the Creative Commons Attribution 4.0 License.
the Creative Commons Attribution 4.0 License.
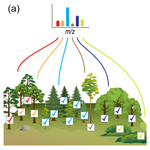
Reviews and syntheses: Opportunities for robust use of peak intensities from high-resolution mass spectrometry in organic matter studies
William Kew
Allison Myers-Pigg
Christine H. Chang
Sean M. Colby
Josie Eder
Malak M. Tfaily
Jeffrey Hawkes
Rosalie K. Chu
Earth's biogeochemical cycles are intimately tied to the biotic and abiotic processing of organic matter (OM). Spatial and temporal variations in OM chemistry are often studied using direct infusion, high-resolution Fourier transform mass spectrometry (FTMS). An increasingly common approach is to use ecological metrics (e.g., within-sample diversity) to summarize high-dimensional FTMS data, notably Fourier transform ion cyclotron resonance mass spectrometry (FT-ICR MS). However, problems can arise when FTMS peak-intensity data are used in a way that is analogous to abundances in ecological analyses (e.g., species abundance distributions). Using peak-intensity data in this way requires the assumption that intensities act as direct proxies for concentrations. Here, we show that comparisons of the same peak across samples (within-peak) may carry information regarding variations in relative concentration, but comparing different peaks (between-peak) within or between samples does not. We further developed a simulation model to study the quantitative implications of using peak intensities to compute ecological metrics (e.g., intensity-weighted mean properties and diversity) that rely on information about both within-peak and between-peak shifts in relative abundance. We found that, despite analytical limitations in linking concentration to intensity, ecological metrics often perform well in terms of providing robust qualitative inferences and sometimes quantitatively accurate estimates of diversity and mean molecular characteristics. We conclude with recommendations for the robust use of peak intensities for natural organic matter studies. A primary recommendation is the use and extension of the simulation model to provide objective guidance on the degree to which conceptual and quantitative inferences can be made for a given analysis of a given dataset. Broad use of this approach can help ensure rigorous scientific outcomes from the use of FTMS peak intensities in environmental applications.
- Article
(4331 KB) - Full-text XML
-
Supplement
(1936 KB) - BibTeX
- EndNote
- Included in Encyclopedia of Geosciences
Organic matter (OM) plays a central role in Earth's biogeochemical cycles and is both a resource for and product of metabolism. The detailed chemistry of OM (e.g., nominal oxidation state) can modulate and reflect biogeochemical rates and fluxes within and across ecosystems (e.g., Boye et al., 2017; Garayburu-Caruso et al., 2020; LaRowe and Van Cappellen, 2011), yet our understanding of this complexity is limited by our analytical abilities to view it (Hawkes and Kew, 2020; Hedges et al., 2000; Steen et al., 2020). Given the importance of OM chemistry in biogeochemical cycling, there is a need to understand how and why that chemistry varies through space and time. To help meet this need, there has been growing interest in using concepts and methods from ecology to study the chemo-geography and chemo-diversity of OM in a variety of ecosystems (e.g., Danczak et al., 2021; Kellerman et al., 2014; Kujawinski et al., 2009; Tanentzap et al., 2019). This is a promising approach as there are many conceptual parallels between the chemical species that comprise OM and the biological species that comprise ecological communities (Danczak et al., 2020).
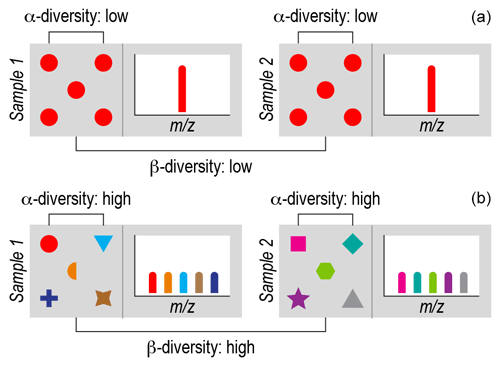
Figure 1Ecological concepts of α diversity and β diversity. Each gray box represents a sample of an ecological community or collection of organic molecules (i.e., a NOM assemblage). Symbols represent individual organisms or molecules. Different biological or molecular species are represented by a combination of shapes and colors. (a) Each sample has one biological species (red circles) or one chemical species (red bar), and the species are the same within and between the samples. This reflects minimal α diversity because there is a single species. This also reflects minimal β diversity because there is no difference in terms of which species are present in each sample. (b) Each sample has five species (biological or chemical) represented by different colors and symbols. There are no shared species between samples. This reflects maximum α diversity because every individual is a different species within each sample and maximum β diversity because there are no species shared between samples. In real ecological and NOM samples, α diversity and β diversity fall between these extremes.
The most fundamental ecological data type is the species-by-site matrix. This matrix indicates how many individuals of each species occur in each sampled community. Ecologists use species-by-site matrices to ask a myriad of questions related to biological diversity and often complement these data with information on the properties or “functional traits” of species (e.g., body size) (McGill et al., 2006; Villéger et al., 2017; Violle et al., 2007). Two common analyses are known as α diversity and β diversity, each with numerous metrics (Anderson et al., 2011; Whittaker, 1972), including versions that include functional trait information (Laliberté and Legendre, 2010). α diversity measures the diversity within a given community. β diversity has been variously defined but essentially measures variations in composition across communities. Both α diversity and β diversity can be quantified using presence-absence data or they can include estimates of each species' relative abundance within and between communities (Fig. 1). In addition to being incorporated into these diversity metrics, functional trait data can be used to estimate community-level mean trait values (Lavorel et al., 2008). As with diversity, mean trait values can be estimated using presence–absence or relative abundance data. Estimates of diversity and mean trait values are examples of ecological metrics often applied to OM chemistry (Bahureksa et al., 2021; Cooper et al., 2022; Sakas et al., 2024; Tanentzap et al., 2019).
The chemistry of OM is commonly studied using high-resolution Fourier transform mass spectrometry (FTMS) techniques (e.g., Hawkes and Kew, 2020), such as Orbitrap or ion cyclotron resonance (ICR) MS, via direct infusion of samples. At present, the highest-resolution approach for un-targeted analysis of OM is via a 21 Tesla FT-ICR MS (Bahureksa et al., 2021; Marshall et al., 1998; Shaw et al., 2016; Smith et al., 2018). The output data produced constitute a spectrum containing peaks represented by a signal intensity (Fig. 2, y axis) and a mass-to-charge ratio () (Fig. 2, x axis), which is equivalent to the mass for singly charged ions as routinely detected in natural organic matter (NOM) measurements. In turn, regardless of the type of MS instrument used, the MS data inherently lead to an OM peak-by-sample data matrix akin to an ecological species-by-site data matrix. The high-resolution data from MS often result in a large matrix, wherein a single sample may contain thousands to tens of thousands of peaks. It is often possible to assign molecular formulas to a large fraction of observed peaks, which enables the calculation of several properties such as stoichiometric ratios (Bahureksa et al., 2021; Cooper et al., 2022) that are akin to organismal functional traits. To take advantage of these rich data, FTMS data have been analyzed using the same α diversity, β diversity, and mean trait metrics that are commonly used by ecologists to study biological communities (e.g., Kellerman et al., 2014). Such analyses are exciting as they enable the same conceptual questions and quantitative frameworks to be applied to biological (e.g., microbial communities) and chemical (i.e., NOM) components that directly interact with each other within ecosystems (Danczak et al., 2020, 2021; Li et al., 2018; Lucas et al., 2016; Osterholz et al., 2016; Tanentzap et al., 2019).
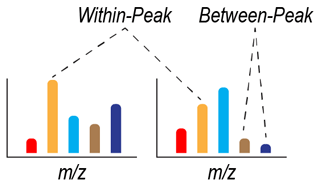
Figure 2Summary of within-peak and between-peak comparisons of peak intensity. Two idealized mass spectra (i.e., from two samples) are shown, with each peak defined by a mass-to-charge ratio () and represented by a different color. The intensity of each peak in each sample is represented by the height of each colored bar. Within-peak comparisons of intensity are based on comparing intensities at the same across two or more samples. Between-peak comparisons of intensity are based on comparing intensities at two or more values. Between-peak comparisons can be done within a sample (as shown) or between samples (not shown).
The use of ecological metrics with MS data is particularly common with FTMS datasets, and there is great potential to continue leveraging concepts from ecology in high-resolution NOM analyses. Care is required, however, in using FTMS peak-intensity data to estimate α diversity, β diversity, mean trait values, and related ecological analyses (e.g., “species” abundance distributions). Key to these ecological analyses is the assumption that, within complex NOM samples, differences in peak intensity are proportional to the differences in the concentrations of the associated molecules. Studies using FTMS often avoid using peak intensities due to uncertainties regarding whether it is valid to assume proportionality between peak intensities and concentrations within and across NOM samples (Bhatia et al., 2010; Danczak et al., 2020; Kujawinski, 2002). These studies may be discarding useful information, though it is unclear what biases and uncertainties are introduced into ecological metrics when using FTMS peak intensities. To help advance robust use of FTMS datasets for NOM studies, we review the theoretical reasons why peak intensities may not reflect true concentrations, provide empirical evaluation of this theory, and invoke in silico simulations to quantify the associated impacts on ecology-inspired analyses. While theory and empirical analyses demonstrate disconnects between peak intensities and concentrations in FTMS data, the simulations show that intensity-weighted ecological metrics often provide robust estimates of NOM diversity and mean trait values. We end with practical recommendations and propose a path forward for increasing the robust use of FTMS peak intensities for NOM studies.
