climate change will decrease LFMC and consequently result in a longer fire season, as determined by critical thresholds for LFMC for all three PFTs.
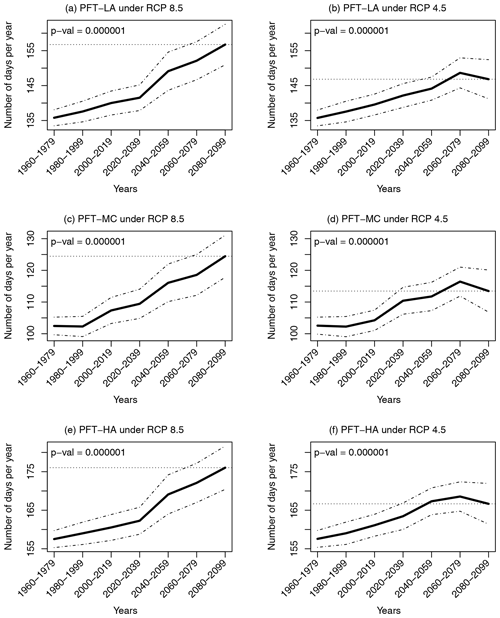
Figure 4Temporal changes in average number of days per year of live fuel moisture content below 79 % (black solid line) and 95 % confidence interval (black dash-dot line) from 1960 to 2099 for three PFTs (see Fig. 1 for an explanation of the PFTs) under climate scenario RCP4.5 and 8.5 with 20 Earth system models considering all climatic variables changes. The p values were calculated using bootstrap sampling to test whether the number of days across different models during the future period (2080–2099) was significantly higher than that during the historical period (1960–1999). The gray horizontal dotted line represents the ensemble mean for 2080–2099.
3.3 Relative effects of individual climate changes on the length of the fire season
In order to better understand the relative contribution to fire season length of different climate variables, we ran FATES-HYDRO for three PFTs using meteorological forcings that isolated and removed changes in individual specific variables. Our results showed that the increase in fire season length mainly resulted from warming, which led to a 16–23 d (9.1 %–18.6 %) per year increase in fire season length for the critical threshold of 79 % LFMC under RCP8.5 (Fig. 5). This is because warming is pushing vapor pressure deficit (VPD) higher, resulting in increased fire season length. For RCP4.5, the warming contributed to a 5–6 d (3.8 %–4.3 %) per year increase in fire season length (Fig. 5). We also found that elevated CO2 concentrations decreased fire season length with a 6–7 d (3.5 %–4.8 %) per year decrease in fire season length under RCP8.5 (Fig. 5). Under RCP4.5, CO2 increases led to a 2–3 d (1.5 %–2.2 %) per year decrease in fire season length (Fig. 5). Because the impact of warming on fire season length was stronger than the mitigation from CO2 enrichment, our results support hypothesis H1 (warming has a stronger impact on LFMC than CO2 fertilization).
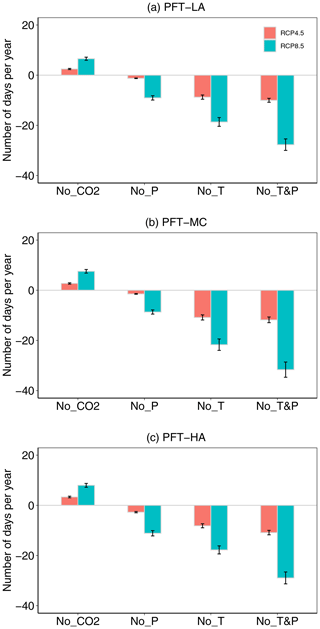
Figure 5Differences in number of days per year of live fuel moisture content below 79 % from 2080 to 2099 for three PFTs (see Fig. 1 for an explanation of the PFTs) under climate scenario RCP4.5 and 8.5 between considering all climatic variable changes and removing the CO2, precipitation, temperature, and precipitation and temperature changes.
Even though total precipitation was projected to increase in the future, lower precipitation in the spring and autumn (Fig. S2a, b) led to an 8–10 d (6.3 %–8.1 %) per year increase in fire season length with the critical threshold of 79 % LFMC under RCP8.5 (Fig. 5). Under RCP4.5, the precipitation changes contributed to a 1–3 d (0.8 %–1.6 %) increase in fire season length (Fig. 5). This result supported hypothesis H2 that the reductions in spring and autumn precipitation lead to a longer fire season as determined by LFMC.
Our results showed that the combined impacts of warming and precipitation on fire season length were equal to the additive impacts of warming and precipitation change individually. This supported hypothesis H3. Specifically, the combined changes in temperature and precipitation caused a 24–33 d yr−1 (15.6 %–26.8 %) increase in fire season length with the critical threshold of 79 % LFMC under RCP8.5 (Fig. 5). Under RCP4.5, the combined changes in temperature and precipitation caused a 6–9 d yr−1 (4.8 %–6.1 %) change in fire season length.
3.4 Comparison of changes in fire season length among three PFTs under climate change
Regarding three PFTs under both climate scenarios, the fire season length for PFT-HA was the longest (167–176 d yr−1), while fire season length for PFT-MC was the shortest (114–124 d yr−1) during 2080–2099 (Fig. 4). However, the response of fire season length to warming was strongest for PFT-MC. Specifically, for PFT-MC, warming under RCP8.5 led to an increase of 21.6 % (22 d) in fire season length (Fig. 5b) and warming under RCP4.5 led to an increase of 10.8 % (11 d) in fire season length. For PFT-LA, warming under RCP8.5 led to an increase of 14.7 % (19 d) in fire season length (Fig. 5a), while warming under RCP4.5 led to an increase of 7.4 % (9 d) in fire season length. Finally, for PFT-HA, warming under RCP8.5 led to an increase of 10.2 % (18 d) in fire season length (Fig. 5c) and 5.3 % (8 d) in fire season length under RCP4.5. Because PFT-MC has a more conservative hydraulic strategy with a less negative P50, turgor loss point and water potential at full turgor, this result supported hypothesis H4 that the LFMC for plants with more conservative hydraulic strategy will be more vulnerable to warming.
