the Creative Commons Attribution 4.0 License.
the Creative Commons Attribution 4.0 License.
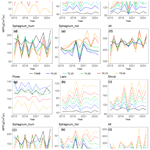
Extending a land-surface model with Sphagnum moss to simulate responses of a northern temperate bog to whole ecosystem warming and elevated CO2
Daniel M. Ricciuto
Peter E. Thornton
Xiaofeng Xu
Fengming Yuan
Richard J. Norby
Anthony P. Walker
Jeffrey M. Warren
Jiafu Mao
Paul J. Hanson
Lin Meng
David Weston
Natalie A. Griffiths
Mosses need to be incorporated into Earth system models to better simulate peatland functional dynamics under the changing environment. Sphagnum mosses are strong determinants of nutrient, carbon, and water cycling in peatland ecosystems. However, most land-surface models do not include Sphagnum or other mosses as represented plant functional types (PFTs), thereby limiting predictive assessment of peatland responses to environmental change. In this study, we introduce a moss PFT into the land model component (ELM) of the Energy Exascale Earth System Model (E3SM) by developing water content dynamics and nonvascular photosynthetic processes for moss. The model was parameterized and independently evaluated against observations from an ombrotrophic forested bog as part of the Spruce and Peatland Responses Under Changing Environments (SPRUCE) project. The inclusion of a Sphagnum PFT with some Sphagnum-specific processes in ELM allows it to capture the observed seasonal dynamics of Sphagnum gross primary production (GPP) albeit with an underestimate of peak GPP. The model simulated a reasonable annual net primary production (NPP) for moss but with less interannual variation than observed, and it reproduced aboveground biomass for tree PFTs and stem biomass for shrubs. Different species showed highly variable warming responses under both ambient and elevated atmospheric CO2 concentrations, and elevated CO2 altered the warming response direction for the peatland ecosystem. Microtopography is critical: Sphagnum mosses on hummocks and hollows were simulated to show opposite warming responses (NPP decreasing with warming on hummocks but increasing in hollows), and hummock Sphagnum was modeled to have a strong dependence on water table height. The inclusion of this new moss PFT in global ELM simulations may provide a useful foundation for the investigation of northern peatland carbon exchange, enhancing the predictive capacity of carbon dynamics across the regional and global scales.
- Article
(1648 KB) - Full-text XML
-
Supplement
(1060 KB) - BibTeX
- EndNote
This paper has been authored by UT-Battelle, LLC, under contract no. DE-AC05-00OR22725 with the US Department of Energy. The publisher, by accepting the article for publication, acknowledges that the United States government retains a nonexclusive, paid-up, irrevocable, worldwide license to publish or reproduce the published form of this paper or allow others to do so for United States government purposes. The Department of Energy will provide public access to these results of federally sponsored research in accordance with the DOE Public Access Plan (http://energy.gov/downloads/doe-public-access-plan).
Boreal peatlands store at least 500 pg of soil carbon due to the incomplete decomposition of plant litter inputs resulting from a combination of low temperature and water-saturated soils. Because of this capacity to store carbon, boreal peatlands have played a critical role in regulating the global climate since the onset of the Holocene (Frolking and Roulet, 2007; Yu et al., 2010). The total carbon stock is large but uncertain: a new estimation of northern peatland carbon stock of 1055 pg was recently reported by Nichols and Peteet (2019). The rapidly changing climate at high latitudes is likely to impact both primary production and decomposition rates in peatlands, contributing to uncertainty in whether peatlands will continue their function as net carbon sinks in the long term (Moore et al., 1998; Turetsky et al., 2002; Wu and Roulet, 2014). Manipulative experiments and process-based models are thus needed to make defensible projections of the net carbon balance of northern peatlands under anticipated global warming (Hanson et al., 2017; Shi et al., 2015).
Peatlands are characterized by a ground layer of bryophytes, and the raised or ombrotrophic bogs of the boreal zone are generally dominated by Sphagnum mosses that contribute significantly to total ecosystem CO2 flux (Oechel and Van Cleve, 1986; Williams and Flanagan, 1998; Robroek et al., 2009; Vitt, 2014). Sphagnum mosses also strongly affect the hydrological and hydrochemical conditions at the raised bog surface (Van, 1995; Van der Schaaf, 2002). As a result, microclimate and Sphagnum species interactions influence the variability of both carbon accumulation rates and water and exchanges within peatland and between peatland and atmosphere (Heijmans et al., 2004a, b; Rosenzweig et al., 2008; Brown et al., 2010; Petrone et al., 2011; Goetz and Price, 2015). Functioning as a keystone species of boreal peatlands, Sphagnum mosses strongly influence the nutrient, carbon, and water cycles of peatland ecosystems (Nilsson and Wardle, 2005; Cornelissen et al., 2007; Lindo and Gonzalez, 2010; Turetsky et al., 2010, 2012) and exert a substantial impact on ecosystem net carbon balance (Clymo and Hayward; 1982; Gorham, 1991; Wieder, 2006; Weston et el., 2015; Walker et al., 2017; Griffiths et al., 2018).
Numerical models are useful tools to identify knowledge gaps, examine long-term dynamics, and predict future changes. Earth system models (ESMs) simulate global processes, including the carbon cycle, and are primarily used to make future climate projections. Poor model representation of carbon processes in peatlands is identified as a deficiency, causing biases in simulated soil organic mass and heterotrophic respiratory fluxes for current ESMs (Todd-Brown et al., 2013; Tian et al., 2015). Although most ESMs do not include moss, a number of offline dynamic vegetation models and ecosystem models do include one or more moss plant functional types (PFTs) (Pastor et al., 2002; Nungesser, 2003; Zhuang et al., 2006; Bond-Lamberty et al., 2007; Heijmans et al., 2008; Euskirchen et al., 2009; Wania et al., 2009; Frolking et al., 2010). Several peatland-specific models contain moss species and have been applied globally or at selected peatland sites. For example, the McGill Wetland Model (MWM) was evaluated using the measurements at Degerö Stormyr and the Mer Bleue bogs (St-Hilaire et al., 2010). The peatland version of the General Ecosystem Simulator – Model of Raw Humus, Moder, and Mull (GUESS-ROMUL) was used to simulate the changes in daily CO2 exchange rates with water table position at a fen (Yurova et al., 2007). The PEATBOG model was implemented to characterize peatland carbon and nitrogen cycles in the Mer Bleue bog, including moss PFTs but without accounting for microtopography (Wu and Blodau, 2013). The CLASS-CTEM model (the coupled Canadian Land Surface Scheme and the Canadian Terrestrial Ecosystem Model), which includes a moss layer as the first soil layer, was applied to simulate water, energy, and carbon fluxes at eight different peatland sites (Wu et al., 2016). The IAP-RAS (Institute of Applied Physics, Russian Academy of Sciences) wetland methane (CH4) model with a 10 cm thick moss layer (Mokhov et al., 2007) was run globally to simulate the distribution of CH4 fluxes (Wania et al., 2013). The CHANGE model (a coupled hydrological and biogeochemical process simulator), which includes a moss cover layer (Launiainen et al., 2015), was used to investigate the effect of moss on soil temperature and carbon flux at a tundra site in northeastern Siberia (Park et al., 2018). Chadburn et al. (2015) added a surface layer of moss to the JULES land-surface model to consider the insulating effects and treated the thermal conductivity of moss depending on its water content to investigate the permafrost dynamics. Porada et al. (2016) integrated a stand-alone dynamic nonvascular vegetation model LiBry (Porada et al., 2013) to land-surface scheme JSBACH, but JSBACH mainly represents bryophyte and lichen cover in upland forest and not a peatland ecosystem. Druel et al. (2017) investigated the vegetation–climate feedbacks at high latitudes by introducing a nonvascular plant type representing mosses and lichens to the global land-surface model ORCHIDEE. Moreover, those models did not consider microtopography and the lateral transports between hummocks and hollows. Two models, the “ecosys” model (Grant et al., 2012) and CLM_SPRUCE (Shi et al., 2015), have been parameterized to represent peatland microtopographic variability (e.g., the hummock and hollow micro-terrain characteristic of raised bogs) with lateral connections across the topography. The prediction of water table dynamics in the “ecosys” model is constrained by specifying a regional water table at a fixed height and a fixed distance from the site of interest, thereby missing key controlling factors of a precipitation-driven dynamic water table (Shi et al., 2015). The CLM_SPRUCE model (Shi et al., 2015) was developed to parameterize the hydrological dynamics of lateral transport for microtopography of hummocks and hollows in the raised bog environment of the SPRUCE (Spruce and Peatland Responses Under Changing Environments) experiment (Hanson et al., 2017). That model version did not include the biophysical dynamics of Sphagnum moss, and it used a prescribed leaf area instead of allowing leaf area to evolve prognostically.
In this study, we introduce a new Sphagnum moss PFT into the model and migrate the entire raised-bog capability into the new Energy Exascale Earth System Model (E3SM), specifically into version 1 of the E3SM land model (ELM v1; Ricciuto et al., 2018). The objectives of this study are as follows: (1) to introduce a Sphagnum PFT to the ELM model with additional Sphagnum-specific processes to better capture the peatland ecosystem and (2) to apply the updated ELM to explore how an ombrotrophic, raised-dome bog peatland ecosystem will respond to different scenarios of warming and elevated atmospheric CO2 concentration.
2.1 Model provenance
ELM v1 is the land component of E3SM v1, which is supported by the US Department of Energy (DOE). Developed by multiple DOE laboratories, E3SM consists of atmosphere, land, ocean, sea ice, and land ice components linked through a coupler that facilitates across-component communication (Golaz et al., 2019). ELM was originally branched from the Community Land Model (CLM4.5; Oleson et al., 2013) with new developments that include representation of coupled carbon, nitrogen, and phosphorus controls on soil and vegetation processes and new plant carbon and nutrient storage pools (Ricciuto et al., 2018; Yang et al., 2019; Burrows et al., 2020). Inputs of new mineral nitrogen of ELM are from atmospheric deposition and biological nitrogen fixation. The fixation of new reactive nitrogen from atmospheric N2 by soil microorganisms is an important component of nitrogen budgets. ELM follows the approach of Cleveland et al. (1999) that uses an empirical relationship of biological nitrogen fixation as a function of net primary production to predict the nitrogen fixation. The model version used in this study is designated ELM_SPRUCE and includes the new implementation of Sphagnum mosses, as well as the hydrological dynamics of lateral transport between hummock and hollow microtopographies. The implementation has been parameterized based on observations from the S1-Bog in northern Minnesota, USA, as described by Shi et al. (2015) with additional details provided below.
2.2 Nonvascular plants: Sphagnum mosses
To represent the nonvascular plant, Sphagnum mosses, we modified the C3 arctic grasses equations as follows. We considered Sphagnum biomass to be represented mainly by leaf and stem carbon (only a very shallow root). In addition, we modified the vascular C3 arctic grasses equations for photosynthesis and stomatal conductance (see below the new model development) and the associated parameters as reported by Tables 1–3. We use the same framework as for C3 arctic grasses, but the Ball–Berry slope term is assumed to be zero, and the intercept term is the conductance term as a function of water content of Sphagnum mosses. For all other processes like the evapo(transpi)ration and associated parameters not described below, we used the C3 arctic grasses equations reported by Oleson et al. (2013). Drying impacts the conductance and affects evapo(transpi)ration of the internal water. The specific leaf area (SLA) and leaf C : N ratio parameters are strong controls on the maximum rate of Rubisco carboxylase activity (Vcmax) and therefore overall productivity and Sphagnum moss leaf area index (LAI). The high sensitivities occur because LAI is a strong control on evapo(transpi)ration.
Table 2PFT-specific optimized model parameters.
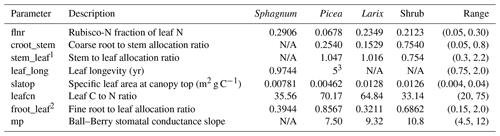
Optimized values of PFT-specific parameters. The range column values in
brackets indicate the range of acceptable parameter values used in the
sensitivity analysis and the optimization across all four PFTs in the format
(minimum, maximum). N/A indicates that parameter is not relevant for that
PFT.
1 For tree PFTs, this parameter depends on NPP. The value shown is the
allocation at an NPP of 800 g C m−2 yr−1.
2 The fine root pool is used as a surrogate for non-photosynthetic
tissue in Sphagnum.
3 This parameter was not optimized; we used the default value.
Table 3Non-PFT-specific optimized model parameters.
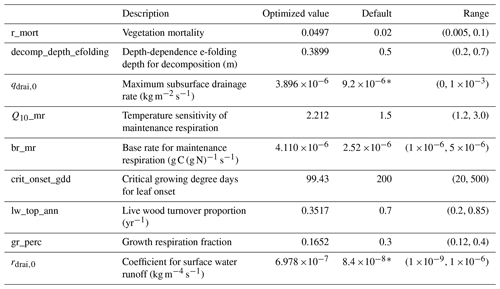
Optimized and default values for non-PFT-specific parameters. The range
column values in brackets indicate the range of acceptable parameter values
used in the sensitivity analysis and the optimization in the format
(minimum, maximum).
∗ Previously calibrated value from Shi et al. (2015).