Here, we provide a review of the theoretical foundations behind why assuming proportionality between peak intensities and concentrations in FTMS can be challenging. This section will be of most value to FTMS data users that are not formally trained in mass spectrometry and serves as a review of mass spectrometry principles (Bahureksa et al., 2021; see also Kujawinski, 2002; Urban, 2016). We focus on FTMS (i.e., FT-ICR and Orbitrap), but many of the principles are applicable across all MS platforms. We highlight three considerations: ionization, ion transfer, and ion signal detection in the context of commercial FTMS instruments. These considerations have practical implications tied to within-peak and between-peak comparisons (Fig. 2). Here, we define “within-peak” comparisons as comparisons of peak intensities of the same feature (i.e., or molecular formula) across different sample spectra and “between-peak” comparisons as comparisons peak intensities across different features. Both within-peak and between-peak comparisons are fundamentally based on the observed within a mass spectrum, and neither addresses comparisons across isomers. Further, we suggest the consistent use of the term “intensity” in FTMS NOM studies to describe how much signal is observed for a given peak as opposed to “height”, “magnitude”, or other alternatives. While terminology is not our central focus, it is useful to pursue consistency across studies. As discussed below, within-peak comparisons can be robust under certain situations, but there are limitations with between-peak comparisons that may be unavoidable. The following discussion is not an exhaustive treatment of all decisions associated with a complete FTMS experiment, and we do not deeply address factors such as sample preparation, choice of ionization mode, and instrument-specific parameter optimization. These topics have been discussed in a recent review (Bahureksa et al., 2021).
2.1 Ionization efficiency and isomers
Electrospray ionization (ESI) is the most common technique for generating ions from NOM samples. When using ESI, the peak intensity for any given molecular mass (or molecular formula) will depend on both concentration and ionization efficiency, the latter of which is dependent on structure, the acid dissociation constant (pKa), and the other molecules in the sample (Kruve et al., 2014). In NOM samples, one detected mass or peak combines signals from multiple isomers which all have the same molecular formula but different structures. The different structures impact ionization efficiency, but FTMS data contain no information about this structural variation. Unfortunately, to date, no liquid chromatography (Han et al., 2021; Kim et al., 2019) or ion mobility separation (Leyva et al., 2020; Tose et al., 2018) technique has yet demonstrated sufficient resolution to completely infer structural variations among isomers within complex NOM samples. Unknown variations in structure can, therefore, lead to unknown variations in peak intensities. This challenge can be compounded by ionization suppression that occurs when the ionization efficiency of one type of molecule (i.e., peak) is altered by the presence of other types of molecules (Ruddy et al., 2018). Ionization suppression can be mitigated by online separation, whereby non-targeted liquid chromatography–mass spectrometry (LC-MS) approaches may yield more quantitative data (Kruve, 2020), but matrix effects remain a significant issue even for LC-MS (Trufelli et al., 2011). In NOM samples with thousands of types of organic molecules, the molecular interactions are likely to have complex influences over realized ionization efficiencies. While it is possible to control for some of these challenges (e.g., using consistent sample concentrations and preparations), many additional factors (e.g., molecular structures, pKa's, and interactions among molecules in NOM samples) cannot yet be accounted for. The interpretation of peak intensities as proxies for concentrations in FTMS data streams may, therefore, be prone to uncertainty.
2.2 Ion transmission and collection
In FTMS, packets of ions are accumulated in a trap prior to their transmission to the analyzer cell (Makarov et al., 2006; Fig. 3i, section d; Senko et al., 1997). The duration of time in which ions are accumulated is often varied to yield an optimal ion population for the analyzer cell. The duration of this event can change the relative abundance and, thus, the observed peak intensities of different ions (Cao et al., 2016). Increases in the true abundance of other ions can decrease the measured peak intensity of a given ion due to a dilution effect resulting from a finite number of ions that can fit within the ion trap. Additional challenges arise due to variations in the speed at which different ions move from the accumulation trap and into the analysis cell. Smaller ions move more quickly and therefore reach the analysis cell sooner than larger ions. Variations in the accumulation time across samples and FTMS instruments, combined with among-ion variations in transmission speed, can introduce additional uncertainty in the relationship between peak intensities and true concentrations.
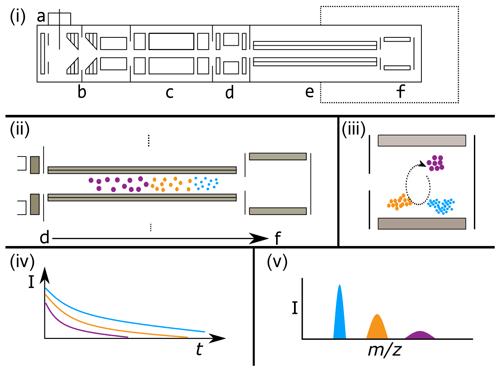
Figure 3Illustrative example of a generic FT-ICR mass spectrometer (i), showing common and key biases between FT-ICR signal intensity and of ions (ii–v). Panel (i) shows the major elements of a generic FT-ICR mass spectrometer (based loosely on a Bruker solariX FT-ICR MS geometry). Panel (i) elements include the following: a – atmospheric pressure ionization source (i.e., ESI source), b – source ion optics (i.e., dual ion funnels), c – mass-selecting quadrupole, d – collision cell, e – transfer multi-poles to ICR cell, f – ICR cell. The dashed line indicates the magnetic field. Note that the diagram is deliberately simplified and not to scale. Panel (ii) demonstrates the time-of-flight bias along the transfer multi-poles (e) in the “flight tube” from the collision cell (d) to the ICR cell (f). Lower ions travel faster, as indicated by the smaller ions reaching the ICR cell first. Ions are shaded to aid visualization. Panel (iii) visualizes the effect of a variable excitation radii for ions of different masses, as may happen with a CHIRP excitation pulse. Lower ions are closer to the detection electrodes (shaded in gray) and therefore will induce a larger image current. Note also that the ion populations have been adjusted from (ii) to indicate biases from the time-of-flight effect. Panel (iv) shows the time domain recorded signal intensity against time, with the ions having an initial intensity roughly proportional to the number of ions in that cloud. However, as time progresses, the less abundant ion clouds lose coherence and destabilize more rapidly, resulting in an attenuation of their signal. Note that the real signal would follow a damped sinusoidal function; here, an absolute-value approximation is shown for simplicity. Panel (v) shows the mass spectrum post-Fourier transform, demonstrating that the impact is not only on peak intensity (shown as height) but also on peak resolution (shown as width). In all cases, effects are deliberately exaggerated and not to scale to aid interpretation.
2.3 Ion signal detection
The final step in data collection via FTMS is signal detection. The intensity of the signal is proportional to the abundance of a given ion in the analysis cell, the proximity of ions to the detector (Kaiser et al., 2013), and the ion charge state (Wörner et al., 2020). Similarly to molecular interactions impacting ionization efficiencies, different types of ions can interact to affect each others' signal intensities. The Fourier transform applied to the data also complicates extremely accurate relative quantification of ion abundance between peaks (Makarov et al., 2019). These challenges at the detection stage can add more uncertainty to the relationship between peak intensity and concentrations, particularly for complex NOM samples.
In this section, we move beyond theoretical considerations to empirical evaluations of the real-world relationships between peak intensities and concentrations. Similarly to above, this section will be of primary value to those without formal training as mass spectrometrists but who use FTMS data to study NOM. The experimental methods used are described in detail in the Supplement.
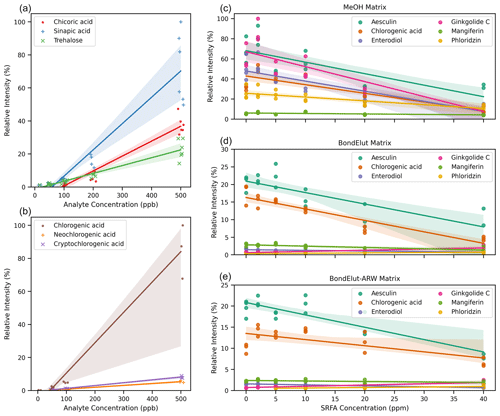
Figure 4(a) Scatterplot visualization of the relationship between signal intensity (relative intensity) and concentration of analyte for three chemically distinct molecules analyzed contemporaneously but independently in pure methanol solvent. Relative intensity indicates that data were scaled to the largest signal in any replicate from the associated series of spectra. Linear regression and confidence intervals were calculated by the Seaborn plotting library with default settings. The x-axis jitter was added to aid visualization of overlapping points. (b) As with (a) but for three structural isomers of chlorogenic acid. The x-axis jitter was added to aid visualization of overlapping points. (c–e) Compounds spiked into three different solvent matrices (methanol; Bond Elut methanol; and Bond Elut artificial river water, ARW) at a fixed concentration (100 ppb) but with the addition of SRFA at varying concentrations from 0 to 40 ppm. In all cases, only the [M−H]− ion is shown, but other ions (i.e., [M+Cl]−) were detected. Relative intensities have been scaled per plot for (a) and (b) and are on the same scale for (c–e). Pearson's r correlation coefficients and p values are reported in Table S1 in the Supplement.