To validate our classification scheme, we compared these PFT-level results to those obtained with single-PFT and 2-PFT simulations and found that using the three PFTs defined by our cluster analysis gives a qualitatively different view of LFMC change than a single- or 2-PFT simulation. We found significant differences in the percentage changes of LFMC and fire season length between the future period (2080–2099) and historical period (1960–1999) using three distinct PFTs but no significant differences between PFTs in 2-PFT simulations under the different climate scenarios (Fig. S6).
Low LFMC within shrub leaves and shoots increases the flammability and likelihood of combustion, making it vitally important to monitor temporal variations in LFMC, especially during the dry season (Dennison et al., 2008). The strong relationships between observed and simulated LFMC of all PFTs (Fig. 2) suggested that the plant hydrodynamic model, FATES-HYDRO, could accurately estimate LFMC seasonal dynamics as a function of modeled leaf water content and consequently be useful to predict fire risks in Mediterranean-type climate regions, although only a small amount of validation data were used and the underlying assumption that a shrub was analogous to a small tree was made. Based on the simulated monthly mean LFMC during 2006–2019 for PFT-LA, which includes the chamise species, we found that our model can capture the seasonal variation and interannual variability, but underestimates the highest wet season peaks in LFMC in 4 of 14 years (Fig. S5). While it would not highly affect the long-term trend of LFMC and fire season length, this may cause biases for future projections . During both the future period (2080–2099) and historical period (1960–1999), lower values in the dry season (April–October) were displayed, which is consistent with lower LFMC during the summer–fall dry season, rather than the winter–spring wet season (Chuvieco et al., 2004; Pellizzaro et al., 2007; Pivovaroff et al., 2019). Extremely low daily LFMC was more likely to occur during the future period, which had higher temperature than the historical period. From the historical to the future period, fire season length could increase by 5.2 %–14.8 % under climate change for chaparral shrub ecosystems (H0). The fire season length was not validated; rather, it was defined as the number of days with LFMC below 79 %.
Quantifying influences of climatic variables on LFMC is crucial to predicting future fire risks (Dennison and Moritz, 2009). Our results showed that future warming was the most important driver of LFMC. This finding suggested that warming would substantially push vapor pressure deficit (VPD) higher, decrease LFMC, and strongly increase the fire season length, which may greatly increase fire risks in the future (e.g., Dennison et al., 2008; Chuvieco et al., 2009; Pimont et al., 2019). CO2 fertilization is expected to reduce stomatal conductance (Pataki et al., 2000; Tognetti et al., 2000) and thus could mitigate the impacts of warming on LFMC. Our results illustrated that even though the CO2 impact did cause a 3.5 %–4.8 % reduction in fire season length, the impact of warming on fire season length is about 5.6 %–13.8 % larger than the CO2 effect (H1, warming has a stronger impact on LFMC than CO2 fertilization). This result suggests that CO2 fertilization cannot offset the LFMC impacts from warming. The FATES-HYDRO model assumes a consistent stomatal sensitivity to CO2 concentration across Mediterranean shrub species. While Mediterranean shrub functional types in arid and semi-arid systems would vary in their stomatal response in the real world (Pataki et al., 2000). Therefore, our model may overestimate or underestimate the CO2 effect on stomatal conductance and its mitigating influence might be smaller in reality for some species.
Previous studies implied that the timing of precipitation may have a strong impact on subsequent LFMC (e.g., Veblen et al., 2000; Westerling et al., 2006; Dennison and Moritz, 2009). In this study, precipitation was also a key driver of LFMC under future climate conditions. Our results showed that even though total precipitation was projected to increase, the reduction in spring and autumn precipitation (Fig. S2) was projected to cause a longer fire season length (H2, the reductions in spring and autumn precipitation lead to a longer fire season as determined by LFMC; Fig. 5). This result was in agreement with a prior study indicating that spring precipitation, particularly in the month of March, was found to be the primary driver of timing of LFMC changes (Dennison and Moritz, 2009). We also found that the combined impacts of warming and precipitation on fire season length were equal to the linearly additive impacts of warming and precipitation change individually (H3). Our results suggested that when evaluating future fire risks, it is critical that we consider the seasonal changes in precipitation and its interaction with the warming impact.
Modeled vegetation responses to environmental changes is a function of variation in plant functional traits (Koven et al., 2020). The three PFTs represented in this study have similar patterns in LFMC in response to climate change during 1960–2099, but we did see some critical differences. Specifically, the plant functional type PFT-MC with more conservative hydraulic strategy had the strongest responses to climate change (Fig. 5). This could be related to the fact that the PFT-MC is a more conservative drought tolerant PFT in terms of hydraulic strategy with less negative P50, turgor loss point, and water potential at full turgor. The PFT-MC plants had a relatively high saturated water content based on observed data (Fig. 2) and the water within plant tissues thus changes more quickly in response to the environmental condition changes (H4, LFMC for plants with more conservative hydraulic strategies will be more vulnerable to warming). However, the three different PFTs coexisted at the same location in model simulations, therefore, coexistence and heterogeneity in LFMC might impact fire behavior and fire season length.
