the Creative Commons Attribution 4.0 License.
the Creative Commons Attribution 4.0 License.
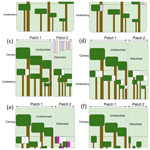
Assessing impacts of selective logging on water, energy, and carbon budgets and ecosystem dynamics in Amazon forests using the Functionally Assembled Terrestrial Ecosystem Simulator
Yi Xu
Marcos Longo
Michael Keller
Ryan G. Knox
Charles D. Koven
Rosie A. Fisher
Tropical forest degradation from logging, fire, and fragmentation not only alters carbon stocks and carbon fluxes, but also impacts physical land surface properties such as albedo and roughness length. Such impacts are poorly quantified to date due to difficulties in accessing and maintaining observational infrastructures, as well as the lack of proper modeling tools for capturing the interactions among biophysical properties, ecosystem demography, canopy structure, and biogeochemical cycling in tropical forests. As a first step to address these limitations, we implemented a selective logging module into the Functionally Assembled Terrestrial Ecosystem Simulator (FATES) by mimicking the ecological, biophysical, and biogeochemical processes following a logging event. The model can specify the timing and aerial extent of logging events, splitting the logged forest patch into disturbed and intact patches; determine the survivorship of cohorts in the disturbed patch; and modifying the biomass and necromass (total mass of coarse woody debris and litter) pools following logging. We parameterized the logging module to reproduce a selective logging experiment at the Tapajós National Forest in Brazil and benchmarked model outputs against available field measurements. Our results suggest that the model permits the coexistence of early and late successional functional types and realistically characterizes the seasonality of water and carbon fluxes and stocks, the forest structure and composition, and the ecosystem succession following disturbance. However, the current version of FATES overestimates water stress in the dry season and therefore fails to capture seasonal variation in latent and sensible heat fluxes. Moreover, we observed a bias towards low stem density and leaf area when compared to observations, suggesting that improvements are needed in both carbon allocation and establishment of trees. The effects of logging were assessed by different logging scenarios to represent reduced impact and conventional logging practices, both with high and low logging intensities. The model simulations suggest that in comparison to old-growth forests the logged forests rapidly recover water and energy fluxes in 1 to 3 years. In contrast, the recovery times for carbon stocks, forest structure, and composition are more than 30 years depending on logging practices and intensity. This study lays the foundation to simulate land use change and forest degradation in FATES, which will be an effective tool to directly represent forest management practices and regeneration in the context of Earth system models.
- Article
(6764 KB) - Full-text XML
-
Supplement
(569 KB) - BibTeX
- EndNote
Land cover and land use in tropical forest regions are highly dynamic, and nearly all tropical forests are subject to significant human influence (Martínez-Ramos et al., 2016; Dirzo et al., 2014). While old-growth tropical forests have been reported to be carbon sinks that remove carbon dioxide from the atmosphere through photosynthesis, these forests could easily become carbon sources once disturbed (Luyssaert et al., 2008). Using data from forest inventory and long-term ecosystem carbon studies from 1990 to 2007, Pan et al. (2011) suggested a net tropical forest can be a net source of carbon source of 1.3±0.7 Pg C yr−1 from land use change, consisting of a gross tropical deforestation loss of 2.9±0.5 Pg C yr−1 that is partially offset by a carbon uptake by tropical secondary forest regrowth of 1.6±0.5 Pg C yr−1. These estimates, however, do not account for a tropical forest that has been degraded through the combined effects of selective logging (cutting and removal of merchantable timber), fuelwood harvest, understory fires, and fragmentation (Nepstad et al., 1999; Bradshaw et al., 2009). To date, the effects of forest degradation remain poorly quantified. Recent studies suggested that degradation may contribute to carbon loss 40 % as large as clear-cut deforestation (Berenguer et al., 2014), and the emission from selective logging alone could be equivalent to ∼10 % to 50 % of that from deforestation in the tropical countries (Pearson et al., 2014; Huang and Asner, 2010; Asner et al., 2009). Selective logging of tropical forests is an important contributor to many local and national economies and corresponds to approximately one-eighth of global timber (Blaser et al., 2011). The integrated impact of timber production and other forest uses has been posited as the cause of up to ∼30 % of the difference between potential and actual biomass stocks globally, comparable in magnitude to the effects of deforestation (Erb et al., 2017). Selective logging includes cutting large trees and additional degradation through widespread damage to remaining trees, subcanopy vegetation, and soils (Asner et al., 2004; Asner et al., 2005). Selective logging accelerates gap-phase regeneration within the degraded forests (Huang et al., 2008).
Over half of all tropical forests have been cleared or logged, and almost half of standing old-growth tropical forests are designated by national forest services for timber production (Sist et al., 2015). Disturbances that result from logging are known to cause forest degradation at the same magnitude as deforestation each year in terms of both geographic extent and intensity, with widespread collateral damage to remaining trees, vegetation, and soils, leading to disturbance to water, energy, and carbon cycling, as well as ecosystem integrity (Keller et al., 2004b; Asner et al., 2004; Huang and Asner, 2010).
In most Earth system models (ESMs) that couple terrestrial and atmospheric processes to investigate global change (e.g., the Community Earth System Model or the Energy Exascale Earth System Model), selective logging is typically represented as simple fractions of affected area or an amount of carbon to be removed on a coarse grid (e.g., 0.5∘). One exception is the representation of wood harvest in the LM3V land model that explicitly accounts for postdisturbance land age distribution, as part of the Geophysical Fluid Dynamics Laboratory (GFDL) Earth system model (Shevliakova et al., 2009). In the ESMs, grid cell fractional areas are typically based on timber production rates estimated from sawmill, sales, and export statistics (Hurtt et al., 2011; Lawrence et al., 2012). This approach, while practical, does not effectively differentiate selective logging that retains forest cover from deforestation.
The realistic representation of wood harvest was absent in most ESMs because the models generally did not represent the demographic structure of forests (tree size and stem number distributions; Bonan, 2008). But progress over the past two decades in ecological theory and observations (Bustamante et al., 2016; Strigul et al., 2008; Hurtt et al., 1998; Moorcroft et al., 2001) has made it feasible to include vegetation demography more directly into Earth system models through individual to cohort-based vegetation in land models (Sato et al., 2007; Watanabe et al., 2011; Smith et al., 2001; Smith et al., 2014; Weng et al., 2015; Baidya Roy et al., 2003; Hurtt et al., 1998; Fisher et al., 2015). These vegetation demography modules are relatively new in land models, so efforts are still underway to improve their parameterizations of resource competition for light, water, nutrients, recruitment, mortality, and disturbance including both natural and anthropogenic components (Fisher et al., 2017).
In this study, we aim to (1) describe the development of a selective logging module implemented into The Functionally Assembled Terrestrial Ecosystem Simulator (FATES), for simulating anthropogenic disturbances of various intensities to forest ecosystems and their short-term and long-term effects on water, energy, carbon cycling, and ecosystem dynamics; (2) assess the capability of FATES in simulating site-level water, energy, and carbon budgets, as well as forest structure and composition; (3) benchmark the simulated variables against available observations at the Tapajós National Forest in the Amazon, thus identifying potential directions for model improvement; and (4) assess the simulated recovery trajectory of tropical forest following disturbance under various logging scenarios. In Sect. 2, we provide a brief summary of FATES, introduce the new selective logging module, and describe numerical experiments performed at two sites with data from field surveys and flux towers. In Sect. 3, FATES-simulated water, energy, and carbon fluxes and stocks in intact and disturbed forests are compared to available observations, and the effects of logging practice and intensity on simulated forest recovery trajectory in terms of carbon budget, size structure, and composition in plant functional types are assessed. Conclusions and future work are discussed in Sect. 4.
2.1 The Functionally Assembled Terrestrial Ecosystem Simulator
The Functionally Assembled Terrestrial Ecosystem Simulator (FATES) has been developed as a numerical terrestrial ecosystem model based on the ecosystem demography representation in the community land model (CLM), formerly known as CLM(ED) (Fisher et al., 2015). FATES is an implementation of the cohort-based ecosystem demography (ED) concept (Hurtt et al., 1998; Moorcroft et al., 2001) that can be called as a library from an ESM land surface scheme, currently including the CLM (Oleson et al., 2013) or Energy Exascale Earth System Model (E3SM) land model (ELM) (https://climatemodeling.science.energy.gov/projects/energy-exascale-earth-system-model, last access: 28 June 2020). In FATES, the landscape is discretized into spatially implicit patches, each of which represents land areas with a similar age since last disturbance. The discretization of ecosystems along a disturbance–recovery axis allows the deterministic simulation of successional dynamics within a typical forest ecosystem. Within each patch, individuals are grouped into cohorts by plant functional types (PFTs) and size classes (SCs), so that cohorts can compete for light based on their heights and canopy positions. Following disturbance, a patch fission process splits the original patch into undisturbed and disturbed new patches. A patch fusion mechanism is implemented to merge patches with similar structures, which helps prevent the number of patches from growing too big. In addition to the ED concept, FATES also adopted a modified version of the perfect plasticity approximation (PPA) (Strigul et al., 2008) concept by splitting growing cohorts between canopy and understory layers as a continuous function of height designed for increasing the probability of coexistence (Fisher et al., 2010). An earlier version of FATES, CLM(ED), has been applied regionally to explore the sensitivity of biome boundaries to plant trait representation (Fisher et al., 2015).
In this study, we specified two plant functional types (PFTs) in FATES corresponding to early successional and late successional plants, representative of the primary axis of variability in tropical forests (Reich, 2014). The early successional PFT is light demanding and grows rapidly under high-light conditions common prior to canopy closure. This PFT has low-density woody tissues, shorter leaf and root lifetimes, and a higher background mortality compared to the late successional PFT that has dense woody tissues, longer leaf and root lifetimes, and lower background mortality (Brokaw, 1985; Whitmore, 1998) and thus can survive under deep shade and grow slowly under closed canopy.
The key parameters that differentiate the two PFTs in FATES are listed in Table 1, including specific leaf area at the canopy top (SLA0), the maximum rate of carboxylation at 25 ∘C (Vcmax25), specific wood density, background mortality, leaf and fine root longevity, and leaf C:N ratio. The parameter ranges were selected based on literature for tropical forests. Specifically, it has been reported that SLA values ranges from 0.007 to 0.039 m2 g C−1 (Wright et al., 2004) and Vcmax25 ranges between 10.1 and 105.7 µmol m−2 s−1 (Domingues et al., 2005). The specific wood densities were set to be 0.5 and 0.9 g cm3 , and the background mortality rates were set to 0.035 and 0.014 yr−1 for early and late succession PFTs respectively, consistent with those used in the Ecosystem Demography Model version 2 for Amazon forests (Longo et al., 2019) . For simplicity, leaf longevity and root longevity were set to be the same for each PFT (i.e., 0.9 and 2.6 years for early and late successional PFTs) following the range in Trumbore and Barbosa De Camargo (2009).
Given that both SLA0 and Vcmax25 span wide ranges and have been identified as the most sensitive parameters in FATES in a previous study (Massoud et al., 2019), we performed one-at-a-time sensitivity tests by perturbing them within the reported ranges. Based on these tests, it is evident that these parameters not only affect water, energy, and carbon budget simulations, but also the coexistence of the two PFTs. In the version of FATES used in this study (Interested readers are referred to the “Code and data availability” section for details), coexistence of PFTs is not assured for all parameter combinations, even if they are both within reasonable ranges, on account of competitive exclusion feedback processes that prevent coexistence in the presence of large discrepancies in plant growth and reproduction rates (Fisher et al., 2010; Bohn et al., 2011). In order to demonstrate FATES' capability in simulating water, energy, carbon budgets, and forest structure and composition in a holistic way, we chose to report results based on a set of parameter values that produces reasonable, stable fractions of two PFTs, as reported in Table 1. Nevertheless, we have included a summary of all sensitivity tests performed in the Supplement for completeness. The sensitivity tests demonstrated that, by tuning SLA0 and Vcmax25 for the different PFTs, FATES is not only capable of capturing coexistence of PFTs, but also capable of reproducing observed water, energy, and carbon cycle fluxes in the tropics.