3.1 Direct comparison of peak intensities in idealized samples
As discussed above, different organic compounds ionize with different efficiencies. In theory, this may lead to variations in observed peak intensities even when all organic compounds have the same true concentration. To evaluate this theoretical expectation, we analyzed several different types of organic compounds in different conditions via FT-ICR MS. We selected chemical standards (see Supplement for details) which are natural products with molecular formulae and chemistries typical of compounds commonly observed in organic matter and were amenable to negative-mode ESI analysis. First, we analyzed three separate dilution ladders of individual pure compounds dissolved in pure methanol. These standards were analyzed at higher concentrations than typically observed for NOM because they were single compounds rather than formula-summed features (with multiple isomers) within a NOM spectrum; higher concentrations were required to compensate for lower isomeric diversity. These three compounds gave rise to different peak intensities under otherwise identical conditions (Fig. 4a). Trehalose, for example, had a much lower peak intensity than Sinapic acid at the same actual concentration. The difference in signal intensity was also apparent amongst compounds that ionize well under negative-mode ESI; for example, two different structures containing the same number of carboxylic acid units exhibited differences in signal intensity. We also observed differences in peak intensities amongst structural isomers (i.e., same molecular formula and mass) (Fig. 4b). Each peak observed via direct-infusion FT-ICR MS may be several isomers. These isomers may be observable through chromatographic separation (Kim et al., 2019), ion mobility separations (Leyva et al., 2019), or statistical inference of tandem mass spectrometry (Zark et al., 2017) but not via direct-infusion FT-ICR MS. We note that absolute differences in signal intensity may be smaller between molecules at lower concentrations, but this does not necessarily mean that low-intensity signals consistently indicate low concentrations, and this does not aid in quantitatively interpreting higher-intensity signals. In summary, differences in peak intensities across organic compounds do not necessarily equate to differences in concentration unless assessed via a calibration curve for each compound.
3.2 Comparison of peak intensities in real-world samples
Routine NOM samples contain a diverse range of thousands of molecules of unknown structures and relative concentrations and often contain inorganic interferences, such as salts. Sample clean-up that focuses on pre-concentration and desalting is imperfect (Li et al., 2017; Raeke et al., 2016) but is commonly used to minimize inorganic interferences. However, interactions among molecules remain a challenge, as discussed above. The collection of molecules in a sample is referred to here as the “matrix”. To explore matrix effects on peak intensities, we prepared solutions of six different pure compounds at a fixed concentration (100 ppb) in three different solvent systems: pure methanol, methanol eluted from a Bond Elut solid-phase extraction (SPE) cartridge, and methanol from elution off of a Bond Elut SPE cartridge which had been loaded with artificial river water (ARW). Additionally, we added to each sample a complex mixture that is often used as a NOM standard, Suwannee River fulvic acid (SRFA), at six different concentrations. Samples were analyzed independently but contemporaneously on the same instrument to mirror a real study.
In methanol-only solvent, with no added SRFA, the six compounds yielded different peak intensities (Fig. 4c), which is consistent with results from the previous subsection. As the concentration of SRFA was increased to 2 ppm, the relative signal intensity increased for some of the six compounds but decreased for others. Above 2 ppm of SRFA, peak intensities for all six compounds were substantially decreased. Use of an “impure” methanol solvent, i.e., the eluent from an SPE blank (Fig. 4d) or from an SPE of artificial river water (Fig. 4e), resulted in further decreases in peak intensities. In both cases, the maximum peak intensity was ∼ 20 % of what was seen in pure methanol (Fig. 4c), and some of the six compounds were no longer observed. Addition of SRFA to these samples with “impure” solvents, again, generally, decreased peak intensities. A “real-world” sample set would have even greater diversity and heterogeneity than presented here, and, thus, the issues with the use of peak intensities for quantitative interpretation would likely be exacerbated.
Combining the empirical results from this subsection and from the previous subsection with the instrument theory discussed above suggests significant uncertainty in the relationships between true concentrations and peak intensities from direct-infusion FT-ICR MS. Calibration curves can be used in the simplest of situations but will be challenging when there are unknown variations in structural isomer and matrix compositions. Modeling of constrained systems may, however, allow for data-driven and mechanistic data normalization strategies for the enhanced use of peak-intensity data.
3.3 Data normalization strategies
In the previous section, we use the peak intensities for each analyte without any normalization, only scaling to the base peak or between spectra to make comparison easier. However, more sophisticated or comprehensive normalization strategies may be useful when trying to make quantitative inferences regarding the data. Considerations may include whether to use the total intensity within a spectrum (including noise, isotopologues, and unannotated features) or to use just the peak intensity apportioned to annotated features. Additionally, non-linear or more sophisticated functions may have benefits. Such post hoc statistical approaches have utility for some applications but do not resolve the fundamental, underlying physical origins of the weak connection between peak intensities and true concentrations. We refer readers to the work of Thompson et al. (2021) for more insights into the theory and for an application of the normalization of FTMS for complex mixtures.
The preceding sections indicate challenges when using FTMS peak intensities as proxies for relative changes in the concentrations of organic molecules. The implication is that some ecologically inspired analyses (e.g., Fig. 1) may be challenging to use with FTMS peak-intensity data. To understand which analyses could be impacted, we differentiate analyses into two classes: those based on within-peak intensity comparisons and those based on between-peak intensity comparisons (Fig. 2). As noted above, within-peak is based on comparing the same feature ( or molecular formula) across spectra or samples, whereas between-peak compares different features ( or molecular formulas) across and within spectra or samples.
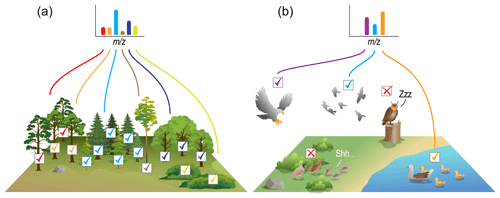
Figure 5Graphical summary of how FTMS peak-intensity data are often treated (a), which is distinct from the reality of those data (b). When surveying the number of individuals of each species within a tree community, there is good confidence that the measured abundances are close to real abundances. This is because there is relatively little variation across species in terms of the ability to detect individuals. FTMS peak-intensity data are often used as though they are like tree community data. However, FTMS data are more like bird community data. That is, the ability to detect different species varies due to intrinsic factors (e.g., activity patterns, how loud and often birds call) and extrinsic factors (e.g., habitat structural complexity, predator-induced behavioral changes). Similarly, the intrinsic physics of a given molecule will impact its ability to ionize and thus its observed peak intensity, and in environmental samples there are thousands of molecular species that impact each others' ionization “behaviors”. FTMS data being more bird-like than tree-like needs to be accounted for when performing ecological analyses using FTMS data.
We posit that analyses using FTMS between-peak intensity comparisons could have the greatest uncertainty. Consider an ecological setting in which a researcher aims to quantify within-sample diversity (α diversity) and among-sample diversity (β diversity) (Fig. 1) of tree communities (Fig. 5a). The researcher will likely set up a plot of a given size and then directly count the number of each tree species in each plot, thus generating the species-by-site matrix filled with directly observed abundance counts for each species. The ability of the researcher to observe individuals of each species does not vary appreciably across species because each tree is not moving, and our ability to see a static object is not influenced by environmental factors. Thus, the number of individuals observed for a given tree species is quantitatively comparable to the number of individuals observed for all other tree species in the plot. The assumption that differences in observed abundances carry robust information about differences in actual abundances is thus supported in this example. In turn, it is valid to use relative abundances to compute α diversity, such as via Shannon evenness (Elliott et al., 1997; Mouillot and Leprêtre, 1999; Redowan, 2015). Furthermore, because the ability to observe each tree species is the same across communities, it is valid to use relative abundances to compute β diversity (e.g., via Bray–Curtis; Anderson et al., 2011) or to conduct other ecological analyses that use abundance data (e.g., species abundance distributions; McGill et al., 2007).
We contrast this tree community example with another ecological setting. Consider a researcher studying bird communities (Fig. 5b) that estimated species abundances solely based on the number of times an observer hears the call of a given species. In this case, those species that call more frequently and/or more loudly will be more likely to be heard, and, thus, an observer will infer a higher abundance even if all species in the community have the same abundance. That is, such a method generates data that may indicate which species are present, but the “call counts” do not carry reliable information regarding absolute or between-species relative abundances. Follow-on analyses of α diversity and β diversity should, therefore, be limited to approaches that use presence–absence data, and species abundance distributions cannot be quantified.
If we continue with the bird community example and assume that the detectability of a given bird species is consistent across sampled locations or times, then it would be appropriate to examine variations in within-species call counts. This within-species analysis is directly analogous to the FTMS within-peak time series analysis in Merder et al. (2021), discussed below. However, if call counts of a given species are suppressed by the presence or abundance of other species then call counts of a given species may not indicate changes in its abundance. The call count example is directly analogous to the influences of the NOM matrix: if the presence or abundance of a given organic molecule modifies the ionization of other molecules, then within-peak changes in intensity may not indicate changes in concentration. In turn, analyses based on within-peak intensity comparisons could lead to error and uncertainty in the values of computed ecological metrics, especially if there are significant cross-sample changes in the NOM matrix.
As described in the previous sections, the unique chemistry of every molecule in a NOM sample can influence ionization properties for other molecules in the sample. Thus, FTMS data align with the bird community example rather than the tree community example, with the differing physics of each molecule influencing between-peak differences in peak intensity. Molecules that more readily ionize will produce higher peak intensities, which is akin to bird species with noisier or more numerous calls producing a larger number of call counts that do not accurately represent the underlying population distribution. Similarly, between-peak differences in intensity as observed via FTMS cannot be directly used as a proxy to indicate between-peak differences in concentration.