Because the moisture content of live fuels (∼ 50 %–200 %) is much higher than that of dead fuels (∼ 7 %–30 %), leaf senescence induced by drought stress and subsequent mortality are potentially vital factors to cause large wildfires (Nolan et al., 2016, 2020). Thus drought-induced canopy dieback and mortality could largely increase surface fine fuel loads and vegetation flammability, which can increase the probability of wildfires (Ruthrof et al., 2016). Since growth and mortality are turned off in model runs by using a reduced-complexity configuration, it is possible that vegetation density might decrease and LFMC could be conserved under future scenarios. In addition, potential vegetation transitions (e.g., shrubs to grassland and species composition changes) might substantially affect flammability and thus fire intensity and frequency. In this study, we used the static mode of FATES-HYDRO to simulate LWC dynamics under climate change. If we need to assess how the leaf senescence and vegetation dynamics will impact the fire behavior, we can use the same model with dynamic mode to assess their impact on fire behavior under future drought and warming conditions.
Application of a hydrodynamic vegetation model to estimate LFMC dynamics could potentially benefit wildfire modeling at the fine scale, landscape scale, and global scale. LFMC is a potentially critical factor influencing fire spread and consumption. Many previous wildfire models focus on the impacts of dead fuel moisture, weather conditions and fuel loads, rather than the representation of live fuel moisture (Anderson and Anderson, 2010; Keeley et al., 2011; Jolly and Johnson, 2018). The implications of including LFMC are that fire potential will change with plant water potential and uptake from soils, photosynthetic and respiratory activity, carbon allocation, and phenology with variability across species over time (Jolly and Johnson, 2018). Therefore, work to incorporate LFMC dynamics in models could play a vitally important role in projections of wildfire behavior and effects under current and future climate.
A hydrodynamic vegetation model, FATES-HYDRO, was used to estimate leaf water status and thus LFMC dynamics of chaparral shrub species in southern California under historical and future conditions. The FATES-HYDRO model was validated using monthly mean LFMC for three PFTs. The fire season length was projected to substantially increase under both climate scenarios from 1960–1999 to 2080–2099. This could increase wildfire risk over time for chaparral shrubs in southern California. Our results showed that temperature was the most important driver of LFMC among all climatic variables. The LFMC estimated by the FATES-HYDRO model offered a baseline of predicting plant hydraulic dynamics subjected to climate change and provided a critical foundation that reductions in LFMC from climate warming may exacerbate future wildfire risk. Longer fire seasons might have a significant impact on overall public health and quality of life in the future.
LFMC measurement data can be found in Pivovaroff et al. (2019). Species abundance data can be found in Venturas et al. (2016). All other data are available within this paper and in the Supplement.
The supplement related to this article is available online at: https://doi.org/10.5194/bg-18-4005-2021-supplement.
WM, LZ and CX were involved in designing the study. WM and CX conducted the model simulations and data analysis in consultation with JS, PB, JD, MM, CDK, and LK. WM and CX wrote the first draft of the manuscript. LZ, AP, JS, PB, JD, BC, RK, MM, RAF, CDK, and LK reviewed and improved the manuscript.
The authors declare that they have no conflict of interest.
Publisher’s note: Copernicus Publications remains neutral with regard to jurisdictional claims in published maps and institutional affiliations.
We thank the editor Martin De Kauwe and the three referees for their help improving this paper.
This project is supported by the University of California Office of the President Lab Fees Research Program and the Next Generation Ecosystem Experiment (NGEE) Tropics, which is supported by the U.S. DOE Office of Science. Charles D. Koven and Junyan Ding are supported by the DOE Office of Science, Regional and Global Model Analysis Program, Early Career Research Program.
This research has been supported by the University of California Office of the President Lab Fees Research Program and the Next Generation Ecosystem Experiment (NGEE) Tropics, which is supported by the U.S. DOE Office of Science; the DOE Office of Science, Regional and Global Model Analysis Program, Early Career Research Program; Los Alamos National Laboratory High Performance Computing; and the U.S.Department of Energy, Office of Science, Office of Biological and Environmental Research.
This paper was edited by Martin De Kauwe and reviewed by Douglas I. Kelley and two anonymous referees.
Abatzoglou, J. T.: Development of gridded surface meteorological data for ecological applications and modelling, Int. J. Climatol., 33, 121–131, https://doi.org/10.1002/joc.3413, 2013.
Abatzoglou, J. T. and Brown, T. J.: A comparison of statistical downscaling methods suited for wildfire applications, Int. J. Climatol., 32, 772–780, https://doi.org/10.1002/joc.2312, 2012.
Agee, J. K., Wright, C. S., Williamson, N., and Huff, M. H.: Foliar moisture content of Pacific Northwest vegetation and its relation to wildland fire behavior, For. Ecol. Manag., 167, 57–66, https://doi.org/10.1016/S0378-1127(01)00690-9, 2002.
Aguado, I., Chuvieco, E., Boren, R., and Nieto, H.: Estimation of dead fuel moisture content from meteorological data in Mediterranean areas. Applications in fire danger assessment, Int. J. Wildland Fire, 16, 390–397, https://doi.org/10.1071/WF06136, 2007.
Anderson, S. A. and Anderson, W. R.: Ignition and fire spread thresholds in gorse (Ulex europaeus), Int. J. Wildland Fire, 19, 589–598, https://doi.org/10.1071/WF09008, 2010.