2.2 The selective logging module
The new selective logging module in FATES mimics the ecological, biophysical, and biogeochemical processes following a logging event. The module (1) specifies the timing and areal extent of a logging event; (2) calculates the fractions of trees that are damaged by direct felling, collateral damage, and infrastructure damage and adds these size-specific plant mortality types to FATES; (3) splits the logged patch into disturbed and intact new patches; (4) applies the calculated survivorship to cohorts in the disturbed patch; and (5) transports harvested logs off-site by reducing site carbon pools and adds remaining necromass to coarse woody debris and litter pools.
The logging module structure and parameterization are based on detailed field and remote sensing studies (Putz et al., 2008; Asner et al., 2004; Pereira et al., 2002; Asner et al., 2005; Feldpausch et al., 2005). Logging infrastructure including roads, skids, trails, and log decks are conceptually represented (Fig. 1). The construction of log decks used to store logs prior to road transport leads to large canopy openings, but their contribution to landscape-level gap dynamics is small. In contrast, the canopy gaps caused by tree felling are small, but their coverage is spatially extensive at the landscape scale. Variations in logging practices significantly affect the level of disturbance to tropical forests following logging (Pereira et al., 2002; Macpherson et al., 2012; Dykstra, 2002; Putz et al., 2008). Logging operations in the tropics are often carried out with little planning and typically use heavy machinery to access the forests accompanied by construction of excessive roads and skid trails, leading to unnecessary tree fall and compaction of the soil. We refer to these typical operations as conventional logging (CL). In contrast, reduced impact logging (RIL) is a practice with extensive preharvest planning, where trees are inventoried and mapped out for the most efficient and cost-effective harvest and seed trees are deliberately left on-site to facilitate faster recovery. Through planning, the construction of skid trails and roads, soil compaction, and disturbance can be minimized. Vines connecting trees are cut, and tree-fall directions are controlled to reduce damages to surrounding trees. Reduced impact logging results in consistently less disturbance to forests than conventional logging (Pereira et al., 2002; Putz et al., 2008).
The FATES logging module was designed to represent a range of logging practices in field operations at a landscape level. Both CL and RIL can be represented in FATES by specifying mortality-rate-associated direct felling, collateral damages, and mechanical damages as follows: once logging events are activated, we define three types of mortality associated with logging practices – direct-felling mortality (lmortdirect), collateral mortality (lmortcollateral), and mechanical mortality (lmortmechanical). The direct-felling mortality represents the fraction of trees selected for harvesting that are greater than or equal to a diameter threshold (this threshold is defined by the diameter at breast height (DBH) = 1.3 m denoted as DBHmin); collateral mortality denotes the fraction of adjacent trees that are killed by felling of the harvested trees; and the mechanical mortality represents the fraction of trees killed by construction of log decks, skid trails, and roads for accessing the harvested trees, as well as storing and transporting logs off-site (Fig. 1a). In a logging operation, the loggers typically avoid large trees when they build log decks, skids, and trails by knocking down relatively small trees as it is not economical to knock down large trees. Therefore, we implemented another DBH threshold, DBH, so that only a fraction of trees ≤ DBH (called mechanical damage fraction) are removed for building infrastructure (Feldpausch et al., 2005).
To capture the disturbance mechanisms and degree of damage associated with logging practices at the landscape level, we apply the mortality types following a workflow designed to correspond to field operations. In FATES, as illustrated in Fig. 2, individual trees of all plant functional types (PFTs) in one patch are grouped into cohorts of similar-sized trees, whose size and population sizes evolve in time through processes of recruitment, growth, and mortality. For the purpose of reporting and visualizing the model state, these cohorts are binned into a set of 13 fixed size classes in terms of the diameter at the breast height (DBH) (i.e., 0–5, 5–10, 10–15, 15–20, 20–30, 30–40, 40–50, 50–60, 60–70, 70–80, 80–90, 90–100, and ≥100 cm). Cohorts are further organized into canopy and understory layers, which are subject to different light conditions (Fig. 2a). When logging activities occur, the canopy trees and a portion of big understory trees lose their crown coverage through direct felling for harvesting logs, or as a result of collateral and mechanical damages (Fig. 2b). The fractions of the canopy trees affected by the three mortality mechanisms are then summed up to specify the areal percentages of an old (undisturbed) and a new (disturbed) patch caused by logging in the patch fission process as discussed in Sect. 2.1 (Fig. 2c). After patch fission, the canopy layer over the disturbed patch is removed, while that over the undisturbed patch stays untouched (Fig. 2d). In the undisturbed patch, the survivorship of understory trees is calculated using an understory death fraction consistent with the default value corresponding to that used for natural disturbance (i.e., 0.5598). To differentiate logging from natural disturbance, a slightly elevated, logging-specific understory death fraction is applied in the disturbed patch instead at the time of the logging event. Based on data from field surveys over logged forest plots in the southern Amazon (Feldpausch et al., 2005), the understory death fraction corresponding to logging is now set to be 0.65 as the default but can be modified via the FATES parameter file (Fig. 2e). Therefore, the logging operations will change the forest from the undisturbed state shown in Fig. 2a to a disturbed state in Fig. 2f in the logging module. It is worth mentioning that the newly generated patches are tracked according to the age since disturbance and will be merged with other patches of similar canopy structure following the patch fusion processes in FATES in later time steps of a simulation, pending the inclusion of separate land use fractions for managed and unmanaged forest.
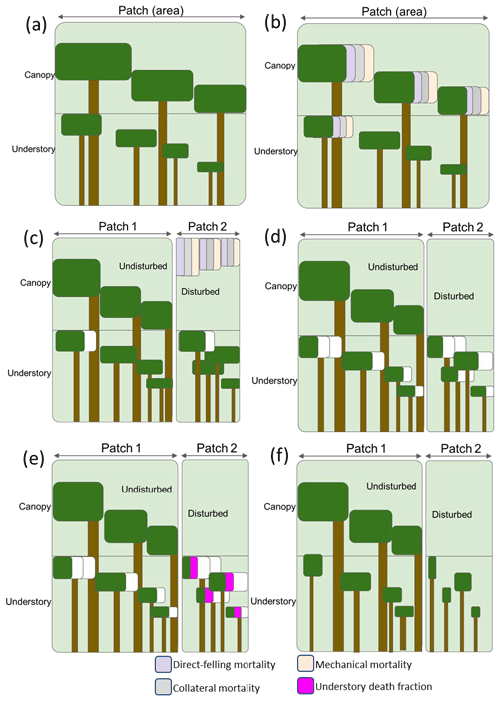
Figure 2The mortality types (direct felling, mechanical, and collateral) and patch-generating process in the FATES logging module. The white fraction in panels (c), (d), and (f) indicates mortality associated with other disturbances in FATES. (a) Canopy and understory layers in each cohort in FATES; (b) mortality applied at the time of a logging event; (c) the patch fission process following a given logging event; (d) canopy removal in the disturbed patch following the logging event; (e) calculation of the understory survivorship based on the understory death fraction in each patch; (d) the final states of the intact and disturbed patches.
Logging operations affect forest structure and composition as well as also carbon cycling (Palace et al., 2008) by modifying the live biomass pools and flow of necromass (Fig. 3). Following a logging event, the logged trunk products from the harvested trees are transported off-site (as an added carbon pool for resource management in the model), while their branches enter the coarse woody debris (CWD) pool, and their leaves and fine roots enter the litter pool. Similarly, trunks and branches of the dead trees caused by collateral and mechanical damages also become CWD, while their leaves and fine roots become litter. Specifically, the densities of dead trees as a result of direct felling, collateral, and mechanical damages in a cohort are calculated as follows:
where A stands for the area of the patch being logged, and n is the number of individuals in the cohort where the mortality types apply (i.e., as specified by the size thresholds, DBHmin and DBH). For each cohort, we denote and .
Leaf litter (Litterleaf, kg C) and root litter (Litterroot, kg C) at the cohort level are then calculated as
where Bleaf and Broot are live biomass in leaves and fine roots, respectively, and Bstore is stored biomass in the labile carbon reserve in all individual trees in the cohort of interest.
Following the existing CWD structure in FATES (Fisher et al., 2015), CWD in the logging module is first separated into two categories: aboveground CWD and belowground CWD. Within each category, four size classes are tracked based on their source, following Thonicke et al. (2010): trunks, large branches, small branches, and twigs. Aboveground CWD from trunks (CWDtrunk_agb, kg C) and branches and twigs (CWDbranch_agb, kg C) are calculated as follows:
where Bstem_agb is the amount of aboveground stem biomass in the cohort, and ftrunk and fbranch represent the fraction of trunks and branches (e.g., large branches, small branches, and twigs). Similarly, the belowground CWD from trunks (CWDtrunk_bg, kg C) and branches and twigs (CWDbranch_bg, kg C) are calculated as follows:
where Bcroot (kg C) is the amount of coarse root biomass in the cohort. Site-level total litter and CWD inputs can then be obtained by integrating the corresponding pools over all the cohorts in the site. To ensure mass conservation, the total loss of live biomass due to logging, ΔB (i.e., carbon in leaf, fine roots, storage, and structural pools), needs to be balanced with increases in litter and CWD pools and the carbon stored in harvested logs shipped off-site as follows:
where Δlitter and ΔCWD are the increments in litter and CWD pools, and trunk_product represents harvested logs shipped off-site.
Following the logging event, the forest structure and composition in terms of cohort distributions, as well as the live biomass and necromass pools, are updated. Following this logging event update to forest structure, the native processes simulating physiology, growth, and competition for resources in and between cohorts resume. Since the canopy layer is removed in the disturbed patch, the existing understory trees are promoted to the canopy layer, but, in general, the canopy is incompletely filled in by these newly promoted trees, and thus the canopy does not fully close. Therefore, more light can penetrate and reach the understory layer in the disturbed patch, leading to increases in light-demanding species in the early stage of regeneration, followed by a succession process in which shade-tolerant species dominate gradually.
Table 2Distributions of stem density (N ha−1), basal area (m2 ha−1), and aboveground biomass (Kg C m−2) before and after logging at km83, separated by diameter of breast height (normal text) and aggregated across all sizes (bold text).
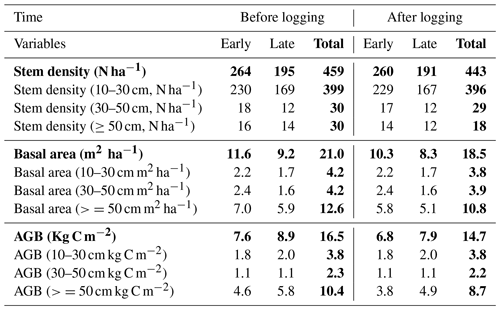
Data in this table obtained based on inventory during the LBA period (Menton et al., 2011; de Sousa et al., 2011).
2.3 Study site and data
In this study, we used data from two evergreen tropical forest sites located in the Tapajós National Forest, Brazil (Fig. 1b). These sites were established during the Large-Scale Biosphere-Atmosphere Experiment in Amazonia (LBA) and are selected because of data availability including those from forest plot surveys and two flux towers established during the LBA period (Keller et al., 2004a). These sites were named after distances along the BR-163 highway from Santarém: km67 (2∘51′ S, 54∘58′ W) and km83 (3∘3′ S, 54∘56′ W). They are situated on a flat plateau and were established as a control-treatment pair for a selective logging experiment. Tree felling operations were initiated at km83 in September 2001 for a period of about 2 months. Both sites are similar with a mean annual precipitation of ∼2000 mm and mean annual temperature of 25 ∘C, on nutrient-poor clay Oxisols with low organic content (Silver et al., 2000).