In contrast to between-peak comparisons, within-peak comparisons examine changes in the relative intensity of a single peak across samples. Such within-peak comparisons may be repeated independently for each peak of interest in a given dataset. For example, Merder et al. (2021) quantified the temporal dynamics of individual FTMS peaks and then binned peaks into different groups with characteristic temporal fluctuations. In those analyses, peak intensities were not compared between peaks. Instead, the temporal dynamics of each peak were compared to the temporal dynamics of other peaks. The underlying assumption of this type of analysis is that a between-sample increase in the intensity of a given peak can be used as a robust proxy of a between-sample increase in the concentration of that peak. Materials presented in the previous sections indicate that this assumption can be met in some instances when using FTMS data. However, great care is required, with strong attention being paid to the assumptions of the analysis methods. For example, using Pearson correlation makes the assumption that the concentration of a given peak is a linear function of the changes in its peak intensity. We showed above (Fig. 4) that this assumption is not always valid, even in ideal conditions. Using a Spearman correlation avoids this assumption because it is based on ranks. That is, Spearman correlations (e.g., Kellerman et al., 2014) make the more realistic assumption (for FTMS data) that an increase in the concentration of a given peak is reflected as an increase in its peak intensity without assuming any statistical or mathematical form of that relationship.
The previous sections highlight challenges in connecting between-peak changes in observed intensity to between-peak changes in true abundance (Fig. 4). These challenges violate an assumption of abundance-based ecological analyses: proxies for abundance (e.g., peak intensity) should be proportional to true abundances. However, the quantitative impacts of this situation likely vary across ecological metrics and with study details. There may be certain metrics or conditions in which robust inferences can be made despite poor linkages between peak intensities and true abundances. These cases are important to understand for robust use of abundance-based ecological metrics in FTMS NOM studies.
To provide initial guidance on best practices for using FTMS peak intensities with ecological metrics, we developed an in silico simulation model (full details are in the Supplement). This model generates synthetic data and introduces errors that degrade the linkage between peak intensity and true abundance. Simulated values that are influenced by introduced errors are conceptually analogous to intensities from real-world FTMS NOM studies. This allows us to probe how errors inherent to FTMS may impact the relationship between true and observed values of ecological metrics. To generate synthetic data, the simulation model produced samples with either 100 or 1000 peaks. This was done to study how the influences of errors change with the number of peaks; going above 1000 peaks did not change the outcomes, and going below 100 peaks is unlikely to be relevant to many FTMS studies. For each run of the model, the true abundances of each peak within each of the two independent samples were randomly assigned from Gaussian distributions that differed across samples and simulation iterations. We simulated two types of errors that modified the true abundances: within-sample error reflects variations in ionization efficiency across molecules (but not across samples), and between-sample error reflects variations in ionization efficiency across molecules and samples.
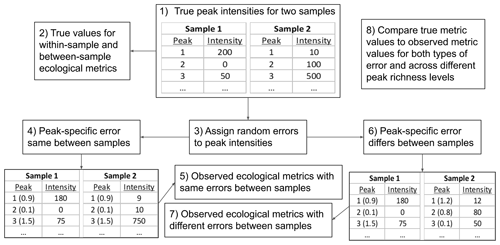
Figure 6Flow diagram for the in silico simulation model. The model was used to evaluate how ecological metrics are impacted by variations in ionization across organic molecules (i.e., peaks). The true peak intensities are what is expected if intensity is connected linearly to concentration, and all peaks fall along the same linear function. Variation in ionization adds error around this idealized linear relationship. The error is modeled in two ways: the error applied to a given peak is either the same between samples (i.e., there are no variable matrix effects on ionization) or varies randomly between samples (i.e., there are variable matrix effects on ionization). In the lower tables, the proportional error applied to each peak is provided parenthetically. The tables are for demonstration and show only three peaks per sample. The number of peaks per sample was set to either 100 or 1000.
To examine how both types of error influence ecological metrics, we used the initial true abundances and the error-modified abundances (analogous to observed peak intensities) to calculate within-sample α diversity via the Shannon diversity metric, the between-sample β diversity via the Bray–Curtis dissimilarity metric, and a generic intensity-weighted sample-level mean trait value based on assigning an arbitrary trait value to each peak (Fig. 6). The mean trait analysis is analogous to the approach commonly used in ecological studies for computing community-level abundance-weighted trait values, such as plant leaf area index or animal body size (Muscarella and Uriarte, 2016). This mean trait approach is also commonly used with FTMS data, such as for sample-level peak-intensity-weighted values of stoichiometric ratios (e.g., H:C) and several other metrics derived from molecular formulae (Roth et al., 2019; Wen et al., 2021).
Relating the error-influenced “observed” values of each ecological metric to their true values revealed that peak-intensity-based ecological metrics are likely to be qualitatively robust despite quantitative biases (Figs. 7–9). All three ecological metrics showed monotonic relationships between observed and true values for both types of error; in Figs. 7–9, all a/c and b/d panels have within-sample and between-sample errors, respectively. Uncertainty was lower when samples had 1000 peaks relative to samples with 100 peaks; in Figs. 7–9, all a/b and c/d panels have 100 and 1000 peaks, respectively. For Shannon diversity, observed values were consistently lower than true values, but all observed vs. true relationships were linear (Fig. 7). For Bray–Curtis, inclusion of between-sample errors resulted in an overestimation of values and non-linear monotonic relationships between observed and true values (Fig. 8). For mean trait values, we found no systematic quantitative biases, and the relationships between observed and true values were consistently linear (Fig. 9).
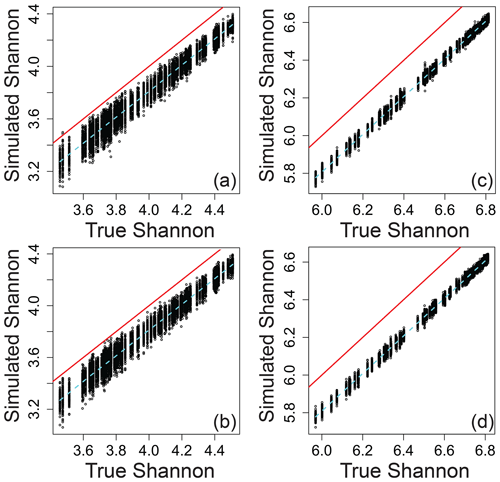
Figure 7Shannon α diversity that includes simulated error regressed against true Shannon across different scenarios. (a) The same error applied to a given peak between samples and 100 peaks per sample. (b) Different errors applied to a given peak between samples and 100 peaks per sample. (c) The same error applied to a given peak between samples and 1000 peaks per sample. (d) Different errors applied to a given peak between samples and 1000 peaks per sample. In all panels, the red line represents the one-to-one line, and the dashed line is a spline fit to the data. All data are from the simulation model.
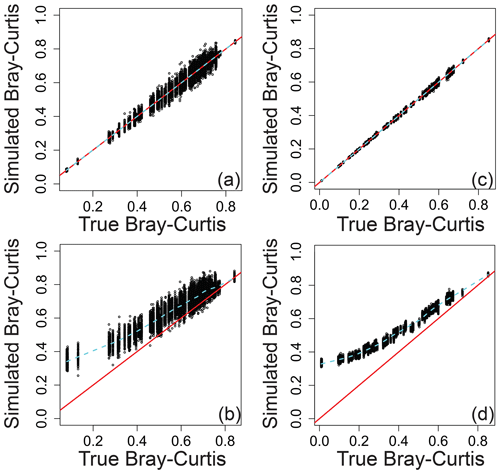
Figure 8Bray–Curtis dissimilarity as a measure of β diversity that includes simulated error regressed against true Bray–Curtis across different scenarios. (a) The same error applied to a given peak between samples and 100 peaks per sample. (b) Different errors applied to a given peak between samples and 100 peaks per sample. (c) The same error applied to a given peak between samples and 1000 peaks per sample. (d) Different errors applied to a given peak between samples and 1000 peaks per sample. In all panels, the red line represents the one-to-one line, and the dashed line is a spline fit to the data. All data are from the simulation model.
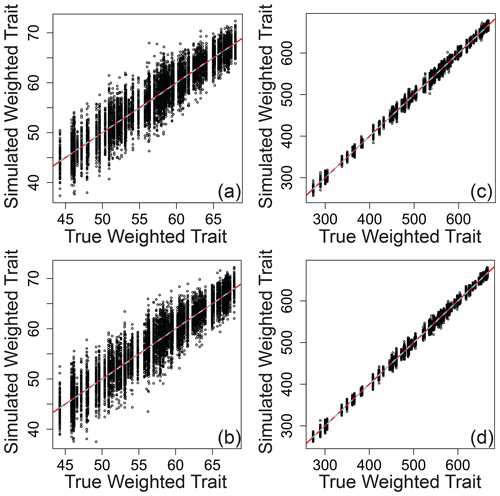
Figure 9Mean peak-intensity-weighted trait values that include simulated error regressed against true mean peak-intensity-weighted trait values across different scenarios. (a) The same error applied to a given peak between samples and 100 peaks per sample. (b) Different errors applied to a given peak between samples and 100 peaks per sample. (c) The same error applied to a given peak between samples and 1000 peaks per sample. (d) Different errors applied to a given peak between samples and 1000 peaks per sample. In all panels, the red line represents the one-to-one line, and the dashed line is a spline fit to the data. All data are from the simulation model.