Balch, J. K., Bradley, B. A., Abatzoglou, J. T., Nagy, R. C., Fusco, E. J., and Mahood, A. L.: Human-started wildfires expand the fire niche across the United States, P. Natl. Acad. Sci. USA, 114, 2946–2951, https://doi.org/10.1073/pnas.1617394114, 2017.
Bartlett, M. K., Scoffoni, C., and Sack, L.: The determinants of leaf turgor loss point and prediction of drought tolerance of species and biomes: a global meta-analysis, Ecol. Lett., 15, 393–405, https://doi.org/10.1111/j.1461-0248.2012.01751.x, 2012.
Bilgili, E. and Saglam, B.: Fire behavior in maquis fuels in Turkey, For. Ecol. Manag., 184, 201–207, https://doi.org/10.1016/S0378-1127(03)00208-1, 2003.
Bistinas, I., Harrison, S. P., Prentice, I. C., and Pereira, J. M. C.: Causal relationships versus emergent patterns in the global controls of fire frequency, Biogeosciences, 11, 5087–5101, https://doi.org/10.5194/bg-11-5087-2014, 2014.
Bridges Jr., C. C.: Hierarchical cluster analysis, Psychol. Rep., 18, 851–854, https://doi.org/10.2466/pr0.1966.18.3.851, 1966.
Caccamo, G., Chisholm, L. A., Bradstock, R. A., and Puotinen, M. L.: Using remotely-sensed fuel connectivity patterns as a tool for fire danger monitoring, Geophys. Res. Lett., 39, L01302, https://doi.org/10.1029/2011GL050125, 2012a.
Caccamo, G., Chisholm, L. A., Bradstock, R. A., Puotinen, M. L., and Pippen, B. G.: Monitoring live fuel moisture content of heathland, shrubland and sclerophyll forest in south-eastern Australia using MODIS data, Int. J. Wildland Fire, 21, 257–269, https://doi.org/10.1071/WF11024, 2012b.
Caldwell, P. M., Mametjanov, A., Tang, Q., Van Roekel, L. P., Golaz, J. C., Lin, W., Bader, D. C., Keen, N. D., Feng, Y., Jacob, R., and Maltrud, M. E.: The DOE E3SM coupled model version 1: Description and results at high resolution, J. Adv. Model. Earth Syst., 11, 4095–4146, https://doi.org/10.1029/2019MS001870, 2019.
Castro, F. X., Tudela, A., and Sebastià, M. T.: Modeling moisture content in shrubs to predict fire risk in Catalonia (Spain), Agric. For. Meteorol., 116, 49–59, https://doi.org/10.1016/S0168-1923(02)00248-4, 2003.
Christoffersen, B. O., Gloor, M., Fauset, S., Fyllas, N. M., Galbraith, D. R., Baker, T. R., Kruijt, B., Rowland, L., Fisher, R. A., Binks, O. J., Sevanto, S., Xu, C., Jansen, S., Choat, B., Mencuccini, M., McDowell, N. G., and Meir, P.: Linking hydraulic traits to tropical forest function in a size-structured and trait-driven model (TFS v.1-Hydro), Geosci. Model Dev., 9, 4227–4255, https://doi.org/10.5194/gmd-9-4227-2016, 2016.
Chuvieco, E., Cocero, D., Riano, D., Martin, P., Martınez-Vega, J., de la Riva, J., and Pérez, F.: Combining NDVI and surface temperature for the estimation of live fuel moisture content in forest fire danger rating, Remote. Sens. Environ., 92, 322–331, https://doi.org/10.1016/j.rse.2004.01.019, 2004.
Chuvieco, E., González, I., Verdú, F., Aguado, I., and Yebra, M.: Prediction of fire occurrence from live fuel moisture content measurements in a Mediterranean ecosystem, Int. J. Wildland Fire, 18, 430–441, https://doi.org/10.1071/WF08020, 2009.
Clarke, H., Pitman, A. J., Kala, J., Carouge, C., Haverd, V., and Evans, J. P.: An investigation of future fuel load and fire weather in Australia, Clim. Change., 139, 591–605, https://doi.org/10.1007/s10584-016-1808-9, 2016.
Dennison, P. E. and Moritz, M. A.: Critical live fuel moisture in chaparral ecosystems: a threshold for fire activity and its relationship to antecedent precipitation, Int. J. Wildland Fire, 18, 1021–1027, https://doi.org/10.1071/WF08055, 2009.
Dennison, P. E., Moritz, M. A., and Taylor, R. S.: Evaluating predictive models of critical live fuel moisture in the Santa Monica Mountains, California, Int. J. Wildland Fire, 17, 18–27, https://doi.org/10.1071/WF07017, 2008.
Dimitrakopoulos, A. P. and Papaioannou, K. K.: Flammability assessment of Mediterranean forest fuels, Fire Technol., 37, 143–152, https://doi.org/10.1023/A:1011641601076, 2001.
Duursma, R. A. and Medlyn, B. E.: MAESPA: a model to study interactions between water limitation, environmental drivers and vegetation function at tree and stand levels, with an example application to [CO2] × drought interactions, Geosci. Model Dev., 5, 919–940, https://doi.org/10.5194/gmd-5-919-2012, 2012.
Fisher, R. A., Muszala, S., Verteinstein, M., Lawrence, P., Xu, C., McDowell, N. G., Knox, R. G., Koven, C., Holm, J., Rogers, B. M., Spessa, A., Lawrence, D., and Bonan, G.: Taking off the training wheels: the properties of a dynamic vegetation model without climate envelopes, CLM4.5(ED), Geosci. Model Dev., 8, 3593–3619, https://doi.org/10.5194/gmd-8-3593-2015, 2015.