Prior to logging, both sites were old-growth forests with limited previous human disturbances caused by hunting, gathering Brazil nuts, and similar activities. A comprehensive set of meteorological variables, as well as land–atmosphere exchanges of water, energy, and carbon fluxes, have been measured by an eddy covariance tower at an hourly time step over the period of 2002 to 2011, including precipitation, air temperature, surface pressure, relative humidity, incoming shortwave and longwave radiation, latent and sensible heat fluxes, and net ecosystem exchange (NEE) (Hayek et al., 2018). Another flux tower was established at km83, the logged site, with hourly meteorological and eddy covariance measurements in the period of 2000–2003 (Miller et al., 2004; Goulden et al., 2004; Saleska et al., 2003). The towers are listed as BR-Sa1 and BR-Sa3 in the AmeriFlux network (https://ameriflux.lbl.gov, last access: 28 June 2020).
These tower- and biometric-based observations were summarized to quantify logging-induced perturbations on old-growth Amazonian forests in Miller et al. (2011) and are used in this study to benchmark the model-simulated carbon budget. Over the period of 1999 to 2001, all trees ≥35 cm in DBH in 20 ha of forest in four 1 km long transects within the km67 footprint were inventoried, as well as trees ≥10 cm in DBH on subplots with an area of ∼4 ha. At km83, inventory surveys on trees ≥55 cm in DBH were conducted in 1984 and 2000, and another survey on trees >10 cm in DBH was conducted in 2000 (Miller et al., 2004). Estimates of aboveground biomass (AGB) were then derived using allometric equations for Amazon forests (Rice et al., 2004; Chambers et al., 2004; Keller et al., 2001). Necromass (≥2 cm diameter) production was also measured approximately every 6 months in a 4.5-year period from November 2001 through February 2006 in a logged and undisturbed forest at km83 (Palace et al., 2008). Field measurements of ground disturbance in terms of number of felled trees, as well as areas disturbed by collateral and mechanical damages were also conducted at a similar site in Pará state along multitemporal sequences of postharvest regrowth of 0.5–3.5 years (Asner et al., 2004; Pereira et al., 2002).
Table 3Cohort-level fractional damage fractions in different logging scenarios.
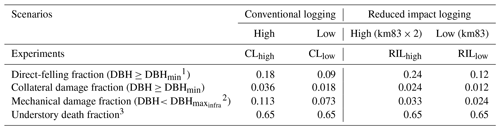
1 DBHmin=50 cm. 2 DBH cm. 3 Applied to the new patch generated by direct felling and collateral damage.
Table 2 provides a summary of stem density and basal area distribution across size classes at km83 based on the biomass survey data (Menton et al., 2011; de Sousa et al., 2011). To facilitate comparisons with simulations from FATES, we divided the inventory into early and late succession PFTs using a threshold of 0.7 g cm−3 for specific wood density, consistent with the definition of these PFTs in Table 1. As shown in Table 2, prior to the logging event in year 2000, this forest was composed of 399, 30, and 30 trees per hectare in size classes of 10–30, 30–50, and ≥50 cm respectively; following logging, the numbers were reduced to 396, 29, and 18 trees per hectare, losing ∼1.3 % of trees ≥10 cm in size. The changes in stem density (SD) were caused by different mechanisms for different size classes. The reduction in stem density of 2 ha−1 in the ≥50 cm size class was caused by timber harvest directly, while the reductions of 3 and 1 ha−1 in the 10–30 and 30–50 cm size classes were caused by collateral and mechanical damages. Corresponding to the loss of trees in logging operations, the basal area (BA) decreased from 3.9, 4.0, and 12.9 m2 ha−1 to 3.8, 3.9, and 10.8 m2 ha−1, and aboveground biomass (AGB) decreased from 3.8, 2.3, and 10.4 kg C m−2 to 3.8, 2.2, and 8.7 kg C m−2 in the 10–30, 30–50, and ≥50 cm size class, respectively.
2.4 Numerical experiments
In this study, the gap-filled meteorological forcing data for the Tapajós National Forest processed by Longo et al. (2019) are used to drive the CLM(FATES) model. Characteristics of the sites, including soil texture, vegetation cover fraction, and canopy height, were obtained from the LBA-Data Model Intercomparison Project (de Gonçalves et al., 2013). Specifically, soil at km67 contains 90 % clay and 2 % sand, while soil at km83 contains 80 % clay and 18 % sand. Both sites are covered by tropical evergreen forest at ∼98 % within their footprints, with the remaining 2 % assumed to be covered by bare soil. As discussed in Longo et al. (2018), who deployed the Ecosystem Demography Model version 2 at this site, soil texture and hence soil hydraulic parameters are highly variable even with the footprint of the same eddy covariance tower, and they could have significant impacts on not only water and energy simulations, but also simulated forest composition and carbon stocks and fluxes. Further, generic pedotransfer functions designed to capture temperate soils typically perform poorly in clay-rich Amazonian soils (Fisher et al., 2008; Tomasella and Hodnett, 1998). Because we focus on introducing the FATES logging, we leave for forthcoming studies the exploration of the sensitivity of the simulations to soil texture and other critical environmental factors.
Table 4Comparison of energy fluxes (mean ± standard deviation) between eddy covariance tower measurements and FATES simulations.
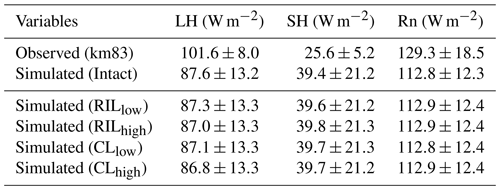
CLM(FATES) was initialized using soil texture at km83 (i.e., 80 % clay and 18 % sand) from bare ground and spun up for 800 years until the carbon pools and forest structure (i.e., size distribution) and composition of PFTs reached equilibrium, by recycling the meteorological forcing at km67 (2001–2011) as the sites are close enough. The final states from spin-up were saved as the initial condition for follow-up simulations. An intact experiment was conducted by running the model over a period of 2001 to 2100 without logging by recycling the 2001–2011 forcing using the parameter set in Table 1. The atmospheric CO2 concentration was assumed to be a constant of 367 ppm over the entire simulation period, consistent with the CO2 levels during the logging treatment (Dlugokencky et al., 2018).
We specified an experimental logging event in FATES on 1 September 2001 (Table 3). It was reported by Figueira et al. (2008) that, following the reduced-impact-logging event in September 2001, 9 % of the trees greater than or equal to DBHmin=50 cm were harvested, with an associated collateral damage fraction of 0.009 for trees ≥ DBHmin. DBH is set to be 30 cm, so that only a fraction of trees ≤30 cm are removed for building infrastructure (Feldpausch et al., 2005). This experiment is denoted as the RILlow experiment in Table 2 and is the one that matches the actual logging practice at km83.
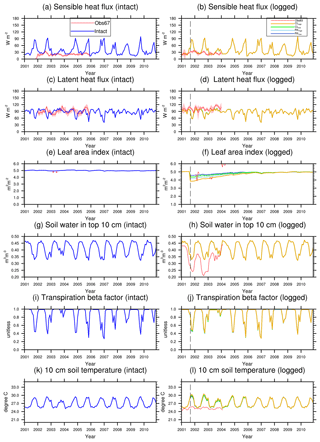
Figure 4Simulated energy budget terms and leaf area indices in intact and logged forests compared to observations from km67 (left) and km83 (right) (Miller et al., 2011). The dashed vertical line indicates the timing of the logging event. The shaded areas in panels (a)–(f) are uncertainty estimates based on u∗-filter cutoff analyses in Miller et al. (2011).
We recognize that the harvest intensity in September 2001 at km83 was extremely low. Therefore, in order to study the impacts of different logging practices and harvest intensities, three additional logging experiments were conducted as listed in Table 3: conventional logging with high intensity (CLhigh), conventional logging with low intensity (CLlow), and reduced impact logging with high intensity (RILhigh). The high-intensity logging doubled the direct-felling fraction in RILlow and CLlow, as shown in the RILhigh and CLhigh experiments. Compared to the RIL experiments, the CL experiments feature elevated collateral and mechanical damages as one would observe in such operations. All logging experiments were initialized from the spun-up state using site characteristics at km83 previously discussed and were conducted over the period of 2001–2100 by recycling meteorological forcing from 2001 to 2011.
3.1 Simulated energy and water fluxes
Simulated monthly mean energy and water fluxes at the two sites are shown and compared to available observations in Fig. 4. The performances of the simulations closest to site conditions were compared to observations and summarized in Table 4 (i.e., intact for km67 and RILlow for km83). The observed fluxes as well as their uncertainty ranges noted as Obs67 and Obs83 from the towers were obtained from Saleska et al. (2013), consistent with those in Miller et al. (2011). As shown in Table 4, the simulated mean (± standard deviation) latent heat (LH), sensible heat (SH), and net radiation (Rn) fluxes at km83 in RILlow over the period of 2001–2003 are 90.2±10.1, 39.6±21.2, and 112.9±12.4 W m−2, compared to tower-based observations of 101.6±8.0, 25.6±5.2, and 129.3±18.5 W m−2. Therefore, the simulated and observed Bowen ratios are 0.35 and 0.20 at km83, respectively. This result suggests that, at an annual time step, the observed partitioning between LH and SH is reasonable, while the net radiation simulated by the model can be improved. At seasonal scales, even though net radiation is captured by CLM(FATES), the model does not adequately partition sensible and latent heat fluxes. This is particularly true for sensible heat fluxes as the model simulates large seasonal variabilities in SH when compared to observations at the site (i.e., standard deviations of monthly mean simulated SH are ∼21.2 W m−2, while observations are ∼5.2 W m−2). As illustrated in Fig. 4c and d, the model significantly overestimates SH in the dry season (June–December), while it slightly underestimates SH in the wet season. It is worth mentioning that incomplete closure of the energy budget is common at eddy covariance towers (Wilson et al., 2002; Foken, 2008) and has been reported to be ∼87 % at the two sites (Saleska et al., 2003).
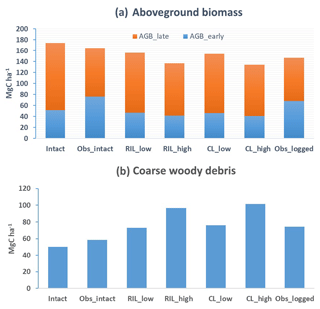
Figure 5Simulated (a) aboveground biomass and (b) coarse woody debris in intact and logged forests in a 1-year period before or after the logging event in the four logging scenarios listed in Table 3. The observations (Obsintact and Obslogged) were derived from inventory (Menton et al., 2011; de Sousa et al., 2011).
Figure 4j shows the comparison between simulated and observed (Goulden et al., 2010) volumetric soil moisture content (m3 m−3) at the top 10 cm. This comparison reveals another model structural deficiency; that is, even though the model simulates higher soil moisture contents compared to observations (a feature generally attributable to the soil moisture retention curve), the transpiration beta factor, the down-regulating factor of transpiration from plants, fluctuates significantly over a wide range and can be as low as 0.3 in the dry season. In reality flux towers in the Amazon generally do not show severe moisture limitations in the dry season (Fisher et al., 2007). The lack of limitation is typically attributed to the plant's ability to extract soil moisture from deep soil layers, a phenomenon that is difficult to simulate using a classical beta function (Baker et al., 2008), and potentially is reconcilable using hydrodynamic representation of plant water uptake (Powell et al., 2013; Christoffersen et al., 2016) as in the final stages of incorporation into the FATES model. Consequently, the model simulates consistently low ET during dry seasons (Fig. 4e and f), while observations indicate that canopies are highly productive owing to adequate water supply to support transpiration and photosynthesis, which could further stimulate coordinated leaf growth with senescence during the dry season (Wu et al., 2016, 2017).