The variation in observed values explained by true values (via a linear model) increases rapidly with the number of peaks and asymptotes beyond ∼ 500–1000 peaks per sample (Fig. S1 in the Supplement). The number of peaks needed to reach the asymptote and to minimize uncertainty is likely to be dataset dependent, and 500–1000 peaks should not be taken as a general rule for real-world datasets. Nonetheless, we propose that qualitative gradients based on sample-to-sample changes in the value of ecological metrics can generally be interpreted with increasing confidence as the number of peaks increases. Quantitative comparisons from one dataset to another may, however, require further simulation-based evaluation as the absolute magnitudes of some ecological metrics are shifted away from their true magnitudes even when there are large numbers of peaks (e.g., Fig. 8d). We encourage researchers to use the simulation model with the numbers of peaks present in their real-world datasets to better understand their ability to make statistical and conceptual inferences.
There is significant value in using FTMS data to study NOM chemistry (Bahureksa et al., 2021; Cooper et al., 2022; Spencer et al., 2015; Stubbins et al., 2010), and it is vital that this be done based on rigorous use of the data. When using ecological metrics with FTMS NOM data, it is important to understand how the assumptions and limitations of the metrics relate to limitations of the data. We suggest that studies using FTMS peak intensities need to include material that directly discusses the data limitations, what peak intensities do and do not represent (e.g., tree-like vs. bird-like data; Fig. 5), and how knowledge of those limitations was used to select specific metrics.
We have provided both theoretical reasoning and empirical observations showing that peak intensities do not necessarily map to concentrations of the associated organic molecules within NOM-like complex mixtures of organic molecules. This is particularly true for between-peak comparisons, and statistical post hoc normalizations of peak-intensity data do not solve this challenge. We caution against using between-peak differences in intensity from FTMS data to make direct inferences related to between-peak differences in abundance or concentration. This has implications for some ecological analyses based directly on variations in species abundances. For example, estimation of “species abundance distributions” are likely to be problematic. Analyses that bin peaks into high- and low-abundance groups based on between-peak differences in concentration are also likely to be problematic. We did not directly evaluate these types of analyses, and we suggest that future work should expand upon the ecological metrics examined here via simulation.
While there are challenges and limitations in the use of ecological metrics with FTMS data, we show that there is a tangible path forward. In particular, our simulation model revealed good performance in terms of some common ecological metrics of α diversity, β diversity, and mean trait values. We infer that conceptual and mechanistic inferences are likely to be valid when based on analyses such as comparing peak-intensity-based ecological metrics across experimental treatments or variations along environmental gradients. The performance of intensity-weighted mean trait values was particularly good, both qualitatively and quantitatively. This indicates that using peak intensities to estimate sample-level mean traits or properties likely provides quantitatively robust estimates, such as for stoichiometric ratios (e.g., , ) and many other commonly calculated quantitative properties related to molecular formulas (e.g., nominal oxidation state of carbon, aromaticity index, double bond equivalent).
As general guidance, we suggest avoiding analyses that make direct use of between-peak comparisons of peak intensity and relying instead on derived metrics that use intensities from large numbers of peaks. For example, while peak-intensity-weighted mean trait values appear to be robust, our physical experiments indicate caution against using direct comparison of peak intensities to infer between-peak differences in concentration. This is relevant to analyses such as comparing peak intensities within a Van Krevelen analysis or across classes of elemental composition (e.g., CHO, CHON). Such analyses could be problematic as quantitative variation in peak intensities across the Van Krevelen space or across classes of elemental composition is likely to be influenced by variations in ionization efficiencies with unclear connections to true concentrations. It is, therefore, not recommended that one use peak intensities to identify parts of Van Krevelen space (e.g., protein-like compounds) or compositional classes that have the highest concentrations in a given sample. It is preferable to report the fractions of peaks contained within different parts of Van Krevelen space or within different compositional classes and avoid quantitative estimates based on peak intensities (e.g., percentage of total sample-level intensity found within a given class). An alternative approach for robust and direct use of peak intensities, based on our physical experiments, is the use of Spearman-based correlations for within-peak comparisons across samples. Such correlations could be done across spatial environmental gradients, through time, and/or with respect to other sample-level quantitative measurements (e.g., organic carbon concentration). This implies that other types of correlation-based analyses are likely to be robust, such as peak-intensity-based network analyses. We further suggest that parametric statistics can often be used to relate the ecological metrics studied here to other quantitative variables, both directly (e.g., via Pearson-based correlation) and indirectly (e.g., via Bray–Curtis-based non-metric multi-dimensional scaling).
There are many other kinds of analyses currently done with FTMS data, and more will be imagined in the future. To develop further guidance on how to best use peak intensities for a broader range of analyses, we recommend the use and further development of the simulation model developed here. Fortunately, it is straightforward to extend the simulation model to additional metrics (e.g., Hill numbers; Hill, 1973) and analyses (e.g., species abundance distributions; McGill et al., 2007). We suggest that users of FTMS data do this before applying abundance-based ecological metrics to real-world datasets. This will provide objective guidance on how to use (and whether to avoid) specific metrics for specific FTMS datasets. The simulation model is the only tool we are aware of that can provide objective evaluations of uncertainty and potential biases associated with using FTMS peak intensities to compute ecological metrics. The model should not be taken as a static or mature tool, however. We encourage future work to expand it to include additional ecological metrics and/or analyses, situations with more than two samples, sample-to-sample variation in peak richness, links between peak richness and peak intensity, explicit molecular properties or traits, non-random errors, and measured levels of error between concentrations and peak intensities. It can be further extended to directly add error into peak intensities from real-world FTMS data and to re-calculate ecological metrics of interest across a range of introduced errors. This would help gauge the robustness of conceptual inferences derived from peak-intensity-based analyses.
Further evaluations are outside of the scope of this work but will be straightforward to include in future versions of the simulation model. We envision future studies customizing the model for their specific applications. For any real-world study, the model can be modified to include the actual number of samples, the number of peaks in each sample, the peak-intensity distributions, the number of replicates, and the specific ecological analyses that will be applied. In turn, simulation model outcomes can provide objective guidance tailored to each study. One may think of the resulting guidance as being akin to a power analysis, whereby the simulation can indicate what can and cannot be inferred from a given dataset. For example, the model indicates that observed Bray–Curtis values have little to no correspondence to true values when the Bray–Curtis value is below ∼ 0.2 (Fig. 8b and d). Bray–Curtis values near and below ∼ 0.2 are commonly observed in FTMS studies (Bao et al., 2018; Derrien et al., 2018; e.g., Hawkes et al., 2016), and this disconnect between observations and truth is maintained even with 1000 peaks per sample (Fig. 8d). In turn, FTMS studies that observe Bray–Curtis values below ∼ 0.2 may not be able to use those observations to make valid conceptual inferences. However, quantitative guidance must be developed for each study, and we recommend that a version of the simulation model should be used by future studies using peak intensities to conduct ecological analyses of FTMS data. It may be that, in time, we will understand the general rules well enough to leave the simulation behind, but, for now, we suggest that its use is warranted to ensure robust inferences.
In addition to further use and development of the simulation model, we recommend the translation of other modeling approaches for use with FTMS data. Two potential approaches are based on machine learning and hierarchical modeling. Machine learning could be used to model the instrument response for a diverse chemical space in typical environmental samples to learn how measured signal intensities may relate to true concentrations. Even if such a model does not yield high-accuracy results, it may, nonetheless, help us understand error and/or biases and may provide additional guidance for the robust use of peak-intensity data. Potentially in concert with machine learning, hierarchical modeling could be translated from its application in ecological analyses (Iknayan et al., 2014) for use with FTMS. This approach has been used to model sources of error that lead to variations in detectability across biological species, such as variations in species visibility (e.g., Dorazio and Royle, 2005). In turn, data can essentially be corrected by accounting for the modeled sources of error (Roth et al., 2018), even revealing “hidden diversity” (Richter et al., 2021). There are likely to be direct analogs to FTMS data in terms of variations among molecules in terms of detectability due to variations in terms of ionization and molecular interactions discussed in previous sections. Machine learning could be used to understand sources of error and, in turn, to inform hierarchical models aimed at improving the mapping between peak intensity and concentration. If successful, this would increase the quality of information provided by peak intensities in both existing and future datasets.
In summary, FTMS has many strengths and weaknesses, just like any analytical platform. Other types of compositional data also contain biases and uncertainties, such as the lack of true quantitation in sequence-based microbiome data (Gloor et al., 2017). Careful use of FTMS peak-intensity data informed by objective, model-based guidance can overcome some of its weaknesses. We encourage further development of the model presented here and the inclusion of additional methods developed to address issues that arise in similar data types (e.g., Gloor et al., 2017; Hardwick et al., 2018; Vieira-Silva et al., 2019). While these are important directions, we emphasize that despite peak intensities not necessarily reflecting concentrations, overall, ecological metrics appear to perform well. This is likely due to the law of large numbers as FTMS, especially FT-ICR MS, datasets often contain 1000 or more peaks per sample. Our simulation results indicate that large numbers of identified peaks allow ecological metrics to essentially track towards their true values. We are encouraged by this outcome and look forward to further applications of ecological metrics, concepts, and theories to NOM chemistry. Such advances could be greatly facilitated by a public database of standardized FT-ICR MS datasets paired with push-button execution of the simulation model for user-defined metrics and subsets of the database.