Fisher, R. A., Koven, C. D., Anderegg, W. R., Christoffersen, B. O., Dietze, M. C., Farrior, C. E., Holm, J. A., Hurtt, G. C., Knox, R. G., Lawrence, P. J., and Lichstein, J. W.: Vegetation demographics in Earth System Models: A review of progress and priorities, Glob. Change. Biol., 24, 35–54, https://doi.org/10.1111/gcb.13910, 2018.
Flannigan, M. D., Krawchuk, M. A., de Groot, W. J., Wotton, B. M., and Gowman, L. M.: Implications of changing climate for global wildland fire, Int. J. Wildland Fire, 18, 483–507, https://doi.org/10.1071/WF08187, 2009.
Gillett, N. P., Weaver, A. J., Zwiers, F. W., and Flannigan, M. D.: Detecting the effect of climate change on Canadian forest fires, Geophys. Res. Lett., 31, L18211, https://doi.org/10.1029/2004GL020876, 2004.
Goss, M., Swain, D. L., Abatzoglou, J. T., Sarhadi, A., Kolden, C. A., Williams, A. P., and Diffenbaugh, N. S.: Climate change is increasing the likelihood of extreme autumn wildfire conditions across California, Environ. Res. Lett., 15, 094016, https://doi.org/10.1088/1748-9326/ab83a7, 2020.
Hantson, S., Arneth, A., Harrison, S. P., Kelley, D. I., Prentice, I. C., Rabin, S. S., Archibald, S., Mouillot, F., Arnold, S. R., Artaxo, P., Bachelet, D., Ciais, P., Forrest, M., Friedlingstein, P., Hickler, T., Kaplan, J. O., Kloster, S., Knorr, W., Lasslop, G., Li, F., Mangeon, S., Melton, J. R., Meyn, A., Sitch, S., Spessa, A., van der Werf, G. R., Voulgarakis, A., and Yue, C.: The status and challenge of global fire modelling, Biogeosciences, 13, 3359–3375, https://doi.org/10.5194/bg-13-3359-2016, 2016.
Holm, J. A., Shugart, H. H., Van Bloem, S. J., and Larocque, G. R.: Gap model development, validation, and application to succession of secondary subtropical dry forests of Puerto Rico, Ecol. Modell., 233, 70–82, https://doi.org/10.1016/j.ecolmodel.2012.03.014, 2012.
Jackson, D. A.: Stopping rules in principal components analysis: a comparison of heuristical and statistical approaches, Ecology, 74, 2204–2214, https://doi.org/10.2307/1939574, 1993.
Jacobsen, A. L., Pratt, R. B., Davis, S. D., and Ewers, F. W.: Comparative community physiology: nonconvergence in water relations among three semi-arid shrub communities, New Phytol., 180, 100–113, https://doi.org/10.1111/j.1469-8137.2008.02554.x, 2008.
Jolly, W. M. and Johnson, D. M.: Pyro-ecophysiology: shifting the paradigm of live wildland fuel research, Fire, 1, 8, https://doi.org/10.3390/fire1010008, 2018.
Keeley, J. E.: Future of California floristics and systematics: wildfire threats to the California flora, Madrono, 42, 175–179, available at: https://www.jstor.org/stable/41425064 (last access: 25 March 2021), 1995.
Keeley, J. E. and Zedler, P. H.: Large, high-intensity fire events in southern California shrublands: debunking the fine-grain age patch model, Ecol. Appl., 19, 69–94, https://doi.org/10.1890/08-0281.1, 2009.
Keeley, J. E., Bond, W. J., Bradstock, R. A., Pausas, J. G., and Rundel, P. W.: Fire in Mediterranean ecosystems: ecology, evolution and management, Cambridge University Press, https://doi.org/10.4000/mediterranee.6936, 2011.
Kelley, D. I., Bistinas, I., Whitley, R., Burton, C., Marthews, T. R., and Dong, N.: How contemporary bioclimatic and human controls change global fire regimes, Nat. Clim. Change., 9, 690–696, https://doi.org/10.1038/s41558-019-0540-7, 2019.
Kennedy, D., Swenson, S., Oleson, K. W., Lawrence, D. M., Fisher, R., Lola da Costa, A. C., and Gentine, P.: Implementing plant hydraulics in the community land model, version 5, J. Adv. Model. Earth Syst., 11, 485–513, https://doi.org/10.1029/2018MS001500, 2019.
Koven, C. D., Knox, R. G., Fisher, R. A., Chambers, J. Q., Christoffersen, B. O., Davies, S. J., Detto, M., Dietze, M. C., Faybishenko, B., Holm, J., Huang, M., Kovenock, M., Kueppers, L. M., Lemieux, G., Massoud, E., McDowell, N. G., Muller-Landau, H. C., Needham, J. F., Norby, R. J., Powell, T., Rogers, A., Serbin, S. P., Shuman, J. K., Swann, A. L. S., Varadharajan, C., Walker, A. P., Wright, S. J., and Xu, C.: Benchmarking and parameter sensitivity of physiological and vegetation dynamics using the Functionally Assembled Terrestrial Ecosystem Simulator (FATES) at Barro Colorado Island, Panama, Biogeosciences, 17, 3017–3044, https://doi.org/10.5194/bg-17-3017-2020, 2020.
Krawchuk, M. A., Moritz, M. A., Parisien, M. A., Van Dorn, J., and Hayhoe, K.: Global pyrogeography: the current and future distribution of wildfire, Plos One, 4, e5102, https://doi.org/10.1371/journal.pone.0005102, 2009.