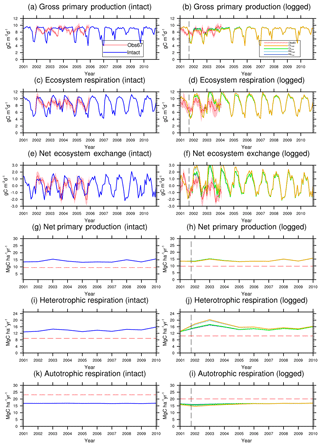
Figure 6Simulated carbon fluxes in intact and logged forests compared to observed fluxes from km67 (left) and km83 (right). The dashed black vertical line indicates the timing of the logging event, while the dashed red horizontal line indicates estimated fluxes derived based on eddy covariance measurements and inventory (Miller et al., 2011). The shaded areas in panels (a)–(f) are uncertainty estimates based on u∗-filter cutoff analyses in Miller et al. (2011). Panels (g)–(i) show comparisons between annual fluxes as only annual estimates of these fluxes are available from Miller et al. (2011).
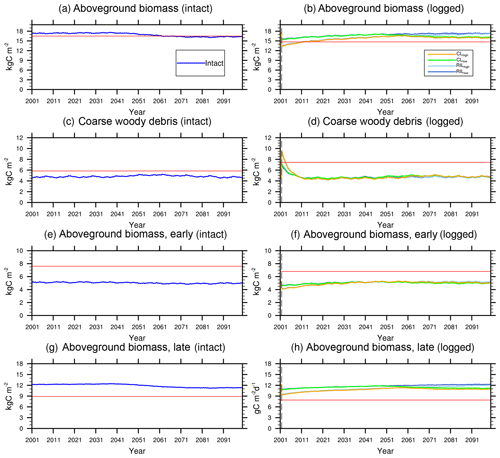
Figure 7Trajectories of carbon pools in intact (left) and logged (right) forests. The dashed black vertical line indicates the timing of the logging event. The dashed red horizontal line indicates observed prelogging (left) and postlogging (right) inventories respectively (Menton et al., 2011; de Sousa et al., 2011).
3.2 Carbon budget as well as forest structure and composition in the intact forest
Figures 5–7 show simulated carbon pools and fluxes, which are tabulated in Table 5 as well. As shown in Fig. 5, prior to logging, the simulated aboveground biomass and necromass (CWD + litter) are 174 and 50 Mg C ha−1, compared to 165 and 58.4 Mg C ha−1 based on permanent plot measurements. The simulated carbon pools are generally lower than observations reported in Miller et al. (2011) but are within reasonable ranges, as errors associated with these estimates could be as high as 50 % due to issues related to sampling and allometric equations, as discussed in Keller et al. (2001). The lower biomass estimates are consistent with the finding of excessive soil moisture stress during the dry season and low leaf area index (LAI) in the model.
Table 5Comparison of carbon budget terms between observation-based estimates* and simulations at km83.
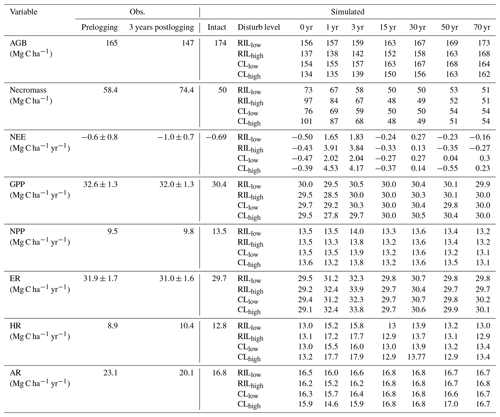
* Source of observation-based estimates: Miller et al. (2011). Uncertainty in carbon fluxes (GPP, ER, NEE) are based on u∗-filter cutoff analyses described in the same paper.
Combining forest inventory and eddy covariance measurements, Miller et al. (2011) also provides estimates for net ecosystem exchange (NEE), gross primary production (GPP), net primary production (NPP), ecosystem respiration (ER), heterotrophic respiration (HR), and autotrophic respiration (AR). As shown in Table 5, the model simulates reasonable values in GPP (30.4 Mg C ha−2 yr−1) and ER (29.7 Mg C ha−2 yr−1), when compared to values estimated from the observations (32.6 Mg C ha−2 yr−1 for GPP and 31.9 Mg C ha−2 yr−1 for ER) in the intact forest. However, the model appears to overestimate NPP (13.5 Mg C ha−2 yr−1 as compared to the observation-based estimate of 9.5 Mg C ha−2 yr−1) and HR (12.8 Mg C ha−2 yr−1 as compared to the estimated value of 8.9 Mg C ha−2 yr−1), while it underestimates AR (16.8 Mg C ha−2 yr−1 as compared to the observation-based estimate of 23.1 Mg C ha−2 yr−1). Nevertheless, it is worth mentioning that we selected the specific parameter set to illustrate the capability of the model in capturing species composition and size structure, while the performance in capturing carbon balance is slightly compromised given the limited number of sensitivity tests performed.
Consistent with the carbon budget terms, Table 5 lists the simulated and observed values of stem density (ha−1) in different size classes in terms of DBH. The model simulates 471 trees per hectare with DBHs greater than or equal to 10 cm in the intact forest, compared to 459 trees per hectare from the observed inventory. In terms of distribution across the DBH classes of 10–30, 30–50, and ≥50 cm, 339, 73, and 59 N ha−1 of trees were simulated, while 399, 30, and 30 N ha−1 were observed in the intact forest. In general, this version of FATES is able to reproduce the size structure and tree density in the tropics reasonably well. In addition to size distribution, by parametrizing early and late successional PFTs (Table 1), FATES is capable of simulating the coexistence of the two PFTs and therefore the PFT-specific trajectories of stem density, basal area, canopy, and understory mortality rates. We will discuss these in Sect. 3.4.
3.3 Effects of logging on water, energy, and carbon budgets
The response of energy and water budgets to different levels of logging disturbances is illustrated in Table 4 and Fig. 4. Following the logging event, the LAI is reduced proportionally to the logging intensities (−9 %, −17 %, −14 %, and −24 % for RLlow, RLhigh, CLlow, and CLhigh respectively in September 2001; see Fig. 4h). The leaf area index recovers within 3 years to its prelogging level or even to slightly higher levels as a result of the improved light environment following logging leading to changes in forest structure and composition (to be discussed in Sect. 3.4). In response to the changes in stem density and LAI, discernible differences are found in all energy budget terms. For example, less leaf area leads to reductions in LH (−0.4 %, −0.7 %, −0.6 %, −1.0 %) and increases in SH (0.6 %, 1.0 %, 0.8 %, and 2.0 %) proportional to the damage levels (i.e., RLlow, RLhigh, CLlow, and CLhigh) in the first 3 years following the logging event when compared to the control simulation. Energy budget responses scale with the level of damage, so that the biggest differences are detected in the CLhigh scenario, followed by RILhigh, CLlow, and RILlow. The difference in simulated water and energy fluxes between the RILlow (i.e., the scenario that is the closest to the experimental logging event) and intact cases is the smallest, as the level of damage is the lowest among all scenarios.
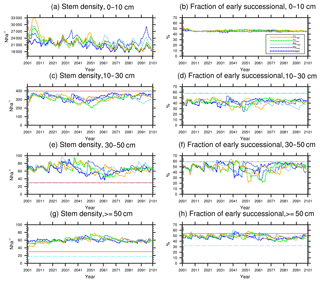
Figure 8Changes in total stem densities and the fractions of the early successional PFT in different size classes following a single logging event on 1 September 2001 at km83. The dashed black vertical line indicates the timing of the logging event, while the solid red line and the dashed cyan horizontal line indicate observed prelogging and postlogging inventories respectively (Menton et al., 2011; de Sousa et al., 2011).
As with LAI, the water and energy fluxes recover rapidly in 3–4 years following logging. Miller et al. (2011) compared observed sensible and latent heat fluxes between the control (km67) and logged sites (km83). They found that, in the first 3 years following logging, the between-site difference (i.e., logged–control) in LH reduced from 19.7±2.4 to 15.7±1.0 W m2 and that in SH increased from 3.6±1.1 to 5.4±0.4 W m2. When normalized by observed fluxes during the same periods at km83, these changes correspond to a −4 % reduction in LH and a 7 % increase in SH, compared to the −0.5 % and 4 % differences in LH and SH between RLlow and the control simulations. In general, both observations and our modeling results suggest that the impacts of reduced impact logging on energy fluxes are modest and that the energy and water fluxes can quickly recover to their prelogging conditions at the site.
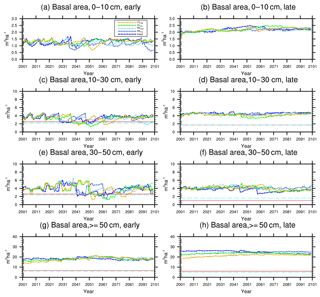
Figure 9Changes in basal area of the two PFTs in different size classes following a single logging event on 1 September 2001 at km83. The dashed black vertical line indicates the timing of the logging event, while the solid red line and the dashed cyan horizontal line indicate observed prelogging and postlogging inventories respectively (Menton et al., 2011; de Sousa et al., 2011). Note that for the size class 0–10 cm, observations are not available from the inventory.
Figures 6 and 7 show the impact of logging on carbon fluxes and pools at a monthly time step, and the corresponding annual fluxes and changes in carbon pools are summarized in Table 5. The logging disturbance leads to reductions in GPP, NPP, AR, and AGB, as well as increases in ER, NEE, HR, and CWD. The impacts of logging on the carbon budgets are also proportional to logging damage levels. Specifically, logging reduces the simulated AGB from 174 Mg C ha−1 (intact) to 156 Mg C ha−1 (RILlow), 137 Mg C ha−1 (RILhigh), 154 Mg C ha−1 (CLlow), and 134 Mg C ha−1 (CLhigh), while it increases the simulated necromass pool (CWD + litter) from 50.0 Mg C ha−1 in the intact case to 73 Mg C ha−1 (RILlow), 97 Mg C ha−1 (RILhigh), 76 Mg C ha−1 (CLlow), and 101 Mg C ha−1 (CLhigh). For the case closest to the experimental logging event (RILlow), the changes in AGB and necromass from the intact case are −18 Mg C ha−1 (10 %) and 23.0 Mg C ha−1 (46 %), in comparison to observed changes of −22 Mg C ha−1 in AGB (12 %) and 16 Mg C ha−1 (27 %) in necromass from Miller et al. (2011), respectively. The magnitudes and directions of these changes are reasonable when compared to observations (i.e., decreases in GPP, ER, and AR following logging). On the other hand, the simulations indicate that the forest could be turned from a carbon sink (−0.69 Mg C ha−1 yr−1) to a larger carbon source in 1–5 years following logging, consistent with observations from the tower suggesting that the forest was a carbon sink or a modest carbon source ( Mg C ha−1 yr−1) prior to logging.
The recovery trajectories following logging are also shown in Figs. 6, 7, and Table 5. It takes more than 70 years for AGB to return to its prelogging levels, but the recovery of carbon fluxes such as GPP, NPP, and AR is much faster (i.e., within 5 years following logging). The initial recovery rates of AGB following logging are faster for high-intensity logging because of increased light reaching the forest floor, as indicated by the steeper slopes corresponding to the CLhigh and RILhigh scenarios compared to those of CLlow and RILlow (Fig. 9h). This finding is consistent with previous observational and modeling studies (Mazzei et al., 2010; Huang and Asner, 2010) in that the damage level determines the number of years required to recover the original AGB, and the AGB accumulation rates in recently logged forests are higher than those in intact forests. For example, by synthesizing data from 79 permanent plots at 10 sites across the Amazon basin, Ruttishauser et al. (2016) and Piponiot et al. (2018) show that it requires 12, 43, and 75 years for the forest to recover with initial losses of 10 %, 25 %, or 50 % in AGB. Corresponding to the changes in AGB, logging introduces a large amount of necromass to the forest floor, with the highest increases in the CLhigh and RILhigh scenarios. As shown in Fig. 7d and Table 5, necromass and CWD pools return to the prelogging level in ∼15 years. Meanwhile, HR in RILlow stays elevated in the 5 years following logging but converges to that from the intact simulation in ∼10 years, which is consistent with observations (Miller et al., 2011; Table 5).