The R code for running the simulation models is available on GitHub: https://github.com/stegen/Peak_Intensity_Sims (Stegen, 2024). Python code used to process the empirical data and to generate the associated figures is available in Kew et al. (2024, https://doi.org/10.5281/zenodo.13887250).
Data are published in Kew et al. (2024) and are available at https://doi.org/10.5281/zenodo.13887250.
The supplement related to this article is available online at: https://doi.org/10.5194/bg-21-4665-2024-supplement.
WK contributed to the conceptualization, experimental data curation, formal analysis, methodology, software, visualization, writing of the original draft, and review and editing of the paper; AMP contributed to the conceptualization, methodology, visualization, writing of the original draft, and review and editing of the paper; CHC and SMC contributed to the investigation and review and editing of the paper; JE contributed to the sample preparation and review and editing of the paper; MMT contributed to the conceptualization, methodology, and review and editing of the paper; JH contributed to the conceptualization and review and editing of the paper; RKC contributed to the project administration, conceptualization, experimental data curation, methodology, funding acquisition, and review and editing of the paper; JCS contributed to the conceptualization, simulation data curation, formal analysis, funding acquisition, investigation, methodology, software, visualization, writing of the original draft, and review and editing of the paper.
The contact author has declared that none of the authors has any competing interests.
Publisher's note: Copernicus Publications remains neutral with regard to jurisdictional claims made in the text, published maps, institutional affiliations, or any other geographical representation in this paper. While Copernicus Publications makes every effort to include appropriate place names, the final responsibility lies with the authors.
A portion of this research was performed on a project award (https://doi.org/10.46936/intm.proj.2020.51667/60000248) from the Environmental Molecular Sciences Laboratory, a DOE Office of Science User Facility sponsored by the Biological and Environmental Research program under contract no. DE-AC05-76RL01830. James C. Stegen was also supported by a United States Department of Energy Early Career Award (grant no. 74193) at Pacific Northwest National Laboratory (PNNL), a multiprogram national laboratory operated by Battelle for the United States Department of Energy under contract no. DE-AC05-76RL01830. We thank Alan Roebuck for the useful feedback on the paper, Nathan Johnson for the graphics development, Charles T. Resch for supplying the artificial river water, and Patricia Miller and Jason Toyoda for the lab support.
This research has been supported by the Environmental Molecular Sciences Laboratory (grant nos. 22142, 74193).
This paper was edited by Jack Middelburg and reviewed by Qing-Zeng Zhu and three anonymous referees.
Anderson, M. J., Crist, T. O., Chase, J. M., Vellend, M., Inouye, B. D., Freestone, A. L., Sanders, N. J., Cornell, H. V., Comita, L. S., Davies, K. F., Harrison, S. P., Kraft, N. J. B., Stegen, J. C., and Swenson, N. G.: Navigating the multiple meanings of β diversity: a roadmap for the practicing ecologist, Ecol. Lett., 14, 19–28, https://doi.org/10.1111/j.1461-0248.2010.01552.x, 2011.
Bahureksa, W., Tfaily, M. M., Boiteau, R. M., Young, R. B., Logan, M. N., McKenna, A. M., and Borch, T.: Soil Organic Matter Characterization by Fourier Transform Ion Cyclotron Resonance Mass Spectrometry (FTICR MS): A Critical Review of Sample Preparation, Analysis, and Data Interpretation, Environ. Sci. Technol., 55, 9637–9656, https://doi.org/10.1021/acs.est.1c01135, 2021.
Bao, H., Niggemann, J., Luo, L., Dittmar, T., and Kao, S.-J.: Molecular composition and origin of water-soluble organic matter in marine aerosols in the Pacific off China, Atmos. Environ., 191, 27–35, https://doi.org/10.1016/j.atmosenv.2018.07.059, 2018.
Bhatia, M. P., Das, S. B., Longnecker, K., Charette, M. A., and Kujawinski, E. B.: Molecular characterization of dissolved organic matter associated with the Greenland ice sheet, Geochim. Cosmochim. Ac., 74, 3768–3784, https://doi.org/10.1016/j.gca.2010.03.035, 2010.
Boye, K., Noël, V., Tfaily, M. M., Bone, S. E., Williams, K. H., Bargar, J. R., and Fendorf, S.: Thermodynamically controlled preservation of organic carbon in floodplains, Nat. Geosci., 10, 415–419, https://doi.org/10.1038/ngeo2940, 2017.
Cao, D., Lv, J., Geng, F., Rao, Z., Niu, H., Shi, Y., Cai, Y., and Kang, Y.: Ion Accumulation Time Dependent Molecular Characterization of Natural Organic Matter Using Electrospray Ionization-Fourier Transform Ion Cyclotron Resonance Mass Spectrometry, Anal. Chem., 88, 12210–12218, https://doi.org/10.1021/acs.analchem.6b03198, 2016.
Cooper, W. T., Chanton, J. C., D'Andrilli, J., Hodgkins, S. B., Podgorski, D. C., Stenson, A. C., Tfaily, M. M., and Wilson, R. M.: A History of Molecular Level Analysis of Natural Organic Matter by FTICR Mass Spectrometry and The Paradigm Shift in Organic Geochemistry, Mass Spectrom. Rev., 41, 215–239, https://doi.org/10.1002/mas.21663, 2022.
Danczak, R. E., Chu, R. K., Fansler, S. J., Goldman, A. E., Graham, E. B., Tfaily, M. M., Toyoda, J., and Stegen, J. C.: Using metacommunity ecology to understand environmental metabolomes, Nat. Commun., 11, 6369, https://doi.org/10.1038/s41467-020-19989-y, 2020.
Danczak, R. E., Goldman, A. E., Chu, R. K., Toyoda, J. G., Garayburu-Caruso, V. A., Tolić, N., Graham, E. B., Morad, J. W., Renteria, L., Wells, J. R., Herzog, S. P., Ward, A. S., and Stegen, J. C.: Ecological theory applied to environmental metabolomes reveals compositional divergence despite conserved molecular properties, Sci. Total Environ., 788, 147409, https://doi.org/10.1016/j.scitotenv.2021.147409, 2021.
Derrien, M., Lee, Y. K., Shin, K.-H., and Hur, J.: Comparing discrimination capabilities of fluorescence spectroscopy versus FT-ICR-MS for sources and hydrophobicity of sediment organic matter, Environ. Sci. Pollut. Res., 25, 1892–1902, https://doi.org/10.1007/s11356-017-0531-z, 2018.
Dorazio, R. M. and Royle, J. A.: Estimating Size and Composition of Biological Communities by Modeling the Occurrence of Species, J. Am. Stat. Assoc., 100, 389–398, https://doi.org/10.1198/016214505000000015, 2005.
Elliott, K. J., Boring, L. R., Swank, W. T., and Haines, B. R.: Successional changes in plant species diversity and composition after clearcutting a Southern Appalachian watershed, Forest. Ecol. Manag., 92, 67–85, https://doi.org/10.1016/S0378-1127(96)03947-3, 1997.
Garayburu-Caruso, V. A., Stegen, J. C., Song, H.-S., Renteria, L., Wells, J., Garcia, W., Resch, C. T., Goldman, A. E., Chu, R. K., Toyoda, J., and Graham, E. B.: Carbon Limitation Leads to Thermodynamic Regulation of Aerobic Metabolism, Environ. Sci. Tech. Let., 7, 517–524, https://doi.org/10.1021/acs.estlett.0c00258, 2020.
Gloor, G. B., Macklaim, J. M., Pawlowsky-Glahn, V., and Egozcue, J. J.: Microbiome Datasets Are Compositional: And This Is Not Optional, Front. Microbiol., 8, 2224, https://doi.org/10.3389/fmicb.2017.02224, 2017.
Han, L., Kaesler, J., Peng, C., Reemtsma, T., and Lechtenfeld, O. J.: Online Counter Gradient LC-FT-ICR-MS Enables Detection of Highly Polar Natural Organic Matter Fractions, Anal. Chem., 93, 1740–1748, https://doi.org/10.1021/acs.analchem.0c04426, 2021.
Hardwick, S. A., Chen, W. Y., Wong, T., Kanakamedala, B. S., Deveson, I. W., Ongley, S. E., Santini, N. S., Marcellin, E., Smith, M. A., Nielsen, L. K., Lovelock, C. E., Neilan, B. A., and Mercer, T. R.: Synthetic microbe communities provide internal reference standards for metagenome sequencing and analysis, Nat. Commun., 9, 3096, https://doi.org/10.1038/s41467-018-05555-0, 2018.
Hawkes, J. A. and Kew, W.: 4 – High-resolution mass spectrometry strategies for the investigation of dissolved organic matter, in: Multidimensional Analytical Techniques in Environmental Research, edited by: Duarte, R. M. B. O. and Duarte, A. C., Elsevier, https://doi.org/10.1016/B978-0-12-818896-5.00004-1, 71–104, 2020.
Hawkes, J. A., Dittmar, T., Patriarca, C., Tranvik, L., and Bergquist, J.: Evaluation of the Orbitrap Mass Spectrometer for the Molecular Fingerprinting Analysis of Natural Dissolved Organic Matter, Anal. Chem., 88, 7698–7704, https://doi.org/10.1021/acs.analchem.6b01624, 2016.