2.3 New model developments
2.3.1 Water content dynamics of Sphagnum mosses
The main sources for water content of Sphagnum mosses are passive capillary water uptake from peat and the interception of atmospheric water on the capitulum (growing tip of the moss) (Robroek et al., 2007). Capillary water uptake, the internal Sphagnum moss water content, is modeled as functions of soil water content and evaporation losses. Water intercepted on the Sphagnum moss capitulum is modeled as a function of moss foliar biomass, current canopy water, water drip, and evaporation losses.
Since evaporation at the Sphagnum surface depends on the atmospheric water vapor deficit, moss–atmosphere conductance, and available water pool which depends on capillary wicking of water up to the surface, we developed a relationship between measured soil water content at depth and surface Sphagnum water content. At SPRUCE, the peat volumetric water content is measured at several depths using automated sensors (model 10HS, Decagon Devices, Inc., Pullman, WA) calibrated for the site-specific upper peat soil using mesocosms (reference Fig. S1 in the Supplement; Hanson et al., 2017). During those calibrations, we periodically sampled the surface Sphagnum for gravimetric water content and water potential using a dew point potentiometer (WP4, Decagon Devices, Inc.) which also provided a surface soil water retention curve. The destructive sampling of surface Sphagnum was primarily hummock species but did include some hollow species. The automated measurements of peat water content at 10 cm depth were shown to be a good indicator of surface Sphagnum water content (Fig. 1). Based on this relationship, we model the water content of Sphagnum moss due to capillary rise (Winternal) (g water ∕ g dry moss) as follows:
where Soilvol is the averaged volumetric soil water of modeled soil layers nearest the 10 cm depth horizon (layers three and four in the ELM v1 vertical layering scheme).
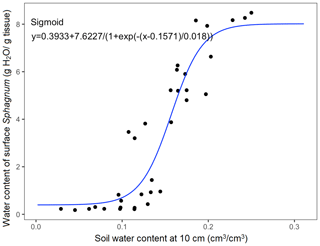
Figure 1The measured relationship between soil water content at depth and the water content of surface Sphagnum based on destructive sampling.
The Sphagnum moss surface water (Wsurface) was calculated using the model predicted canopy water and the dry foliar biomass as follows:
where Wsurface (g water ∕ g dry moss) is the surface water content and fmass is the foliar biomass of Sphagnum mosses. The can_water is the Sphagnum moss canopy water, and it is simulated by a function of interception, canopy drip, dew, and canopy evaporation (Oleson et al., 2013).
The total water content (Wtotal) of Sphagnum mosses is the sum of water taken up from peat and the surface water content (St-Hilaire et al., 2010; Wu et al., 2013).
2.3.2 Modeling Sphagnum CO2 conductance and photosynthesis
ELM_SPRUCE computes photosynthetic carbon uptake (gross primary production or GPP) for each vascular PFT on a half-hourly time step based on the Farquhar biochemical approach (Farquhar et al., 1980; Collatz et al., 1991, 1992) with implementation as described by Oleson et al. (2013). While Sphagnum lacks a leaf cuticle and stomata that regulate water loss and CO2 uptake in vascular plants (Titus et al., 1983), the primary transport pathway for CO2 is through the cells and is analogous to mesophyll conductance in higher plants. Thus, we calculate the total conductance to CO2 for Sphagnum mosses by using total water content following the method reported by Williams and Flanagan (1998) described below. Goetz and Price (2015) also indicated that capillary rise through the peat is essential to maintain a water content sufficient for photosynthesis for Sphagnum moss species but that atmospheric inputs can provide small but critical amounts of water for physiological processes.
The stomatal conductance for vascular plant types in ELM_SPRUCE is derived from the Ball–Berry conductance model (Collatz et al., 1991). That model relates stomatal conductance to net leaf photosynthesis scaled by the relative humidity and the CO2 concentration at the leaf surface. The stomatal conductance (gs) and boundary layer conductance (gb) are required to obtain the internal leaf CO2 partial pressure (Ci) of vascular PFTs:
where Ci is the internal leaf CO2 partial pressure, Ca is the atmospheric CO2 partial pressure, An is leaf net photosynthesis (µmol CO2 m−2 s−1), Patm is the atmospheric pressure, and values 1.4 and 1.6 are the ratios of the diffusivity of CO2 to H2O for stomatal conductance and the leaf boundary layer conductance, respectively.
For Sphagnum moss photosynthesis, we followed the method from the McGill Wetland Model (St-Hilaire et al., 2010; Wu et al., 2013), which is based on the effects of Sphagnum moss water content on photosynthetic capacity (Tenhunen et al., 1976) and total conductance of CO2 (Williams and Flanagan, 1998) and replaces the stomatal conductance representation used for vascular PFTs.
The total conductance to CO2 (gtc) was determined from a least-squares regression described by Williams and Flanagan (1998) as follows:
where Wtotal is as defined in Eq. (3). This relationship is only valid up to the maximum water holding capacity of mosses. Note that we assume that the boundary layer conductance is greater than moss surface layer conductance, and the moss surface layer conductance is greater than chloroplast conductance.
In addition to the water content, the effects of moss submergence were taken into account in the calculation of moss photosynthesis. Walker et al. (2017) reported significant impacts of submergence on measured Sphagnum GPP and modeled the effect by modifying the Sphagnum leaf (stem) area index. Submergence in Walker et al. (2017) was expressed as photosynthesizing stem area index (SAI) as a logistic function of water table depth. A maximum SAI of 3 was used, and the parameter combination that most closely described the GPP data gave a range of water table depth from −10 cm for complete submergence and SAI of ∼ 2.5 at 10 cm. This allowed for a range of processes such as floatation of Sphagnum with the water table and adhesion of water to the Sphagnum capitula. For simplicity, in ELM_SPRUCE, we calculated such impacts on Sphagnum GPP directly as a function of the height of simulated surface water, assuming that GPP from the submerged portion of photosynthetic tissue is negligible. GPP is thus reduced linearly according to the following equation:
where GPPsub is the GPP corrected for submergence effects, GPPorig is the original GPP, H2Osfc is the surface water height, and hmoss is the height of the photosynthesizing Sphagnum layer above the soil surface, set to 5 cm in our simulations. If H2Osfc is equal to or greater than hmoss, GPP is reduced to zero. Because in our simulations surface water is never predicted to occur in the hummocks, in practice this submergence effect only affects the moss GPP in the hollows.
3.1 Site description
We focused on a high C, ombrotrophic peatland (the S1-Bog) that has a perched water table with limited groundwater influence (Sebestyen et al., 2011; Griffiths and Sebestyen, 2016). This southern boreal bog is located in the Marcell Experimental Forest approximately 40 km north of Grand Rapids, Minnesota, USA (lat 47.50283∘, long −93.48283∘) (Sebestyen et al., 2011), and is the site of the SPRUCE climate change experiment (http://mnspruce.ornl.gov, last access: 13 January 2021; Hanson et al., 2017). The S1-Bog has a raised hummock and sunken hollow microtopography, and it is nearly covered by Sphagnum mosses. S. angustifolium (C.E.O. Jensen ex Russow) and S. fallax (Klinggr.) occupy 68 % of the moss layer and exist in both hummocks and hollows. S. magellenicum (Brid.) occupies ∼ 20 % of the moss layer and is primarily limited to the hummocks (Norby et al., 2019). The vascular plant community at the S1-Bog is dominated by the evergreen tree Picea mariana (Mill.) B.S.P., the deciduous tree Larix laricina (Du Roi) K. Koch, and a variety of ericaceous shrubs. Trees are present due to natural regeneration following strip cut harvesting in 1969 and 1974 (Sebestyen et al., 2011). The soil of this peat bog is the Greenwood series, a Typic Haplohemist (https://websoilsurvey.sc.egov.usda.gov, last access: 13 January 2021), and its average peat depth is 2 to 3 m (Parsekian et al., 2012)
Northern Minnesota has a subhumid continental climate with average annual precipitation of 768 mm and annual air temperature of 3.3 ∘C for the time period from 1965 to 2005. Mean annual air temperatures at the bog have increased about 0.4 ∘C per decade over the last 40 years (Verry et al., 2011).
3.2 Field measurements
Multiple observational pretreatment data (the data were collected prior to the initiation of the warming and CO2 treatments) were used in this study. The flux-partitioned GPP of Sphagnum mosses was derived from measured hourly Sphagnum–peat net ecosystem exchange (NEE) flux (Walker et al., 2017). The GPP–NEE relationship was also evaluated using observed vegetation growth and productivity allometric and biomass data on tree species, stem biomass for shrub species (Hanson et al., 2018a, b), and Sphagnum pretreatment net primary productivity (NPP) (Norby et al., 2019). ELM_SPRUCE was driven by climate data (temperature, precipitation, relative humidity, solar radiation, wind speed, pressure, and long-wave radiation) from 2011 to 2017 measured at the SPRUCE S1-Bog (Hanson et al., 2015a, b). The surface weather station is outside of the enclosures and not impacted by the experimental warming treatments that began in 2015. These data are available at https://mnspruce.ornl.gov/ (last access: 13 January 2021).
3.3 Simulation of the SPRUCE experiment
Based on measurements at the SPRUCE site, ELM_SPRUCE includes four PFTs: boreal evergreen needleleaf tree (Picea), boreal deciduous needleleaf tree (Larix), boreal deciduous shrub (representing several shrub species), and the newly introduced Sphagnum moss PFT. Currently, ELM_SPRUCE does not include light competition among multiple PFTs and thus does not represent cross-PFT shading effects. Our model also allows the canopy density of PFTs to change prognostically, and their fractional coverage is held constant. We used measurements from Sphagnum moss collected at a tussock tundra site in Alaska (Hobbie, 1996) to set several of the model leaf litter parameters for our simulations (Table 1). The values for other parameters have been optimized based on observations at the SPRUCE site (Tables 2 and 3; optimization methods described in Sect. 3.4). We prescribe both hummock and hollow microtopographies to have the same fractional PFT distribution. Consistent with Shi et al. (2015), hummocks and hollows were modeled on separate columns with lateral flow of water between them. All the ELM_SPRUCE simulations were conducted using a prognostic scheme for canopy phenology (Oleson et al., 2013).
The SPRUCE experiment at the S1-Bog consists of combined manipulations of temperature (various differentials up to +9 ∘C above ambient) and atmospheric CO2 concentration (ambient and ambient plus 500 ppm) applied in 12 m diameter and 8 m tall enclosures constructed in the S1-Bog. The whole ecosystem warming began in August 2015, elevated CO2 started from June 2016, and various treatments are envisioned to continue until 2025. Extensive pretreatment observations at the site began in 2009.
For the ELM_SPRUCE, we continuously cycled the 2011–2017 climate forcing (see Sect. 3.2) to equilibrate carbon and nitrogen pools under preindustrial atmospheric CO2 concentrations and nitrogen deposition and then launched a simulation starting from year 1850 through year 2017. This transient simulation includes historically varying CO2 concentrations, nitrogen deposition, and the land-use effects of a strip cut and harvest at the site in 1974. These simulations were used to compare model performance with pretreatment observations. A subset of these observations was also used for optimization and calibration (Sect. 3.4).
To investigate how the bog vegetation may respond to different warming scenarios and elevated atmospheric CO2 concentrations, we performed 11 model runs from the same starting point in the year 2015. These simulations were designed to reflect the warming treatments and CO2 concentrations being implemented in the SPRUCE experiment enclosures. The model simulations include one ambient case (both ambient temperature and CO2 concentration) and five simulations with modified input air temperatures to represent the whole ecosystem warming treatments at five levels (+0, +2.25, +4.50, +6.75, and +9.00 ∘C above ambient) and at ambient CO2 and another five simulations with the same increasing temperature levels and at elevated CO2 (900 ppm). In the treatment simulations, we also considered the passive enclosure effects which reduce incoming shortwave radiation and increase incoming longwave radiation (Hanson et al., 2017). Following the SPRUCE experimental design, there was no water vapor added so that the simulations used constant specific humidity instead of constant relative humidity across the warming levels. All the treatment simulations were performed through the year 2025 by continuing to cycle the 2011–2017 meteorological inputs (with modified temperature and radiation to reflect the treatments) to simulate future years.
3.4 Model sensitivity analysis and calibration
The vegetation physiology parameters in ELM_SPRUCE were originally derived from CLM4.5 and its predecessor, Biome-BGC, and represent broad aggregations of plant traits over many species and varied environmental conditions (White et al., 2000). To achieve reasonable model performance at SPRUCE, site-specific parameters and targeted parameter calibration are needed. Since the ELM_SPRUCE contains over 100 uncertain parameters, parameter optimization is not computationally feasible without first performing some dimensionality reduction. Based on previous ELM sensitivity analyses (e.g., Lu et al., 2018; Ricciuto et al., 2018; Griffiths et al., 2018), we chose 35 model parameters for further calibration (Tables 2 and 3). An ensemble of 3000 ELM_SPRUCE simulations were conducted, with each ensemble member using a randomly selected set of parameter values within uniform prior ranges. This model ensemble was first used to construct a polynomial chaos surrogate model which was then used to perform a global sensitivity analysis (Sargsyan et al., 2014; Ricciuto et al., 2018). Main sensitivity indices, reflecting the proportion of output variance that occurs for each parameter, are described in Sect. 4.1.