Linn, R., Reisner, J., Colman, J. J., and Winterkamp, J.: Studying wildfire behavior using FIRETEC, Int. J. Wildland Fire, 11, 233–246, https://doi.org/10.1071/WF02007, 2002.
Liu, Y., Stanturf, J., and Goodrick, S.: Trends in global wildfire potential in a changing climate, For. Ecol. Manag., 259, 685–697, https://doi.org/10.1016/j.foreco.2009.09.002, 2010.
Massoud, E. C., Xu, C., Fisher, R. A., Knox, R. G., Walker, A. P., Serbin, S. P., Christoffersen, B. O., Holm, J. A., Kueppers, L. M., Ricciuto, D. M., Wei, L., Johnson, D. J., Chambers, J. Q., Koven, C. D., McDowell, N. G., and Vrugt, J. A.: Identification of key parameters controlling demographically structured vegetation dynamics in a land surface model: CLM4.5(FATES), Geosci. Model Dev., 12, 4133–4164, https://doi.org/10.5194/gmd-12-4133-2019, 2019.
Matthews, S., Sullivan, A. L., Watson, P., and Williams, R. J.: Climate change, fuel and fire behaviour in a eucalypt forest, Glob. Change Biol., 18, 3212–3223, https://doi.org/10.1111/j.1365-2486.2012.02768.x, 2012.
McDowell, N. G., Fisher, R. A., Xu, C., Domec, J. C., Hölttä, T., Mackay, D. S., Sperry, J. S., Boutz, A., Dickman, L., Gehres, N., and Limousin, J. M.: Evaluating theories of drought-induced vegetation mortality using a multimodel–experiment framework, New Phytol., 200, 304–321, https://doi.org/10.1111/nph.12465, 2013.
Meinshausen, M., Smith, S. J., Calvin, K., Daniel, J. S., Kainuma, M. L., Lamarque, J. F., Matsumoto, K., Montzka, S. A., Raper, S. C., Riahi, K., and Thomson, A. G.: The RCP greenhouse gas concentrations and their extensions from 1765 to 2300, Clim. Change., 109, 213–241, https://doi.org/10.1007/s10584-011-0156-z, 2011.
Meinzer, F. C., James, S. A., Goldstein, G., and Woodruff, D.: Whole-tree water transport scales with sapwood capacitance in tropical forest canopy trees, Plant Cell Environ., 26, 1147–1155, https://doi.org/10.1046/j.1365-3040.2003.01039.x, 2003.
Meinzer, F. C., Johnson, D. M., Lachenbruch, B., McCulloh, K. A., and Woodruff, D. R.: Xylem hydraulic safety margins in woody plants: coordination of stomatal control of xylem tension with hydraulic capacitance, Funct. Ecol., 23, 922–930, https://doi.org/10.1111/j.1365-2435.2009.01577.x, 2009.
Mencuccini, M., Manzoni, S., and Christoffersen, B.: Modelling water fluxes in plants: from tissues to biosphere, New Phytol., 222, 1207–1222, https://doi.org/10.1111/nph.15681, 2019.
Mikkelsen, T. N., Beier, C., Jonasson, S., Holmstrup, M., Schmidt, I. K., Ambus, P., Pilegaard, K., Michelsen, A., Albert, K., Andresen, L. C., and Arndal, M. F.: Experimental design of multifactor climate change experiments with elevated CO2, warming and drought: the CLIMAITE project, Funct. Ecol., 22, 185–195, https://doi.org/10.1111/j.1365-2435.2007.01362.x, 2008.
Moorcroft, P. R., Hurtt, G. C., and Pacala, S. W.: A method for scaling vegetation dynamics: the ecosystem demography model (ED), Ecol. Monogr., 71, 557–586, https://doi.org/10.1890/0012-9615(2001)071[0557:AMFSVD]2.0.CO;2, 2001.
Moritz, M. A., Parisien, M. A., Batllori, E., Krawchuk, M. A., Van Dorn, J., Ganz, D. J., and Hayhoe, K.: Climate change and disruptions to global fire activity, Ecosphere, 3, 1–22, https://doi.org/10.1890/ES11-00345.1, 2012.
Nolan, R. H., Boer, M. M., Resco de Dios, V., Caccamo, G., and Bradstock, R. A.: Large-scale, dynamic transformations in fuel moisture drive wildfire activity across southeastern Australia, Geophys. Res. Lett., 43, 4229–4238, https://doi.org/10.1002/2016GL068614, 2016.
Nolan, R. H., Blackman, C. J., de Dios, V. R., Choat, B., Medlyn, B. E., Li, X., Bradstock, R. A., and Boer, M. M.: Linking forest flammability and plant vulnerability to drought, Forests, 11, 779, https://doi.org/10.3390/f11070779, 2020.
Pataki, D. E., Huxman, T. E., Jordan, D. N., Zitzer, S. F., Coleman, J. S., Smith, S. D., Nowak, R. S., and Seemann, J. R.: Water use of two Mojave Desert shrubs under elevated CO2, Glob. Change Biol., 6, 889–897, https://doi.org/10.1046/j.1365-2486.2000.00360.x, 2000.
Pellizzaro, G., Cesaraccio, C., Duce, P., Ventura, A., and Zara, P.: Relationships between seasonal patterns of live fuel moisture and meteorological drought indices for Mediterranean shrubland species, Int. J. Wildland Fire, 16, 232–241, https://doi.org/10.1071/WF06081, 2007.