Hedges, J. I., Eglinton, G., Hatcher, P. G., Kirchman, D. L., Arnosti, C., Derenne, S., Evershed, R. P., Kögel-Knabner, I., de Leeuw, J. W., Littke, R., Michaelis, W., and Rullkötter, J.: The molecularly-uncharacterized component of nonliving organic matter in natural environments, Org. Geochem., 31, 945–958, https://doi.org/10.1016/S0146-6380(00)00096-6, 2000.
Hill, M. O.: Diversity and Evenness: A Unifying Notation and Its Consequences, Ecology, 54, 427–432, https://doi.org/10.2307/1934352, 1973.
Iknayan, K. J., Tingley, M. W., Furnas, B. J., and Beissinger, S. R.: Detecting diversity: emerging methods to estimate species diversity, Trends Ecol. Evol., 29, 97–106, https://doi.org/10.1016/j.tree.2013.10.012, 2014.
Kaiser, N. K., McKenna, A. M., Savory, J. J., Hendrickson, C. L., and Marshall, A. G.: Tailored Ion Radius Distribution for Increased Dynamic Range in FT-ICR Mass Analysis of Complex Mixtures, Anal. Chem., 85, 265–272, https://doi.org/10.1021/ac302678v, 2013.
Kellerman, A. M., Dittmar, T., Kothawala, D. N., and Tranvik, L. J.: Chemodiversity of dissolved organic matter in lakes driven by climate and hydrology, Nat. Commun., 5, 3804, https://doi.org/10.1038/ncomms4804, 2014.
Kew, W., Myers-Pigg, A. N., Chang, C. H., Eder, J., Colby, S. M., Tfaily, M., Hawkes, J., Chu, R., and Stegen, J.: FTICR MS data for standards and mixtures for quantitative peak intensity investigation, Zenodo [data set and code], https://doi.org/10.5281/zenodo.13887250, 2024.
Kim, D., Kim, S., Son, S., Jung, M.-J., and Kim, S.: Application of Online Liquid Chromatography 7 T FT-ICR Mass Spectrometer Equipped with Quadrupolar Detection for Analysis of Natural Organic Matter, Anal. Chem., 91, 7690–7697, https://doi.org/10.1021/acs.analchem.9b00689, 2019.
Kruve, A.: Strategies for Drawing Quantitative Conclusions from Nontargeted Liquid Chromatography–High-Resolution Mass Spectrometry Analysis, Anal. Chem., 92, 4691–4699, https://doi.org/10.1021/acs.analchem.9b03481, 2020.
Kruve, A., Kaupmees, K., Liigand, J., and Leito, I.: Negative Electrospray Ionization via Deprotonation: Predicting the Ionization Efficiency, Anal. Chem., 86, 4822–4830, https://doi.org/10.1021/ac404066v, 2014.
Kujawinski, E. B.: Electrospray Ionization Fourier Transform Ion Cyclotron Resonance Mass Spectrometry (ESI FT-ICR MS): Characterization of Complex Environmental Mixtures, Environ. Forensics, 3, 207–216, https://doi.org/10.1006/enfo.2002.0109, 2002.
Kujawinski, E. B., Longnecker, K., Blough, N. V., Vecchio, R. D., Finlay, L., Kitner, J. B., and Giovannoni, S. J.: Identification of possible source markers in marine dissolved organic matter using ultrahigh resolution mass spectrometry, Geochim. Cosmochim. Ac., 73, 4384–4399, https://doi.org/10.1016/j.gca.2009.04.033, 2009.
Laliberté, E. and Legendre, P.: A distance-based framework for measuring functional diversity from multiple traits, Ecology, 91, 299–305, https://doi.org/10.1890/08-2244.1, 2010.
LaRowe, D. E. and Van Cappellen, P.: Degradation of natural organic matter: A thermodynamic analysis, Geochim. Cosmochim. Ac., 75, 2030–2042, https://doi.org/10.1016/j.gca.2011.01.020, 2011.
Lavorel, S., Grigulis, K., McIntyre, S., Williams, N. S. G., Garden, D., Dorrough, J., Berman, S., Quétier, F., Thébault, A., and Bonis, A.: Assessing functional diversity in the field – methodology matters!, Funct. Ecol., 22, 134–147, https://doi.org/10.1111/j.1365-2435.2007.01339.x, 2008.
Leyva, D., Tose, L. V., Porter, J., Wolff, J., Jaffé, R., and Fernandez-Lima, F.: Understanding the structural complexity of dissolved organic matter: isomeric diversity, Faraday Discuss., 218, 431–440, https://doi.org/10.1039/C8FD00221E, 2019.
Leyva, D., Jaffe, R., and Fernandez-Lima, F.: Structural Characterization of Dissolved Organic Matter at the Chemical Formula Level Using TIMS-FT-ICR MS/MS, Anal. Chem., 92, 11960–11966, https://doi.org/10.1021/acs.analchem.0c02347, 2020.
Li, H.-Y., Wang, H., Wang, H.-T., Xin, P.-Y., Xu, X.-H., Ma, Y., Liu, W.-P., Teng, C.-Y., Jiang, C.-L., Lou, L.-P., Arnold, W., Cralle, L., Zhu, Y.-G., Chu, J.-F., Gilbert, J. A., and Zhang, Z.-J.: The chemodiversity of paddy soil dissolved organic matter correlates with microbial community at continental scales, Microbiome, 6, 187, https://doi.org/10.1186/s40168-018-0561-x, 2018.
Li, Y., Harir, M., Uhl, J., Kanawati, B., Lucio, M., Smirnov, K. S., Koch, B. P., Schmitt-Kopplin, P., and Hertkorn, N.: How representative are dissolved organic matter (DOM) extracts? A comprehensive study of sorbent selectivity for DOM isolation, Water Res., 116, 316–323, https://doi.org/10.1016/j.watres.2017.03.038, 2017.
Lucas, J., Koester, I., Wichels, A., Niggemann, J., Dittmar, T., Callies, U., Wiltshire, K. H., and Gerdts, G.: Short-Term Dynamics of North Sea Bacterioplankton-Dissolved Organic Matter Coherence on Molecular Level, Front. Microbiol., 7, 321, https://doi.org/10.3389/fmicb.2016.00321, 2016.
Makarov, A., Denisov, E., Kholomeev, A., Balschun, W., Lange, O., Strupat, K., and Horning, S.: Performance Evaluation of a Hybrid Linear Ion Trap/Orbitrap Mass Spectrometer, Anal. Chem., 78, 2113–2120, https://doi.org/10.1021/ac0518811, 2006.
Makarov, A., Grinfeld, D., and Ayzikov, K.: Chapter 2 – Fundamentals of Orbitrap analyzer, in: Fundamentals and Applications of Fourier Transform Mass Spectrometry, edited by: Kanawati, B. and Schmitt-Kopplin, P., Elsevier, https://doi.org/10.1016/B978-0-12-814013-0.00002-8, 37–61, 2019.
Marshall, A. G., Hendrickson, C. L., and Jackson, G. S.: Fourier transform ion cyclotron resonance mass spectrometry: A primer, Mass Spectrom. Rev., 17, 1–35, https://doi.org/10.1002/(SICI)1098-2787(1998)17:1<1::AID-MAS1>3.0.CO;2-K, 1998.
McGill, B. J., Enquist, B. J., Weiher, E., and Westoby, M.: Rebuilding community ecology from functional traits, Trends Ecol. Evol., 21, 178–185, https://doi.org/10.1016/j.tree.2006.02.002, 2006.
McGill, B. J., Etienne, R. S., Gray, J. S., Alonso, D., Anderson, M. J., Benecha, H. K., Dornelas, M., Enquist, B. J., Green, J. L., He, F., Hurlbert, A. H., Magurran, A. E., Marquet, P. A., Maurer, B. A., Ostling, A., Soykan, C. U., Ugland, K. I., and White, E. P.: Species abundance distributions: moving beyond single prediction theories to integration within an ecological framework, Ecol. Lett., 10, 995–1015, https://doi.org/10.1111/j.1461-0248.2007.01094.x, 2007.
Merder, J., Röder, H., Dittmar, T., Feudel, U., Freund, J. A., Gerdts, G., Kraberg, A., and Niggemann, J.: Dissolved organic compounds with synchronous dynamics share chemical properties and origin, Limnol. Oceanogr., 66, 4001–4016, https://doi.org/10.1002/lno.11938, 2021.
Mouillot, D. and Leprêtre, A.: A comparison of species diversity estimators, Res. Popul. Ecol., 41, 203–215, https://doi.org/10.1007/s101440050024, 1999.
Muscarella, R. and Uriarte, M.: Do community-weighted mean functional traits reflect optimal strategies?, P. R. Soc. B, 283, 20152434, https://doi.org/10.1098/rspb.2015.2434, 2016.
Osterholz, H., Singer, G., Wemheuer, B., Daniel, R., Simon, M., Niggemann, J., and Dittmar, T.: Deciphering associations between dissolved organic molecules and bacterial communities in a pelagic marine system, ISME J., 10, 1717–1730, https://doi.org/10.1038/ismej.2015.231, 2016.
Raeke, J., Lechtenfeld, O. J., Wagner, M., Herzsprung, P., and Reemtsma, T.: Selectivity of solid phase extraction of freshwater dissolved organic matter and its effect on ultrahigh resolution mass spectra, Environ. Sci. Process. Impacts, 18, 918–927, https://doi.org/10.1039/C6EM00200E, 2016.