To minimize potential biases in model predictions of treatment responses, we calibrated the same 35 model parameters using pretreatment observations as data constraints. We employed a quantum particle swarm optimization (QPSO) algorithm (Lu et al., 2018). While this method does not allow for the calculation of posterior prediction uncertainties, it is much more computationally efficient than other methods such as Markov chain Monte Carlo (MCMC). The constraining data included year 2012–2013 tree growth and biomass (Hanson et al., 2018a), year 2012–2013 shrub growth and biomass (Hanson et al., 2018b), year 2012 and 2014 Sphagnum net primary productivity (Norby et al., 2019; Norby and Childs, 2018), enclosure-averaged leaf area index by PFT (year 2011 for tree and year 2012 for shrub and Sphagnum), and year 2011–2013 water table depth (WTD) observations aggregated to seasonal averages (Hanson et al., 2015b). The goal of the optimization is to minimize a cost function, which we define here as a sum of squared errors over all observation types weighted by observation uncertainties. When observation uncertainties were not available, we assumed a range of ±25 % from the default value. Site measurements were also used to constrain the ranges of two parameters: leafcn (leaf carbon to nitrogen ratio) and slatop (specific leaf area at canopy top). The uniform prior ranges for these parameters represent the range of plot to plot variability. Optimized parameter values are shown in Tables 2 and 3. Section 4 reports the results of simulations using these optimized parameters which were used to perform a spinup, transient (1850–2017), and set of 11 treatment simulations (2015–2025) as described above.
4.1 Model sensitivity analysis
Main effect (first-order) sensitivities are shown for eight model output quantities of interest: total site gross primary productivity (GPP), GPP for the moss PFT only (GPP_moss), total site net primary productivity (NPP), NPP for the moss PFT only (NPP_moss), total site vegetation transpiration (QVEGT), evaporation from the moss surface (QVEG_moss), net ecosystem exchange (NEE), and site total vegetation carbon (TOTVEGC) (Fig. 2). Out of 35 parameters investigated, 25 show a sensitivity index of at least 0.01 for one of the quantities of interest, and these are plotted in Fig. 2. In that figure, sensitivities are stacked in order from highest to lowest for each variable with the height of the bar equal to the sensitivity index. The first order sensitivities sum to at least 0.95 for all variables, indicating that higher order sensitivities (i.e., contributions to the sensitivity from combinations of two or more parameters) contribute relatively little to the variance in these quantities of interest.
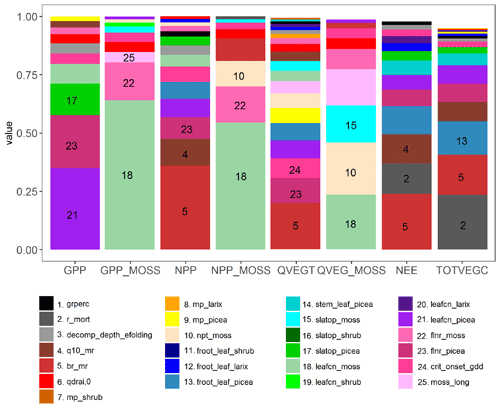
Figure 2Sensitivity analysis of ELM_SPRUCE for selected parameters (Tables 2 and 3). The colored bars indicate the fraction of variance in site gross primary productivity (GPP), moss-only GPP (GPP_MOSS), site net primary productivity (NPP), moss-only NPP (NPP_MOSS), total vegetation transpiration (QVEGT), moss evaporation (QVEG_MOSS), site net ecosystem exchange (NEE), and total vegetation carbon (TOTVEGC) controlled by each parameter. The legend shows the top 25 most influential parameters; the remaining parameters not shown have sensitivities of no more than 0.01 for any of the outputs. All variables represent 2011–2017 average values over the ambient conditions. For parameters that are treated as PFT dependent, the PFT is indicated with a suffix (picea, larix, shrub, or moss).
According to this analysis, the variance in total site GPP is dominated by three Picea parameters: the fraction of leaf nitrogen in Rubisco (flnr_picea), leaf carbon to nitrogen ratio (leafcn_picea), and the specific leaf area at canopy top (slatop_picea). GPP sensitivity for the moss PFT is dominated by the same three parameters but for the moss PFT instead of Picea (flnr_moss, leafcn_moss, and slatop_moss). For NPP, QVEGT, and NEE, the highest sensitivity is the maintenance respiration base rate br_mr, similar to earlier results in Griffiths et al. (2018). The maintenance respiration temperature sensitivity Q10_mr is also a key parameter for NPP and NEE. The critical onset growing degree day threshold (crit_onset_gdd), which drives deciduous phenology in the spring for the Larix and shrub PFTs, is an important parameter for NPP and NEE. The flnr_picea parameter is important for both NPP and QVEGT. For NPP_moss and QVEG_moss, leafcn_moss and the ratio of non-photosynthesizing tissue to photosynthesizing tissue (npt_moss) are sensitive. For TOTVEGC and NEE, vegetation mortality (r_mort) is also a sensitive parameter. For the site-level quantities of interest, at least 10 parameters contribute significantly to the uncertainty, illustrating the complexity of the model and large number of processes contributing to uncertainty in SPRUCE predictions. For the moss variables, there are some cases where significant sensitivities exist for non-moss PFT parameters. For example, leafcn_shrub is the seventh most sensitive parameter for GPP_moss, indicating that competition between the PFTs for resources may be important. In this case, uncertainty about parameters on one PFT may drive uncertainties in the simulated productivity of other PFTs.
4.2 Model evaluation
Our model simulates GPP for vascular plants and Sphagnum moss in both hummock and hollow settings with separate calculations for each PFT. Here we use the model estimate of GPP prior to downregulation by nutrient limitation from the ambient case based on recent studies indicating that nutrient limitation effects are occurring downstream of GPP (Raczka et al., 2016; Metcalfe et al., 2017; Duarte et al., 2017). This treatment of nutrient limitation on GPP has been modified in a more recent version of ELM, and our moss modifications will be merged to that version as a next step. For now, by referring to the pre-downregulation GPP, we are capturing the most significant impact of those changes for the purpose of comparison to observations.
Our model simulated two seasonal maxima of Sphagnum moss GPP: one at the end of May and the other in August (Fig. 3). Both peaks are lower than the maximum of observed (flux-partitioned) GPP, which occurs in August. Based on results of the sensitivity analysis, it could be that the base rate for maintenance respiration for moss is too high, causing an underestimate of NPP and biomass, which leads to a low bias in peak GPP.
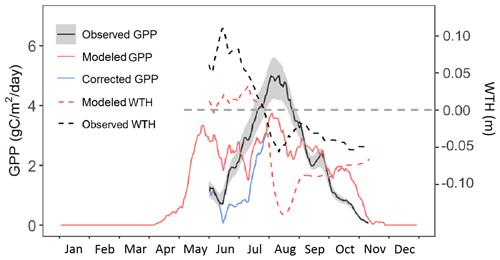
Figure 3Predicted GPP (solid red line) compared with flux-partitioned GPP (solid black line; GPP data were not used in the parameters optimization) of Sphagnum mosses for the year 2014. The blue line is the predicted GPP corrected with the observed water table height. The dashed black and red lines are observed and modeled water table height (the dashed gray line is the hollow surface).
During June and October, observations suggest that ELM_SPRUCE overpredicts GPP. The model does limit GPP as a function of the depth of standing water on the bog surface (Eq. 7). The water table height (WTH) above the bog surface is being predicted by the model (dashed red line in Fig. 3), and while the seasonal pattern of higher water table in the spring and lower water table in the fall agrees well with observations (dashed black line in Fig. 3), the predicted WTH is generally too low by 5–10 cm. The modeled WTH here is for hollow. We turned off the lateral transport when there is ice on the soil layers above the water table to avoid an unreasonable amount of ice accumulation on the frozen layers which results when there is no flow from hummock to hollow. Forcing the modeled GPP to respond to observed WTH (during the period with observations) gives a pattern of increasing GPP through June and July which is more consistent with observations (blue line in Fig. 3). We do not have observations for GPP earlier than June due to limitations of the instrumentation when the bog surface is flooded.
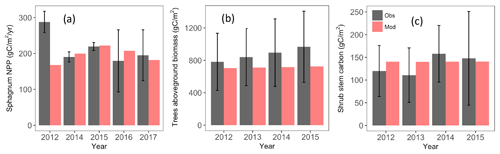
Figure 4Predicted (red bars) Sphagnum NPP (a), aboveground tree biomass (b), and shrub stem carbon (c) compared with the observations (black bars). Observed NPP data are based on growth of 12–17 bundles of 10 Sphagnum stems in 2012–2015 (unpublished data) and in two ambient plots by the method described by Norby et al. (2019) in 2016–2017 (data in Norby and Childs, 2018). The Sphagnum NPP data for the years 2015–2017 and aboveground tree biomass and shrub stem carbon for the years 2014–2015 are independent of the related parameter optimization.
The model simulated reasonable annual values for Sphagnum NPP for the period 2014–2017 but showed much lower NPP compared to observation (139 vs. 288 g C m−2 yr−1) for the year 2012 (Fig. 4a). Measurement uncertainties are larger in 2016–2017 than in earlier years, perhaps related to a new measurement protocol for those years, and the model estimates are within measurement uncertainty bounds for years 2014–2017 (Griffiths et al., 2018; Norby et al., 2019). The observed Sphagnum NPP was measured at different plots, and each plot included different species abundances. As a result, the scaled NPP includes spatial variations and uncertainty in species distribution (Norby and Childs, 2018).
Simulated tree aboveground biomass is within the observed inter-plot variability (Fig. 4b). Observations suggest an increasing trend in tree biomass which was not predicted by the model. The optimized parameters show increased mortality and autotrophic respiration rate parameters compared to the default model (Table 3), which causes the simulations to approach steady state relatively quickly after the 1974 disturbance. However, the sensitivity analysis also identifies these mortality and maintenance respiration parameters as highly sensitive; therefore, this simulated response is uncertain. For the shrub stem carbon, the simulated mean from year 2012 to 2015 was 140.4 g C m−2, slightly higher than the observation (133.9 g C m−2) but well within the observed range of inter-plot variability (Fig. 4c).
4.3 Simulated carbon cycle response to warming and elevated atmospheric CO2 concentration
Different PFTs demonstrated different warming responses for both ambient CO2 and elevated CO2 concentration conditions (Fig. 5). Both Larix and shrub NPP increased with warming under both CO2 concentration conditions (Fig. 5b, c, h, and i). In addition, CO2 fertilization stimulates the growth of these two PFTs, and the fertilization effect further increases with warming (Fig. S1). In contrast, Picea NPP decreased with warming levels (Fig. 5a and g) for both CO2 conditions. For Sphagnum, NPP decreased in hummocks but increased in hollows with increasing temperature (Fig. 5d, e, j, and k). The CO2 fertilization also stimulates the growth of the Picea and Sphagnum PFTs (Fig. 5a, d, e, g, j, and k). The total enclosure NPP for all PFTs responded differently to the warming only and warming with elevated CO2 (Fig. 5f and l). The total enclosure NPP for each warming level changed less under the ambient CO2 condition than those with the elevated CO2 condition, and NPP decreased with warming in most of the years under the ambient CO2 condition but increased under the elevated CO2 condition (Fig. 5f and l). This result demonstrated that the elevated CO2 scenario changes the sign of the NPP warming response for the bog peatland ecosystem.
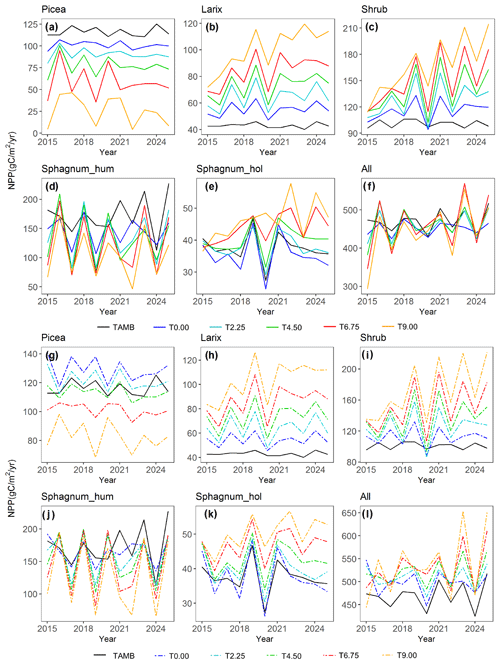
Figure 5Predicted NPP response to warming with ambient atmospheric CO2 (a–f, solid lines) and warming with elevated atmospheric CO2 concentration (g–l, dashed lines). The solid black line TAMB is the ambient temperature and CO2 case, and T0.00 to T9.00 means increasing temperature from 0 to 9 ∘C.
Compared with the ambient biomass, the biomass of black spruce (Picea) significantly decreased, but the biomass of Larix significantly increased under the greatest warming treatment (+9.00 ∘C; Fig. 6). Biomass of shrub and hollow Sphagnum also increased but less than Larix did. The hummock Sphagnum biomass also showed a strong correlation with water table height at roughly a 3-month lag (the maximum correlation occurs with an 82 d lag; R2=0.56). NPP is allocated instantaneously into biomass. A positive NPP anomaly caused by water table shifts leads to higher LAI, which also increases future productivity for some amount of time even if the water table returns to normal. Sphagnum biomass has a 1-year turnover time in the simulation. This combination of effects leads to a roughly 3-month time lag. Due to the relative lower height of the water table in the hummock than the hollow, the simulated hummock Sphagnum was more significantly water-stressed than the hollow Sphagnum as the water table height declines. This is consistent with multiple studies finding that an increase in temperatures associated with drought (low water table height) reduces Sphagnum growth (Bragazza et al., 2016; Granath et al., 2014; Mazziotta et al., 2018). We plotted the predicted canopy evaporation for hummock and hollow Sphagnum responses to warming and found that both hummock and hollow Sphagnum canopy evaporation amounts increase with warming for both ambient and elevated atmospheric CO2 conditions despite the Larix and shrubs growing with warming. Moreover, the hollow Sphagnum canopy evaporation warming response is stronger than that of the hummock Sphagnum (Fig. S2). In summary, the growth of bog vegetation is predicted to have species-specific warming responses that differ in sign and magnitude.