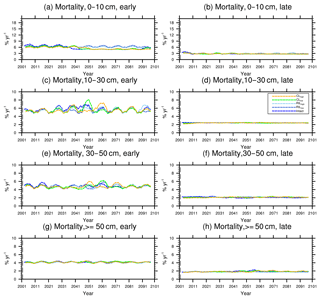
Figure 10Changes in mortality (5-year running average) of the (a) early and (b) late successional trees in different size classes following a single logging event on 1 September 2001. The dashed black vertical line indicates the timing of the logging event.
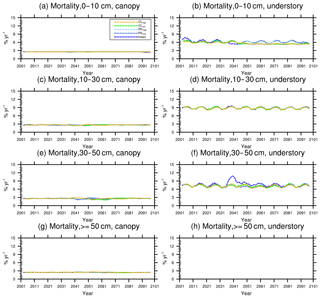
Figure 11Changes in mortality (5-year running average) of the (a) canopy and (b) understory trees in different size classes following a single logging event on 1 September 2001. The dashed black vertical line indicates the timing of the logging event.
3.4 Effects of logging on forest structure and composition
The capability of the CLM(FATES) model to simulate vegetation demographics, forest structure, and composition while simulating the water, energy, and carbon budgets simultaneously (Fisher et al., 2017) allows for the interrogation of the modeled impacts of alternative logging practices on the forest size structure. Table 6 shows the forest structure in terms of stem density distribution across size classes from the simulations compared to observations from the site, while Figs. 8 and 9 further break it down into early and late succession PFTs and size classes in terms of stem density and basal areas. As discussed in Sect. 2.2 and summarized in Table 3, the logging practices, reduced impact logging and conventional logging, differ in terms of preharvest planning and actual field operation to minimize collateral and mechanical damages, while the logging intensities (i.e., high and low) indicate the target direct-felling fractions. The corresponding outcomes of changes in forest structure in comparison to the intact forest, as simulated by FATES, are summarized in Tables 6 and 7. The conventional-logging scenarios (i.e., CLhigh and CLlow) feature more losses in small trees less than 30 cm in DBH, when compared to the smaller reduction in stem density in size classes less than 30 cm in DBH in the reduced-impact-logging scenarios (i.e., RILhigh and RILlow). Scenarios with different logging intensities (i.e., high and low) result in different direct-felling intensity. That is, the numbers of surviving large trees (DBH ≥ 30 cm) in RILlow and CLlow are 117 ha−1 and 115 ha−1, but those in RILhigh and CLhigh are 106 and 103 ha−1.
In response to the improved light environment after the removal of large trees, early successional trees quickly establish and populate the tree-fall gaps following logging in 2–3 years as shown in Fig. 8a. Stem density in the <10 cm size classes is proportional to the damage levels (i.e., ranked as CLhigh>RILhigh>CLlow>RILlow), followed by a transition to late successional trees in later years when the canopy is closed again (Fig. 8b). Such a successional process is also evident in Fig. 9a and b in terms of basal areas. The number of early successional trees in the <10 cm size classes then slowly declines afterwards but is sustained throughout the simulation as a result of natural disturbances. Such a shift in the plant community towards light-demanding species following disturbances is consistent with observations reported in the literature (Baraloto et al., 2012; Both et al., 2018). Following regeneration in logging gaps, a fraction of trees win the competition within the 0–10 cm size classes and are promoted to the 10–30 cm size classes in about 10 years following the disturbances (Figs. 8d and 9d). Then a fraction of those trees subsequently enter the 30–50 cm size classes in 20–40 years following the disturbance (Figs. 8f and 9f) and so on through larger size classes afterwards (Figs. 8h and 9h). We note that, despite the goal of achieving a deterministic and smooth averaging across discrete stochastic disturbance events using the ecosystem demography approach (Moorcroft et al., 2001) in FATES, the successional process described above, as well as the total numbers of stems in each size bin, shows evidence of episodic and discrete waves of population change. These arise due to the required discretization of the continuous time-since-disturbance heterogeneity into patches, combined with the current maximum cap on the number of patches in FATES (10 per site).
As discussed in Sect. 2.4, the early successional trees have a high mortality (Fig. 10a, c, e, and g) compared to the mortality (Fig. 10b, d, f, and h) of late successional trees as expected given their higher background mortality rate. Their mortality also fluctuates at an equilibrium level because of the periodic gap dynamics due to natural disturbances, while the mortality of late successional trees remains stable. The mortality rates of canopy trees (Fig. 11a, c, e, and g) remain low and stable over the years for all size classes, indicating that canopy trees are not light-limited or water-stressed. In comparison, the mortality rate of small understory trees (Fig. 11b) shows a declining trend following logging, consistent with the decline in mortality of the small early successional tree (Fig. 10a). As the understory trees are promoted to larger size classes (Fig. 11d, f), their mortality rates stay high. It is evident that it is hard for the understory trees to be promoted to the largest size class (Fig. 11h); therefore the mortality cannot be calculated due to the lack of population.
In this study, we developed a selective logging module in FATES and parameterized the model to simulate different logging practices (conventional and reduced impact) with various intensities. This newly developed selective logging module is capable of mimicking the ecological, biophysical, and biogeochemical processes at a landscape level following a logging event in a lumped way by (1) specifying the timing and areal extent of a logging event; (2) calculating the fractions of trees that are damaged by direct felling, collateral damage, and infrastructure damage and adding these size-specific plant mortality types to FATES; (3) splitting the logged patch into disturbed and intact new patches; (4) applying the calculated survivorship to cohorts in the disturbed patch; and (5) transporting harvested logs off-site and adding the remaining necromass from damaged trees into coarse woody debris and litter pools.
We then applied FATES coupled to CLM to the Tapajós National Forest by conducting numerical experiments driven by observed meteorological forcing, and we benchmarked the simulations against long-term ecological and eddy covariance measurements. We demonstrated that the model is capable of simulating site-level water, energy, and carbon budgets, as well as forest structure and composition holistically, with responses consistent with those documented in the existing literature as follows:
-
The model captures perturbations on energy and water budget terms in response to different levels of logging disturbances. Our modeling results suggest that logging leads to reductions in canopy interception, canopy evaporation and transpiration, and elevated soil temperature and soil heat fluxes in magnitudes proportional to the damage levels.
-
The logging disturbance leads to reductions in GPP, NPP, AR, and AGB, as well as increases in ER, NEE, HR, and CWD. The initial impacts of logging on the carbon budget are also proportional to damage levels as results of different logging practices.
-
Following the logging event, simulated carbon fluxes such as GPP, NPP, and AR recover within 5 years, but it takes decades for AGB to return to its prelogging levels. Consistent with existing observation-based literature, initial recovery of AGB is faster when the logging intensity is higher in response to the improved light environment in the forest, but the time to full AGB recovery in higher intensity logging is longer.
-
Consistent with observations at Tapajós, the prescribed logging event introduces a large amount of necromass to the forest floor proportional to the damage level of the logging event, which returns to prelogging level in ∼15 years. Simulated HR in the low-damage reduced-impact-logging scenario stays elevated in the 5 years following logging and declines to be the same as the intact forest in ∼10 years.
-
The impacts of alternative logging practices on forest structure and composition were assessed by parameterizing cohort-specific mortality corresponding to direct felling, collateral damage, mechanical damage in the logging module to represent different logging practices (i.e., conventional logging and reduced impact logging), and intensity (i.e., high and low). In all scenarios, the improved light environment after the removal of large trees facilitates the establishment and growth of early successional trees in the 0–10 cm DBH size class proportional to the damage levels in the first 2–3 years. Thereafter there is a transition to late successional trees in later years when the canopy is closed. The number of early successional trees then slowly declines but is sustained throughout the simulation as a result of natural disturbances.
Given that the representation of gas exchange processes is related to, but also somewhat independent of, the representation of ecosystem demography, FATES shows great potential in its capability to capture ecosystem successional processes in terms of gap-phase regeneration; competition among light-demanding and shade-tolerant species following disturbance; and responses of energy, water, and carbon budget components to disturbances. The model projections suggest that while most degraded forests rapidly recover energy fluxes, the recovery times for carbon stocks, forest size structure, and forest composition are much longer. The recovery trajectories are highly dependent on logging intensity and practices, the difference between which can be directly simulated by the model. Consistent with field studies, we find through numerical experiments that reduced impact logging leads to more rapid recovery of the water, energy, and carbon cycles, allowing forest structure and composition to recover to their prelogging levels in a shorter time frame.
Currently, the selective logging module can only simulate single logging events. We also assumed that for a site such as km83, once logging is activated, trees will be harvested from all patches. For regional-scale applications, it will be crucial to represent forest degradation as a result of logging, fire, and fragmentation and their combinations that could repeat over a period. Therefore, structural changes in FATES have been made by adding prognostic variables to track disturbance histories associated with fire, logging, and transitions among land use types. The model also needs to include the dead tree pool (snags and standing dead wood) as harvest operations (especially thinning) can lead to live tree death from machine damage and windthrow. This will be more important for using FATES in temperate, coniferous systems, and the varied biogeochemical legacy of standing versus downed wood is important (Edburg et al., 2011, 2012). To better understand how nutrient limitation or enhancement (e.g., via deposition or fertilization) can affect the ecosystem dynamics, a nutrient-enabled version of FATES is also under testing and will shed more lights on how biogeochemical cycling could impact vegetation dynamics once available. Nevertheless, this study lays the foundation to simulate land use change and forest degradation in FATES, leading the way to direct representation of forest management practices and regeneration in Earth system models.
We also acknowledge that as a model development study, we applied the model to a site using a single set of parameter values, and therefore we ignored the uncertainty associated with model parameters. Nevertheless, the sensitivity study in the Supplement shows that the model parameters can be calibrated with a good benchmarking dataset with various aspects of ecosystem observations. For example, Koven et al. (2020) demonstrated a joint team effort of modelers and field observers toward building field-based benchmarks from Barro Colorado Island, Panama, and a parameter sensitivity test platform for physiological and ecosystem dynamics using FATES. We expect to see more of such efforts to better constrain the model in future studies.
CLM(FATES) has two separate repositories for CLM and FATES available at https://github.com/ESCOMP/ctsm (last access: 25 June 2020; https://doi.org/10.5281/zenodo.3739617, CTSM Development Team, 2020) and https://github.com/NGEET/fates (last access: 25 June 2020; https://doi.org/10.5281/zenodo.3825474, FATES Development Team, 2020).
Site information and data at km67 and km83 can be found at http://sites.fluxdata.org/BR-Sa1 (last access: 10 Februray 2020; https://doi.org/10.18140/FLX/1440032, Saleska, 2002–2011) and http://sites.fluxdata.org/BR-Sa13 (last access: 10 February 2020; https://doi.org/10.18140/FLX/1440033, Goulden, 2000–2004).
A README guide to run the model and formatted datasets used to drive model in this study will be made available from the open-source repository https://github.com/huangmy/FATES_Logging_Manuscript.git (Huang, 2020).
The supplement related to this article is available online at: https://doi.org/10.5194/bg-17-4999-2020-supplement.
MH, MK, and ML conceived the study, conceptualized the design of the logging module, and designed the numerical experiments and analysis. YX, MH, and RGK coded the module. YX, RGK, CDK, RAF, and MH integrated the module into FATES. MH performed the numerical experiments and wrote the manuscript with inputs from all coauthors.
The authors declare that they have no conflict of interest.