Redowan, M.: Spatial pattern of tree diversity and evenness across forest types in Majella National Park, Italy, For. Ecosyst., 2, 24, https://doi.org/10.1186/s40663-015-0048-1, 2015.
Richter, A., Nakamura, G., Agra Iserhard, C., and da Silva Duarte, L.: The hidden side of diversity: Effects of imperfect detection on multiple dimensions of biodiversity, Ecol. Evol., 11, 12508–12519, https://doi.org/10.1002/ece3.7995, 2021.
Roth, T., Allan, E., Pearman, P. B., and Amrhein, V.: Functional ecology and imperfect detection of species, Methods Ecol. Evol., 9, 917–928, https://doi.org/10.1111/2041-210X.12950, 2018.
Roth, V.-N., Lange, M., Simon, C., Hertkorn, N., Bucher, S., Goodall, T., Griffiths, R. I., Mellado-Vázquez, P. G., Mommer, L., Oram, N. J., Weigelt, A., Dittmar, T., and Gleixner, G.: Persistence of dissolved organic matter explained by molecular changes during its passage through soil, Nat. Geosci., 12, 755–761, https://doi.org/10.1038/s41561-019-0417-4, 2019.
Ruddy, B. M., Hendrickson, C. L., Rodgers, R. P., and Marshall, A. G.: Positive Ion Electrospray Ionization Suppression in Petroleum and Complex Mixtures, Energy Fuels, 32, 2901–2907, https://doi.org/10.1021/acs.energyfuels.7b03204, 2018.
Sakas, J., Kitson, E., Bell, N. G. A., and Uhrín, D.: MS and NMR Analysis of Isotopically Labeled Chloramination Disinfection Byproducts: Hyperlinks and Chemical Reactions, Anal. Chem., 96, 8263–8272, https://doi.org/10.1021/acs.analchem.3c03888, 2024.
Senko, M. W., Hendrickson, C. L., Emmett, M. R., Shi, S. D.-H., and Marshall, A. G.: External Accumulation of Ions for Enhanced Electrospray Ionization Fourier Transform Ion Cyclotron Resonance Mass Spectrometry, J. Am. Soc. Mass Spectrom., 8, 970–976, https://doi.org/10.1016/S1044-0305(97)00126-8, 1997.
Shaw, J. B., Lin, T.-Y., Leach, F. E., Tolmachev, A. V., Tolić, N., Robinson, E. W., Koppenaal, D. W., and Paša-Tolić, L.: 21 Tesla Fourier Transform Ion Cyclotron Resonance Mass Spectrometer Greatly Expands Mass Spectrometry Toolbox, J. Am. Soc. Mass Spectrom., 27, 1929–1936, https://doi.org/10.1007/s13361-016-1507-9, 2016.
Smith, D. F., Podgorski, D. C., Rodgers, R. P., Blakney, G. T., and Hendrickson, C. L.: 21 Tesla FT-ICR Mass Spectrometer for Ultrahigh-Resolution Analysis of Complex Organic Mixtures, Anal. Chem., 90, 2041–2047, https://doi.org/10.1021/acs.analchem.7b04159, 2018.
Spencer, R. G. M., Mann, P. J., Dittmar, T., Eglinton, T. I., McIntyre, C., Holmes, R. M., Zimov, N., and Stubbins, A.: Detecting the signature of permafrost thaw in Arctic rivers, Geophys. Res. Lett., 42, 2830–2835, https://doi.org/10.1002/2015GL063498, 2015.
Steen, A. D., Kusch, S., Abdulla, H. A., Cakić, N., Coffinet, S., Dittmar, T., Fulton, J. M., Galy, V., Hinrichs, K.-U., Ingalls, A. E., Koch, B. P., Kujawinski, E., Liu, Z., Osterholz, H., Rush, D., Seidel, M., Sepúlveda, J., and Wakeham, S. G.: Analytical and Computational Advances, Opportunities, and Challenges in Marine Organic Biogeochemistry in an Era of “Omics”, Front. Mar. Sci., 7, 718, https://doi.org/10.3389/fmars.2020.00718, 2020.
Stegen, J. C.: Peak_Intensity_Sims, GitHub [code], https://github.com/stegen/Peak_Intensity_Sims, last access: 10 October 2024.
Stubbins, A., Spencer, R. G. M., Chen, H., Hatcher, P. G., Mopper, K., Hernes, P. J., Mwamba, V. L., Mangangu, A. M., Wabakanghanzi, J. N., and Six, J.: Illuminated darkness: Molecular signatures of Congo River dissolved organic matter and its photochemical alteration as revealed by ultrahigh precision mass spectrometry, Limnol. Oceanogr., 55, 1467–1477, https://doi.org/10.4319/lo.2010.55.4.1467, 2010.
Tanentzap, A. J., Fitch, A., Orland, C., Emilson, E. J. S., Yakimovich, K. M., Osterholz, H., and Dittmar, T.: Chemical and microbial diversity covary in fresh water to influence ecosystem functioning, P. Natl. Acad. Sci. USA, 116, 24689–24695, https://doi.org/10.1073/pnas.1904896116, 2019.
Thompson, A. M., Stratton, K. G., Bramer, L. M., Zavoshy, N. S., and McCue, L. A.: Fourier transform ion cyclotron resonance mass spectrometry (FT-ICR-MS) peak intensity normalization for complex mixture analyses, Rapid Commun. Mass Sp., 35, e9068, https://doi.org/10.1002/rcm.9068, 2021.
Tose, L. V., Benigni, P., Leyva, D., Sundberg, A., Ramírez, C. E., Ridgeway, M. E., Park, M. A., Romão, W., Jaffé, R., and Fernandez-Lima, F.: Coupling trapped ion mobility spectrometry to mass spectrometry: trapped ion mobility spectrometry–time-of-flight mass spectrometry versus trapped ion mobility spectrometry–Fourier transform ion cyclotron resonance mass spectrometry, Rapid Commun. Mass Sp., 32, 1287–1295, https://doi.org/10.1002/rcm.8165, 2018.
Trufelli, H., Palma, P., Famiglini, G., and Cappiello, A.: An overview of matrix effects in liquid chromatography–mass spectrometry, Mass Spectrom. Rev., 30, 491–509, https://doi.org/10.1002/mas.20298, 2011.
Urban, P. L.: Quantitative mass spectrometry: an overview, Philos. T. R. Soc. A, 374, 20150382, https://doi.org/10.1098/rsta.2015.0382, 2016.
Vieira-Silva, S., Sabino, J., Valles-Colomer, M., Falony, G., Kathagen, G., Caenepeel, C., Cleynen, I., van der Merwe, S., Vermeire, S., and Raes, J.: Quantitative microbiome profiling disentangles inflammation- and bile duct obstruction-associated microbiota alterations across PSC/IBD diagnoses, Nat. Microbiol., 4, 1826–1831, https://doi.org/10.1038/s41564-019-0483-9, 2019.
Villéger, S., Brosse, S., Mouchet, M., Mouillot, D., and Vanni, M. J.: Functional ecology of fish: current approaches and future challenges, Aquat. Sci., 79, 783–801, https://doi.org/10.1007/s00027-017-0546-z, 2017.
Violle, C., Navas, M.-L., Vile, D., Kazakou, E., Fortunel, C., Hummel, I., and Garnier, E.: Let the concept of trait be functional!, Oikos, 116, 882–892, https://doi.org/10.1111/j.0030-1299.2007.15559.x, 2007.
Wen, Z., Shang, Y., Lyu, L., Liu, G., Hou, J., He, C., Shi, Q., He, D., and Song, K.: Sources and composition of riverine dissolved organic matter to marginal seas from mainland China, J. Hydrol., 127152, https://doi.org/10.1016/j.jhydrol.2021.127152, 2021.
Whittaker, R. H.: Evolution and Measurement of Species Diversity, TAXON, 21, 213–251, https://doi.org/10.2307/1218190, 1972.
Wörner, T. P., Snijder, J., Bennett, A., Agbandje-McKenna, M., Makarov, A. A., and Heck, A. J. R.: Resolving heterogeneous macromolecular assemblies by Orbitrap-based single-particle charge detection mass spectrometry, Nat. Methods, 17, 395–398, https://doi.org/10.1038/s41592-020-0770-7, 2020.
Zark, M., Christoffers, J., and Dittmar, T.: Molecular properties of deep-sea dissolved organic matter are predictable by the central limit theorem: Evidence from tandem FT-ICR-MS, Mar. Chem., 191, 9–15, https://doi.org/10.1016/j.marchem.2017.02.005, 2017.
- Abstract
- Introduction
- Theoretical foundations
- Empirical evaluations
- Conceptual implications for the use of ecological metrics
- Ecological metrics using peak intensities are often robust
- Conclusions and recommendations
- Code availability
- Data availability
- Author contributions
- Competing interests
- Disclaimer
- Acknowledgements
- Financial support
- Review statement
- References
- Supplement
- Article
(4331 KB) - Full-text XML
- Abstract
- Introduction
- Theoretical foundations
- Empirical evaluations
- Conceptual implications for the use of ecological metrics
- Ecological metrics using peak intensities are often robust
- Conclusions and recommendations
- Code availability
- Data availability
- Author contributions
- Competing interests
- Disclaimer
- Acknowledgements
- Financial support
- Review statement
- References
- Supplement