Sphagnum moss is the principal plant involved in the peat accumulation in peatland ecosystems, and the effective characterization of its biophysical and physiological responses has implications for predicting peatland and global carbon, water, and climate feedbacks. This study moves us closer to our long-term goal of improving the prediction of peatland water, carbon, and nutrient cycles in ELM_SPRUCE by introducing a new Sphagnum moss PFT and implementing water content dynamics and photosynthetic processes for this nonvascular plant. The Sphagnum model development combined with our previous hummock and hollow microtopography representation and laterally coupled two-column hydrology scheme enhance the capability of ELM_SPRUCE in simulating high-carbon wetland hydrology and carbon interactions and their responses to plausible environmental changes.
5.1 Uncertainties in simulating Sphagnum productivity
Our predicted peak GPP is similar to the results found by Walker et al. (2017) when they calculated the internal resistance to CO2 diffusion as a function of Sphagnum water content using a stand-alone photosynthesis model. In both cases, the predicted peak GPP is lower than observations. Walker et al. (2017) were, however, able to capture the observed peak magnitude with a combination of light extinction coefficient, canopy clumping coefficient, maximum SAI, and a logistic function describing the effective Sphagnum SAI in relation to the water table. Here we used model default values for the light extinction and canopy clumping coefficients. While the water table impacts Sphagnum productivity in our simulation, modeled LAI is mainly controlled by NPP and turnover. In addition, we use the default formulation for the acclimation of Vcmax in ELM which is based on a 10 d mean growing temperature. At this point, we do not have sufficient measurements to test this assumption, but we can prioritize these measurements in the future. Sphagnum temperature is computed from surface energy balance, but because the current model does not estimate the shading effects from trees and shrubs, this may be overestimated. Moreover, biases in predicted water table height contribute to errors in the calculated submergence effect. Improving these biases and assuming an exponential rather than a linear CO2 uptake profile may improve representation of the submergence effect. All these aspects may be attributed to the biases of the simulated Sphagnum GPP. We can consider this in the future when we have more detailed measurements. Further investigation is thus needed to understand how representative the chamber-based observations of the larger-scale SPRUCE enclosures from Walker et al. (2017) are and to reconcile these GPP estimates with plot-level NPP observations (Norby et al., 2019).
The hydrology cycle, especially water table depth (WTD), is also a key factor that influences the seasonality of GPP in Sphagnum mosses (Lafleur et al., 2005; Riutta et al., 2007, Sonnentag et al., 2010; Grant et al., 2012; Kuiper et al., 2014; Walker et al., 2017). One key feedback is that if the water table declines, there can be enhanced decomposition and subsidence of the peat layer, which brings the surface down closer to the water table again. But we currently did not consider the peat layer elevation changes in our model, and this will be one of the future development directions. The capillary rise plays into the Sphagnum hydrological balance, which varies depending on water table depth and evaporative demand. At short timescales or under rapidly changing conditions, there may not be equilibration between the Sphagnum water content and the peat moisture. Generally, the Sphagnum water content will equilibrate with the peat on a daily basis outside the plot since the dew point is often reached at night. But since the vapor pressure deficit does not go to zero inside the warmer plots, some disequilibration could remain. High-frequency latent heat flux data from the site are currently lacking but could help to constrain these effects in the future.
The current phenology observations also include whether Sphagnum hummock and hollow are wet or dry, and we could look at the relationship with soil water content sensors in the future. Moreover, the timescales for rewetting may change as the peat dries since the cross section for capillary rise will decline, and thus the maximum flux to the surface will decline. At some point between gravity potential and reduced hydraulic conductivity, we expect that the capillarity will no longer satisfy evaporative demand. Alternately, under saturated conditions when the water table is close to the Sphagnum surface, Sphagnum photosynthesizing tissue can become submerged or surrounded by a film of water that is likely to reduce the effective LAI of the Sphagnum and thus reduce photosynthesis (Walker et al., 2017). Submerged Sphagnum can take up carbon derived from CH4 via symbiotic methanotrophs (Raghoebarsing et al., 2005), but in any case, CO2 diffusion for photosynthesis will dramatically decrease under water. Larmola et al. (2014) also reported that the activity of oxidizing bacteria provides not only carbon but also nitrogen to peat mosses and, thus, contributes to carbon and nitrogen accumulation in peatlands, which store approximately one-third of the global soil carbon pool. We currently did not consider this kind of CH4 associated carbon and nitrogen uptake by Sphagnum.
The live green Sphagnum moss layer buffers the exchange of energy and water at the soil surface and regulates the soil temperature and moisture because of its high water holding capacity and the insulating effect (McFadden et al., 2003; Block et al., 2011; Turetsky et al., 2012; Park et al., 2018). Currently, we apply the same method for the hummock and hollow Sphagnum water content prediction and can test the model against the measured data when more data are available. Our model still can predict Sphagnum water content differences between these microtopographies as expected, though with the water content of hollows greater than that of hummocks. In addition, our model is able to represent the self-cooling effect, although we do not yet have measurements available to validate the model. The relationship of the differences between vegetation temperature (TV) and 2 m air temperature (TBOT) (TV-TBOT) and canopy evaporation for both hummock and hollow Sphagnum demonstrated that the differences of TV-TBOT was negative and the canopy evaporation had a negative relationship with TV-TBOT (Fig. S3). Moreover, Walker et al. (2017) reported that the function of Sphagnum water content to soil water content or to water table depth they used for the SPRUCE site was empirical and may not be representative for a peatland ecosystem. To better represent the peatland ecosystem in our model, we will eventually treat the Sphagnum mosses as the “top” soil layer with a lower thermal conductivity and higher hydraulic capacity (Beringer et al., 2001; Wu et al., 2016; Porada et al., 2016).
5.2 Predicted warming and elevated CO2 concentration response uncertainties
Our model warming simulations suggested that increasing temperature reduced the Picea growth but increased the growth of Larix under both ambient and elevated atmospheric CO2 conditions. The main reason for this model difference in response for the two tree species is that despite their similar productivity under ambient conditions, Picea has more respiring leaf and fine root biomass because of lower SLA, longer leaf longevity, and higher fine root allocation. Therefore, warming results in a much larger increase in maintenance respiration relative to changes in NPP for Picea compared to Larix (Figs. 5 and S4). Increased tree growth and productivity in response to the recent climate warming for high-latitude forests has been reported (Myneni et al., 1997; Chen et al., 1999; Wilmking et al., 2004; Chavardes, 2013). On the other hand, reductions in tree growth and negative correlations between growth and temperature have also been shown (Barber et al., 2000; Wilmking et al., 2004; Silva et al., 2010; Juday and Alix 2012; Girardin et al., 2016; Wolken et at., 2016).
Our model also predicted the increasing growth of shrubs with increased temperature in a similar way to the simulated increase in shrub cover caused mainly by warmer temperatures and longer growing seasons reported by Miller and Smith (2012) using their model LPJ-GUESS. In addition, several other modeling studies have also found increased biomass production and LAI related to shrub invasion and replacement of low shrubs by taller shrubs and trees in response to increased temperatures in tundra regions (Zhang et al., 2013; Miller and Smith, 2012; Wolf et al., 2008; Porada et al., 2016; Rydssa et al., 2017).
The responses of Sphagnum mosses to warming simulated by ELM_SPRUCE showed that Sphagnum growth in hollows was consistently higher with increased temperatures when water availability was not limited. Sphagnum growing on hummocks, on the other hand, showed negative warming responses that are related to the strong dependency on water table height. A recent study of the same SPRUCE site (Norby et al., 2019) had suggested that the hummock and hollow microtopography had a larger influence on Sphagnum responses to warming than species-specific traits. In addition, the previous studies had demonstrated that the most dominant mechanism of Sphagnum warming response was probably through the effect of warming on depth to the water table and water content of the acrotelm, both of which responded to increasing temperature (Grosvernier et al., 1997; Rydin, 1985; Weltzin et al., 2001; Norby et al., 2019). Moreover, desiccation of capitula due to increased evaporation associated with higher temperatures and vapor pressure deficits can reduce Sphagnum growth independent of the water table depth (Gunnarsson et al., 2004). We currently used the same parameters for both hummock and hollow but could consider species differences in the future. Norby et al. (2019) investigated different Sphagnum species at the same site and reported there was no support for the hypothesis that species more adapted to dry conditions (e.g., S. magellanicum and Polytrichum mainly on hummocks) would be more resistant to the stress and would increase in dominance, and both hummock and hollow Sphagnum decline with warming despite the differences between them. This declining trend may be in part due to increased shading from the shrub layer which expands with warming (McPartland et al., 2020).
Ecosystem warming can have direct and indirect effects on Sphagnum moss growth. The growth of Sphagnum may be reduced directly by higher air temperature due to the relatively low temperature optima of moss photosynthesis (Hobbie et al.,1999; Van Gaalen, 2007; Walker et al., 2017). On the other hand, increased shading by the shrub canopy and associated leaf litter could indirectly decrease moss growth (Chapin et al., 1995; Hobbie and Chapin 1998; Van der Wal et al., 2005; Walker et al., 2006; Breeuwer et al., 2008). In contrast, other studies suggest that Sphagnum growth can be promoted by a cooling effect of shading on the peat surface, by alleviating photo-inhibition of photosynthesis, and also by reducing evaporation stress (Busby et al., 1978; Murray et al., 1993; Man et al., 2008; Walker et al., 2015; Bragazza et al., 2016; Mazziotta et al., 2018). Our model sensitivity analysis also indicated that the parameters of shrub showed significant sensitivities to Sphagnum mosses' GPP, indicating that competition between the PFTs for resources might be important. Moreover, ELM_SPRUCE did predict the enhancement of shrub and Larix tree with increased temperatures in both ambient and elevated CO2 conditions (LAI increasing with warming; Fig. S5). Currently, ELM_SPRUCE does not include light competition among multiple PFTs and thus does not represent cross-PFT shading effects, which may contribute to the warming and elevated CO2 response differences between our model prediction and the observed result of Norby et al. (2019). Meanwhile, we have fixed cover fraction for PFTs in our model which may also contribute to the disagreement of predicted and observed warming responses, while Norby et al. (2019) showed that the fractional cover of different Sphagnum species declined with warming.
Sphagnum mosses are sitting on top of high CO2 sources. CH4 can be a significant carbon source of submerged Sphagnum (Raghoebarsing et al., 2005; Larmola et al., 2014); the refixation of CO2 derived from decomposition processes is also an important source of carbon for Sphagnum (Rydin and Clymo, 1989; Turetsky and Wieder, 1999). The effects of the elevation of atmospheric CO2 on Sphagnum moss are currently disputed, with studies indicating an increase in growth rate (Jauhiainen and Silvde 1999; Heijmans et al., 2001; Saarnio et al., 2003), decreases in growth rate (Grosvernier et al., 2001; Fenner et al., 2007), and no response (Van der Hejiden et al., 2000; Hoosbeek et al., 2002; Toet et al., 2006). Norby et al. (2019) indicated no growth stimulation of both hummock and hollow Sphagnum under elevated CO2 condition but significant negative effects of elevated CO2 on Sphagnum NPP in the year 2018 at the same study site. Contrasting responses between Sphagnum species are thought to be coupled with the water availability. In contrast, our model results showed that both hummock and hollow Sphagnum growths were stimulated by the elevated CO2 concentration, which may be attributed to the fact that we did not consider the light competition between the PFTS (shrub and tree shading effects) but used a fixed cover fraction of Sphagnum.
The CO2 vertical concentration profile is assumed to be uniform in the simulations. In the experiment, the enclosure's regulated additions of pure CO2 are distributed to a manifold that splits the gas into four equal streams feeding each of the four air handling units (Hanson et al., 2017, Fig. 2a) and injects it into the duct work of each furnace just ahead of each blower and heat exchanger. Horizontal and vertical mixing within each enclosure homogenizes the air volume distributing the CO2 along with the heated air. The horizontal blowers in the enclosures together with external wind eddies ensure vertical mixing. We do not have routine automated CO2 concentration data below 0.5 m. The moss layer may well be experiencing higher concentrations than assumed by the model, but such an impact will be minimized during daylight hours. Preliminary isotopic measurements imply that a significant fraction of carbon assimilated by the moss may come from subsurface-respired CO2 (i.e., CO2 with older 14C signatures predating bomb carbon that can only be sourced from deeper peat; Hanson et al., 2017). However, the observed elevated CO2 response is smaller than simulated (Hanson et al., 2020). Understanding the drivers of elevated CO2 response or lack thereof is a key topic for future work.