Pimont, F., Ruffault, J., Martin-StPaul, N. K., and Dupuy, J. L.: Why is the effect of live fuel moisture content on fire rate of spread underestimated in field experiments in shrublands?, Int. J. Wildland Fire, 28, 127–137, https://doi.org/10.1071/WF18056, 2019.
Pineda-Garcia, F., Paz, H., and Meinzer, F. C.: Drought resistance in early and late secondary successional species from a tropical dry forest: the interplay between xylem resistance to embolism, sapwood water storage and leaf shedding, Plant Cell Environ., 36, 405–418, https://doi.org/10.1111/j.1365-3040.2012.02582.x, 2013.
Pivovaroff, A. L., Emery, N., Sharifi, M. R., Witter, M., Keeley, J. E., and Rundel, P. W.: The effect of ecophysiological traits on live fuel moisture content, Fire, 2, 28, https://doi.org/10.3390/fire2020028, 2019.
Plucinski, M. P.: The investigation of factors governing ignition and development of fires in heathland vegetation, PhD thesis, University of New South Wales, Sydney, Australia, 2003.
Powell, T. L., Koven, C. D., Johnson, D. J., Faybishenko, B., Fisher, R. A., Knox, R. G., McDowell, N. G., Condit, R., Hubbell, S. P., Wright, S. J., and Chambers, J. Q.: Variation in hydroclimate sustains tropical forest biomass and promotes functional diversity, New Phytol., 219, 932–946, https://doi.org/10.1111/nph.15271, 2018.
Rabin, S. S., Melton, J. R., Lasslop, G., Bachelet, D., Forrest, M., Hantson, S., Kaplan, J. O., Li, F., Mangeon, S., Ward, D. S., Yue, C., Arora, V. K., Hickler, T., Kloster, S., Knorr, W., Nieradzik, L., Spessa, A., Folberth, G. A., Sheehan, T., Voulgarakis, A., Kelley, D. I., Prentice, I. C., Sitch, S., Harrison, S., and Arneth, A.: The Fire Modeling Intercomparison Project (FireMIP), phase 1: experimental and analytical protocols with detailed model descriptions, Geosci. Model Dev., 10, 1175–1197, https://doi.org/10.5194/gmd-10-1175-2017, 2017.
Rind, D., Goldberg, R., Hansen, J., Rosenzweig, C., and Ruedy, R.: Potential evapotranspiration and the likelihood of future drought, J. Geophys. Res. Atmos., 95, 9983–10004, https://doi.org/10.1029/JD095iD07p09983, 1990.
Rossa, C. G. and Fernandes, P. M.: Live fuel moisture content: The “pea under the mattress” of fire spread rate modeling?, Fire, 1, 43, https://doi.org/10.3390/fire1030043, 2018.
Rothermel, R. C.: A mathematical model for predicting fire spread in wildland fuels (Vol. 115), Intermountain Forest & Range Experiment Station, Forest Service, US Department of Agriculture, Minneapolis, USA, 1972.
Ruthrof, K. X., Fontaine, J. B., Matusick, G., Breshears, D. D., Law, D. J., Powell, S., and Hardy, G.: How drought-induced forest die-off alters microclimate and increases fuel loadings and fire potentials, Int. J. Wildland Fire, 25, 819–830, https://doi.org/10.1071/WF15028, 2016.
Saura-Mas, S. and Lloret, F.: Leaf and shoot water content and leaf dry matter content of Mediterranean woody species with different post-fire regenerative strategies, Ann. Bot., 99, 545–554, https://doi.org/10.1093/aob/mcl284, 2007.
Schroeder, M. J., Glovinsky, M., Henricks, V. F., Hood, F. C., and Hull, M. K.: Synoptic weather types associated with critical fire weather, USDA Forest Service, Pacific Southwest Range and Experiment Station, Berkeley, CA, USA, 1964.
Seiler, C., Hutjes, R. W. A., Kruijt, B., Quispe, J., Añez, S., Arora, V. K., Melton, J. R., Hickler, T., and Kabat, P.: Modeling forest dynamics along climate gradients in Bolivia, J. Geophys. Res.-Biogeo., 119, 758–775, https://doi.org/10.1002/2013JG002509, 2014.
Stocks, B. J., Fosberg, M. A., Lynham, T. J., Mearns, L., Wotton, B. M., Yang, Q., Jin, J. Z., Lawrence, K., Hartley, G. R., Mason, J. A., and McKenney, D. W.: Climate change and forest fire potential in Russian and Canadian boreal forests, Clim. Change., 38, 1–13, https://doi.org/10.1023/A:1005306001055, 1998.
Sturtevant, B. R., Scheller, R. M., Miranda, B. R., Shinneman, D., and Syphard, A.: Simulating dynamic and mixed-severity fire regimes: a process-based fire extension for LANDIS-II, Ecol. Modell., 220, 3380–3393, https://doi.org/10.1016/j.ecolmodel.2009.07.030, 2009.
Thonicke, K., Spessa, A., Prentice, I. C., Harrison, S. P., Dong, L., and Carmona-Moreno, C.: The influence of vegetation, fire spread and fire behaviour on biomass burning and trace gas emissions: results from a process-based model, Biogeosciences, 7, 1991–2011, https://doi.org/10.5194/bg-7-1991-2010, 2010.
Tjiputra, J. F., Roelandt, C., Bentsen, M., Lawrence, D. M., Lorentzen, T., Schwinger, J., Seland, Ø., and Heinze, C.: Evaluation of the carbon cycle components in the Norwegian Earth System Model (NorESM), Geosci. Model Dev., 6, 301–325, https://doi.org/10.5194/gmd-6-301-2013, 2013.