This research was supported by the Next-Generation Ecosystem Experiments–Tropics project through the Terrestrial Ecosystem Science (TES) program within the U.S. Department of Energy's Office of Biological and Environmental Research (BER). The Pacific Northwest National Laboratory is operated by the DOE and by the Battelle Memorial Institute under contract DE-AC05-76RL01830. LBNL is managed and operated by the Regents of the University of California under prime contract no. DE-AC02-05CH11231. The research carried out at the Jet Propulsion Laboratory, California Institute of Technology, was under a contract with the National Aeronautics and Space Administration (80NM0018D004). Marcos Longo was supported by the São Paulo State Research Foundation (FAPESP, grant 2015/07227-6) and by the NASA Postdoctoral Program, administered by the Universities Space Research Association under contract with NASA (80NM0018D004). Rosie A. Fisher acknowledges the National Science Foundation's support of the National Center for Atmospheric Research.
This research has been supported by the U.S. Department of Energy, Office of Science (grant no. 66705), the São Paulo State Research Foundation (grant no. 2015/07227-6), the National Aeronautics and Space Administration (grant no. 80NM0018D004), and the National Science Foundation (grant no. 1458021).
This paper was edited by Christopher Still and reviewed by two anonymous referees.
Asner, G. P., Keller, M., Pereira, J. R., Zweede, J. C., and Silva, J. N. M.: Canopy damage and recovery after selective logging in amazonia: field and satellite studies, Ecol. Appl., 14, 280–298, https://doi.org/10.1890/01-6019, 2004.
Asner, G. P., Knapp, D. E., Broadbent, E. N., Oliveira, P. J. C., Keller, M., and Silva, J. N.: Selective Logging in the Brazilian Amazon, Science, 310, 480–482, https://doi.org/10.1126/science.1118051, 2005.
Asner, G. P., Keller, M., Pereira, R., and Zweed, J. C.: LBA-ECO LC-13 GIS Coverages of Logged Areas, Tapajos Forest, Para, Brazil: 1996, 1998. ORNL DAAC, Oak Ridge, Tennessee, USA, https://doi.org/10.3334/ORNLDAAC/893, 2008.
Asner, G. P., Rudel, T. K., Aide, T. M., Defries, R., and Emerson, R.: A Contemporary Assessment of Change in Humid Tropical Forests Una Evaluación Contemporánea del Cambio en Bosques Tropicales Húmedos, Conserv. Biol., 23, 1386–1395, https://doi.org/10.1111/j.1523-1739.2009.01333.x, 2009.
Baidya Roy, S., Hurtt, G. C., Weaver, C. P., and Pacala, S. W.: Impact of historical land cover change on the July climate of the United States, J. Geophys. Res.-Atmos., 108, 4793, https://doi.org/10.1029/2003JD003565, 2003.
Baker, I. T., Prihodko, L., Denning, A. S., Goulden, M., Miller, S., and Da Rocha, H. R.: Seasonal drought stress in the Amazon: Reconciling models and observations, J. Geophys. Res.-Biogeo., 113, https://doi.org/10.1029/2007JG000644, 2008.
Baraloto, C., Hérault, B., Paine, C. E. T., Massot, H., Blanc, L., Bonal, D., Molino, J.-F., Nicolini, E. A., and Sabatier, D.: Contrasting taxonomic and functional responses of a tropical tree community to selective logging, J. Appl. Ecol., 49, 861–870, https://doi.org/10.1111/j.1365-2664.2012.02164.x, 2012.
Berenguer, E., Ferreira, J., Gardner, T. A., Aragão, L. E. O. C., De Camargo, P. B., Cerri, C. E., Durigan, M., Oliveira, R. C. D., Vieira, I. C. G., and Barlow, J.: A large-scale field assessment of carbon stocks in human-modified tropical forests, Glob. Change Biol., 20, 3713–3726, https://doi.org/10.1111/gcb.12627, 2014.
Blaser, J., Sarre, A., Poore, D., and Johnson, S.: Status of Tropical Forest Management 2011, International Tropical Timber Organization, Yokohama, Japan, 2011.
Bohn, K., Dyke, J. G., Pavlick, R., Reineking, B., Reu, B., and Kleidon, A.: The relative importance of seed competition, resource competition and perturbations on community structure, Biogeosciences, 8, 1107–1120, https://doi.org/10.5194/bg-8-1107-2011, 2011.
Bonan, G. B.: Forests and Climate Change: Forcings, Feedbacks, and the Climate Benefits of Forests, Science, 320, 1444–1449, https://doi.org/10.1126/science.1155121, 2008.
Both, S., Riutta, T., Paine, C. E. T., Elias, D. M. O., Cruz, R. S., Jain, A., Johnson, D., Kritzler, U. H., Kuntz, M., Majalap-Lee, N., Mielke, N., Montoya Pillco, M. X., Ostle, N. J., Arn Teh, Y., Malhi, Y., and Burslem, D. F. R. P.: Logging and soil nutrients independently explain plant trait expression in tropical forests, New Phytol., 221, 1853–1865, https://doi.org/10.1111/nph.15444, 2018.
Bradshaw, C. J. A., Sodhi, N. S., and Brook, B. W.: Tropical turmoil: a biodiversity tragedy in progress, Front. Ecol. Environ., 7, 79–87, https://doi.org/10.1890/070193, 2009.
Brokaw, N.: Gap-Phase Regeneration in a Tropical Forest, Ecology, 66, 682–687, https://doi.org/10.2307/1940529, 1985.
Bustamante, M. M. C., Roitman, I., Aide, T. M., Alencar, A., Anderson, L., Aragão, L., Asner, G. P., Barlow, J., Berenguer, E., Chambers, J., Costa, M. H., Fanin, T., Ferreira, L. G., Ferreira, J. N., Keller, M., Magnusson, W. E., Morales, L., Morton, D., Ometto, J. P. H. B., Palace, M., Peres, C., Silvério, D., Trumbore, S., and Vieira, I. C. G.: Towards an integrated monitoring framework to assess the effects of tropical forest degradation and recovery on carbon stocks and biodiversity, Glob. Change Biol., 22, 92–109, https://doi.org/10.1111/gcb.13087, 2016.
Chambers, J. Q., Tribuzy, E. S., Toledo, L. C., Crispim, B. F., Higuchi, N., Santos, J. d., Araújo, A. C., Kruijt, B., Nobre, A. D., and Trumbore, S. E.: RESPIRATION FROM A TROPICAL FOREST ECOSYSTEM: PARTITIONING OF SOURCES AND 725 LOW CARBON USE EFFICIENCY, Ecol. Appl., 14, 72–88, https://doi.org/10.1890/01-6012, 2004.
Christoffersen, B. O., Gloor, M., Fauset, S., Fyllas, N. M., Galbraith, D. R., Baker, T. R., Kruijt, B., Rowland, L., Fisher, R. A., Binks, O. J., Sevanto, S., Xu, C., Jansen, S., Choat, B., Mencuccini, M., McDowell, N. G., and Meir, P.: Linking hydraulic traits to tropical forest function in a size-structured and trait-driven model (TFS v.1-Hydro), Geosci. Model Dev., 9, 4227–4255, https://doi.org/10.5194/gmd-9-4227-2016, 2016.
CTSM Development Team: ESCOMP/CTSM: Update documentation for release-clm5.0 branch, and fix issues with no-anthro surface dataset creation (Version release-clm5.0.34), Zenodo, https://doi.org/10.5281/zenodo.3779821, 2020.
de Gonçalves, L. G. G., Borak, J. S., Costa, M. H., Saleska, S. R., Baker, I., Restrepo-Coupe, N., Muza, M. N., Poulter, B., Verbeeck, H., Fisher, J. B., Arain, M. A., Arkin, P., Cestaro, B. P., Christoffersen, B., Galbraith, D., Guan, X., van den Hurk, B. J. J. M., Ichii, K., Imbuzeiro, H. M. A., Jain, A. K., Levine, N., Lu, C., Miguez-Macho, G., Roberti, D. R., Sahoo, A., Sakaguchi, K., Schaefer, K., Shi, M., Shuttleworth, W. J., Tian, H., Yang, Z.-L., and Zeng, X.: Overview of the Large-Scale Biosphere–Atmosphere Experiment in Amazonia Data Model Intercomparison Project (LBA-DMIP), Agr. Forest Meteorol., 182–183, 111–127, https://doi.org/10.1016/j.agrformet.2013.04.030, 2013.
de Sousa, C. A. D., Elliot, J. R., Read, E. L., Figueira, A. M. S., Miller, S. D., and Goulden, M. L.: LBA-ECO CD-04 Logging Damage, km 83 Tower Site, Tapajos National Forest, Brazil. ORNL DAAC, Oak Ridge, Tennessee, USA, https://doi.org/10.3334/ORNLDAAC/1038, 2011.
Dirzo, R., Young, H. S., Galetti, M., Ceballos, G., Isaac, N. J. B., and Collen, B.: Defaunation in the Anthropocene, Science, 345, 401–406, https://doi.org/10.1126/science.1251817, 2014.
Dlugokencky, E. J., Hall, B. D., Montzka, S. A., Dutton, G., Mühle, J., and Elkins, J. W.: Atmospheric composition [in State of the Climate in 2017], B. Am. Meteorol. Soc., 99, S46–S49, 2018.
Domingues, T. F., Berry, J. A., Martinelli, L. A., Ometto, J. P. H. B., and Ehleringer, J. R.: Parameterization of Canopy Structure and Leaf-Level Gas Exchange for an Eastern Amazonian Tropical Rain Forest (Tapajós National Forest, Pará, Brazil), Earth Interact., 9, 1–23, https://doi.org/10.1175/ei149.1, 2005.
Dykstra, D. P.: Reduced impact logging: concepts and issues, Applying Reduced Impact Logging to Advance Sustainable Forest Management, Food and Agriculture Organization of the United Nations Regional Office for Asia and the Pacific, Bangkok, Thailand, 23–39, 2002.
Edburg, S. L., Hicke, J. A., Lawrence, D. M., and Thornton, P. E.: Simulating coupled carbon and nitrogen dynamics following mountain pine beetle outbreaks in the western United States, J. Geophys. Res.-Biogeo., 116, G04033, https://doi.org/10.1029/2011JG001786, 2011.
Edburg, S. L., Hicke, J. A., Brooks, P. D., Pendall, E. G., Ewers, B. E., Norton, U., Gochis, D., Gutmann, E. D., and Meddens, A. J. H.: Cascading impacts of bark beetle-caused tree mortality on coupled biogeophysical and biogeochemical processes, Front. Ecol. Environ., 10, 416–424, 2012.
Erb, K.-H., Kastner, T., Plutzar, C., Bais, A. L. S., Carvalhais, N., Fetzel, T., Gingrich, S., Haberl, H., Lauk, C., Niedertscheider, M., Pongratz, J., Thurner, M., and Luyssaert, S.: Unexpectedly large impact of forest management and grazing on global vegetation biomass, Nature, 553, 73–76, https://doi.org/10.1038/nature25138, 2017.
FATES Development Team: The Functionally Assembled Terrestrial Ecosystem Simulator (FATES) (Version sci.1.35.5_api.11.0.0), Zenodo, https://doi.org/10.5281/zenodo.3825474, 2020.
Feldpausch, T. R., Jirka, S., Passos, C. A. M., Jasper, F., and Riha, S. J.: When big trees fall: Damage and carbon export by reduced impact logging in southern Amazonia, Forest Ecol. Manag., 219, 199–215, https://doi.org/10.1016/j.foreco.2005.09.003, 2005.
Figueira, A. M. E. S., Miller, S. D., de Sousa, C. A. D., Menton, M. C., Maia, A. R., da Rocha, H. R., and Goulden, M. L.: Effects of selective logging on tropical forest tree growth, J. Geophys. Res.-Biogeo., 113, G00B05, https://doi.org/10.1029/2007JG000577, 2008.
Fisher, R., McDowell, N., Purves, D., Moorcroft, P., Sitch, S., Cox, P., Huntingford, C., Meir, P., and Ian Woodward, F.: Assessing uncertainties in a second-generation dynamic vegetation model caused by ecological scale limitations, New Phytol., 187, 666–681, https://doi.org/10.1111/j.1469-8137.2010.03340.x, 2010.