To better investigate the Sphagnum warming and elevated CO2 responses, we should also focus on revealing the interactions with shrub and nitrogen availability (Norby et al., 2019). Nitrogen (N2) fixation is a major source of available N in ecosystems that receive low amounts of atmospheric N deposition, like boreal forests and subarctic tundra (Lindo et al., 2013; Weston et al., 2015; Rousk and Michelsen, 2016; Kostka et al., 2016). For example, diazotrophs are estimated to supply 40 %–60 % of N input to peatlands (Vile et at., 2014) with a high accumulation of fixed N in plant biomass (Berg et al., 2013). Nevertheless, N2 fixation is an energy-expensive process and is inhibited when N availability and reactive nitrogen deposition are high (Gundale et al., 2011; Ackermann et al., 2012; Rousk et al., 2013). This could limit ecosystem N input via the N2 fixation pathway. We are measuring Sphagnum-associated N2 fixation at the SPRUCE site and found that rates decline with increasing temperature (Carrell et al., 2019; Global Change Biology). We are continuing these measurements to see if they correlate with the GPP empirical relationship from Cleveland et al. (1999) or if temperature disrupts that association. Once finished, results will be used to represent N fixation by the Sphagnum layer and testing with measurements.
It is also encouraging that while we did not use leaf-level gas exchange observations in our optimization, the increased maintenance respiration base rate and temperature sensitivity compared to default (Table 2) are largely consistent with pretreatment leaf level observations (Jensen et al., 2018). In the future, a multiscale optimization framework that can assimilate leaf- and plot-level observations simultaneously should lead to improved model predictions and reduced uncertainties for the treatment simulations. If similar patterns observed in ambient conditions continue during the treatments, incorporating seasonal variations in leaf photosynthetic parameters may also further improve the simulated response to warming (Jensen et al., 2019).
Overall, while the sensitivity analysis is useful to indicate the key parameters and mechanisms responsible for uncertainty, our ability to quantify prediction uncertainty is limited because we consider only a single simulation with optimized parameters. Ideally, we should perform a model ensemble that represents the full range of posterior uncertainty over simulations that are consistent with the pretreatment observations and also a range of possible future meteorological conditions. This is currently being done for SPRUCE with the TECO carbon cycle model (Jiang et al., 2018), but the computational expense of ELM_SPRUCE currently prohibits this approach. By combining new surrogate modeling approaches (e.g., Lu and Ricciuto, 2019) with Markov chain Monte Carlo (MCMC) techniques, it may be possible to achieve this in the near future. This will help to reduce prediction uncertainties which currently prevail in the future carbon budget of peatlands and its feedback to climate change (McGuire et al., 2009).
The algorithms used to represent moss (Williams and Flanagan, 1998) are transferable to and have been applied by other modeling groups in other peatlands. However, we expect that certain parameters will vary, for example, the microtopographic parameters, the relationship between peat moisture and internal water content, and moss properties such as the C : N ratio. The parameter sensitivity analysis informs us as to the most important parameters responsible for prediction uncertainty and can inform us how to prioritize these measurements. Collecting these measurements from a variety of sites will be a necessary preliminary exercise. In addition to the simulations aimed at improved understanding of bog response to experimental manipulations at the plot scale, we are pursuing model implementations at larger spatial scales. The model framework described in this study is capable of performing regional simulations, although the current simulations were designed for the mechanistic understanding of Sphagnum mosses' hydrological and physiological dynamics at the plot level.
In this study, we reported the development of a Sphagnum moss PFT and associated processes within the ELM_SPRUCE model. Before being used to examine the ecosystem response to warming and elevated CO2 at a temperate bog ecosystem, the updated model was evaluated against the observed Sphagnum GPP and annual NPP, aboveground tree biomass, and shrub stem biomass. The new model can capture the seasonal dynamics of moss Sphagnum GPP but with lower peak GPP compared to site-level observations, and it can predict reasonable annual values for Sphagnum NPP but with lower interannual variation. Our model largely agrees with observed tree and shrub biomass. The model predicts that different PFTs responded differently to warming levels under both ambient and elevated CO2 concentration conditions. The NPP of the two dominant tree PFTs (black spruce and Larix) showed contrasting responses to warming scenarios (increasing with warming for Larix but decreasing for black spruce), while shrub NPP had a similar warming response to Larix. Hummock and hollow Sphagnum showed opposite warming responses: hollow Sphagnum shows generally higher growth with warming, but the hummock Sphagnum demonstrates more variability and strong dependence on water table height. The ELM predictions further suggest that the effects of CO2 fertilization can change the direction of the warming response for the bog peatland ecosystem, although observations of Sphagnum species at the site does not yet appear to support this (Norby et al., 2019).
The model code we used is available here: https://doi.org/10.5281/zenodo.3733924 (Ricciuto et al., 2020). The datasets and scripts used for the figures are here: https://doi.org/10.5281/zenodo.4439419 (Shi and Ricciuto, 2021).
The supplement related to this article is available online at: https://doi.org/10.5194/bg-18-467-2021-supplement.
XS, DMR, and PET designed the research. XS performed model experiments and analyzed the data, and LM and JM helped with analyzing the data and plotting the figures. FY and XX contributed some good ideas for model implements. RJN, APW, JMW, PJH, NAG, and DW provided the field-observed data. XS drafted the paper, and all authors contributed to writing the paper.
The authors declare that they have no conflict of interest.
The authors would like to thank the two reviewers for their very nice comments.
This research has been supported by the US Department of Energy, Office of Science, and Biological and Environmental Research Program. The Oak Ridge National Laboratory is managed by UT-Battelle, LLC, for the US Department of Energy under contract DE-AC05–00OR22725.
This paper was edited by Sebastiaan Luyssaert and reviewed by Samuli Launiainen and one anonymous referee.
Ackermann, K., Zackrisson, O., Rousk, J., Jones, D. L., and DeLuca, T. H.: N2 fixation in feather mosses is a sensitive indicator of N deposition in boreal forests, Ecosystems, 15, 986–998, 2012.
Barber, V. A., Juday, G. P., and Finney, B. P.: Reduced growth of Alaskan white spruce in the twentieth century from temperature-induced drought stress, Nature, 405, 668–673, 2000.
Berg, A., Danielsson, A., and Sevensson, B. H.: Transfer of fixed-N from N2-fixing cyanobacteria associated with moss sphagnum riparium results in enhanced growth of the moss, Plant Soil, 362, 271–278, https://doi.org/10.1007/s11104-012-1278-4, 2013.
Beringer, J., Lynch, A., Chapin, F., Mack, M., and Bonan, G.: The Representation of Arctic Soils in the Land Surface Model: The Importance of Mosses, J. Climate, 14, 3324–3335, https://doi.org/10.1175/1520-0442(2001)014<3324:TROASI>2.0.CO;2, 2001.
Blok, D., Heijmans, M., Schaepman-Strub, G., Van Ruijven, J., Parmentier, F., Maximov, T., and Berendse, F.: The cooling capacity of mosses: Controls on water and energy fluxes in a Siberian tundra site, Ecosystems, 14, 1055–1065, 2011.
Bond-Lamberty, B., Peckham, S. D., Ahl, D. E., and Gower, S. T.: Fire as the dominant driver of central Canadian boreal forest carbon balance, Nature, 450, 89–92, 2007.
Bragazza, L., Buttler, A., Robroek, B. J., Albrecht, R., Zaccone, C., Jassey, V. E., and Signarbieux, C.: Persistent high temperature and low precipitation reduce peat carbon accumulation, Glob. Change Biol., 22, 4114–4123, https://doi.org/10.1111/gcb.13319, 2016.
Breeuwer, A., Heijmans, M. M., Robroek, B. J., and Berendse, F.: The effect of temperature on growth and competition between Sphagnum species, Oecologia, 156, 155–167, https://doi.org/10.1007/s00442-008-0963-8, 2008.
Brown, S. M., Petrone, R. M., Mendoza, C., and Devito, K. J.: Surface vegetation controls on evapotranspiration from a sub-humid Western Boreal Plain wetland, Hydrol. Process., 24, 1072–1085, 2010.
Burrows, S. M., Maltrud, M. E., Yang, X., Zhu, Q., Jeffery, N., Shi., X., Ricciuto, D. M., Wang, S., Bisht, G., Tang, J., Wolfe, J. D., Harrop, B. E., Singh, B., Brent, L., Zhou, Tian, Cameron-Smith P. J., Keen, N., Collier, N., Xu, M., Hunke, E. C., Elliott, S. M., Turner, A. K., Li, H., Wang, H., Golaz, J.-C., Bond-Lamberty, B., Hoffman, F. M., Riley, W. J., Thornton, P. E., Calvin, K., and Leung, L. R.: The DOE E3SM coupled model v1.1 biogeochemistry configuration: overview and evaluation of coupled carbon-climate experiments, J. Adv. Model Earth Sy., 12, e2019MS001766, https://doi.org/10.1029/2019MS001766, 2020.
Busby, J. R., Bliss, L. C., and Hamilton, C. D.: Microclimate control of growth rates and habitats of the Boreal Forest Mosses, Tomenthypnum nitens and Hylocomium splenden, Ecol. Monogr., 48, 95–110, 1978.
Carrell, A. A., Kolton, M., Warren, M. J., Kostka, J. E., and Weston, D. J.: Experimental warming alters the community composition, diversity, and N2 fixation activity of peat moss (Sphagnum fallax) microbiomes, Glob. Change Biol., 25, 2993–3004, dio:10.1111/gcb.14715, 2019.
Chadburn, S., Burke, E., Essery, R., Boike, J., Langer, M., Heikenfeld, M., Cox, P., and Friedlingstein, P.: An improved representation of physical permafrost dynamics in the JULES land-surface model, Geosci. Model Dev., 8, 1493–1508, https://doi.org/10.5194/gmd-8-1493-2015, 2015.
Chapin III, F. S., Shaver, G. R., Giblin, A. E., Nadelhoffer, K. J., and Laundre, J. A.: Responses of Arctic tundra to experimental and observed changes in climate, Ecology, 76, 694–711, 1995.
Chavardes, R. D., Daniels, L. D., Waeber P. O., Innes, J. L., and Nitschke, C. R.: Unstable climate-growth relations for white spruce in southwest Yukon, Canada, Climatic Change, 116, 593–611, 2013.
Chen, W. J., Black, T. A., Yang, P. C. Barr, A. G. Neumann, H. H., Nešić, Z., Blanken, P. D. Novak, M. D., Eley, J., Ketler, R., and Cuenca, R. H.: Effects of climatic variability on the annual carbon sequestration by a boreal aspen forest, Glob. Change Biol., 5, 41–53, 1999.
Cleveland, C. C., Townsend, A. R., Schimel, D. S., Fisher, H., Howarth, Lars O. H., Perakis, S. S., Latty, E. F., Von Fishcher, J. C., Elseroad, A., and Wasson, M. F.: Global patterns of terrestrial biological nitrogen (N2) fixation in natural ecosystem, Global Biogeochem. Cy., 13, 623–645, 1999.
Clymo, R. S. and Hayward, P. M.: The ecology of Sphagnum, in: Bryophyte Ecology, edited by: Smith, A. I. E., Chapman and Hall Ltd., London, UK, New York, USA, 229–289, 1982.
Collatz, G. J., Ball, J. T., Grivet, C., and Berry, J. A.: Physiological and environmental-regulation of stomatal conductance, photosynthesis and transpiration – a model that includes a laminar boundary-layer, Agr. For. Meteorol., 54, 107–136,1991.
Collatz, G. J., Ribas-Carbo, M., and Berry, J. A.: Coupled photosynthesis- stomatal model for leaves of C4 plants, Austr. J. Plant Physiol., 19, 519–538, 1992.
Cornelissen, H. C., Lang, S. I., Soudzilovskaia, N. A., and During, H. J.: Comparative cryptogam ecology: a review of bryophyte and lichen traits that drive biogeochemistry, Ann. Bot.-London, 99, 987–1001, 2007.
Druel, A., Peylin, P., Krinner, G., Ciais, P., Viovy, N., Peregon, A., Bastrikov, V., Kosykh, N., and Mironycheva-Tokareva, N.: Towards a more detailed representation of high-latitude vegetation in the global land surface model ORCHIDEE (ORC-HL-VEGv1.0), Geosci. Model Dev., 10, 4693–4722, https://doi.org/10.5194/gmd-10-4693-2017, 2017.
Duarte, H. F., Raczka, B. M., Ricciuto, D. M., Lin, J. C., Koven, C. D., Thornton, P. E., Bowling, D. R., Lai, C.-T., Bible, K. J., and Ehleringer, J. R.: Evaluating the Community Land Model (CLM4.5) at a coniferous forest site in northwestern United States using flux and carbon-isotope measurements, Biogeosciences, 14, 4315–4340, https://doi.org/10.5194/bg-14-4315-2017, 2017.
Euskirchen, E. S., McGuire, A. D., Chapin III, F. S., Yi, S., and Thompson, C. C.: Changes in vegetation in northern Alaska under scenarios of climate change, 2003–2100: implications for climate feedbacks, Ecol. Appl., 19, 1022–1043, 2009.
Farquhar, G. D., von Caemmerer, S., and Berry, J. A.: A biochemical model of photosynthetic CO2 assimilation in leaves of C3 species, Planta, 149, 78–90, 1980.