Tobin, M. F., Lopez, O. R., and Kursar, T. A.: Responses of Tropical Understory Plants to a Severe Drought: Tolerance and Avoidance of Water Stress, Biotropica, 31, 570–578, https://doi.org/10.1111/j.1744-7429.1999.tb00404.x, 1999.
Tognetti, R., Minnocci, A., Peñuelas, J., Raschi, A., and Jones, M. B.: Comparative field water relations of three Mediterranean shrub species co-occurring at a natural CO2 vent, J. Exp. Bot., 51, 1135–1146, https://doi.org/10.1093/jexbot/51.347.1135, 2000.
Tyree, M. T. and Hammel, H. T.: The measurement of the turgor pressure and the water relations of plants by the pressure-bomb technique, J. Exp. Bot., 23, 267–282, https://doi.org/10.1093/jxb/23.1.267, 1972.
Tyree, M. T. and Yang, S.: Water-storage capacity of Thuja, Tsuga and Acer stems measured by dehydration isotherms, Planta, 182, 420–426, https://doi.org/10.1007/BF02411394, 1990.
Veblen, T. T., Kitzberger, T., and Donnegan, J.: Climatic and human influences on fire regimes in ponderosa pine forests in the Colorado Front Range, Ecol. Appl., 10, 1178–1195, https://doi.org/10.1890/1051-0761(2000)010[1178:CAHIOF]2.0.CO;2, 2000.
Venturas, M. D., MacKinnon, E. D., Dario, H. L., Jacobsen, A. L., Pratt, R. B., and Davis, S. D.: Chaparral shrub hydraulic traits, size, and life history types relate to species mortality during California's historic drought of 2014, Plos One, 11, e0159145, https://doi.org/10.1371/journal.pone.0159145, 2016.
Wei, L., Xu, C., Jansen, S., Zhou, H., Christoffersen, B. O., Pockman, W. T., Middleton, R. S., Marshall, J. D., and McDowell, N. G.: A heuristic classification of woody plants based on contrasting shade and drought strategies, Tree Physiol., 39, 767–781, https://doi.org/10.1093/treephys/tpy146, 2019.
Westerling, A. L., Gershunov, A., Brown, T. J., Cayan, D. R., and Dettinger, M. D.: Climate and wildfire in the western United States, Bull. Am. Meteorol. Soc., 84, 595–604, https://doi.org/10.1175/BAMS-84-5-595, 2003.
Westerling, A. L., Hidalgo, H. G., Cayan, D. R., and Swetnam, T. W.: Warming and earlier spring increase western US forest wildfire activity, Science, 313, 940–943, https://doi.org/10.1098/rstb.2015.0178, 2006.
Williams, A. P., Abatzoglou, J. T., Gershunov, A., Guzman-Morales, J., Bishop, D. A., Balch, J. K., and Lettenmaier, D. P.: Observed impacts of anthropogenic climate change on wildfire in California, Earths Future, 7, 892–910, https://doi.org/10.1029/2019EF001210, 2019.
Wu, J., Serbin, S. P., Ely, K. S., Wolfe, B. T., Dickman, L. T., Grossiord, C., Michaletz, S. T., Collins, A. D., Detto, M., McDowell, N. G., and Wright, S. J.: The response of stomatal conductance to seasonal drought in tropical forests, Glob. Change Biol., 26, 823–839, https://doi.org/10.1111/gcb.14820, 2020.
Wullschleger, S. D., Gunderson, C. A., Hanson, P. J., Wilson, K. B., and Norby, R. J.: Sensitivity of stomatal and canopy conductance to elevated CO2 concentration–interacting variables and perspectives of scale, New Phytol., 153, 485–496, https://doi.org/10.1046/j.0028-646X.2001.00333.x, 2002.
Xu, C., McDowell, N. G., Sevanto, S., and Fisher, R. A.: Our limited ability to predict vegetation dynamics under water stress, New Phytol., 200, 298–300, https://doi.org/10.1111/nph.12450, 2013.
Xu, C., McDowell, N. G., Fisher, R. A., Wei, L., Sevanto, S., Christoffersen, B. O., Weng, E., and Middleton, R. S.: Increasing impacts of extreme droughts on vegetation productivity under climate change, Nat. Clim. Change., 9, 948–953, https://doi.org/10.1038/s41558-019-0630-6, 2019.
Xu, X., Medvigy, D., Powers, J. S., Becknell, J. M., and Guan, K.: Diversity in plant hydraulic traits explains seasonal and inter-annual variations of vegetation dynamics in seasonally dry tropical forests, New Phytol., 212, 80–95, https://doi.org/10.1111/nph.14009, 2016.
Yebra, M., Chuvieco, E., and Riaño, D.: Estimation of live fuel moisture content from MODIS images for fire risk assessment, Agric. For. Meteorol., 148, 523–536, https://doi.org/10.1016/j.agrformet.2007.12.005, 2008.
Yebra, M., Quan, X., Riaño, D., Larraondo, P. R., van Dijk, A. I., and Cary, G. J.: A fuel moisture content and flammability monitoring methodology for continental Australia based on optical remote sensing, Remote. Sens. Environ., 212, 260–272, https://doi.org/10.4225/41/5837cd92ada9f, 2018.
Zarco-Tejada, P. J., Rueda, C. A., and Ustin, S. L.: Water content estimation in vegetation with MODIS reflectance data and model inversion methods, Remote. Sens. Environ., 85, 109–124, https://doi.org/10.1016/S0034-4257(02)00197-9, 2003.