Fisher R. A., Williams, M., Lola da Costa, A., Malhi, Y., da Costa, R. F., Almeida, S., and Meir, P.: The response of an Eastern Amazonian rain forest to drought stress: Results and modeling analyses from a through-fall exclusion experiment, Glob. Change Biol., 13, 2361–2378, 2007.
Fisher R. A., Williams M., Ruivo M. L., Sombroek W. G., and Lola da Costa, A.: Evaluating climatic and edaphic controls of drought stress at two Amazonian rain forest sites, Agr. Forest Meteorol., 148, 850–861, 2008.
Fisher, R. A., Muszala, S., Verteinstein, M., Lawrence, P., Xu, C., McDowell, N. G., Knox, R. G., Koven, C., Holm, J., Rogers, B. M., Spessa, A., Lawrence, D., and Bonan, G.: Taking off the training wheels: the properties of a dynamic vegetation model without climate envelopes, CLM4.5(ED), Geosci. Model Dev., 8, 3593–3619, https://doi.org/10.5194/gmd-8-3593-2015, 2015.
Fisher, R. A., Koven, C. D., Anderegg, W. R. L., Christoffersen, B. O., Dietze, M. C., Farrior, C. E., Holm, J. A., Hurtt, G. C., Knox, R. G., Lawrence, P. J., Lichstein, J. W., Longo, M., Matheny, A. M., Medvigy, D., Muller-Landau, H. C., Powell, T. L., Serbin, S. P., Sato, H., Shuman, J. K., Smith, B., Trugman, A. T., Viskari, T., Verbeeck, H., Weng, E., Xu, C., Xu, X., Zhang, T., and Moorcroft, P. R.: Vegetation demographics in Earth System Models: A review of progress and priorities, Glob. Change Biol., 24, 35–54, https://doi.org/10.1111/gcb.13910, 2017.
Foken, T.: THE ENERGY BALANCE CLOSURE PROBLEM: AN OVERVIEW, Ecol. Appl., 18, 1351–1367, https://doi.org/10.1890/06-0922.1, 2008.
Goulden, M.: FLUXNET2015 BR-Sa3 Santarem-Km83-Logged Forest, Dataset, https://doi.org/10.18140/FLX/1440033, 2000–2004.
Goulden, M. L., Miller, S. D., da Rocha, H. R., Menton, M. C., de Freitas, H. C., e Silva Figueira, A. M., and de Sousa, C. A. D.: DIEL AND SEASONAL PATTERNS OF TROPICAL FOREST CO2 EXCHANGE, Ecol. Appl., 14, 42–54, https://doi.org/10.1890/02-6008, 2004.
Goulden, M. L., Miller, S. D., and da Rocha, H. R.: LBA-ECO CD-04 Soil Moisture Data, km 83 Tower Site, Tapajos National Forest, Brazil. ORNL DAAC, Oak Ridge, Tennessee, USA, https://doi.org/10.3334/ORNLDAAC/979, 2010.
Hayek, M. N., Wehr, R., Longo, M., Hutyra, L. R., Wiedemann, K., Munger, J. W., Bonal, D., Saleska, S. R., Fitzjarrald, D. R., and Wofsy, S. C.: A novel correction for biases in forest eddy covariance carbon balance, Agr. Forest Meteorol., 250–251, 90–101, https://doi.org/10.1016/j.agrformet.2017.12.186, 2018.
Huang, M.: Script Repository for the FATES Logging Manuscript, GitHub, available at: https://github.com/huangmy/FATES_Logging_Manuscript.git (last access: 28 June 2020), 2020.
Huang, M. and Asner, G. P.: Long-term carbon loss and recovery following selective logging in Amazon forests, Global Biogeochem. Cy., 24, GB3028, https://doi.org/10.1029/2009GB003727, 2010.
Huang, M., Asner, G. P., Keller, M., and Berry, J. A.: An ecosystem model for tropical forest disturbance and selective logging, J. Geophys. Res.-Biogeo., 113, G01002, https://doi.org/10.1029/2007JG000438, 2008.
Hurtt, G. C., Moorcroft, P. R., And, S. W. P., and Levin, S. A.: Terrestrial models and global change: challenges for the future, Glob. Change Biol., 4, 581–590, https://doi.org/10.1046/j.1365-2486.1998.t01-1-00203.x, 1998.
Hurtt, G. C., Chini, L. P., Frolking, S., Betts, R. A., Feddema, J., Fischer, G., Fisk, J. P., Hibbard, K., Houghton, R. A., Janetos, A., Jones, C. D., Kindermann, G., Kinoshita, T., Klein Goldewijk, K., Riahi, K., Shevliakova, E., Smith, S., Stehfest, E., Thomson, A., Thornton, P., van Vuuren, D. P., and Wang, Y. P.: Harmonization of land-use scenarios for the period 1500–2100: 600 years of global gridded annual land-use transitions, wood harvest, and resulting secondary lands, Climatic Change, 109, 117–161, https://doi.org/10.1007/s10584-011-0153-2, 2011.
Keller, M., Palace, M., and Hurtt, G.: Biomass estimation in the Tapajos National Forest, Brazil: Examination of sampling and allometric uncertainties, Forest Ecol. Manag., 154, 371–382, https://doi.org/10.1016/S0378-1127(01)00509-6, 2001.
Keller, M., Alencar, A., Asner, G. P., Braswell, B., Bustamante, M., Davidson, E., Feldpausch, T., Fernandes, E., Goulden, M., Kabat, P., Kruijt, B., Luizão, F., Miller, S., Markewitz, D., Nobre, A. D., Nobre, C. A., Priante Filho, N., da Rocha, H., Silva Dias, P., von Randow, C., and Vourlitis, G. L.: ECOLOGICAL RESEARCH IN THE LARGE-SCALE BIOSPHERE–ATMOSPHERE EXPERIMENT IN AMAZONIA: EARLY RESULTS, Ecol. Appl., 14, 3–16, https://doi.org/10.1890/03-6003, 2004a.
Keller, M., Palace, M., Asner, G. P., Pereira, R., and Silva, J. N. M.: Coarse woody debris in undisturbed and logged forests in the eastern Brazilian Amazon, Glob. Change Biol., 10, 784–795, https://doi.org/10.1111/j.1529-8817.2003.00770.x, 2004b.
Koven, C. D., Knox, R. G., Fisher, R. A., Chambers, J. Q., Christoffersen, B. O., Davies, S. J., Detto, M., Dietze, M. C., Faybishenko, B., Holm, J., Huang, M., Kovenock, M., Kueppers, L. M., Lemieux, G., Massoud, E., McDowell, N. G., Muller-Landau, H. C., Needham, J. F., Norby, R. J., Powell, T., Rogers, A., Serbin, S. P., Shuman, J. K., Swann, A. L. S., Varadharajan, C., Walker, A. P., Wright, S. J., and Xu, C.: Benchmarking and parameter sensitivity of physiological and vegetation dynamics using the Functionally Assembled Terrestrial Ecosystem Simulator (FATES) at Barro Colorado Island, Panama, Biogeosciences, 17, 3017–3044, https://doi.org/10.5194/bg-17-3017-2020, 2020.
Lawrence, P. J., Feddema, J. J., Bonan, G. B., Meehl, G. A., O'Neill, B. C., Oleson, K. W., Levis, S., Lawrence, D. M., Kluzek, E., Lindsay, K., and Thornton, P. E.: Simulating the Biogeochemical and Biogeophysical Impacts of Transient Land Cover Change and Wood Harvest in the Community Climate System Model (CCSM4) from 1850 to 2100, J. Climate, 25, 3071–3095, https://doi.org/10.1175/jcli-d-11-00256.1, 2012.
Longo, M., Knox, R. G., Levine, N. M., Alves, L. F., Bonal, D., Camargo, P. B., Fitzjarrald, D. R., Hayek, M. N., Restrepo-Coupe, N., Saleska, S. R., da Silva, R., Stark, S. C., Tapaj ìos, R. P., Wiedemann, K. T., Zhang, K., Wofsy, S. C., and Moorcroft, P. R.: Ecosystem heterogeneity and diversity mitigate Amazon forest resilience to frequent extreme droughts, New Phytol., 219, 914–931, https://doi.org/10.1111/nph, 2018.
Longo, M., Knox, R. G., Levine, N. M., Swann, A. L. S., Medvigy, D. M., Dietze, M. C., Kim, Y., Zhang, K., Bonal, D., Burban, B., Camargo, P. B., Hayek, M. N., Saleska, S. R., da Silva, R., Bras, R. L., Wofsy, S. C., and Moorcroft, P. R.: The biophysics, ecology, and biogeochemistry of functionally diverse, vertically and horizontally heterogeneous ecosystems: the Ecosystem Demography model, version 2.2 – Part 2: Model evaluation for tropical South America, Geosci. Model Dev., 12, 4347–4374, https://doi.org/10.5194/gmd-12-4347-2019, 2019.
Luyssaert, S., Schulze, E. D., Borner, A., Knohl, A., Hessenmoller, D., Law, B. E., Ciais, P., and Grace, J.: Old-growth forests as global carbon sinks, Nature, 455, 213–215, https://doi.org/10.1038/nature07276, 2008.
Macpherson, A. J., Carter, D. R., Schulze, M. D., Vidal, E., and Lentini, M. W.: The sustainability of timber production from Eastern Amazonian forests, Land Use Policy, 29, 339–350, https://doi.org/10.1016/j.landusepol.2011.07.004, 2012.
Martínez-Ramos, M., Ortiz-Rodríguez, I. A., Piñero, D., Dirzo, R., and Sarukhán, J.: Anthropogenic disturbances jeopardize biodiversity conservation within tropical rainforest reserves, P. Natl. Acad. Sci. USA, 113, 5323–5328, https://doi.org/10.1073/pnas.1602893113, 2016.
Massoud, E. C., Xu, C., Fisher, R. A., Knox, R. G., Walker, A. P., Serbin, S. P., Christoffersen, B. O., Holm, J. A., Kueppers, L. M., Ricciuto, D. M., Wei, L., Johnson, D. J., Chambers, J. Q., Koven, C. D., McDowell, N. G., and Vrugt, J. A.: Identification of key parameters controlling demographically structured vegetation dynamics in a land surface model: CLM4.5(FATES), Geosci. Model Dev., 12, 4133–4164, https://doi.org/10.5194/gmd-12-4133-2019, 2019.
Mazzei, L., Sist, P., Ruschel, A., Putz, F. E., Marco, P., Pena, W., and Ferreira, J. E. R.: Above-ground biomass dynamics after reduced-impact logging in the Eastern Amazon, Forest Ecol. Manag., 259, 367–373, https://doi.org/10.1016/j.foreco.2009.10.031, 2010.
Menton, M. C., Figueira, A. M. S., de Sousa, C. A. D., Miller, S. D., da Rocha, H. R., and Goulden, M. L.: LBA-ECO CD-04 Biomass Survey, km 83 Tower Site, Tapajos National Forest, Brazil. ORNL DAAC, Oak Ridge, Tennessee, USA, https://doi.org/10.3334/ORNLDAAC/990, 2011.
Miller, S. D., Goulden, M. L., Menton, M. C., da Rocha, H. R., de Freitas, H. C., Figueira, A. M. e. S., and Dias de Sousa, C. A.: BIOMETRIC AND MICROMETEOROLOGICAL MEASUREMENTS OF TROPICAL FOREST CARBON BALANCE, Ecol. Appl., 14, 114–126, https://doi.org/10.1890/02-6005, 2004.
Miller, S. D., Goulden, M. L., Hutyra, L. R., Keller, M., Saleska, S. R., Wofsy, S. C., Figueira, A. M. S., da Rocha, H. R., and de Camargo, P. B.: Reduced impact logging minimally alters tropical rainforest carbon and energy exchange, P. Natl. Acad. Sci. USA, 108, 19431–19435, https://doi.org/10.1073/pnas.1105068108, 2011.