Fenner, N., Ostle, N. J., Mcnamara, N., Sparks, T., Harmens, H., Reynolds, B., and Freeman, C.: Elevated CO2 effects on peatland plant community carbon dynamics and DOC production, Ecosystem, 10, 635–647, 2007.
Frolking, S. and Roulet, N. T.: Holocene radiative forcing impact of northern peatland carbon accumulation and methane emissions, Glob. Change Biol., 13, 1079–1088, 2007.
Frolking, S., Roulet, N. T., Tuittila, E., Bubier, J. L., Quillet, A., Talbot, J., and Richard, P. J. H.: A new model of Holocene peatland net primary production, decomposition, water balance, and peat accumulation, Earth Syst. Dynam., 1, 1–21, https://doi.org/10.5194/esd-1-1-2010, 2010.
Girardin, M. P., Bouriaud, O., Hogg, E. H., Kurz, W., Zimmermann, N. E., Metsaranta, J. M., de Jong, R., Frank, D. C., Esper, J., Büntgen, U., Guo, X., and Bhatti, J.: No growth stimulation of Canada's boreal forest under half-century of combined warming and CO2 fertilization, P. Natl. Acad. Sci. USA, 113, E8406–E8414, 2016.
Goetz, J. D. and Price, J. S.: Role of morphological structure and layering of Sphagnum and Tomenthypnum mosses on moss productivity and evaporation rates, Can. J. Soil Sci., 95, 109–124, https://doi.org/10.4141/CJSS-2014-092, 2015.
Golaz, J.-C., Caldwell, P. M., Van Roekel, L. P., Petersen, M. R., Tang, Q., Wolfe, J. D., Abeshu, G., Anantharaj, V., Asay-Davis, X. S., Bader, D. C., Baldwin, S. A., Bisht, G., Bogenschutz, P. A., Branstetter, M., Brunke, M., A., Brus, S. R., Burrows, S. M., Cameron-Smith, P. J., Donahue, A. S., Deakin, M., Easter, R. C., Evans, K. J., Feng, Y., Flanner, M., Foucar, J., G., Fyke, J. G., Griffin, B. M., Hannay, C., Harrop, B. E., Hoffman, M. J., Hunke, E. C., Jacob, R. L., Jacobsen, D. W., Jeffery, N., Jones, P. W., Klein, S. A., Larson, V. E., Leung, L. R., Li, H., Lin, W., Lipscomb, W. H., Ma, P.-L., Mahajan, S., Maltrud, M., E., Mametjanov, A., McClean, J. L., McCoy, R. B., Neale, R. B., Price, S. F., Qian, Y., Rasch, P. J., Reeves Eyre, J. E. J., Riley, W. J., Ringler, T. D., Roberts, A. F., Roesler, E. L., Salinger, A. G., Shaheen, Z., Shi, X., Singh, B., Tang, J., Taylor, M. A., Thornton, P. E., Tuner, A. K., Veneziani, M., Wan, H., Wang, H., Wang, S., Williams, D. N., Wolfram, P. J., Worley, P. H., Xie, S., Yang, Y., Yoon, J.-H., Zelinka, M. D., Zender, C. S., Zeng, X., Zhang, C., Zhang, K., Zhang, Y., Zheng, X., Zhou, T., and Zhu, Q.: The DOE E3SM coupled model version 1: Overview and evaluation at standard resolution, J. Adv. Model Earth Sy., 11, 2089–2129, https://doi.org/10.1029/2018MS001603, 2019.
Gorham, E.: Northern peatlands: role in the carbon cycle and probable responses to climatic warming, Ecol. Appl., 1, 182–195, 1991.
Granath, G., Limpens, J., Posch, M., Mücher, S., and De Vries, W.: Spatio-temporal trends of nitrogen deposition and climate effects on Sphagnum productivity in European peatlands, Environ. Poll., 187, 73–80, https://doi.org/10.1016/j.envpol.2013.12.023, 2014.
Grant, R. F., Desai, A. R., and Sulman, B. N.: Modelling contrasting responses of wetland productivity to changes in water table depth, Biogeosciences, 9, 4215–4231, https://doi.org/10.5194/bg-9-4215-2012, 2012.
Griffiths, N. A. and Sebestyen, S. D.: Dynamic vertical profiles of peat porewater chemistry in a northern peatland, Wetland, 36, 1119-1130, https://doi.org/10.1007/s13157-016-0829-5, 2016.
Griffiths, N. A., Hanson, P. J., Ricciuto, Iversen, C. M., Jensen, A. M., Malhotra, A., McFarlane, K. J., Norby, R. J., Sargsyan, K., Sebestyen, S. D., Shi, X., Walker, A. P., Ward, E. J., Warren, J. M., and Weston, D, J.: Temporal and spatial variation in peatland carbon cycling and implications for interpreting responses of an ecosystem-scale warming experiment, Soil Sci. Soc. Am. J., 81, 1668–1688, https://doi.org/10.2136/sssaj2016.12.0422, 2018.
Grosvernier, P., Matthey, Y., and Buttler, A.: Growth potential of three Sphagnum species in relation to water table level and peat properties with implications for their restoration in cut-over bogs, J. Appl. Ecol., 34, 471–483, https://doi.org/10.2307/2404891, 1997.
Grosvernier, P. R., Mitchell, E. A. D., Buttler, A., and Gobat, J. M.: Effects of elevated CO2 and nitrogen deposition on natural regeneration processes of cut-over ombrotrophic peat bogs in the Swiss Jura mountains, Glob. Change Prot. Areas, 9, 347–35, 2001.
Gundale, M. J., DeLuca, T. H., and Nordin, A.: Bryophytes attenuate anthropogenic nitrogen inputs in boreal forests, Glob. Change Biol., 17, 2743–2753, 2011.
Gunnarsson, U., Granberg, G., and Nilsson, M.: Growth, production and interspecific competition in Sphagnum: effects of temperature, nitrogen and sulphur treatments on a boreal mire, New Phytol., 163, 349–359, https://doi.org/10.1111/j.1469-8137.2004.01108.x, 2004.
Hanson, P. J., Riggs, J. S., Nettles, W. R., Krassovski, M. B., and Hook L. A.: SPRUCE deep peat heating (DPH) environmental data, February 2014 through July 2015, Oak Ridge National Laboratory, TES SFA, U.S. Department of Energy, Oak Ridge, Tennessee, USA, https://doi.org/10.3334/CDIAC/spruce.013, 2015a.
Hanson, P. J., Riggs, J. S. Dorrance, C., Nettles, W. R., and Hook, L. A.: SPRUCE Environmental Monitoring Data: 2010-2016. Carbon Dioxide Information Analysis Center, Oak Ridge National Laboratory, U.S. Department of Energy, Oak Ridge, Tennessee, USA, https://doi.org/10.3334/CDIAC/spruce.001, 2015b.
Hanson, P. J., Riggs, J. S., Nettles, W. R., Phillips, J. R., Krassovski, M. B., Hook, L. A., Gu, L., Richardson, A. D., Aubrecht, D. M., Ricciuto, D. M., Warren, J. M., and Barbier, C.: Attaining whole-ecosystem warming using air and deep-soil heating methods with an elevated CO2 atmosphere, Biogeosciences, 14, 861–883, https://doi.org/10.5194/bg-14-861-2017, 2017.
Hanson, P. J., Phillips, J. R., Wullschelger, S. D., Nettles, W. R., Warren, J. M., Ward, E. J.: SPRUCE Tree Growth Assessments of Picea and Larix in S1-Bog Plots and SPRUCE Experimental Plots beginning in 2011, Oak Ridge National Laboratory, TES SFA, U.S. Department of Energy, Oak Ridge, Tennessee, USA, https://doi.org/10.25581/spruce.051/1433836, 2018a.
Hanson, P. J., Phillips, J. R., Brice, D. J., and Hook, L. A.: SPRUCE Shrub-Layer Growth Assessments in S1-Bog Plots and SPRUCE Experimental Plots beginning in 2010, Oak Ridge National Laboratory, TES SFA, U.S. Department of Energy, Oak Ridge, Tennessee, USA, https://doi.org/10.25581/spruce.052/1433837, 2018b.
Hanson, P. J., Griffiths, N. A., Iversen, C. M., Norby, R. J., Sebestyen, S. D., Phillips, J. R., Chanton, P., Kolka, R. K., Malhotra, A., Oleheiser, K. C., Warren, J. M., Shi, X., Yang, X., Mao, J., and Ricciuto, D. M.: Rapid net carbon loss from a whole-ecosystem warmed peatland, AGU Adv., 1, e2020AV000163, https://doi.org/10.1029/2020AV000163, 2020.
Heijmans, M., Arp, W. J., and Berendse, F.: Effects of elevated CO2 and vascular plants on evapotranspiration in bog vegetation, Glob. Change Biol., 7, 817–827, 2001.
Heijmans, M. M. P. D., Arp, W. J., and Chapin III, F. S.: Carbon dioxide and water vapour exchange from understory species in boreal forest, Agr. For. Meteorol., 123, 135–147, https://doi.org/10.1016/j.agrformet.2003.12.006, 2004a.
Heijmans, M. M. P. D., Arp, W. J., and Chapin III, F. S.: Controls on moss evaporation in a boreal black spruce forest, Glob. Biogeochem. Cy., 18, 1–8, https://doi.org/10.1029/2003GB002128, 2004b.
Heijmans, M. M. P. D., Mauquoy, D., van Geel, B., and Berendse, F.: Long-term effects of climate change on vegetation and carbon dynamics in peat bogs, J. Veg. Sci., 19, 307–320, 2008.
Hobbie, S. E.: Temperature and plant species control over litter decomposition in Alaskan tundra, Ecol. Appl., 66, 503–522, 1996.
Hobbie, S. E. and Chapin III, F. S.: The response of tundra plant biomass, aboveground production, nitrogen, and CO2 flux to experimental warming, Ecology, 79, 1526–1544, 1998.
Hobbie, S. E., Shevtsova, A., and Chapin III, F. S.: Plant responses to species removal and experimental warming in Alaskan Tussock Tundra, Oikos 84, 417–434, 1999.
Hoosbeek, M. R., Van Breemen, N., Vasander, H., Buttler, A., and Berendse, F.: Potassium limits potential growth of bog vegetation under elevated atmospheric CO2 and N deposition, Glob. Change Biol., 8, 1130–1138, https://doi.org/10.1046/j.1365-2486.2002.00535.x, 2002.
Jauhiainen, J. and Silvola, J.: Photosynthesis of Sphagnum fuscum at long-term raised CO2 concentrations, Ann. Bot. Fenn., 36, 11–19, 1999.
Jensen, A. M., Warren, J. M., Hook, L. A., Wullschleger, S. D., Brice, D. J., Childs, J., and Vander Stel, H. M.: SPRUCE S1 Bog Pretreatment Seasonal Photosynthesis and Respiration of Trees, Shrubs, and Herbaceous Plants, 2010–2015, Oak Ridge National Laboratory, TES SFA, U.S. Department of Energy, Oak Ridge, Tennessee, USA, https://doi.org/10.3334/CDIAC/spruce.008, 2018.
Jensen, A. M., Warren, J. M., King, A., Ricciuto, D. M., Hanson, P. J., and Wullschleger, S. D.: Simulated projections of boreal forest peatland ecosystem productivity are sensitive to observed seasonality in leaf phenology, Tree Physiol., 39, 556–572, https://doi.org/10.1093/treephys/tpy140, 2019.
Jiang, J., Huang, Y., Ma, S., Stacy, M., Shi, Z., Ricciuto, D. M., Hanson, P. J., and Luo, Y.: Forecasting Responses of a Northern Peatland Carbon Cycle to Elevated CO2 and a Gradient of Experimental Warming, J. Geophys. Res.-Biogeo., 123, 1057–1071, https://doi.org/10.1002/2017JG004040, 2018.
Juday, G. P. and Alix, C.: Consistent negative temperature sensitivity and positive influence of precipitation on growth of floodplain Picea glaucain Interior Alaska, Can. J. For. Res., 42, 561–573, 2012.
Kostka, J. E., Weston, D. J., Glass, J. B., Lilleskov, E. A., Shaw, A. J., and Turetsky, M. R.: The Sphagnum microbiome: new insights from an ancient plant lineage, New Phytol., 211, 57–64, 2016.
Kuiper, J. J., Mooij, W. M., Bragazza, L., and Robroek, B. J.: Plant functional types define magnitude of drought response in peatland CO2 exchange, Ecology, 95, 123–131, https://doi.org/10.1890/13-0270.1, 2014.
Lafleur, P. M., Hember, R. A., Admiral, S. W., and Roulet, N. T.: Annual and seasonal variability in evapotranspiration and water table at a shrub-covered bog in southern Ontario, Canada, Hydrol. Process., 19, 3533–3550, https://doi.org/10.1002/hyp.5842, 2005.
Larmola, T., Leppänen, S. M., Tuittila, E.-S., Aarva, M., Merilä, P., Fritze, H., and Tiirola, M.: Methanotrophy induces nitrogen fixation during peatland development, P. Natl. Acad. Sci. USA, 111, 734–739, https://doi.org/10.1073/pnas.1314284111, 2014.
Launiainen, S., Katul, G. G., Lauren, A., and Kolari, P.: Coupling boreal forest CO2, H2O and energy flow by a vertically structured forest canopy-Soil model with separate bryophyte layer, Ecol. Model., 312, 385–405. https://doi.org/10.1016/j.ecolmodel.2015.06.007, 2015.
Lindo, Z. and Gonzalez, A.: The bryosphere: an integral and influential component of the earth's biosphere, Ecosystems, 13, 612–627, 2010.
Lindo, Z., Nilsson, M. C., and Gundale, M. J.: Bryophyte-cyanobacteria associations as regulators of the northern latitude carbon balance in response to global change, Glob. Change Biol., 19, 2022–2035, 2013.
Lu, D. and Ricciuto, D.: Efficient surrogate modeling methods for large-scale Earth system models based on machine-learning techniques, Geosci. Model Dev., 12, 1791–1807, https://doi.org/10.5194/gmd-12-1791-2019, 2019.
Lu, D., Ricciuto, D. M., Stoyanov, M., and Gu, L.: Calibration of the E3SM Land Model Using Surrogate-Based Global Optimization, J. Adv. Model. Earth Sy., 10, 1337–1356, https://doi.org/10.1002/2017ms001134, 2018.
Man, R., Kayahara, G. J., Rice, J. A., and MacDonald, G. B.: Eleven-year responses of a boreal mixedwood stand to partial harvesting: light, vegetation, and regeneration dynamics, For. Ecol. Manag., 255, 697–706, 2008.
Mazziotta, A., Granath, G., Rydin, H., and Bengtsson F.: Scaling functional traits to ecosystem processes: Towards a mechanistic understanding in peat mosses, J. Ecol., 107, 843–859, https://doi.org/10.1111/1365-2745.13110, 2018.
McFadden, J. P., Eugster, W., and Chapin III, F. S.: A regional study of the controls on water vapor and CO2 exchange in Arctic tundra, Ecology, 84, 2762–2776, 2003.
McGuire, A. D., Anderson, L. G., Christensen, T. R., Dallimore, S., Guo, L., Hayes, D. J., Heimann, M., Lorenson, T. D., Macdonald, R. W., and Roulet, N.: Sensitivity of the carbon cycle in the Arctic to climate change, Ecol. Monogr., 79, 523–555, 2009.
McPartland, M. Y., Montgomery, R. A., Hanson, P. J., Phillips, J. R., Kolka, R., and Palik, B.: Vascular plant species response to warming and elevated carbon dioxide in a boreal peatland, Environ. Res. Lett., 15, 124066, https://doi.org/10.1088/1748-9326/abc4fb, 2020.
Metcalfe, D. B., Ricciuto D. M., Palmroth, S., Campbell, Hurry, C. V., Mao, J., Keel, S. G., Linder, S., Shi, X., Näsholm, T., Ohlsson, K. E. A., Blackburn, M., Thornton, P. E., and Oren, R.: Informing climate models with rapid chamber measurements of forest carbon uptake, Glob. Change Biol., 23, 2130–2139, https://doi.org/10.1111/gcb.13451, 2017.
Miller, P. A. and Smith, B.: Modelling Tundra Vegetation Response to Recent Arctic Warming, Ambio, 41, 281–291, https://doi.org/10.1007/s13280-012-0306-1, 2012.
Mokhov, I. I., Eliseev, A. V., and Denisov, S. N.: Model diagnostics of variations in methane emissions by wetlands in the second half of the 20th century based on reanalysis data, Dokl. Earth Sci., 417, 1293–1297, 2007.
Moore, T. R., Roulet, N. T., and Waddington, J. M.: Uncertainty in predicting the effect of climate change on the carbon cycling of Canadian peatlands, Clim. Change, 40, 229–245, 1998.
Murray, K. J., Tenhunen, J. D., and Nowak, R. S.: Photoinhibition as a control on photosynthesis and production of Sphagnum mosses, Oecologia, 96, 200–207, 1993.
Myneni, R. B., Keeling, C. D., Tucker, C. J., Asrar, G., and Nemani, R. R.: Increased plant growth in the northern high latitudes from 1981 to 1991, Nature, 386, 698–702, 1997.
Nichols, J. E. and Peteet, D. M.: Rapid expansion of northern peatlands and doubled estimate of carbon storage, Nat. Geosci. 12, 917–921, https://doi.org/10.1038/s41561-019-0454-z, 2019.
Nilsson, M. C. and Wardle, D. A.: Understory vegetation as a forest ecosystem driver: evidence from the northern Swedish boreal forest, Front. Ecol. Environ., 3, 421–428, 2005.
Norby, R. J. and Childs, J.: SPRUCE: Sphagnum Productivity and Community Composition in the SPRUCE Experimental Plots, Oak Ridge National Laboratory, TES SFA, U.S. Department of Energy, Oak Ridge, Tennessee, USA, https://doi.org/10.25581/spruce.049/1426474, 2018.
Norby, R. J., Childs, J., Hanson, P. J., and Warren, J. M..: Rapid loss of an ecosystem engineer: Sphagnum decline in an experimentally warmed bog, Ecol. Evol., 9, 12571–12585, https://doi.org/10.1002/ece3.5722, 2019.
Nungesser, M. K.: Modelling microtopography in boreal peatlands: Hummocks and hollows, Ecol. Model., 165, 175–207, 2003.
Oechel, W. C. and Van Cleve, K.: The role of bryophytes in nutrient cycling in the taiga, in: Ecological Studies, Vol. 57: Forest Ecosystems in the Alaskan Taiga, edited by: Van Cleve, K., Chapin III, F. S., Flanagan, P. W., Viereck, L. A., and Dyrness, C. T., Springer, New York, USA, 121–137, 1986.
Oleson, K. W., Lawrence, D. W., Bonan, G. B., Drewniak, B., Huang, M., Koven, C. D., Levis, S., Li, F., Riley, W. J., Subin, Z. M., Swenson, S. C., Thornton, P. E., Bozbiyik, A., Fisher, R., Heald, C. L., Kluzek, E., Lamarque, J., Lawrence, P. J., Leung, L. R., Lipscomb, W., Muszala, S., Ricciuto, D. M., Sacks, W., Sun,Y., Tang, J., and Yang, Z.: Technical description of version 4.5 of the Community Land Model (CLM), NCAR/TN-503+STR, NCAR Technical Note, https://doi.org/10.5065/D6RR1W7M, 2013.
Park, H., Launiainen, S., Konstantinov, P. Y., Iijima, Y., and Fedorov, A. N.: Modeling the effect of moss cover on soil temperature and carbon fluxes at a tundra site in northeastern Siberia, J. Geophys. Res.-Biogeo., 123, 3028–3044, https://doi.org/10.1029/2018JG004491, 2018.
Parsekian, A. D., Slater, L., Ntarlagiannis, D., Nolan, J., S. Sebestyen, D., Kolka, R. K., and Hanson, P. J.: Uncertainty in peat volume and soil carbon estimated using ground-penetrating radar and probing, Soil Sci. Soc. Am. J., 76, 1911–1918. https://doi.org/10.2136/sssaj2012.0040, 2012.
Pastor, J., Peckham, B., Bridgham, S., Weltzin, J., and Chen, J.: Plant community dynamics, nutrient cycling, and alternative stable equilibria in peatlands, Am. Nat., 160, 553–568, 2002.
Petrone, R., Solondz, D., Macrae, M., Gignac, D., and Devito, K. J.: Microtopographical and canopy cover controls on moss carbon dioxide exchange in a western boreal plain peatland, Ecohydrology, 4, 115–129, 2011.
Porada, P., Weber, B., Elbert, W., Pöschl, U., and Kleidon, A.: Estimating global carbon uptake by lichens and bryophytes with a process-based model, Biogeosciences, 10, 6989–7033, https://doi.org/10.5194/bg-10-6989-2013, 2013.
Porada, P., Ekici, A., and Beer, C.: Effects of bryophyte and lichen cover on permafrost soil temperature at large scale, The Cryosphere, 10, 2291–2315, https://doi.org/10.5194/tc-10-2291-2016, 2016.
Raczka, B., Duarte, H. F., Koven, C. D., Ricciuto, D., Thornton, P. E., Lin, J. C., and Bowling, D. R.: An observational constraint on stomatal function in forests: evaluating coupled carbon and water vapor exchange with carbon isotopes in the Community Land Model (CLM4.5), Biogeosciences, 13, 5183–5204, https://doi.org/10.5194/bg-13-5183-2016, 2016.
Raghoebarsing, A. A., Smolders, A. J. P., Schmid, M. C., Rijpstra, W. I. C., Wolters-Arts, M., Derksen, J., Jetten, M. S. M., Schouten, S., Sinninghe Damsté, J. S., Lamers, L. P. M., Roelofs, J. G. M., Op den Camp, H. J. M., and Strous, M.: Methanotrophic symbionts provide carbon for photosynthesis in peat bogs, Nature, 436, 1153–1156, https://doi.org/10.1038/nature03802, 2005.
Ricciuto, D. M., Sargsyan, K., and Thornton, P. E.: The Impact of Parametric Uncertainties on Biogeochemistry in the E3SM Land Model, J. Adv. Model Earth Sy., 10, 297–319, https://doi.org/10.1002/2017ms000962, 2018.
Ricciuto, D. M., Xu, X., and Shi, X.: dmricciuto/CLM_SPRUCE: Release of CLM_SPRUCE (Version v1.0.0), Zenodo, https://doi.org/10.5281/zenodo.3733924, 2020.
Riutta, T., Laine, J., and Tuittila, E.-S.: Sensitivity of CO2 exchange of fen ecosystem componetns to water level variation, Ecosystem, 10, 718–733, 2007.
Robroek, B. J. M., Limpens, J., Breeuwer, A., and Schouten, M. G. C.: Effects of water level and temperature on performance of four Sphagnum mosses, Plant Ecol., 190, 97–107, 2007.
Robroek, B. J. M., Schouten, M. G. C., Limpens, J., Berendse F. and Poorter, H.: Interactive effects of water table and precipitation on net CO2 assimilation of three co-occurring Sphagnum mosses differing in distribution above the water table, Glob. Change Biol., 15, 680–691, 2009.
Rosenzweig, C., Karoly, D., Vicarelli, M., Neofotis, P., Wu, Q., Casassa, G., Menzel, A., Root, T., Estrella, N., Seguin, B., Tryjanowski, P., Liu, C., Ravlins, S., and Imeson, A.: Attributing physical and biological impacts to anthropogenic climate change, Nature, 453, 353–357, https://doi.org/10.1038/nature06937, 2008.
Rousk, K. and Michelsen, A.: The sensitivity of Moss-Associated Nitrogen Fixtion towards Repeated Nitrogen Input, Plos One, 11, e0146655, https://doi.org/10.1371/journal.pone.0146655, 2016.
Rousk, K., Rousk, J., Jones, D. L., Zackrisson, O., and DeLuca, T. H.: Feather moss nitrogen acquisition across natural fertility gradients in boreal forests, Soil Biol. Biochem., 61, 86–95, 2013.
Rydin, H.: Effect of water level on desiccation of Sphagnum in relation to surrounding Sphagna, Oikos, 45, 374–379, https://doi.org/10.2307/3565573, 1985.
Rydin, H. and Clymo, R. S.: Transport of carbon and phosphorus-compounds about Sphagnum, Proc. R. Soc. Ser. B-Bio., 237, 63–84, https://doi.org/10.1098/rspb.1989.0037, 1989.
Rydsaa, J. H., Stordal, F., Bryn, A., and Tallaksen, L. M.: Effects of shrub and tree cover increase on the near-surface atmosphere in northern Fennoscandia, Biogeosciences, 14, 4209–4227, https://doi.org/10.5194/bg-14-4209-2017, 2017.
Saarnio, S., Jarvio, S., Saarinen, T., Vasander, H., and Silvola, J.: Minor changes in vegetation and carbon gas balance in a boreal mire under a raised CO2 or NH4NO3 supply, Ecosystems 6, 46–60, https://doi.org/10.1007/s10021-002-0208-3, 2003.
Sargsyan, K., Safta, C., Najm, H. N., Debusschere, B. J., Ricciuto, D. M., and Thornton, P. E.: Dimensionality Reduction for Complex Models Via Bayesian Compressive Sensing, Int. J. Uncertain. Quan., 4, 63–93, https://doi.org/10.1615/Int.J.UncertaintyQuantification.2013006821, 2014.
Sebestyen, S. D., Dorrance, C., Olson, D. M., Verry, E. S., Kolka, R. K., Elling, A. E., and Kyllander, R.: Long-term monitoring sites and trends at the Marcell Experimental Forest, in: Peatland biogeochemistry and watershed hydrology at the Marcell Experimental Forest, edited by: Kolka, R. K., Sebestyen, S. D., Verry, E. S., and Brooks, K., CRC Press, New York, USA, 15–71, 2011.
Shi, X., Thornton, P. E., Ricciuto, D. M., Hanson, P. J., Mao, J., Sebestyen, S. D., Griffiths, N. A., and Bisht, G.: Representing northern peatland microtopography and hydrology within the Community Land Model, Biogeosciences, 12, 6463–6477, https://doi.org/10.5194/bg-12-6463-2015, 2015.
Shi, X. and Ricciuto, D.: dmricciuto/ELM_SPRUCE_scripts, Zenodo, https://doi.org/10.5281/zenodo.4439419, 2021.
Silva, L. C. R., Anand, M., and Leithead, M. D.: Recent widespread tree growth decline despite increasing atmospheric CO2, Plos One, 5, e11543, https://doi.org/10.1371/journal.pone.0011543, 2010.