Moorcroft, P. R., Hurtt, G. C., and Pacala, S. W.: A METHOD FOR SCALING VEGETATION DYNAMICS: THE ECOSYSTEM DEMOGRAPHY MODEL (ED), Ecol. Monogr., 71, 557–586, https://doi.org/10.1890/0012-9615(2001)071[0557:AMFSVD]2.0.CO;2, 2001.
Nepstad, D. C., Verssimo, A., Alencar, A., Nobre, C., Lima, E., Lefebvre, P., Schlesinger, P., Potter, C., Moutinho, P., Mendoza, E., Cochrane, M., and Brooks, V.: Large-scale impoverishment of Amazonian forests by logging and fire, Nature, 398, 505–508, 1999.
Oleson, K. W., Lawrence, D. M., Bonan, G. B., Drewniak, B., Huang, M., Koven, C. D., Levis, S., Li, F., Riley, W. J., Subin, Z. M., Swenson, S. C., Thornton, P. E., Bozbiyik, A., Fisher, R., Kluzek, E., Lamarque, J.-F., Lawrence, P. J., Leung, L. R., Lipscomb, W., Muszala, S., Ricciuto, D. M., Sacks, W., Sun, Y., Tang, J., and Yang, Z.-L.: Technical Description of version 4.5 of the Community Land Model (CLM), National Center for Atmospheric Research, Boulder, CONcar Technical Note NCAR/TN-503+STR, 2013.
Palace, M., Keller, M., and Silva, H.: NECROMASS PRODUCTION: STUDIES IN UNDISTURBED AND LOGGED AMAZON FORESTS, Ecol. Appl., 18, 873–884, https://doi.org/10.1890/06-2022.1, 2008.
Pan, Y., Birdsey, R. A., Fang, J., Houghton, R., Kauppi, P. E., Kurz, W. A., Phillips, O. L., Shvidenko, A., Lewis, S. L., Canadell, J. G., Ciais, P., Jackson, R. B., Pacala, S. W., McGuire, A. D., Piao, S., Rautiainen, A., Sitch, S., and Hayes, D.: A Large and Persistent Carbon Sink in the World's Forests, Science, 333, 988–993, 2011.
Pearson, T., Brown, S., and Casarim, F.: Carbon emissions from tropical forest degradation caused by logging, Environ. Res. Lett., 9, 034017, https://doi.org/10.1088/1748-9326/9/3/034017, 2014.
Pereira Jr., R., Zweede, J., Asner, G. P., and Keller, M.: Forest canopy damage and recovery in reduced-impact and conventional selective logging in eastern Para, Brazil, Forest Ecol. Manag., 168, 77–89, https://doi.org/10.1016/S0378-1127(01)00732-0, 2002.
Piponiot, C., Derroire, G., Descroix, L., Mazzei, L., Rutishauser, E., Sist, P., and Hérault, B.: Assessing timber volume recovery after disturbance in tropical forests – a new modelling framework, Ecol. Model., 384, 353–369, https://doi.org/10.1016/j.ecolmodel.2018.05.023, 2018.
Powell, T. L., Galbraith, D. R., Christoffersen, B. O., Harper, A., Imbuzeiro, H. M., Rowland, L., Almeida, S., Brando, P. M., da Costa, A. C. L., Costa, M. H., and Levine, N. M.: Confronting model predictions of carbon fluxes with measurements of Amazon forests subjected to experimental drought, New Phytol., 200, 350–365, 2013.
Putz, F. E., Sist, P., Fredericksen, T., and Dykstra, D.: Reduced-impact logging: Challenges and opportunities, Forest Ecol. Manag., 256, 1427–1433, https://doi.org/10.1016/j.foreco.2008.03.036, 2008.
Reich, P. B: The world‐wide `fast–slow' plant economics spectrum: a traits manifesto, J. Ecol., 102, 275–301, https://doi.org/10.1111/1365-2745.12211, 2014.
Rice, A. H., Pyle, E. H., Saleska, S. R., Hutyra, L., Palace, M., Keller, M., de Camargo, P. B., Portilho, K., Marques, D. F., and Wofsy, S. C.: CARBON BALANCE AND VEGETATION DYNAMICS IN AN OLD-GROWTH AMAZONIAN FOREST, Ecol. Appl., 14, 55–71, https://doi.org/10.1890/02-6006, 2004.
Saleska, S.: FLUXNET2015 BR-Sa1 Santarem-Km67-Primary Forest, Dataset, https://doi.org/10.18140/FLX/1440032, 2002–2011.
Saleska, S. R., Miller, S. D., Matross, D. M., Goulden, M. L., Wofsy, S. C., da Rocha, H. R., de Camargo, P. B., Crill, P., Daube, B. C., de Freitas, H. C., Hutyra, L., Keller, M., Kirchhoff, V., Menton, M., Munger, J. W., Pyle, E. H., Rice, A. H., and Silva, H.: Carbon in Amazon Forests: Unexpected Seasonal Fluxes and Disturbance-Induced Losses, Science, 302, 1554–1557, https://doi.org/10.1126/science.1091165, 2003.
Saleska, S. R., da Rocha, H. R., Huete, A. R., Nobre, A.D., Artaxo, P., and Shimabukuro, Y. E.: LBA-ECO CD-32 Flux Tower Network Data Compilation, Brazilian Amazon: 1999–2006, Oak Ridge National Laboratory Distributed Active Archive Center, Oak Ridge, Tennessee, USA, https://doi.org/10.3334/ORNLDAAC/1174, 2013.
Sato, H., Itoh, A., and Kohyama, T.: SEIB–DGVM: A new Dynamic Global Vegetation Model using a spatially explicit individual-based approach, Ecol. Model., 200, 279–307, https://doi.org/10.1016/j.ecolmodel.2006.09.006, 2007.
Shevliakova, E., Pacala, S. W., Malyshev, S., Hurtt, G. C., Milly, P. C. D., Caspersen, J. P., Sentman, L. T., Fisk, J. P., Wirth, C., and Crevoisier, C.: Carbon cycling under 300 years of land use change: Importance of the secondary vegetation sink, Global Biogeochem. Cy., 23, GB2022, https://doi.org/10.1029/2007GB003176, 2009.
Silver, W. L., Neff, J., McGroddy, M., Veldkamp, E., Keller, M., and Cosme, R.: Effects of Soil Texture on Belowground Carbon and Nutrient Storage in a Lowland Amazonian Forest Ecosystem, Ecosystems, 3, 193–209, https://doi.org/10.1007/s100210000019, 2000.
Sist, P., Rutishauser, E., Peña-Claros, M., Shenkin, A., Hérault, B., Blanc, L., Baraloto, C., Baya, F., Benedet, F., da Silva, K. E., Descroix, L., Ferreira, J. N., Gourlet-Fleury, S., Guedes, M. C., Bin Harun, I., Jalonen, R., Kanashiro, M., Krisnawati, H., Kshatriya, M., Lincoln, P., Mazzei, L., Medjibé, V., Nasi, R., d'Oliveira, M. V. N., de Oliveira, L. C., Picard, N., Pietsch, S., Pinard, M., Priyadi, H., Putz, F. E., Rodney, K., Rossi, V., Roopsind, A., Ruschel, A. R., Shari, N. H. Z., Rodrigues de Souza, C., Susanty, F. H., Sotta, E. D., Toledo, M., Vidal, E., West, T. A. P., Wortel, V., and Yamada, T.: The Tropical managed Forests Observatory: a research network addressing the future of tropical logged forests, Appl. Veg. Sci., 18, 171–174, https://doi.org/10.1111/avsc.12125, 2015.
Smith, B., Prentice, I. C., and Sykes, M. T.: Representation of vegetation dynamics in the modelling of terrestrial ecosystems: comparing two contrasting approaches within European climate space, Global Ecol. Biogeogr., 10, 621–637, https://doi.org/10.1046/j.1466-822X.2001.t01-1-00256.x, 2001.
Smith, B., Wårlind, D., Arneth, A., Hickler, T., Leadley, P., Siltberg, J., and Zaehle, S.: Implications of incorporating N cycling and N limitations on primary production in an individual-based dynamic vegetation model, Biogeosciences, 11, 2027–2054, https://doi.org/10.5194/bg-11-2027-2014, 2014.
Strigul, N., Pristinski, D., Purves, D., Dushoff, J., and Pacala, S.: SCALING FROM TREES TO FORESTS: TRACTABLE MACROSCOPIC EQUATIONS FOR FOREST DYNAMICS, Ecol. Monogr., 78, 523–545, https://doi.org/10.1890/08-0082.1, 2008.
Thonicke, K., Spessa, A., Prentice, I. C., Harrison, S. P., Dong, L., and Carmona-Moreno, C.: The influence of vegetation, fire spread and fire behaviour on biomass burning and trace gas emissions: results from a process-based model, Biogeosciences, 7, 1991–2011, https://doi.org/10.5194/bg-7-1991-2010, 2010.
Tomasella, J. and Hodnett, M. G.: Estimating soil water retention characteristics from limited data in Brazilian Amazonia, Soil Sci., 163, 190–202, 1998.
Trumbore, S. and Barbosa De Camargo, P.: Soil carbon dynamics, Amazonia and global change, American Geophysical Union, Washington DC, 451–462, 2009.
Watanabe, S., Hajima, T., Sudo, K., Nagashima, T., Takemura, T., Okajima, H., Nozawa, T., Kawase, H., Abe, M., Yokohata, T., Ise, T., Sato, H., Kato, E., Takata, K., Emori, S., and Kawamiya, M.: MIROC-ESM 2010: model description and basic results of CMIP5-20c3m experiments, Geosci. Model Dev., 4, 845–872, https://doi.org/10.5194/gmd-4-845-2011, 2011.
Weng, E. S., Malyshev, S., Lichstein, J. W., Farrior, C. E., Dybzinski, R., Zhang, T., Shevliakova, E., and Pacala, S. W.: Scaling from individual trees to forests in an Earth system modeling framework using a mathematically tractable model of height-structured competition, Biogeosciences, 12, 2655–2694, https://doi.org/10.5194/bg-12-2655-2015, 2015.
Whitmore, T. C.: An Introduction to Tropical Rain Forests, Oxford University Press, Inc., New York, USA, 1998.
Wilson, K., Goldstein, A., Falge, E., Aubinet, M., Baldocchi, D., Berbigier, P., Bernhofer, C., Ceulemans, R., Dolman, H., Field, C., Grelle, A., Ibrom, A., Law, B. E., Kowalski, A., Meyers, T., Moncrieff, J., Monson, R., Oechel, W., Tenhunen, J., Valentini, R., and Verma, S.: Energy balance closure at FLUXNET sites, Agr. Forest Meteorol., 113, 223–243, https://doi.org/10.1016/S0168-1923(02)00109-0, 2002.
Wright, I. J., Reich, P. B., Westoby, M., and Ackerly, D. D.: The worldwide leaf economics spectrum, Nature, 428, 821–827, https://doi.org/10.1038/nature02403, 2004.
Wu, J., Albert, L. P., Lopes, A. P., Restrepo-Coupe, N., Hayek, M., Wiedemann, K. T., Guan, K., Stark, S. C., Christoffersen, B., Prohaska, N., and Tavares, J. V.: Leaf development and demography explain photosynthetic seasonality in Amazon evergreen forests, Science, 351, 972–976, 2016.
Wu, J., Guan, K., Hayek, M., Restrepo-Coupe, N., Wiedemann, K. T., Xu, X., Wehr, R., Christoffersen, B. O., Miao, G., da Silva, R., de Araujo, A. C., Oliviera, R. C., Camargo, P. B., Monson, R. K., Huete, A. R., and Saleska, S. R.: Partitioning controls on Amazon forest photosynthesis between environmental and biotic factors at hourly to interannual timescales, Glob. Change Biol., 23, 1240–1257, https://doi.org/10.1111/gcb.13509, 2017.