Sonnentag, O., Van Der Kamp, G., Barr, A. G., and Chen, J.: on the relationship between water table depth and water vapor and carbon dioxide fluxes in a minerotrophic fen, Glob. Change Biol., 16, 1761–1776, https://doi.org/10.1111/j.1365-2486.2009.02032.x, 2010.
St-Hilaire, F., Wu, J., Roulet, N. T., Frolking, S., Lafleur, P. M., Humphreys, E. R., and Arora, V.: McGill wetland model: evaluation of a peatland carbon simulator developed for global assessments, Biogeosciences, 7, 3517–3530, https://doi.org/10.5194/bg-7-3517-2010, 2010.
Tenhunen, J. D., Weber, J. A., Yocum, C. S., and Gates, D. M.: Development of a photosynthesis model with an emphasis on ecological applications, Oecologia, 26, 101–119, 1976.
Tian, H., Lu, C., Yang, J., Banger, K., Huntinzger, D. N., Schwalm, C. R., Michalak, A. M., Cook, R., Ciais, P., Hayes, D., Huang, M., Ito, A., Jacobson, A., Jain, A., Lei, H., Mao, J., Pan, S., Post, W. M, Peng, S., Poulter, B., Ren, W., Ricciuto, D. M., Schaefer, K., Shi, X., Tao, B., Wang, W., Wei, Y., Yang, Q., Zhang, B., and Zeng, N.: Global patterns of soil carbon stocks and fluxes as simulated by multiple terrestrial biosphere models: sources and magnitude uncertainty, Glob. Biogeochem. Cy., 29, 775–792, https://doi.org/10.1002/2014GB005021, 2015.
Titus, J. E., Wagner, D. J., and Stephens, M. D.: Contrasting Water Relations of Photosynthesis for 2 Sphagnum Mosses, Ecology, 64, 1109–1115, 1983.
Todd-Brown, K. E. O., Randerson, J. T., Post, W. M., Hoffman, F. M., Tarnocai, C., Schuur, E. A. G., and Allison, S. D.: Causes of variation in soil carbon simulations from CMIP5 Earth system models and comparison with observations, Biogeosciences, 10, 1717–1736, https://doi.org/10.5194/bg-10-1717-2013, 2013.
Toet, S., Cornelissen, J. H., Aerts, R., van Logtestijn, R. S., de Beus, M., Stoevelaar, R.: Moss responses to elevated CO2 and variation in hydrology in a temperate lowland peatland, Plants and climate change, Springer, Netherlands, 27–42, 2006.
Turetsky, M. R. and Wieder, R. K.: Boreal bog Sphagnum refixes soil-produced and respired 14CO2, Ecoscience, 6, 587–591, https://doi.org/10.1080/11956860.1999.11682559, 1999.
Turetsky, M. R., Wieder, R. K., and Vitt, D. H.: Boreal peatland C fluxes under varying permafrost regimes, Soil Biol. Biochem., 34, 907–912, 2002.
Turetsky, M. R., Mack, M .C., Hollingsworth, T. N., and Harden, J. W.: The role of mosses in ecosystem succession and function in Alaska's boreal forest, Can. J. For. Res., 4, 1237–1264, 2010.
Turetsky, M. R., Bond-Lamberty, B., Euskirchen, E., Talbot, J., Frolking, S., McGuire, A. D., and Tuittila, E.-S.: The resilience and functional role of moss in boreal and arctic ecosystems, New Phytol., 196, 49–67, https://doi.org/10.1111/j.1469-8137.2012.04254.x, 2012.
Van, B. N.: How Sphagnum bogs down other plants, Trends Ecol. Evol., 10, 270–275, 1995.
Van Der Heijden, E., Verbeek, S. K., Kuiper, P. J. C.: Elevated atmospheric CO2 and increased nitrogen deposition: effects on C and N metabolism and growth of the peat moss Sphagnum recurvum P. Beauv. var. mucronatum (Russ.), Warnst. Glob. Change Biol., 6, 201–212, https://doi.org/10.1046/j.1365-2486.2000.00303.x, 2000.
van der Schaaf, S.: Bog hydrology, in: Conservation and Restoration of Raised Bogs: Geological, Hydrological and Ecological Studies, edited by: Schouten, M. G. C., The Government Stationery Office, Dublin, Ireland, 54–109, 2002.
van der Wal, R., Pearce, I. S. K., and Brooker, R. W.: Mosses and the struggle for light in a nitrogen-polluted world, Oecologia, 142, 159–168, 2005.
Van Gaalen, K. E., Flanagan, L. B., and Peddle, D. R.: Photosynthesis, chlorophyll fluorescence and spectral reflectance in Sphagnum moss at varying water contents, Oecologia, 153, 19–28, https://doi.org/10.1007/s00442-007-0718-y, 2007.
Verry, E. S. and Jansenns, J.: Geology, vegetation, and hydrology of the S2 bog at the MEF: 12,000 years in northern Minnesota, in Peatland biogeochemistry and watershed hydrology at the Marcell Experimental Forest, edited by Kolka, R. K., Sebestyen, S. D., Verry, E. S., and Brooks, K. N., CRC Press, New York, USA, 93–134, 2011.
Vile, M. A., Kelman Wieder, R., Živkovicì, T., Scott, K. D., Vitt, D. H., Hartsock, J. A., Iosue, C. L., Quinn, J. C., Petix, M., Fillingim, H. M., Popma, J. M. A., Dynarski, K. A., Jackman, T. R., Albright, C. M., and Wykoff, D. D.: N2-fixation by methanotrophs sustains carbon and nitrogen accumulation in pristine peatlands, Biogeochemistry, 121, 317–328, https://doi.org/10.1007/s10533-014-0019-6, 2014.
Vitt, D. H.: A key and review of bryophytes common in North American peatlands, Evansia, 31, 121–158, 2014.
Walker, A. P., Carter, K. R., Gu, L., Hanson, P. J., Malhotra, A., Norby, R. J., Sebestyen, S. D., Wullschleger, S. D., Weston, D. J.: 2017. Biophysical drivers of seasonal variability in Sphagnum gross primary production in a northern temperate bog, J. Geophys. Res.-Biogeo., 122, 1078–1097, https://doi.org/10.1002/2016JG003711, 2017.
Walker, M. D., Wahren, C. H., Hollister, R. D., Henry, G. H. R., Ahlquist, L. E., Alatalo, J. M., Bret-Harte, M. S., Calef, M. P., Callaghan, T. V., Carroll, A. B., Epstein, H. E., Jonsdottir, I. S., Klein, J. A., Magnusson, B., Molau, U., Oberbauer,S. F., Rewa, S. P., Robinson, C. H., Shaver, G. R., Suding, K. N., Thompson, C. C., Tolvanen, A., Totland, O., Turner, P. L., Tweedie, C. E., Webber, and P. J., Wookey, P. A.: Plant community responses to experimental warming across the tundra biome, P. Natl. Acad. Sci. USA, 103 1342–1346, 2006.
Walker, T. N., Ward, S. E., Ostle, N. J., and Bardgett, R. D.: Contrasting growth responses of dominant peatland plants to warming and vegetation composition, Oecologia, 178, 141–151, https://doi.org/10.1007/s00442-015-3254-1, 2015.
Wania, R., Ross, I., Prentice, I. C.: Integrating peatlands and permafrost into a dynamic global vegetation model: 1. Evaluation and sensitivity of physical land surface processes, Glob. Biogeochem. Cy., 23, GB3014, https://doi.org/10.1029/2008GB003412, 2009.
Wania, R., Melton, J. R., Hodson, E. L., Poulter, B., Ringeval, B., Spahni, R., Bohn, T., Avis, C. A., Chen, G., Eliseev, A. V., Hopcroft, P. O., Riley, W. J., Subin, Z. M., Tian, H., van Bodegom, P. M., Kleinen, T., Yu, Z. C., Singarayer, J. S., Zürcher, S., Lettenmaier, D. P., Beerling, D. J., Denisov, S. N., Prigent, C., Papa, F., and Kaplan, J. O.: Present state of global wetland extent and wetland methane modelling: methodology of a model inter-comparison project (WETCHIMP), Geosci. Model Dev., 6, 617–641, https://doi.org/10.5194/gmd-6-617-2013, 2013.
Weltzin, J. F., Harth, C., Bridgham, S. D., Pastor, J., and Vonderharr, M.: Production and microtopography of bog bryophytes: response to warming and water-table manipulations, Oecologia, 128, 557–565, https://doi.org/10.1007/s004420100691, 2001.
Weston, D. J., Timm, C. M., Walker, A. P., Gu, L., Muchero, W., Schmuta, J., Shaw, A. J., Tuskan, G. A., Warren, J. M., and Wllschleger, S. D.: Sphagnum physiology in the context of changing climate: Emergent influences of genomics, modeling and host-microbiome interactions on understanding ecosystem function, Plant Cell Environ., 38, 1737–1751, https://doi.org/10.1111/pce.12458, 2015.
White, M. A., Thornton, P. E., Running, S. W., and Nemani, R. R.: Parameterization and sensitivity analysis of the BIOME-BGC terrestrial ecosystem model: Net primary production controls, Earth Interact., 4, 1–85, 2000.
Wieder R. K.: Primary production in boreal peatlands, in: Boreal peatland ecosystems, edited by: Wieder, R. K. and Vitt, D. H., Springer-Verlag, Berlin, Heidelberg, Germany, 145–163, 2006.
Williams, T. G. and Flanagan, L. B.: Measuring and modelling environmental influences on photosynthetic gas exchange in Sphagnum and Pleurozium, Plant Cell Environ., 21, 555–564, 1998.
Wilmking, M., Juday, G. P., Barber, V. A., and Zald, H. S. J.: Recent climate warming forces contrasting growth responses of white spruce at treeline in Alaska through temperature thresholds, Glob. Change Biol. 10, 1724–1736, 2004.
Wolf, A., Callaghan, T. V., and Larson, K.: Future changes in vegetation and ecosystem function of the Barents Region, Clim. Change, 87, 51–73, https://doi.org/10.1007/s10584-007-9342-4, 2008.
Wolken, J. M., Mann, D. H., Grant, T. A., Lloyd, A. H., Rupp, T. S., and Hollingsworth, T. N.: 2016. Climate-growth relationships along a black spruce topose-quence in interior Alaska, Arct. Antarct. Alp. Res., 48, 637–652, 2016.
Wu, J. and Roulet, N. T.: Climate change reduces the capacity of northern peatlands to absorb the atmospheric carbon dioxide: The different responses of bogs and fens, Glob. Biogeochem. Cy., 28, 1005–1024, https://doi.org/10.1002/2014GB004845, 2014.
Wu, J., Roulet, N. T., Sagerfors, J., Nilsson, M. B.: Simulation of six years of carbon fluxes for a sedge-dominated oligotrophic minerogenic peatland in Northern Sweden using the McGill Wetland Model (MWM), J. Geophys. Res.-Biogeo., 118, 795–807, https://doi.org/10.1002/jgrg.20045, 2013.
Wu, Y. and Blodau, C.: PEATBOG: a biogeochemical model for analyzing coupled carbon and nitrogen dynamics in northern peatlands, Geosci. Model Dev., 6, 1173–1207, https://doi.org/10.5194/gmd-6-1173-2013, 2013.
Wu, Y., Verseghy, D. L., and Melton, J. R.: Integrating peatlands into the coupled Canadian Land Surface Scheme (CLASS) v3.6 and the Canadian Terrestrial Ecosystem Model (CTEM) v2.0, Geosci. Model Dev., 9, 2639–2663, https://doi.org/10.5194/gmd-9-2639-2016, 2016.
Yang, X., Ricciuto, D. M., Thornton, P. E., Shi, X., Xu, M., Hoffman, F., Norby R. J.: The effects of phosphorus cycle dynamics on carbon sources and sinks in the Amazon region: a modeling study using ELM v1, J. Geophys. Res.-Biogeo, 124, 3686–3698, https://doi.org/10.1029/2019JG005082, 2019.
Yu, Z., Loisel, J., Brosseau, D. P., Beilman, D. W., and Hunt, S. J.: Global peatland dynamics since the Last Glacial Maximum, Geophys. Res. Lett., 37, L13402, https://doi.org/10.1029/2010GL043584, 2010.
Yurova, A., Wolf, A., Sagerfors, J., and Nilsson, M.: Variations in net ecosystem exchange of carbon dioxide in a boreal mire: Modeling mechanisms linked to water table position, J. Geophys. Res.-Biogeo., 112, G02025, https://doi.org/10.1029/2006JG000342, 2007.
Zhang, W. X., Miller, P. A., Smith, B., Wania, R., Koenigk, T., and Doscher, R.: Tundra shrubification and tree-line advance amplify arctic climate warming: results from an individual-based dynamic vegetation model, Environ. Res. Lett., 8, 034023, https://doi.org/10.1088/1748-9326/8/3/034023, 2013.
Zhuang, Q., Melillo, J. M., Sarofim, M. C., Kicklighter, D. W., McGuire, A. D., Felzer, B. S., Sokolov, A., Prinn, R. G., Steudler, P. A., and Hu, S.: CO2 and CH4 exchanges between land ecosystems and the atmosphere in northern high latitudes over the 21st century, Geophys. Res. Lett., 33, L17403, https://doi.org/10.1029/2006GL026972, 2006.